This is a guest blog post by David A. Smith at Thermo Fisher. In their own words, “Thermo Fisher Scientific is the world leader in serving science. Our Mission is to enable our customers to make the world healthier, cleaner, and safer. Whether our customers are accelerating life sciences research, solving complex analytical challenges, improving patient diagnostics and therapies, or increasing productivity in their laboratories, we are here to support them”
Researchers in Life Sciences perform increasingly complex work in an industry that’s changing at an accelerated pace. With the recent focus on the COVID-19 pandemic, scientists around the world are under the microscope as they work to deliver a cure. At Thermo Fisher, our driving principle is to provide these researchers, and others like them, the tools and materials they need to study the world’s most pressing problems.
The specialized products we sell have always necessitated personalized customer experiences. We sell nearly every type of product related to scientific work, from everyday essentials like labware and chemical reagents to specialized instrumentation for genetic sequencing. Our goal is to let our customers know that they can get everything they need at Thermo Fisher. Traditionally, we approached this problem via dedicated commercial sales teams trained to handle specific products. In today’s world, customer data comes from many different touchpoints, which makes it increasingly difficult for our sales teams to understand which products their customers need to do their research.
Over the last three years, my team has maintained a custom portal for these sales teams where they can see data for every part of their customers’ journey. This fast-moving environment presents a unique opportunity for us to use data science to deliver personalized product recommendations that target the right products for the right customers at the right time.
In this post, we discuss how and why we decided to use Amazon Personalize and how that decision has empowered our team to deliver highly personalized, multi-channel content in an ever-developing ecosystem.
First-generation recommendations
Our team initially developed a rules-based recommendation system based on content curated by in-house scientists and run using SQL queries within our Amazon Redshift cluster.
We had this system in place for a year, and it worked well, but as our data volume grew, our team was spending more and more time maintaining the system. We felt that our current infrastructure wasn’t keeping up, and we wanted to migrate to a completely serverless infrastructure for improved scalability and fault tolerance. The following diagram illustrates our existing recommendations infrastructure.

Another risk we identified was that these recommendations relied on an internal content creation process to understand where products fit in the customer journey. Although this was a powerful tool, we struggled to provide high-quality recommendations for new or recently introduced products. This is a classic “cold-start” problem for recommender systems, and one of our requirements for any new system was that it could surface new items without additional maintenance.
Custom recommendations
Our team initially looked at third-party vendors to help improve our recommendations. However, we found that purchasing a solution would be costly to implement and force us to sacrifice some of the flexibility required to operate in a commercial organization. We quickly decided against buying an off the-shelf solution.
The consensus was that we would build a custom machine learning (ML)-based system from scratch. We explored a few different options, including hierarchical recurrent neural network (HRNN) models. Eventually, we settled on a factorization machine model as the best combination of performance, ease of implementation, and scalability.
Personalized recommendations
About 8 weeks later, we were wrapping up the initial phases of model development and validation. The new system was performing well. We had significantly improved our predictions, and we were getting good feedback from some sample recommendations we had sent out.
We were gearing up to productionize our new solution when our team learned about Amazon Personalize. It was immediately apparent to us that Amazon Personalize had the ideal balance of flexibility, scalability, and measurability we were looking for when we had evaluated off-the-shelf solutions 2 months prior.
We decided to run some initial tests with Amazon Personalize to see how it performed on real data and get a feel for how much effort would be required to implement it. It took 2 days to prepare the data, train a model, and begin generating high-quality recommendations.
Bringing the test together
As a team that had recently planned for 4–6 weeks spent deploying our custom model into production, this was very attractive. For me, the data scientist responsible for successfully designing, building, and evaluating a completely homegrown solution, it was less attractive. I was excited about finally deploying our custom solution, and I was proud of its performance. We eventually decided to put the two models head-to-head, with the winner determined by the best combination of model performance, scalability, and flexibility.
Like any proud parent, I immediately set out to prove the custom model was better. I designed 32 tests for each model, and, over the next week, I ran each test on over 100 different slices of data to see which performed better on a holdout dataset. The deeper and more expressive neural network models provided by Amazon Personalize did a better job of predicting user behavior over roughly 80% of the testing criteria.
If you’re a data scientist, this story might make you cringe, but it has a happy ending. Designing this testing process forced me to examine our data even more deeply and creatively than I had while building our custom recommendation system. I was able to rapidly test all the different hypotheses and use the results to develop a deep understanding of each model’s relative strengths and weaknesses related to the business problem we initially set out to solve.
Our team couldn’t have performed such a thorough analysis if we were also managing the infrastructure required for deep learning models. As a team, we had the choice to either spend 6–8 weeks deploying our custom model or 2 weeks implementing a recommender system using Amazon Personalize.
Serverless infrastructure
Scalability and fault tolerance were our main priorities when designing the infrastructure for our scientific product recommendations. We also wanted a system that would allow us to visually monitor progress and track errors.
We opted to use AWS Step Functions to build the backbone of our recommendations inference pipeline with customized AWS Lambda functions to pull data from our Amazon Redshift cluster, prepare the datasets for ingestion by Amazon Personalize, and trigger and monitor Amazon Personalize jobs. The following graph illustrates this inference pipeline.
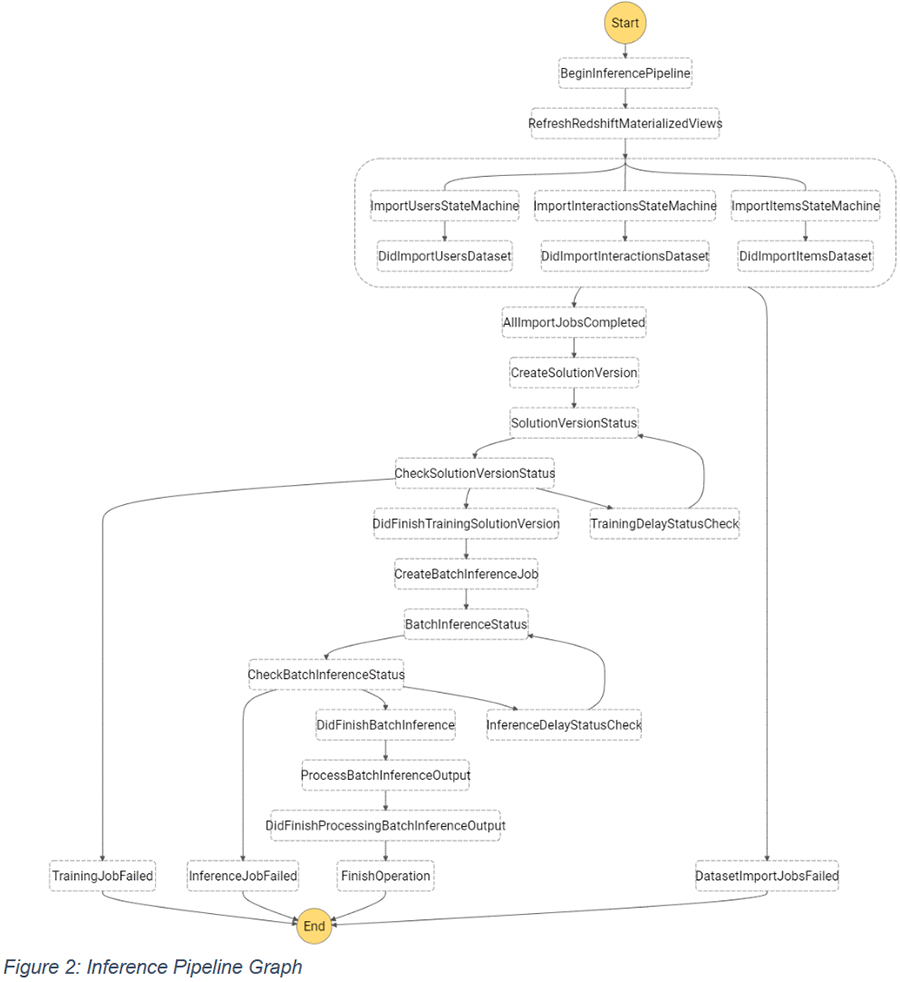
Flexibility in a changing world
Like many companies, our customers changed their habits significantly when the COVID-19 pandemic struck and businesses around the world shifted to work-from-home policies. There was a new demand to increase multi-channel targeting using email advertising campaigns.
Our team received a request to use the recommendation system we built with Amazon Personalize for targeted product email recommendations. Although we had never planned for this, it only took us a week to take our existing serverless inference pipeline and modify it to build, test, and validate an entirely new inference pipeline tuned specifically to email recommendations. Pivoting quickly is always challenging, but our commitment to building scalable and flexible infrastructure allowed us to overcome many of the challenges traditionally faced by teams when managing ML deployments and infrastructure. The following diagram illustrates the architecture of the email inference pipeline.
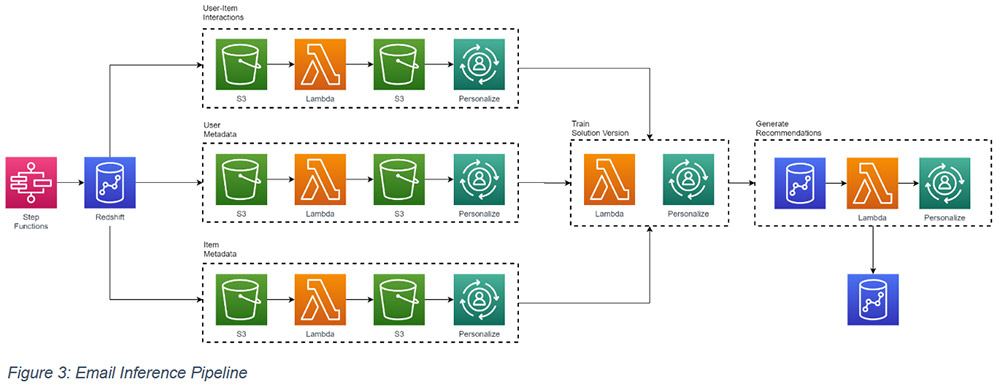
Despite the short turnaround time, the emails we’ve sent out following these recommendations have performed significantly better than previous baselines.
Looking back, it’s clear to me that we would have had significantly more difficulty meeting this request if we had opted to deploy our custom factorization machine model instead of using Amazon Personalize.
Conclusion
Thermo Fisher is constantly striving to help scientists around the world solve some of our greatest challenges. With Amazon Personalize, we’ve dramatically improved our ability to understand the work our customers do and serve them personalized experiences via multiple channels. Using Amazon Personalize has allowed us to focus on solving difficult problems instead of managing ML infrastructure.
About the Author
David A. Smith is a data scientist for Thermo Fisher Scientific based out of Carlsbad, California. He works with cross-organizational teams to design, build, and deploy automated models to drive customer intelligence and create business value. His interests include NLP, serverless ML, and blockchain technology. Outside of work, you can find David rock climbing, playing tennis, or swimming with his dog.
Read More