Creative workflows are riddled with hurry up and wait.
GeForce RTX 30 Series GPUs, powered by our second-generation RTX architecture, aim to reduce the wait, giving creators more time to focus on what matters: creating amazing content.
These new graphics cards deliver faster ray tracing and the next generation of AI-powered tools, turning the tedious tasks in creative workflows into things of the past.
With up to 24GB of new, blazing-fast GDDR6X memory, they’re capable of powering the most demanding multi-app workflows, 8K HDR video editing and working with extra-large 3D models.
Plus, two new apps, available to all NVIDIA RTX users, are joining NVIDIA Studio. NVIDIA Broadcast turns any room into a home broadcast studio with AI-enhanced video and voice comms. NVIDIA Omniverse Machinima enables creators to tell amazing stories with video game assets, animated by AI.
Ray Tracing at the Speed of Light
The next generation of dedicated ray tracing cores and improved CUDA performance on GeForce RTX 30 Series GPUs speeds up 3D rendering times by up to 2x across top renderers.
The RT Cores also feature new hardware acceleration for ray-traced motion blur rendering, a common but computationally intensive technique. It’s used to enhance 3D visuals with cinematic flair. But to date, it requires using either an inaccurate motion vector-based post-process, or an accurate but time-consuming rendering step. Now with RTX 30 Series and RT Core accelerated apps like Blender Cycles, creators can enjoy up to 5x faster motion blur rendering than prior generation RTX.
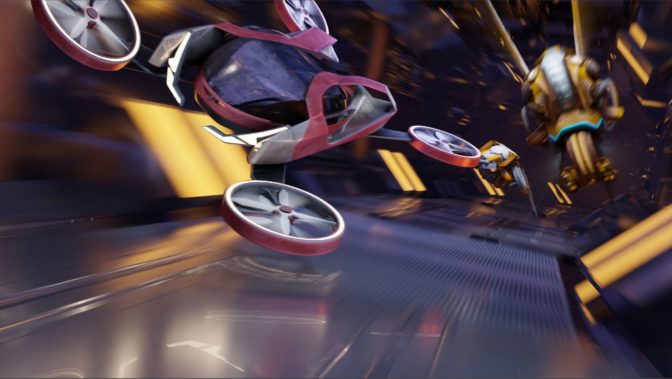
Next-Gen AI Means Less Wait and More Create
GeForce RTX 30 Series GPUs are enabling the next wave of AI-powered creative features, reducing or even eliminating repetitive creative tasks such as image denoising, reframing and retiming of video, and creation of textures and materials.
Along with the release of our next-generation RTX GPUs, NVIDIA is bringing DLSS — real-time super resolution that uses the power of AI to boost frame rates — to creative apps. D5 Render and SheenCity Mars are the first design apps to add DLSS support, enabling crisp, real-time exploration of designs.
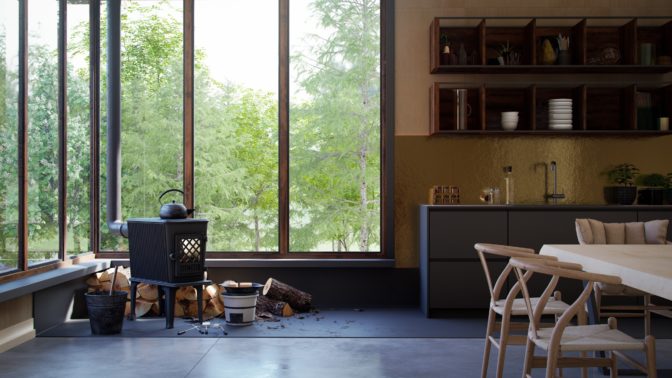
Hardware That Zooms
Increasingly, complex digital content creation requires hardware that can run multiple apps concurrently. This requires a large frame buffer on the GPU. Without sufficient memory, systems start to chug, wasting precious time as they swap geometry and textures in and out of each app.
The new GeForce RTX 3090 GPU houses a massive 24GB of video memory. This lets animators and 3D artists work with the largest 3D models. Video editors can tackle the toughest 8K scenes. And creators of all types can stay hyper-productive in multi-app workflows.
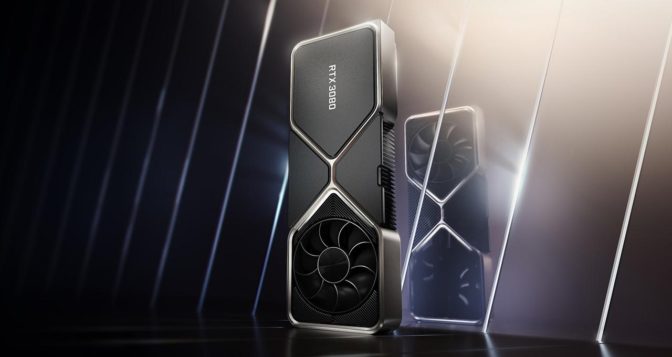
The new GPUs also use PCIe 4.0, doubling the connection speed between the GPU and the rest of the PC. This improves performance when working with ultra-high-resolution and HDR video.
GeForce RTX 30 Series graphics cards are also the first discrete GPUs with decode support for the AV1 codec, enabling playback of high-resolution video streams up to 8K HDR using significantly less bandwidth.
AI-Accelerated Studio Apps
Two new Studio apps are making their way into creatives’ arsenals this fall. Best of all, they’re free for NVIDIA RTX users.
NVIDIA Broadcast upgrades any room into an AI-powered home broadcast studio. It transforms standard webcams and microphones into smart devices, offering audio noise removal, virtual background effects and webcam auto framing compatible with most popular live streaming, video conferencing and voice chat applications.
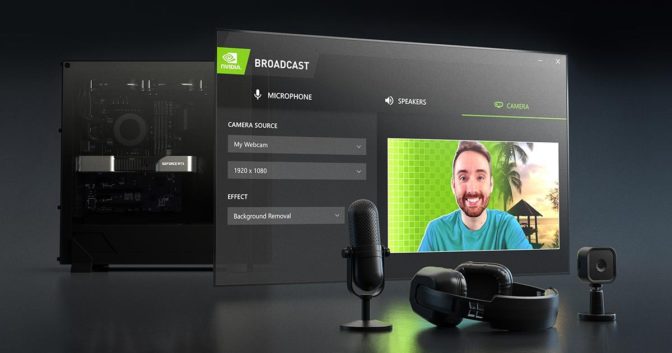
NVIDIA Omniverse Machinima enables creators to tell amazing stories with video game assets, animated by NVIDIA AI technologies. Through NVIDIA Omniverse, creators can import assets from supported games or most third-party asset libraries, then automatically animate characters using an AI-based pose estimator and footage from their webcam. Characters’ faces can come to life with only a voice recording using NVIDIA’s new Audio2Face technology.
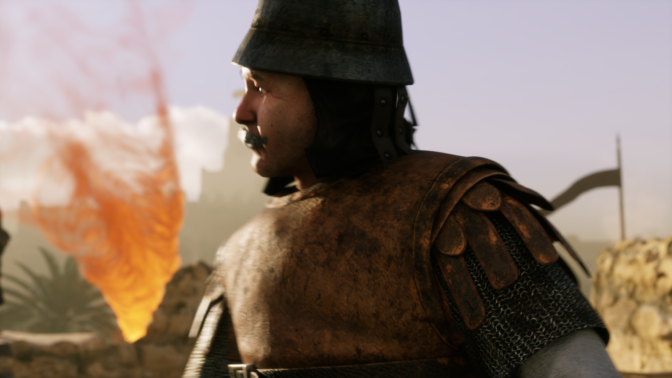
NVIDIA is also updating in September GeForce Experience, our companion app for GeForce GPUs, to support desktop and application capture for up to 8K and HDR, enabling creators to record video at incredibly high resolution and dynamic range.
These apps, like most of the world’s top creative apps, are supported by NVIDIA Studio Drivers, which provide optimal levels of performance and reliability.
GeForce RTX 30 Series: Get Creating Soon
GeForce RTX 30 Series graphics cards are available starting September 17.
While you wait for the next generation of creative performance, perfect your creative skillset by visiting the NVIDIA Studio YouTube channel to watch tutorials and tips and tricks from industry-leading artists.
The post Up Your Creative Game: GeForce RTX 30 Series GPUs Amp Up Performance appeared first on The Official NVIDIA Blog.