Amazon Rekognition content moderation is a deep learning-based service that can detect inappropriate, unwanted, or offensive images and videos, making it easier to find and remove such content at scale. Amazon Rekognition provides a detailed taxonomy of moderation categories, such as Explicit Nudity, Suggestive, Violence, and Visually Disturbing.
You can now detect six new categories: Drugs, Tobacco, Alcohol, Gambling, Rude Gestures, and Hate Symbols. In addition, you get improved detection rates for already supported categories.
In this post, we learn about the details of the content moderation service, how to use the APIs, and how you can perform comprehensive moderation using AWS machine learning (ML) services. Lastly, we see how customers in social media, broadcast media, advertising, and ecommerce create better user experiences, provide brand safety assurances to advertisers, and comply with local and global regulations.
Challenges with content moderation
The daily volume of user-generated content (UGC) and third-party content has been increasing substantially in industries like social media, ecommerce, online advertising, and photo sharing. You may want to review this content to ensure that your end-users aren’t exposed to potentially inappropriate or offensive material, such as nudity, violence, drug use, adult products, or disturbing images. In addition, broadcast and video-on-demand (VOD) media companies may be required to ensure that the content they create or license carries appropriate ratings as per compliance guidelines for various geographies or target audiences.
Many companies employ teams of human moderators to review content, while others simply react to user complaints to take down offensive images, ads, or videos. However, human moderators alone can’t scale to meet these needs at sufficient quality or speed, which leads to poor user experience, prohibitive costs to achieve scale, or even loss of brand reputation.
Amazon Rekognition content moderation enables you to streamline or automate your image and video moderation workflows using ML. You can use fully managed image and video moderation APIs to proactively detect inappropriate, unwanted, or offensive content containing nudity, suggestiveness, violence, and other such categories. Amazon Rekognition returns a hierarchical taxonomy of moderation-related labels that make it easy to define granular business rules as per your own standards and practices, user safety, or compliance guidelines—without requiring any ML experience. You can then use machine predictions to automate certain moderation tasks completely or significantly reduce the review workload of trained human moderators, so they can focus on higher-value work.
In addition, Amazon Rekognition allows you to quickly review millions of images or thousands of videos using ML, and flag only a small subset of assets for further action. This makes sure that you get comprehensive but cost-effective moderation coverage for all your content as your business scales, and your moderators can reduce the burden of looking at large volumes of disturbing content.
Granular moderation using a hierarchical taxonomy
Different use cases need different business rules for content review. For example, you may want to just flag content with blood, or detect violence with weapons in addition to blood. Content moderation solutions that only provide broad categorizations like violence don’t provide you with enough information to create granular rules. To address this, Amazon Rekognition designed a hierarchical taxonomy with 4 top-level moderation categories (Explicit Nudity, Suggestive, Violence, and Visually Disturbing) and 18 subcategories, which allow you to build nuanced rules for different scenarios.
We have now added 6 new top-level categories (Drugs, Hate Symbols, Tobacco, Alcohol, Gambling, and Rude Gestures), and 17 new subcategories to provide enhanced coverage for a variety of use cases in domains such as social media, photo sharing, broadcast media, gaming, marketing, and ecommerce. The full taxonomy is provided in the following table.
Top-level Category |
Second-level Category |
Explicit Nudity |
Nudity |
Graphic Male Nudity |
Graphic Female Nudity |
Sexual Activity |
Illustrated Explicit Nudity |
Adult Toys |
Suggestive |
Female Swimwear Or Underwear |
Male Swimwear Or Underwear |
Partial Nudity |
Barechested Male |
Revealing Clothes |
Sexual Situations |
Violence |
Graphic Violence Or Gore |
Physical Violence |
Weapon Violence |
Weapons |
Self Injury |
Visually Disturbing |
Emaciated Bodies |
Corpses |
Hanging |
Air Crash |
Explosions and Blasts |
Rude Gestures |
Middle Finger |
Drugs |
Drug Products |
Drug Use |
Pills |
Drug Paraphernalia |
Tobacco |
Tobacco Products |
Smoking |
Alcohol |
Drinking |
Alcoholic Beverages |
Gambling |
Gambling |
Hate Symbols |
Nazi Party |
White Supremacy |
Extremist |
How it works
For analyzing images, you can use the DetectModerationLabels API to pass in the Amazon Simple Storage Service (Amazon S3) location of your stored images, or even use raw image bytes in the request itself. You can also specify a minimum prediction confidence. Amazon Rekognition automatically filters out results that have confidence scores below this threshold.
The following code is an image request:
{
"Image": {
"S3Object": {
"Bucket": "bucket",
"Name": "input.jpg"
}
},
"MinConfidence": 60
}
You get back a JSON response with detected labels, the prediction confidence, and information about the taxonomy in the form of a ParentName field:
{
"ModerationLabels": [
{
"Confidence": 99.24723052978516,
"ParentName": "",
"Name": "Explicit Nudity"
},
{
"Confidence": 99.24723052978516,
"ParentName": "Explicit Nudity",
"Name": "Sexual Activity"
},
]
}
For more information and a code sample, see Content Moderation documentation. To experiment with your own images, you can use the Amazon Rekognition console.
In the following screenshot, one of our new categories (Smoking) was detected (image sourced from Pexels.com).
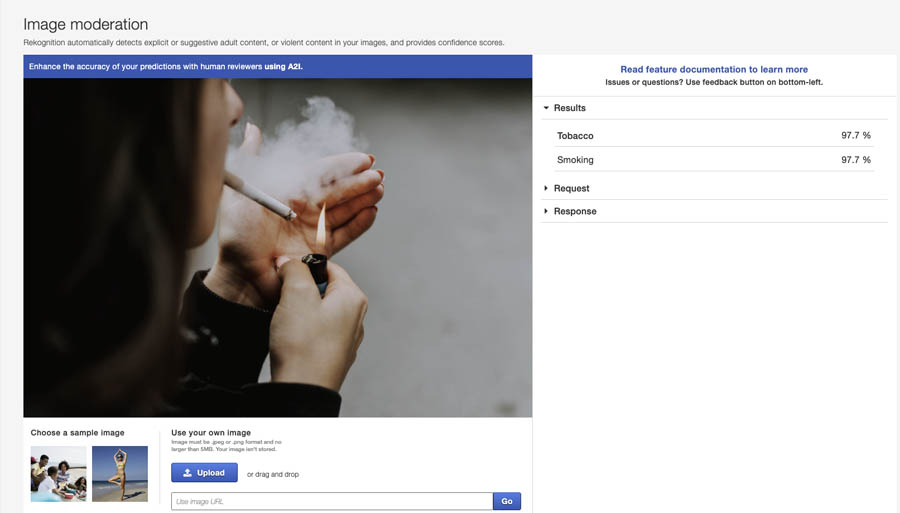
For analyzing videos, Amazon Rekognition provides a set of asynchronous APIs. To start detecting moderation categories on your video that is stored in Amazon S3, you can call StartContentModeration. Amazon Rekognition publishes the completion status of the video analysis to an Amazon Simple Notification Service (Amazon SNS) topic. If the video analysis is successful, you call GetContentModeration to get the analysis results. For more information about starting video analysis and getting the results, see Calling Amazon Rekognition Video Operations. For each detected moderation label, you also get its timestamp. For more information and a code sample, see Detecting Inappropriate Stored Videos.
For nuanced situations or scenarios where Amazon Rekognition returns low-confidence predictions, content moderation workflows still require human reviewers to audit results and make final judgements. You can use Amazon Augmented AI (Amazon A2I) to easily implement a human review and improve the confidence of predictions. Amazon A2I is directly integrated with Amazon Rekognition moderation APIs. Amazon A2I allows you to use in-house, private, or even third-party vendor workforces with a user-defined web interface that has instructions and tools to carry out review tasks. For more information about using Amazon A2I with Amazon Rekognition, see Build alerting and human review for images using Amazon Rekognition and Amazon A2I.
Audio, text, and customized moderation
You can use Amazon Rekognition text detection for images and videos to read text, and then check it against your own list of prohibited words or phrases. To detect profanities or hate speech in videos, you can use Amazon Transcribe to convert speech to text, and then check it against a similar list. If you want to further analyze text using natural language processing (NLP), you can use Amazon Comprehend.
If you have very specific or fast-changing moderation needs and access to your own training data, Amazon Rekognition offers Custom Labels to easily train and deploy your own moderation models with a few clicks or API calls. For example, if your ecommerce platform needs to take action on a new product carrying an offensive or politically sensitive message, or your broadcast network needs to detect and blur the logo of a specific brand for legal reasons, you can quickly create and operationalize new models with custom labels to address these scenarios.
Use cases
In this section, we discuss three potential use cases for expanded content moderation labels, depending on your industry.
Social media and photo-sharing platforms
Social media and photo-sharing platforms work with very large amounts of user-generated photos and videos daily. To make sure that uploaded content doesn’t violate community guidelines and societal standards, you can use Amazon Rekognition to flag and remove such content at scale even with small teams of human moderators. Detailed moderation labels also allow for creating a more granular set of user filters. For example, you might find images containing drinking or alcoholic beverages to be acceptable in a liquor ad, but want to avoid ones showing drug products and drug use under any circumstances.
Broadcast and VOD media companies
As a broadcast or VOD media company, you may have to ensure that you comply with the regulations of the markets and geographies in which you operate. For example, content that shows smoking needs to carry an onscreen health advisory warning in countries like India. Furthermore, brands and advertisers want to prevent unsuitable associations when placing their ads in a video. For example, a toy brand for children may not want their ad to appear next to content showing consumption of alcoholic beverages. Media companies can now use the comprehensive set of categories available in Amazon Rekognition to flag the portions of a movie or TV show that require further action from editors or ad traffic teams. This saves valuable time, improves brand safety for advertisers, and helps prevent costly compliance fines from regulators.
Ecommerce and online classified platforms
Ecommerce and online classified platforms that allow third-party or user product listings want to promptly detect and delist illegal, offensive, or controversial products such as items displaying hate symbols, adult products, or weapons. The new moderation categories in Amazon Rekognition help streamline this process significantly by flagging potentially problematic listings for further review or action.
Customer stories
We now look at some examples of how customers are deriving value from using Amazon Rekognition content moderation:
SmugMug operates two very large online photo platforms, SmugMug and Flickr, enabling more than 100M members to safely store, search, share, and sell tens of billions of photos. Flickr is the world’s largest photographer-focused community, empowering photographers around the world to find their inspiration, connect with each other, and share their passion with the world.
“As a large, global platform, unwanted content is extremely risky to the health of our community and can alienate photographers. We use Amazon Rekognition’s content moderation feature to find and properly flag unwanted content, enabling a safe and welcoming experience for our community. At Flickr’s huge scale, doing this without Amazon Rekognition is nearly impossible. Now, thanks to content moderation with Amazon Rekognition, our platform can automatically discover and highlight amazing photography that more closely matches our members’ expectations, enabling our mission to inspire, connect, and share.”
– Don MacAskill, Co-founder, CEO & Chief Geek
Mobisocial is a leading mobile software company, focused on building social networking and gaming apps. The company develops Omlet Arcade, a global community where tens of millions of mobile gaming live-streamers and esports players gather to share gameplay and meet new friends.
“To ensure that our gaming community is a safe environment to socialize and share entertaining content, we used machine learning to identify content that doesn’t comply with our community standards. We created a workflow, leveraging Amazon Rekognition, to flag uploaded image and video content that contains non-compliant content. Amazon Rekognition’s content moderation API helps us achieve the accuracy and scale to manage a community of millions of gaming creators worldwide. Since implementing Amazon Rekognition, we’ve reduced the amount of content manually reviewed by our operations team by 95%, while freeing up engineering resources to focus on our core business. We’re looking forward to the latest Rekognition content moderation model update, which will improve accuracy and add new classes for moderation.”
-Zehong, Senior Architect at Mobisocial
Conclusion
In this post, we learned about the six new categories of inappropriate or offensive content now available in the Amazon Rekognition hierarchical taxonomy for content moderation, which contains 10 top-level categories and 35 subcategories overall. We also saw how Amazon Rekognition moderation APIs work, and how customers in different domains are using them to streamline their review workflows.
For more information about the latest version of content moderation APIs, see Content Moderation. You can also try out your own images on the Amazon Rekognition console. If you want to test visual and audio moderation with your own videos, check out the Media Insights Engine (MIE)—a serverless framework to easily generate insights and develop applications for your video, audio, text, and image resources, using AWS ML and media services. You can easily spin up your own MIE instance using the provided AWS CloudFormation template, and then use the sample application.
About the Author
Venkatesh Bagaria is a Principal Product Manager for Amazon Rekognition. He focuses on building powerful but easy-to-use deep learning-based image and video analysis services for AWS customers. In his spare time, you’ll find him watching way too many stand-up comedy specials and movies, cooking spicy Indian food, and pretending that he can play the guitar.
Read More