According to the 2018 National Highway Traffic Safety Administration (NHTSA) Traffic Safety Facts, in 2018, there were 857 fatal bicycle and motor vehicle crashes and an additional estimated 47,000 cycling injuries in the US .
While motorists often accuse cyclists of being the cause of bike-car accidents, the analysis shows that this is not the case. The most common type of crash involved a motorist entering an intersection controlled by a stop sign or red light and either failing to stop properly or proceeding before it was safe to do so. The second most common crash type involved a motorist overtaking a cyclist unsafely. In fact, cyclists are the cause of less than 10% of bike-car accidents. For more information, see Pedestrian and Bicycle Crash Types.
Many city cyclists are on the lookout for new ways to make cycling safer. In this post, you learn how to create a Smartcycle using two AWS DeepLens devices—one mounted on the front of your bicycle, the other mounted on the rear of the bicycle—to detect road hazards. You can visually highlight these hazards and play audio alerts corresponding to the road hazards detected. You can also track wireless sensor data about the ride, display metrics, and send that sensor data to the AWS Cloud using AWS IoT for reporting purposes.
This post discusses how the Smartcycle project turns an ordinary bicycle into an integrated platform capable of transforming raw sensor and video data into valuable insights by using AWS DeepLens, the Amazon SageMaker built-in object detection algorithm, and AWS Cloud technologies. This solution demonstrates the possibilities that machine learning solutions can bring to improve cycling safety and the overall ride experience for cyclists.
By the end of this post, you should have enough information to successfully deploy the hardware and software required to create your own Smartcycle implementation. The full instructions are available on the GitHub repo.
Smartcycle and AWS
AWS DeepLens is a deep learning-enabled video camera designed for developers to learn machine learning in a fun, hands-on way. You can order your own AWS DeepLens on Amazon.com (US), Amazon.ca (Canada), Amazon.co.jp (Japan), Amazon.de (Germany), Amazon.fr (France), Amazon.es (Spain), Amazon.it (Italy).
A Smartcycle has AWS DeepLens devices mounted on the front and back of the bike, which provide edge compute and inference capabilities, and wireless sensors mounted on the bike or worn by the cyclist to capture performance data that is sent back to the AWS Cloud for analysis.
The following image is of the full Smartcycle bike setup.
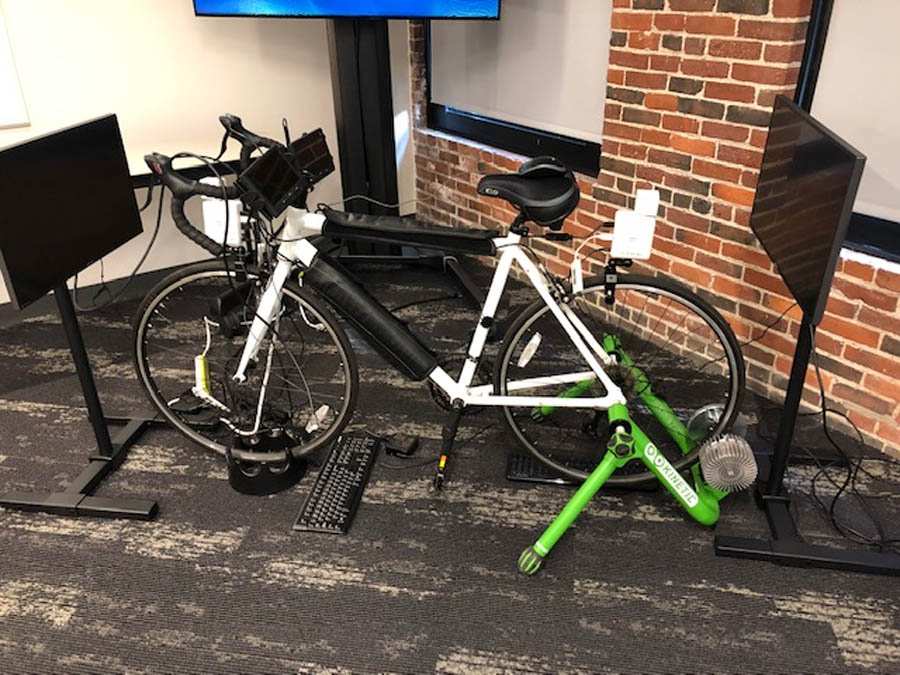
The following image is an example of AWS DeepLens rendered output from the demo video.
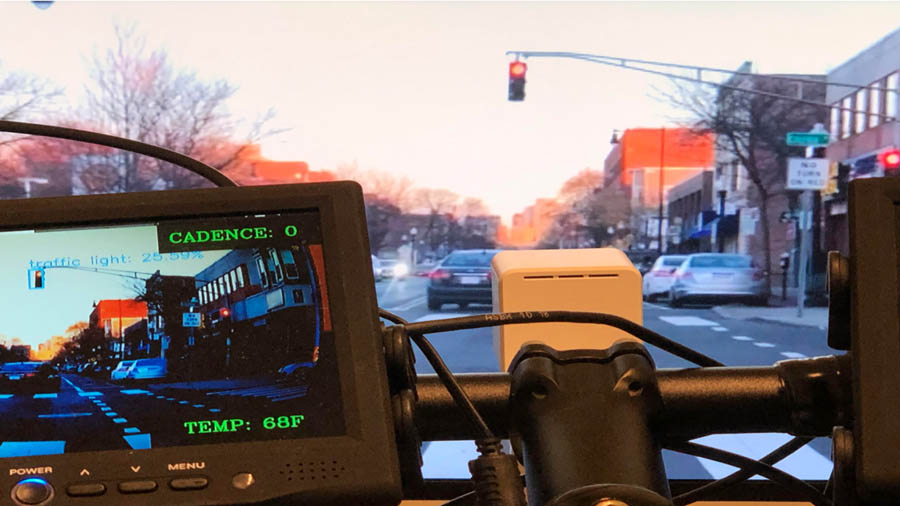
AWS IoT Greengrass seamlessly extends AWS to edge devices so they can act locally on the data they generate, while still using the AWS Cloud for management, analytics, and durable storage. With AWS IoT Greengrass, connected devices can run AWS Lambda functions, run predictions based on machine learning (ML) models, keep device data in sync, and communicate with other devices securely—even when not connected to the internet.
Amazon SageMaker is a fully managed ML service. With Amazon SageMaker, you can quickly and easily build and train ML models and directly deploy them into a production-ready hosted environment. Amazon SageMaker provides an integrated Jupyter notebook authoring environment for you to perform initial data exploration, analysis, and model building.
Amazon DynamoDB is a key-value and document database that delivers single-digit millisecond performance at any scale. It’s a fully managed, multi-Region, multi-master database with built-in security, backup and restore, and in-memory caching for internet-scale applications. Amazon DynamoDB is suitable for easily storing and querying the Smartcycle sensor data.
Solution overview
The following diagram illustrates the high-level architecture of the Smartcycle.
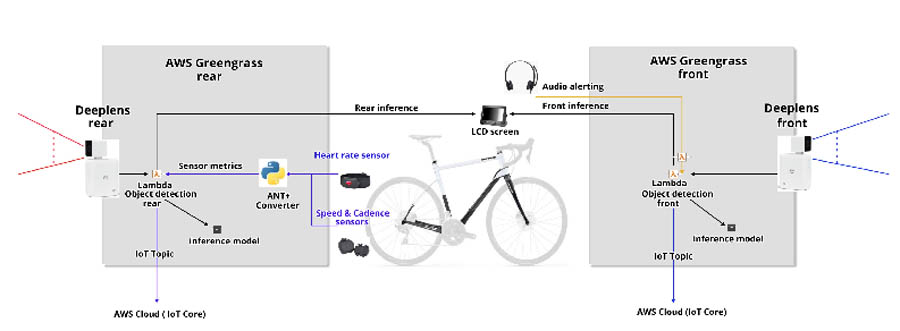
The architecture contains the following elements:
- Two AWS DeepLens devices provide the compute, video cameras, and GPU-backed inference capabilities for the Smartcycle project, as well as a Linux-based operating system environment to work in.
- A Python-based Lambda function (
greengrassObjectDetector.py
), running in the AWS IoT Greengrass container on each AWS DeepLens, takes the video stream input data from the built-in camera, splits the video into individual image frames, and references the custom object detection model artifact to perform the inference required to identify hazards using the doInference()
function.
- The
doInference()
function returns a probability score for each class of hazard object detected in an image frame; the object detection model is optimized for the GPU built into the AWS DeepLens device and the inference object detection happens locally.
- The
greengrassObjectDetector.py
uses the object detection inference data to draw a graphical bounding box around each hazard detected and displays it back to the cyclist in the processed output video stream.
- The Smartcycle has small LCD screens attached to display the processed video output.
The greengrassObjectDetector.py
Lambda function running on both front and rear AWS DeepLens devices sends messages containing information about the detected hazards to the AWS IoT GreenGrass topic. Another Lambda function, called audio-service.py
, subscribes to that IoT topic and plays an MP3 audio message for the type of object hazard detected (the MP3 files were created in advance using Amazon Polly). The audio-service.py
function plays audio alerts for both front and rear AWS DeepLens devices (because both devices publish to a common IoT topic). Because of this, the audio-service.py
function is usually run on the front-facing AWS DeepLens device only, which is plugged into a speaker or pair of headphones for audio output.
The Lambda functions and Python scripts running on the AWS DeepLens devices use a local Python database module called DiskCache to persist data and state information tracked by the Smartcycle. A Python script called multi_ant_demo.py
runs on the front AWS DeepLens device from a terminal shell; this script listens for specific ANT+ wireless sensors (such as heart rate monitor, temperature, and speed) using a USB ANT+ receiver plugged into the AWS DeepLens. It processes and stores sensor metrics in the local DiskCache database using a unique key for each type of ANT+ sensor tracked. The greengrassObjectDetector.py
function reads the sensor records from the local DiskCache database and renders that information as labels in the processed video stream (alongside the previously noted object detection bounding boxes).
With respect to sensor analytics, the greengrassObjectDetector.py
function exchanges MQTT messages containing sensor data with AWS IoT Core. An AWS IoT rule created in AWS IoT Core inserts messages sent to the topic into the Amazon DynamoDB table. Amazon DynamoDB provides a persistence layer where data can be accessed using RESTful APIs. The solution uses a static webpage hosted on Amazon Simple Storage Service (Amazon S3) to aggregate sensor data for reporting. Javascript executed in your web browser sends and receives data from a public backend API built using Lambda and Amazon API Gateway. You can also use Amazon QuickSight to visualize hot data directly from Amazon S3.
Hazard object detection model
The Smartcycle project uses a deep learning object detection model built and trained using Amazon SageMaker to detect the following objects from two AWS DeepLens devices:
- Front device – Stop signs, traffic lights, pedestrians, other bicycles, motorbikes, dogs, and construction sites
- Rear device – Approaching pedestrians, cars, and heavy vehicles such as buses and trucks
The Object Detection AWS DeepLens Project serves as the basis for this solution, which is modified to work with the hazard detection model and sensor data.
The Deep Learning Process for this solution includes the following:
- Business understanding
- Data understanding
- Data preparation
- Training the model
- Evaluation
- Model deployment
- Monitoring
The following diagram illustrates the model development process.
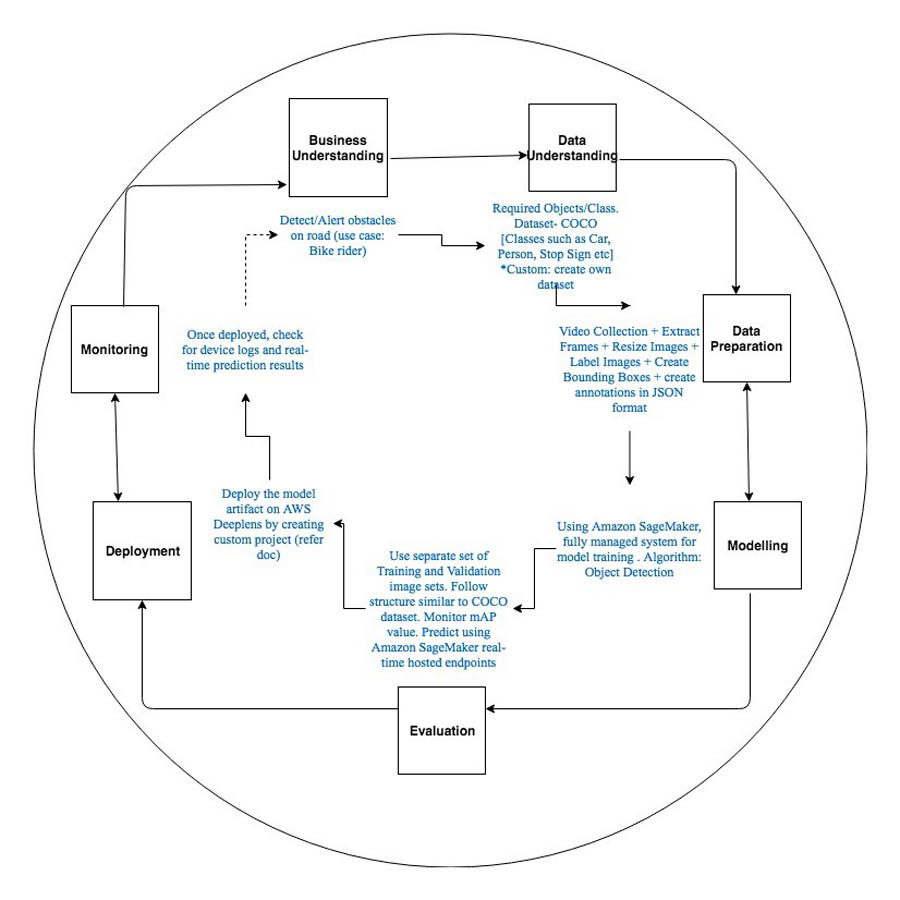
Business Understanding
You use object detection to identify road hazards. You can localize objects such as stop signs, traffic lights, pedestrians, other bicycles, motorbikes, dogs, and more.
Understanding the Training Dataset
Object detection is the process of identifying and localizing objects in an image. The object detection algorithm takes image classification further by rendering a bounding box around the detected object in an image, while also identifying the type of object detected. Smartcycle uses the built-in Amazon SageMaker object detection algorithm to train the object detection model.
This solution uses the Microsoft Common Objects in Context (COCO) dataset. It’s a large-scale dataset for multiple computer vision tasks, including object detection, segmentation, and captioning. The training dataset train2017.zip includes 118,000 images (approximately 18 GB), and the validation dataset val2017.zip includes 5,000 images (approximately 1 GB).
To demonstrate the deep learning step using Amazon SageMaker, this post references the val2017.zip dataset for training. However, with adequate infrastructure and time, you can also use the train2017.zip dataset and follow the same steps. If needed, you can also build and/or enhance on a custom dataset followed by data augmentation techniques or create a new class, such as construction
or potholes
, by collecting sufficient number of images representing that class. You can use Amazon SageMaker Ground Truth to provide the data annotation. Amazon SageMaker Ground Truth is a fully managed data labeling service that makes it easy to build highly accurate training datasets for machine learning. You can also label these images using image annotation tools such as RectLabel, preferably in PASCAL VOC format.
Here are some examples from Microsoft COCO: Common Objects in Context Study to help illustrate what object detection entails.
The following image is an example of object localization; there are bounding boxes over three different image classes.
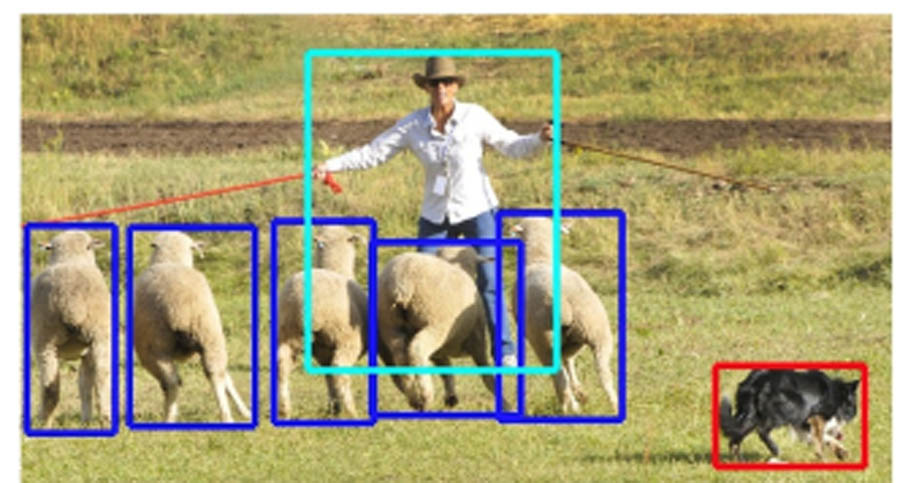
The following image is an example of prediction results for a single detected object.
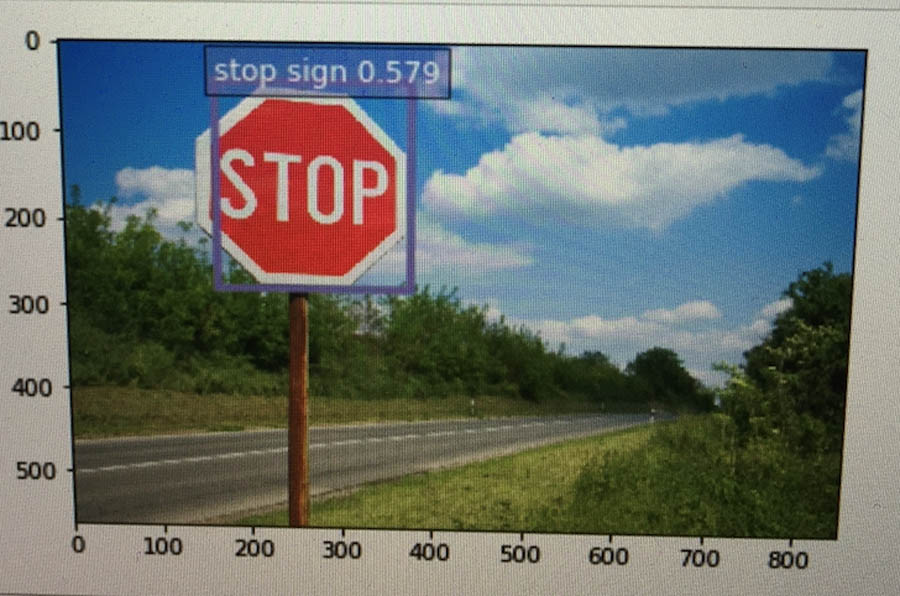
The following image is an example of prediction results for multiple objects.
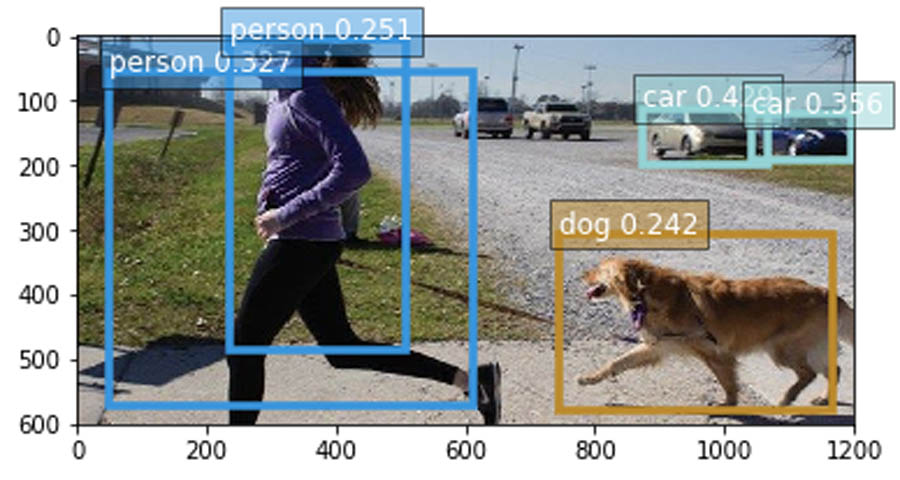
Data Preparation
The sample notebook provides instructions on downloading the dataset (via the wget
utility), followed by data preparation and training an object detection model using the Single Shot mlutibox Detector (SSD) algorithm.
Data preparation includes annotating each image within the training dataset, followed by a mapper job that can index the class from 0. The Amazon SageMaker object detection algorithm expects labels to be indexed from 0. You can use the fix_index_mapping
function for this purpose. To avoid errors while training, you should also eliminate the images with no annotation files.
For validation purposes, you can split this dataset and create separate training and validation datasets. Use the following code:
train_jsons = jsons[:4452]
val_jsons = jsons[4452:]
Training the Model
After you prepare the data, you need to host your dataset on Amazon S3. The built-in algorithm can read and write the dataset using multiple channels (for this use case, four channels). Channels are simply directories in the bucket that differentiate between training and validation data.
The following screenshot shows the Amazon S3 folder structure . It contains folders to hold the data and annotation files (the output
folder stores the model artifacts).
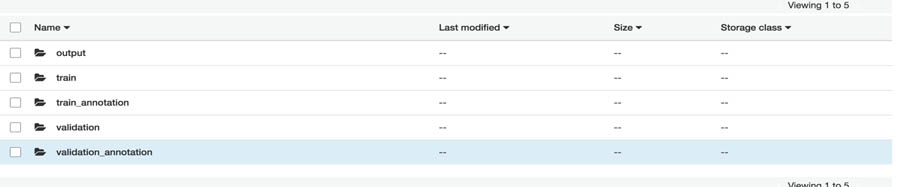
When the data is available, you can train the object detector. The sageMaker.estimator.Estimator
object can launch the training job for you. Use the following code:
od_model = sagemaker.estimator.Estimator(training_image,
role, train_instance_count=1, train_instance_type='ml.p3.16xlarge',
train_volume_size = 50,
train_max_run = 360000,
input_mode = 'File',
output_path=s3_output_location,
sagemaker_session=sess)
The Amazon SageMaker object detection algorithm requires you to train models on a GPU instance type such as ml.p3.2xlarge
, ml.p3.8xlarge
, or ml.p3.16xlarge
.
The algorithm currently supports VGG-16 and ResNet-50 base neural nets. It also has multiple options for hyperparameters, such as base_network
, learning_rate
, epochs
, lr_scheduler_step
, lr_scheduler_factor
, and num_training_samples
, which help to configure the training job. The next step is to set up these hyperparameters and data channels to kick off the model training job. Use the following code:
od_model.set_hyperparameters(base_network=
'resnet-50',use_pretrained_model=1,
num_classes=80, mini_batch_size=16,
epochs=200, learning_rate=0.001,
lr_scheduler_step='10',
lr_scheduler_factor=0.1,
optimizer='sgd', momentum=0.9,
weight_decay=0.0005,
overlap_threshold=0.5,
nms_threshold=0.45,
image_shape=300, label_width=372,
num_training_samples=4452)
You can now create the sagemaker.session.s3_input
objects from your data channels mentioned earlier, with content_type as image/jpeg for the image channels and the annotation channels. Use the following code:
train_data = sagemaker.session.s3_input(
s3_train_data, distribution='FullyReplicated',
content_type='image/jpeg', s3_data_type='S3Prefix')
validation_data = sagemaker.session.s3_input(
s3_train_data, distribution='FullyReplicated',
content_type='image/jpeg', s3_data_type='S3Prefix')
train_annotation =sagemaker.session.s3_input(
s3_train_annotation, distribution='FullyReplicated',
content_type='image/jpeg', s3_data_type='S3Prefix')
validation_annotation = sagemaker.session
.s3_input(s3_train_annotation, distribution='FullyReplicated',
content_type='image/jpeg', s3_data_type='S3Prefix')
data_channels = {'train': train_data, 'validation': validation_data, 'train_annotation': train_annotation, 'validation_annotation':validation_annotation}
You can train the model with the data arranged in Amazon S3 as od_model.fit(inputs=data_channels, logs=True)
.
Model Evaluation
The displayed logs during training shows the mean average precision (mAP) on the validation data, among other metrics, and this metric can be used to infer the actual model performance. This metric is a proxy for the quality of the algorithm. Alternatively, you can also further evaluate the trained model on a separate set of test data.
Deploying the Model
When deploying an Amazon SageMaker-trained SSD model, you must first run deploy.py
(available on GitHub) to convert the model artifact into a deployable format. After cloning or downloading the MXNet repository, enter the
git reset –hard 73d88974f8bca1e68441606fb0787a2cd17eb364
command before calling to convert the model, if the latest version doesn’t work.
To convert the model, execute the following command in your terminal:
python3 deploy.py --prefix <path> --data-shape 512 --num-class 80 --network resnet50 —epoch 500
After the model artifacts are converted, prepare to deploy the solution on AWS DeepLens. An AWS DeepLens project is a deep learning-based computer vision application. It consists of a trained, converted model and a Lambda function to perform inferences based on the model.
For more information, see Working with AWS DeepLens Custom Projects.
Monitoring
AWS DeepLens automatically configures AWS IoT Greengrass Logs. AWS IoT Greengrass Logs writes logs to Amazon CloudWatch Logs and to local file system of your device. For more information about CloudWatch and File Systems logs see AWS DeepLens Project Logs.
Sensor Integration and Analytics
In addition to detecting road hazards, the solution captures various forms of data from sensors attached to either the bicycle or the cyclist. Smartcycle uses ANT+ wireless sensors for this project for the following reasons:
- The devices are widely available for cycling and other types of fitness equipment
- The sensors themselves are inexpensive
- ANT+ offers a mostly standardized non-proprietary approach for interpreting sensor data programmatically
For more information about ANT/ANT+ protocols, see the ANT+ website.
To capture the wireless sensor data, this solution uses a Python script that runs on an AWS DeepLens device, called multi_ant_demo.py
. This script executes from a terminal shell on the AWS DeepLens device. For instructions on setting up and running this script, including dependencies, see the GitHub repo.
Each ANT+ sensor category has a specific configuration. For example, for heart rate sensors, you need to use a specific channel ID, period, and frequency (120
, 57
, and 8070
, respectively). Use the following code:
#Channel 3 - Heartrate
self.channel3 = self.antnode.getFreeChannel()
self.channel3.name = 'C:HR'
self.channel3.assign('N:ANT+',
CHANNEL_TYPE_TWOWAY_RECEIVE)
self.channel3.setID(120, 0, 0)
self.channel3.setSearchTimeout(TIMEOUT_NEVER)
self.channel3.setPeriod(8070)
self.channel3.setFrequency(57)
self.channel3.open()
#Channel 4 - Temperature
self.channel4 = self.antnode.getFreeChannel()
self.channel4.name = 'C:TMP'
self.channel4.assign('N:ANT+',
CHANNEL_TYPE_TWOWAY_RECEIVE)
self.channel4.setID(25, 0, 0)
self.channel4.setSearchTimeout(TIMEOUT_NEVER)
self.channel4.setPeriod(8192)
self.channel4.setFrequency(57)
self.channel4.open()
As the multi_ant_demo.py
function receives wireless sensor information, it interprets the raw data based on the sensor type the script recognizes to make it human-readable. The processed data is inserted into the local DiskCache database keyed on the sensor type. The greengrassObjectDetector.py
function reads from the DiskCache database records to render those metrics on the AWS DeepLens video output stream. The function also sends the data to the IoT topic for further processing and persistence into Amazon DynamoDB for reporting.
Sensor Analytics
The AWS DeepLens devices that are registered for the project are associated with the AWS IoT cloud and authorized to publish messages to a unique IoT MQTT topic. In addition to showing the output video from the AWS DeepLens device, the solution also publishes sensor data to the MQTT topic. You also have a dynamic dashboard that makes use of Amazon DynamoDB, AWS Lambda, Amazon API Gateway, and a static webpage hosted in Amazon S3. In addition, you can query the hot data in Amazon S3 using pre-created Amazon Athena queries and visualize it in Amazon QuickSight.
The following diagram illustrates the analytics workflow.
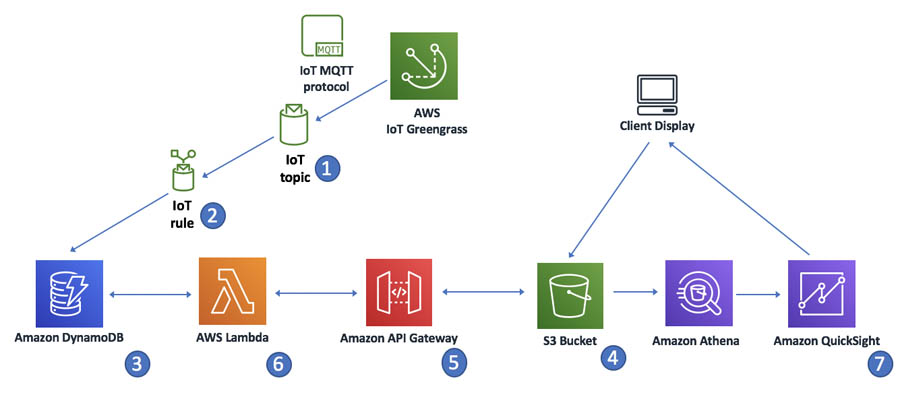
The workflow contains the following steps
- The Lambda function for AWS IoT Greengrass exchanges MQTT messages with AWS IoT Core.
- An IoT rule in AWS IoT Core listens for incoming messages from the MQTT topic. When the condition for the AWS IoT rule is met, it launches an action to send the message to the Amazon DynamoDB table.
- Messages are sent to the Amazon DynamoDB table in a time-ordered sequence. The following screenshot shows an example of timestamped sensor data in Amazon DynamoDB.
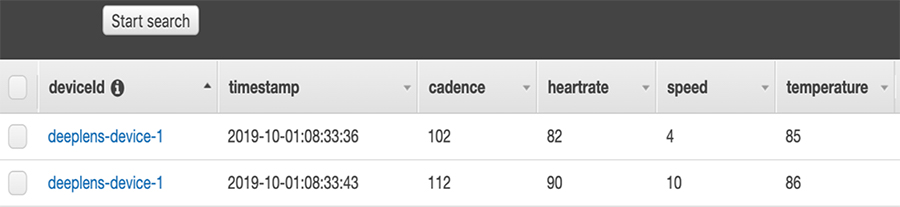
- A static webpage on Amazon S3 displays the aggregated messages.
- The GET request triggers a Lambda function to select the most recent records in the Amazon DynamoDB table and cache them in the static website.
- Amazon QuickSight provides data visualizations and one-time queries from Amazon S3 directly. The following screenshot shows an example of a near-real time visualization using Amazon QuickSight.
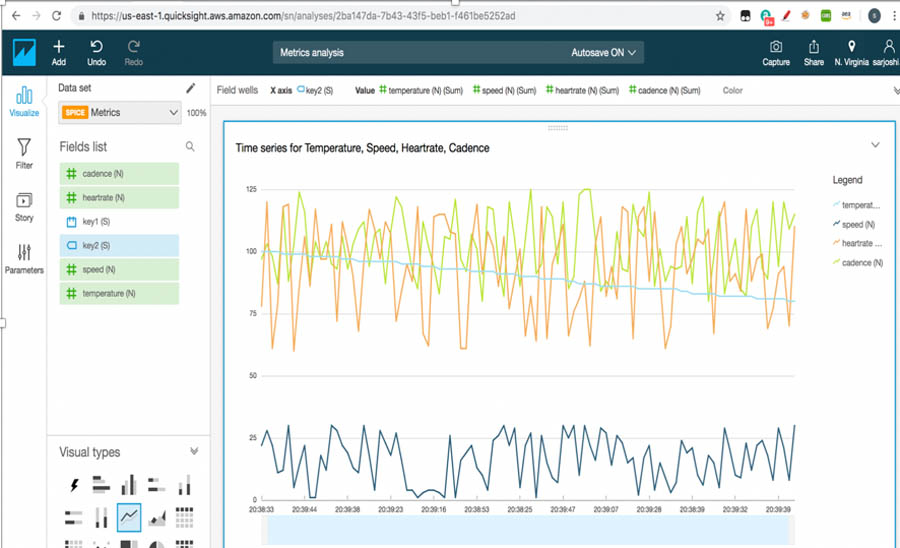
Conclusion
This post explained how to use an AWS DeepLens and the Amazon SageMaker built-in object detection algorithm to detect and localize obstacles while riding a bicycle. For instructions on implementing this solution, see the GitHub repo. You can also clone and extend this solution with additional data sources for model training. Users that implement this solution should do so at their own risk. As with all cycling activities, remember to always obey all applicable laws when cycling.
References
About the Authors
Sarita Joshi is a AI/ML Architect with AWS Professional Services. She has a Master’s Degree in Computer Science, Specialty Data from Northeastern University and has several years of experience as a consultant advising clients across many industries and technical domain – AI, ML, Analytics, SAP. Today she is passionately working with customers to develop and implement machine learning and AI solutions on AWS.
David Simcik is an AWS Solutions Architect focused on supporting ISV customers and is based out of Boston. He has experience architecting solutions in the areas of analytics, IoT, containerization, and application modernization. He holds a M.S. in Software Engineering from Brandeis University and a B.S. in Information Technology from the Rochester Institute of Technology.
Andrea Sabet leads a team of solutions architects supporting customers across the New York Metro region. She holds a M.Sc. in Engineering Physics and a B.Sc in Electrical Engineering from Uppsala University, Sweden.
Read More