Over the past few years, many automatic speech recognition (ASR) services have entered the market, offering a variety of different features. When deciding whether to use a service, you may want to evaluate its performance and compare it to another service. This evaluation process often analyzes a service along multiple vectors such as feature coverage, customization options, security, performance and latency, and integration with other cloud services.
Depending on your needs, you’ll want to check for features such as speaker labeling, content filtering, and automatic language identification. Basic transcription accuracy is often a key consideration during these service evaluations. In this post, we show how to measure the basic transcription accuracy of an ASR service in six easy steps, provide best practices, and discuss common mistakes to avoid.
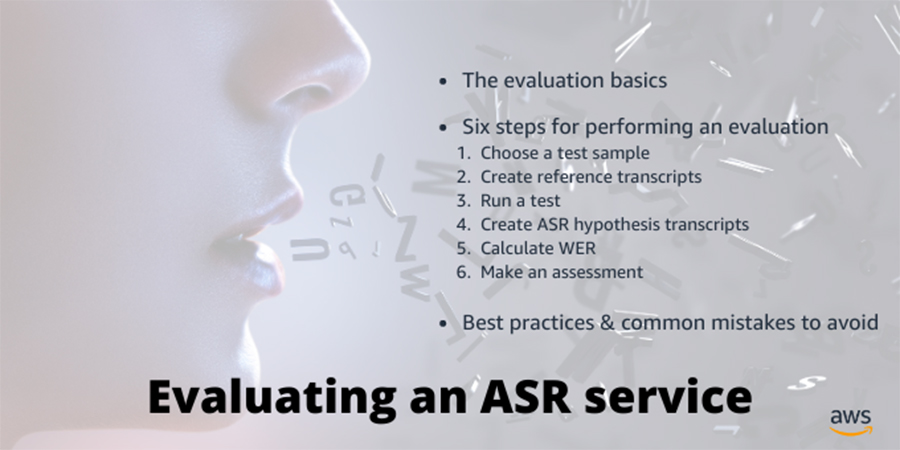
The evaluation basics
Defining your use case and performance metric
Before starting an ASR performance evaluation, you first need to consider your transcription use case and decide how to measure a good or bad performance. Literal transcription accuracy is often critical. For example, how many word errors are in the transcripts? This question is especially important if you pay annotators to review the transcripts and manually correct the ASR errors, and you want to minimize how much of the transcript needs to be re-typed.
The most common metric for speech recognition accuracy is called word error rate (WER), which is recommended by the US National Institute of Standards and Technology for evaluating the performance of ASR systems. WER is the proportion of transcription errors that the ASR system makes relative to the number of words that were actually said. The lower the WER, the more accurate the system. Consider this example:
Reference transcript (what the speaker said): well they went to the store to get sugar
Hypothesis transcript (what the ASR service transcribed): they went to this tour kept shook or
In this example, the ASR service doesn’t appear to be accurate, but how many errors did it make? To quantify WER, there are three categories of errors:
- Substitutions – When the system transcribes one word in place of another. Transcribing the fifth word as
this
instead of the
is an example of a substitution error.
- Deletions – When the system misses a word entirely. In the example, the system deleted the first word
well
.
- Insertions – When the system adds a word into the transcript that the speaker didn’t say, such as
or
inserted at the end of the example.
Of course, counting errors in terms of substitutions, deletions, and insertions isn’t always straightforward. If the speaker says “to get sugar” and the system transcribes kept shook or
, one person might count that as a deletion (to
), two substitutions (kept
instead of get
and shook
instead of sugar
), and an insertion (or
). A second person might count that as three substitutions (kept
instead of to
, shook
instead of get
, and or
instead of sugar
). Which is the correct approach?
WER gives the system the benefit of the doubt, and counts the minimum number of possible errors. In this example, the minimum number of errors is six. The following aligned text shows how to count errors to minimize the total number of substitutions, deletions, and insertions:
REF: WELL they went to THE STORE TO GET SUGAR
HYP: **** they went to THIS TOUR KEPT SHOOK OR
D S S S S S
Many ASR evaluation tools use this format. The first line shows the reference transcript, labeled REF
, and the second line shows the hypothesis transcript, labeled HYP
. The words in each transcript are aligned, with errors shown in uppercase. If a word was deleted from the reference or inserted into the hypothesis, asterisks are shown in place of the word that was deleted or inserted. The last line shows D
for the word that was deleted by the ASR service, and S
for words that were substituted.
Don’t worry if these aren’t the actual errors that the system made. With the standard WER metric, the goal is to find the minimum number of words that you need to correct. For example, the ASR service probably didn’t really confuse “get” and “shook,” which sound nothing alike. The system probably misheard “sugar” as “shook or,” which do sound very similar. If you take that into account (and there are variants of WER that do), you might end up counting seven or eight word errors. However, for the simple case here, all that matters is counting how many words you need to correct without needing to identify the exact mistakes that the ASR service made.
You might recognize this as the Levenshtein edit distance between the reference and the hypothesis. WER is defined as the normalized Levenshtein edit distance:

In other words, it’s the minimum number of words that need to be corrected to change the hypothesis transcript into the reference transcript, divided by the number of words that the speaker originally said. Our example would have the following WER calculation:

WER is often multiplied by 100, so the WER in this example might be reported as 0.67, 67%, or 67. This means the service made errors for 67% of the reference words. Not great! The best achievable WER score is 0, which means that every word is transcribed correctly with no inserted words. On the other hand, there is no worst WER score—it can even go above 1 (above 100%) if the system made a lot of insertion errors. In that case, the system is actually making more errors than there are words in the reference—not only does it get all the words wrong, but it also manages to add new wrong words to the transcript.
For other performance metrics besides WER, see the section Adapting the performance metric to your use case later in this post.
Normalizing and preprocessing your transcripts
When calculating WER and many other metrics, keep in mind that the problem of text normalization can drastically affect the calculation. Consider this example:
Reference: They will tell you again: our ballpark estimate is $450.
ASR hypothesis: They’ll tell you again our ball park estimate is four hundred fifty dollars.
The following code shows how most tools would count the word errors if you just leave the transcripts as-is:
REF: THEY WILL tell you AGAIN: our **** BALLPARK estimate is **** ******* ***** $450.
HYP: **** THEY'LL tell you AGAIN our BALL PARK estimate is FOUR HUNDRED FIFTY DOLLARS.
D S S I S I I I S
The word error rate would therefore be:

According to this calculation, there were errors for 90% of the reference words. That doesn’t seem right. The ASR hypothesis is basically correct, with only small differences:
- The words
they will
are contracted to they’ll
- The colon after
again
is omitted
- The term
ballpark
is spelled as a single compound word in the reference, but as two words in the hypothesis
$450
is spelled with numerals and a currency symbol in the reference, but the ASR system spells it using the alphabet as four hundred fifty dollars
The problem is that you can write down the original spoken words in more than one way. The reference transcript spells them one way and the ASR service spells them in a different way. Depending on your use case, you may or may not want to count these written differences as errors that are equivalent to missing a word entirely.
If you don’t want to count these kinds of differences as errors, you should normalize both the reference and the hypothesis transcripts before you calculate WER. Normalizing involves changes such as:
- Lowercasing all words
- Removing punctuation (except apostrophes)
- Contracting words that can be contracted
- Expanding written abbreviations to their full forms (such
Dr.
as to doctor
)
- Spelling all compound words with spaces (such as
blackboard
to black board
or part-time
to part time
)
- Converting numerals to words (or vice-versa)
If you there are other differences that you don’t want to count as errors, you might consider additional normalizations. For example, some languages have multiple spellings for some words (such as favorite
and favourite
) or optional diacritics (such as naïve
vs. naive
), and you may want to convert these to a single spelling before calculating WER. We also recommend removing filled pauses like uh
and um
, which are irrelevant for most uses of ASR, and therefore shouldn’t be included in the WER calculation.
A second, related issue is that WER by definition counts the number of whole word errors. Many tools define words as strings separated by spaces for this calculation, but not all writing systems use spaces to separate words. In this case, you may need to tokenize the text before calculating WER. Alternatively, for writing systems where a single character often represents a word (such as Chinese), you can calculate a character error rate instead of a word error rate, using the same procedure.
Six steps for performing an ASR evaluation
To evaluate an ASR service using WER, complete the following steps:
- Choose a small sample of recorded speech.
- Transcribe it carefully by hand to create reference transcripts.
- Run the audio sample through the ASR service.
- Create normalized ASR hypothesis transcripts.
- Calculate WER using an open-source tool.
- Make an assessment using the resulting measurement.
Choosing a test sample
Choosing a good sample of speech to evaluate is critical, and you should do this before you create any ASR transcripts in order to avoid biasing the results. You should think about the sample in terms of utterances. An utterance is a short, uninterrupted stretch of speech that one speaker produces without any silent pauses. The following are three example utterances:
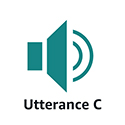
An utterance is sometimes one complete sentence, but people don’t always talk in complete sentences—they hesitate, start over, or jump between multiple thoughts within the same utterance. Utterances are often only one or two words long and are rarely more than 50 words. For the test sample, we recommend selecting utterances that are 25–50 words long. However, this is flexible and can be adjusted if your audio contains mostly short utterances, or if short utterances are especially important for your application.
Your test sample should include at least 800 spoken utterances. Ideally, each utterance should be spoken by a different person, unless you plan to transcribe speech from only a few individuals. Choose utterances from representative portions of your audio. For example, if there is typically background traffic noise in half of your audio, then half of the utterances in your test sample should include traffic noise as well. If you need to extract utterances from long audio files, you can use a tool like Audacity.
Creating reference transcripts
The next step is to create reference transcripts by listening to each utterance in your test sample and writing down what they said word-for-word. Creating these reference transcripts by hand can be time-consuming, but it’s necessary for performing the evaluation. Write the transcript for each utterance on its own line in a plain text file named reference.txt
, as shown below.
hi i'm calling about a refrigerator i bought from you the ice maker stopped working and it's still under warranty so i wanted to see if someone could come look at it
no i checked everywhere the mailbox the package room i asked my neighbor who sometimes gets my packages but it hasn't shown up yet
i tried to update my address on the on your web site but it just says error code 402 disabled account id after i filled out the form
The reference transcripts are extremely literal, including when the speaker hesitates and restarts in the third utterance (on the on your
). If the transcripts are in English, write them using all lowercase with no punctuation except for apostrophes, and in general be sure to pay attention to the text normalization issues that we discussed earlier. In this example, besides lowercasing and removing punctuation from the text, compound words have been normalized by spelling them as two words (ice maker
, web site
), the initialism I.D.
has been spelled as a single lowercase word id
, and the number 402
is spelled using numerals rather than the alphabet. By applying these same strategies to both the reference and the hypothesis transcripts, you can ensure that different spelling choices aren’t counted as word errors.
Running the sample through the ASR service
Now you’re ready to run the test sample through the ASR service. For instructions on doing this on the Amazon Transcribe console, see Create an Audio Transcript. If you’re running a large number of individual audio files, you may prefer to use the Amazon Transcribe developer API.
Creating ASR hypothesis transcripts
Take the hypothesis transcripts generated by the ASR service and paste them into a plain text file with one utterance per line. The order of the utterances must correspond exactly to the order in the reference transcript file that you created: if line 3 of your reference transcripts file has the reference for the utterance pat went to the store
, then line 3 of your hypothesis transcripts file should have the ASR output for that same utterance.
The following is the ASR output for the three utterances:
Hi I'm calling about a refrigerator I bought from you The ice maker stopped working and it's still in the warranty so I wanted to see if someone could come look at it
No I checked everywhere in the mailbox The package room I asked my neighbor who sometimes gets my packages but it hasn't shown up yet
I tried to update my address on the on your website but it just says error code 40 to Disabled Accounts idea after I filled out the form
These transcripts aren’t ready to use yet—you need to normalize them first using the same normalization conventions that you used for the reference transcripts. First, lowercase the text and remove punctuation except apostrophes, because differences in case or punctuation aren’t considered as errors for this evaluation. The word website
should be normalized to web site
to match the reference transcript. The number is already spelled with numerals, and it looks like the initialism I.D.
was transcribed incorrectly, so no need to do anything there.
After the ASR outputs have been normalized, the final hypothesis transcripts look like the following:
hi i'm calling about a refrigerator i bought from you the ice maker stopped working and it's still in the warranty so i wanted to see if someone could come look at it
no i checked everywhere in the mailbox the package room i asked my neighbor who sometimes gets my packages but it hasn't shown up yet
i tried to update my address on the on your web site but it just says error code 40 to disabled accounts idea after i filled out the form
Save these transcripts to a plain text file named hypothesis.txt
.
Calculating WER
Now you’re ready to calculate WER by comparing the reference and hypothesis transcripts. This post uses the open-source asr-evaluation evaluation tool to calculate WER, but other tools such as SCTK or JiWER are also available.
Install the asr-evaluation tool (if you’re using it) with pip install asr-evaluation, which makes the wer
script available on the command line. Use the following command to compare the reference and hypothesis text files that you created:
wer -i reference.txt hypothesis.txt
The script prints something like the following:
REF: hi i'm calling about a refrigerator i bought from you the ice maker stopped working and it's still ** UNDER warranty so i wanted to see if someone could come look at it
HYP: hi i'm calling about a refrigerator i bought from you the ice maker stopped working and it's still IN THE warranty so i wanted to see if someone could come look at it
SENTENCE 1
Correct = 96.9% 31 ( 32)
Errors = 6.2% 2 ( 32)
REF: no i checked everywhere ** the mailbox the package room i asked my neighbor who sometimes gets my packages but it hasn't shown up yet
HYP: no i checked everywhere IN the mailbox the package room i asked my neighbor who sometimes gets my packages but it hasn't shown up yet
SENTENCE 2
Correct = 100.0% 24 ( 24)
Errors = 4.2% 1 ( 24)
REF: i tried to update my address on the on your web site but it just says error code ** 402 disabled ACCOUNT ID after i filled out the form
HYP: i tried to update my address on the on your web site but it just says error code 40 TO disabled ACCOUNTS IDEA after i filled out the form
SENTENCE 3
Correct = 89.3% 25 ( 28)
Errors = 14.3% 4 ( 28)
Sentence count: 3
WER: 8.333% ( 7 / 84)
WRR: 95.238% ( 80 / 84)
SER: 100.000% ( 3 / 3)
If you want to calculate WER manually instead of using a tool, you can do so by calculating the Levenshtein edit distance between the reference and hypothesis transcript pairs divided by the total number of words in the reference transcripts. When you’re calculating the Levenshtein edit distance between the reference and hypothesis, be sure to calculate word-level edits, rather than character-level edits, unless you’re evaluating a written language where every character is a word.
In the evaluation output above, you can see the alignment between each reference transcript REF
and hypothesis transcript HYP
. Errors are printed in uppercase, or using asterisks if a word was deleted or inserted. This output is useful if you want to re-count the number of errors and recalculate WER manually to exclude certain types of words and errors from your calculation. It’s also useful to verify that the WER tool is counting errors correctly.
At the end of the output, you can see the overall WER: 8.333%. Before you go further, skim through the transcript alignments that the wer
script printed out. Check whether the references correspond to the correct hypotheses. Do the error alignments look reasonable? Are there any text normalization differences that are being counted as errors that shouldn’t be?
Making an assessment
What should the WER be if you want good transcripts? The lower the WER, the more accurate the system. However, the WER threshold that determines whether an ASR system is suitable for your application ultimately depends on your needs, budget, and resources. You’re now equipped to make an objective assessment using the best practices we shared, but only you can decide what error rate is acceptable.
You may want to compare two ASR services to determine if one is significantly better than the other. If so, you should repeat the previous three steps for each service, using exactly the same test sample. Then, count how many utterances have a lower WER for the first service compared to the second service. If you’re using asr-evaluation
, the WER for each individual utterance is shown as the percentage of Errors
below each utterance.
If one service has a lower WER than the other for at least 429 of the 800 test utterances, you can conclude that this service provides better transcriptions of your audio. 429 represents a conventional threshold for statistical significance when using a sign test for this particular sample size. If your sample doesn’t have exactly 800 utterances, you can manually calculate the sign test to decide if one service has a significantly lower WER than the other. This test assumes that you followed good practices and chose a representative sample of utterances.
Adapting the performance metric to your use case
Although this post uses the standard WER metric, the most important consideration when evaluating ASR services is to choose a performance metric that reflects your use case. WER is a great metric if the hypothesis transcripts will be corrected, and you want to minimize the number of words to correct. If this isn’t your goal, you should carefully consider other metrics.
For example, if your use case is keyword extraction and your goal is to see how often a specific set of target keywords occur in your audio, you might prefer to evaluate ASR transcripts using metrics such as precision, recall, or F1 score for your keyword list, rather than WER.
If you’re creating automatic captions that won’t be corrected, you might prefer to evaluate ASR systems in terms of how useful the captions are to viewers, rather than the minimum number of word errors. With this in mind, you can roughly divide English words into two categories:
- Content words – Verbs like “run”, “write”, and “find”; nouns like “cloud”, “building”, and “idea”; and modifiers like “tall”, “careful”, and “quickly”
- Function words – Pronouns like “it” and “they”; determiners like “the” and “this”; conjunctions like “and”, “but”, and “or”; prepositions like “of”, “in”, and “over”; and several other kinds of words
For creating uncorrected captions and extracting keywords, it’s more important to transcribe content words correctly than function words. For these use cases, we recommend ignoring function words and any errors that don’t involve content words in your calculation of WER. There is no definite list of function words, but this file provides one possible list for North American English.
Common mistakes to avoid
If you’re comparing two ASR services, it’s important to evaluate the ASR hypothesis transcript produced by each service using a true reference transcript that you create by hand, rather than comparing the two ASR transcripts to each other. Comparing ASR transcripts to each other lets you see how different the systems are, but won’t give you any sense of which service is more accurate.
We emphasized the importance of text normalization for calculating WER. When you’re comparing two different ASR services, the services may offer different features, such as true-casing, punctuation, and number normalization. Therefore, the ASR output for two systems may be different even if both systems correctly recognized exactly the same words. This needs to be accounted for in your WER calculation, so you may need to apply different text normalization rules for each service to compare them fairly.
Avoid informally eyeballing ASR transcripts to evaluate their quality. Your evaluation should be tailored to your needs, such as minimizing the number of corrections, maximizing caption usability, or counting keywords. An informal visual evaluation is sensitive to features that stand out from the text, like capitalization, punctuation, proper names, and numerals. However, if these features are less important than word accuracy for your use case—such as if the transcripts will be used for automatic keyword extraction and never seen by actual people—then an informal visual evaluation won’t help you make the best decision.
Useful resources
The following are tools and open-source software that you may find useful:
- Tools for calculating WER:
- Tools for extracting utterances from audio files:
Conclusion
This post discusses a few of the key elements needed to evaluate the performance aspect of an ASR service in terms of word accuracy. However, word accuracy is only one of the many dimensions that you need to evaluate when choosing on a particular ASR service. It’s critical that you include other parameters such as the ASR service’s total feature set, ease of use, existing integrations, privacy and security, customization options, scalability implications, customer service, and pricing.
About the Authors
Scott Seyfarth is a Data Scientist at AWS AI. He works on improving the Amazon Transcribe and Transcribe Medical services. Scott is also a phonetician and a linguist who has done research on Armenian, Javanese, and American English.
Paul Zhao is Product Manager at AWS AI. He manages Amazon Transcribe and Amazon Transcribe Medical. In his past life, Paul was a serial entrepreneur, having launched and operated two startups with successful exits.
Read More