We’re excited to announce the global availability of AWS Contact Center Intelligence (AWS CCI) solutions powered by AWS AI Services and made available through the AWS Partner Network. AWS CCI solutions enable you to leverage AWS machine learning (ML) capabilities with your current contact center provider to gain greater efficiencies and deliver increasingly tailored customer experiences —with no ML expertise required.
AWS CCI solutions use a combination of AWS AI-powered services for text-to-speech, translation, intelligent search, conversational AI, transcription, and language comprehension capabilities. We’re delighted to announce the addition of AWS Technology Partners: Salesforce, Avaya, Talkdesk, 8×8, Clarabridge, Clevy, XappAI, and Voiceworx. We are also adding new AWS Consulting Partners: Inawisdom, Cation Consulting, HCL Technologies, Wipro, First Derivatives, Servion, and Lucy in the Cloud/Micropole for customers who require a custom solution or seek additional assistance with AWS CCI. These new partners provide customers across the globe more opportunities to benefit from AWS ML-powered contact center intelligence solutions to enhance self-service, analyze calls in real time to assist agents, and learn from all contact center interactions with post-call analytics.
Around the world, the volume of interactions in contact centers continues to increase. Companies see multiple opportunities to leverage AI technology to improve the customer experience. This can include 24/7 self-serve virtual agents that can provide timely and accurate answers to customer queries, call analytics and agent assist to improve agent productivity, or call analytics to generate further improvements in their operations. However, piecing together the various technologies to build an ML-driven intelligent contact center unique to the goals and needs of each business can be a significant undertaking. You want the benefits that intelligent contact center technologies bring, but the resources, time and cost to implement are often too high to overcome. AWS CCI provides a simple and fast route to deploy AWS ML solutions no matter which contact center provider you use.
AWS CCI customer success stories
Multiple customers already benefit from an improved customer experience and reduced operational costs as a result of using AWS CCI solutions through AWS Partners. Here are some example of AWS CCI customer stories.
Maximus is a leading pure-play provider in the administration of government health and human services programs, and is the largest provider of contact center services to the government. Tom Romeo, the General Manager at Maximus Federal, says, “At Maximus, we are constantly looking for new ways to innovate and improve the Citizen Journey and contact center experience. With AWS Partner SuccessKPI, we were able to add AWS CCI into our Genesys Cloud environment in a matter of hours and deliver a 360-degree view of the citizen experience. This program allowed us to deliver increased capacity, automated quality review, and agent compliance and performance improvements for government agencies.”
Magellan Health is a large managed health care company focused on special population, complete pharmacy benefits and other specialty areas. Brian Lichtle, the Senior Director of Software Engineering at Magellan Rx says,
“We chose Amazon Kendra, a service within AWS CCI to build a secure and scalable agent assist application. This helped call center agents, and the customer they serve quickly uncover the information they need. Since implementing CCI and Amazon Kendra, early results show an average reduction in call times of about 9-15 seconds, which saves more than 4.4k hours on over 2.2 million calls per calendar year.”
Cation Consulting is an AWS consulting partner focused on delivering robust, conversational AI experiences to customers. Alan Kiernan, the co-founder and CTO at Cation Consulting says, “At Cation Consulting, we provide customers with conversational AI and self-service experiences that allow them to significantly reduce customer support costs while improving the customer experience. AWS Contact Center Intelligence enables us to move quickly and scale seamlessly with customers such as Ryanair, the largest airline in Europe. The Ryanair chatbot has handled millions of customer enquiries per year as a trusted extension of the Ryanair’s customer care team. We are excited to leverage Amazon Lex’s recent expansion into European languages and design virtual agents who can resolve customer issues quickly and improve customer service ratings.”
New AWS CCI language support and partner additions
In addition to our new partners, AWS CCI continues to expand its global capabilities with new language expansions. AWS CCI has 3 pre-configured solutions available through participating APN partners, focused on the contact center workflow: Self-Service, Live Call Analytics and Agent Assist, and Post-Call Analytics. The Self-Service solution uses ML-driven chatbots and Interactive Voice Response (IVR) to address and deflect the most common tasks and queries so that the contact center workforce can focus on resolving interactions that need a human touch. The Self-Service solution utilizes the conversational interface of Amazon Lex and the text to speech voices of Amazon Polly to create a dynamic virtual agent in multiple languages such as French, German, Italian, and Spanish. Adding Amazon Kendra can boost the ability of these virtual agents to answer questions by finding the best answers from internal knowledge bases. The Live Call Analytics & Agent Assist and Post-Call Analytics solutions use Amazon Transcribe to perform real-time or post-call speech transcription with Amazon Comprehend to automatically analyze the interaction, detect call sentiment, and identify key words and phrases in the conversation using natural language processing (NLP) to increase agent productivity. These key words can then be utilized by the intelligent search capabilities of Amazon Kendra to help agents find timely and relevant information to resolve live call issues more quickly. Transcribing live calls is now available in German, Italian, Japanese, Korean, and Portuguese languages. Amazon Translate can also be used to translate calls into an agent’s preferred language and supports a total of 71 languages and variants.
“At Amazon, we want to meet the customer wherever they are in their contact center journey. With AWS CCI, we wanted to make it easy for customers who use different contact centers providers to add AI and achieve new levels of operational efficiency.” says Vasi Philomin, GM of AWS Language Services, AI. “Having a global partner network is critical to enabling our customers to realize the benefits of cloud-based machine learning services and removing the need to hire specialized developers to build and maintain these systems.”
Talkdesk is a cloud contact center for innovative enterprises, combining enterprise performance with consumer simplicity resulting in higher customer satisfaction, productivity and cost savings. Tiago Paiva, chief executive officer at Talkdesk, shares, “Combining Talkdesk cloud innovations with powerful AI and machine learning services from AWS extends the capabilities and choices available to Talkdesk customers. We are excited to add new, out-of-the-box options through AWS Contact Center Intelligence solutions to help the Talkdesk user base rise above their market peers through superior customer service.”
8×8 is a leading contact center provider. Manu Mukerji, the Vice President of Engineering at 8×8, Inc., says, “By partnering with AWS, we can deliver to businesses and organizations superior bi-directional integration with AWS CCI, providing a best-in-class experience for customers. The 8×8 integration with AWS CCI makes it easy for customers to leverage AI capabilities even if they have no AI experience. The 8×8 Virtual Agent is the only fully managed and customizable solution in the market that works seamlessly for both unified communications and contact center use cases, enhancing contact center efficiency for reduced wait times and faster time to resolution.”
Pat Higbie, Co-founder and CEO of XAPP AI, an AWS Technology Partner, says, “Amazon Lex, Amazon Kendra and Amazon Polly provide a powerful combination of AI services that enables contact centers to transcend the limitations of traditional chatbots and IVR to transform their operations with truly conversational self-service that improves the customer experience and delivers dramatic ROI. And, AWS CCI solutions can be integrated with all contact center brands to bring the value of AWS AI services to any enterprise quickly.”
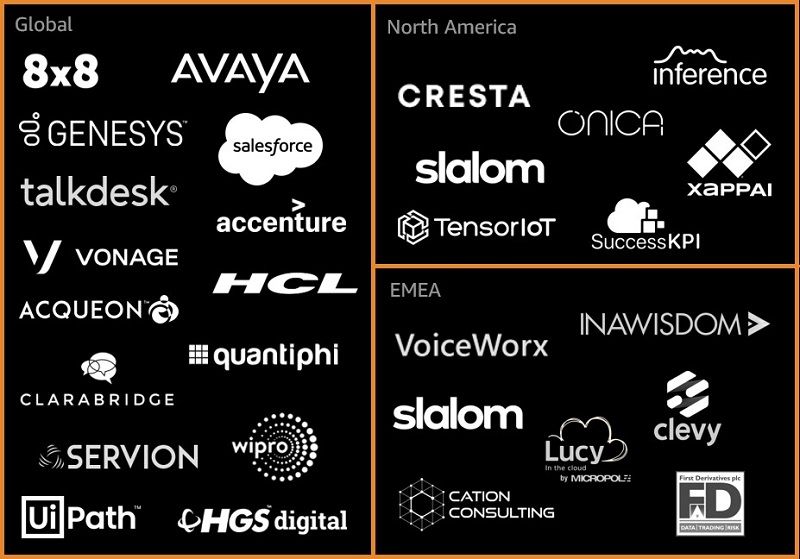
Getting started
There are multiple ways to get started with AWS CCI. To find a participating partner, see the AWS CCI partner page for more information and contact details.
To learn more, please join us for any or all of the following sessions hosted by AWS and our AWS CCI partners.
re:Invent sessions
Learn how you can leverage AWS CCI solutions to improve the customer experience and reduce cost with AI. Explore how AWS CCI solutions can be built easily through an expanding network of partners to provide self-service interactions, live and post-call analytics, and agent assist on existing contact center systems. AWS Partner SuccessKPI shares how it uses CCI solutions to improve the customer experience and tackle challenging business problems such as reducing call volume, improving agent effectiveness, and automating quality management in enterprise contact centers for customers like Maximus.
Numerous stakeholders including content designers, developers, and business owners collaborate to create a bot. In this session, hear how Dropbox used the Amazon Lex interface to build a chatbot as a support offering. The session covers how the Amazon Lex console allows you to easily create flows and manage them, and it details the decoupling that should exist between the bot author and developer for an optimal collaborative model. Finally, this session provides insights into conversational interface (CI) and conversational design (CD), language localization, and deployment practices.
Answering customer questions is essential to the customer support experience. Powered by ML, Amazon Kendra is an enterprise search service that can add Q&A capabilities to your virtual agents or boost call center agent productivity with live call agent assistance. In this session, you hear how Magellan RX Management augmented the call center experience using Amazon Kendra to help agents find accurate information faster.
In this session, learn how to train custom language models in Amazon Transcribe that supercharge speech recognition accuracy. Octopus Energy, a UK-based utility company, shares how it leverages domain-specific data to train a custom language model that is fine-tuned for its business needs and specific use case.
Partner sessions
- How to boost the return on your contact center investments with AI
January 26 at 10:00 am PST – REGISTER HERE
Presented by Acqueon and AWS
With AI technologies maturing, enterprises are embracing them to delight customers and improve the operational productivity of their contact centers. In this educational webinar, AI expert Chris Featherstone, Global Business Development Leader for AWS CCI and industry veteran Nicolas de Kouchkovsky, CMO at Acqueon, discuss how to integrate AI into your contact center software stack. They will provide an update on industry adoption and share the art of the possible without having to overhaul your technology investments.
- Gain Control of your CX with a 360 CCI Power View: A step by step guide
January 27, 2021 at 1PM EST/10AM PST – REGISTER HERE
Presented by SuccessKPI and AWS
Managing customer experience requires tackling a complex set of metrics across agents, queues, geographies, customer types, and channels. Mix in the data from speech analytics, chatbots, and post call surveys, and the picture gets blurry very quickly. In this informative webinar, we explore the factors that make customer experience management such a quagmire and provide a series of recommendations and steps to help put you in control of your customer experience.
- Add Intelligence to your existing contact center with AWS Contact Center Intelligence and Talkdesk
February 24, 2021 at 9am BRT, 9am MXT, and 9am PST – REGISTER HERE
Presented by Talkdesk and AWS at AWS Innovate – AI/ML Edition
Learn how your organization can leverage AWS Contact Center Intelligence (CCI) solutions and AWS Partner, Talkdesk, to improve customer experience and reduce cost with AI. We will explore how AWS CCI solutions can be built easily to provide self-service interactions, live and post-call analytics and agent assist on existing contact center systems. Talkdesk will also share how they improve customer experience and tackle challenging business problems such as improving agent effectiveness, and automating quality management in enterprise contact centers.
About the Author
Eron Kelly is the worldwide leader of Product Marketing for a broad portfolio of AWS services that cover Compute, Storage, Networking, Contact Centers, End User Computing and Business Applications. In this capacity, his team leads all aspects of product marketing including messaging, positioning, launches, web strategy and execution, service adoption, and field enablement. Prior to AWS, he has led sales and marketing teams at Microsoft, Proctor and Gamble and was a Captain in the Air Force. Outside of work, Mr. Kelly is very active raising a family of four kids. He is a member of the Board of Trustees at Eastside Catholic School in Sammamish, WA, and spent the last 10 years coaching youth lacrosse.
Esther Lee is a Product Manager for AWS Language AI Services. She is passionate about the intersection of technology and education. Out of the office, Esther enjoys long walks along the beach, dinners with friends and friendly rounds of Mahjong.
Read More