
We’ve discovered neurons in CLIP that respond to the same concept whether presented literally, symbolically, or conceptually. This may explain CLIP’s accuracy in classifying surprising visual renditions of concepts, and is also an important step toward understanding the associations and biases that CLIP and similar models learn.
Fifteen years ago, Quiroga et al. discovered that the human brain possesses multimodal neurons. These neurons respond to clusters of abstract concepts centered around a common high-level theme, rather than any specific visual feature. The most famous of these was the “Halle Berry” neuron, a neuron featured in both Scientific American and The New York Times, that responds to photographs, sketches, and the text “Halle Berry” (but not other names).
Two months ago, OpenAI announced CLIP, a general-purpose vision system that matches the performance of a ResNet-50, but outperforms existing vision systems on some of the most challenging datasets. Each of these challenge datasets, ObjectNet, ImageNet Rendition, and ImageNet Sketch, stress tests the model’s robustness to not recognizing not just simple distortions or changes in lighting or pose, but also to complete abstraction and reconstruction—sketches, cartoons, and even statues of the objects.
Now, we’re releasing our discovery of the presence of multimodal neurons in CLIP. One such neuron, for example, is a “Spider-Man” neuron (bearing a remarkable resemblance to the “Halle Berry” neuron) that responds to an image of a spider, an image of the text “spider,” and the comic book character “Spider-Man” either in costume or illustrated.
Our discovery of multimodal neurons in CLIP gives us a clue as to what may be a common mechanism of both synthetic and natural vision systems—abstraction. We discover that the highest layers of CLIP organize images as a loose semantic collection of ideas, providing a simple explanation for both the model’s versatility and the representation’s compactness.
Biological neurons, such as the famed Halle Berry neuron, do not fire for visual clusters of ideas, but semantic clusters. At the highest layers of CLIP, we find similar semantic invariance. Note that images are replaced by higher resolution substitutes from Quiroga et al., and that the images from Quiroga et al. are themselves substitutes of the original stimuli.
Using the tools of interpretability, we give an unprecedented look into the rich visual concepts that exist within the weights of CLIP. Within CLIP, we discover high-level concepts that span a large subset of the human visual lexicon—geographical regions, facial expressions, religious iconography, famous people and more. By probing what each neuron affects downstream, we can get a glimpse into how CLIP performs its classification.
Multimodal neurons in CLIP
Our paper builds on nearly a decade of research into interpreting convolutional networks, beginning with the observation that many of these classical techniques are directly applicable to CLIP. We employ two tools to understand the activations of the model: feature visualization, which maximizes the neuron’s firing by doing gradient-based optimization on the input, and dataset examples, which looks at the distribution of maximal activating images for a neuron from a dataset.
Using these simple techniques, we’ve found the majority of the neurons in CLIP RN50x4 (a ResNet-50 scaled up 4x using the EfficientNet scaling rule) to be readily interpretable. Indeed, these neurons appear to be extreme examples of “multi-faceted neurons,” neurons that respond to multiple distinct cases, only at a higher level of abstraction.
Any
Text
Face
Logo
Architecture
Indoor
Nature
Selected neurons from the final layer of four CLIP models. Each neuron is represented by a feature visualization with a human-chosen concept labels to help quickly provide a sense of each neuron. Labels were picked after looking at hundreds of stimuli that activate the neuron, in addition to feature visualizations. We chose to include some of the examples here to demonstrate the model’s proclivity towards stereotypical depictions of regions, emotions, and other concepts. We also see discrepancies in the level of neuronal resolution: while certain countries like the US and India were associated with well-defined neurons, the same was not true of countries in Africa, where neurons tended to fire for entire regions. We discuss some of these biases and their implications in later sections.
Indeed, we were surprised to find many of these categories appear to mirror neurons in the medial temporal lobe documented in epilepsy patients with intracranial depth electrodes. These include neurons that respond to emotions, animals, and famous people.
But our investigation into CLIP reveals many more such strange and wonderful abstractions, including neurons that appear to count [17, 202, 310], neurons responding to art styles [75, 587, 122], even images with evidence of digital alteration [1640].
Absent concepts
While this analysis shows a great breadth of concepts, we note that a simple analysis on a neuron level cannot represent a complete documentation of the model’s behavior. The authors of CLIP have demonstrated, for example, that the model is capable of very precise geolocation, (Appendix E.4, Figure 20) with a granularity that extends down to the level of a city and even a neighborhood. In fact, we offer an anecdote: we have noticed, by running our own personal photos through CLIP, that CLIP can often recognize if a photo was taken in San Francisco, and sometimes even the neighbourhood (e.g., “Twin Peaks”).
Despite our best efforts, however, we have not found a “San Francisco” neuron, nor did it seem from attribution that San Francisco decomposes nicely into meaningful unit concepts like “California” and “city.” We believe this information to be encoded within the activations of the model somewhere, but in a more exotic way, either as a direction or as some other more complex manifold. We believe this to be a fruitful direction for further research.
How multimodal neurons compose
These multimodal neurons can give us insight into understanding how CLIP performs classification. With a sparse linear probe, we can easily inspect CLIP’s weights to see which concepts combine to achieve a final classification for ImageNet classification:
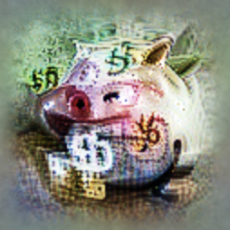
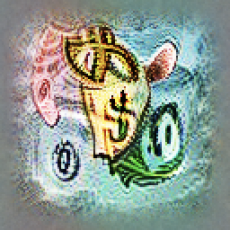
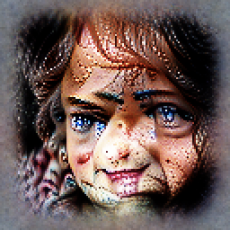
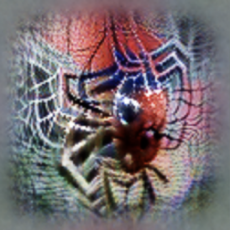
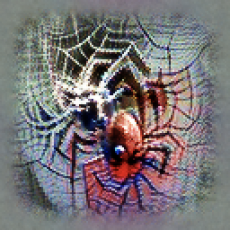
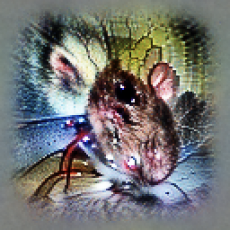
The piggy bank class appears to be a composition of a “finance” neuron along with a porcelain neuron. The Spider-Man neuron referenced in the first section of the paper is also a spider detector, and plays an important role in the classification of the class “barn spider.”
For text classification, a key observation is that these concepts are contained within neurons in a way that, similar to the word2vec objective, is almost linear. The concepts, therefore, form a simple algebra that behaves similarly to a linear probe. By linearizing the attention, we too can inspect any sentence, much like a linear probe, as shown below:
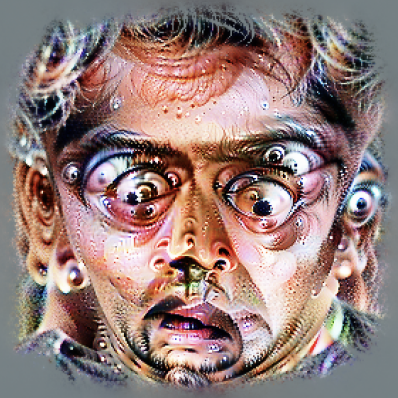
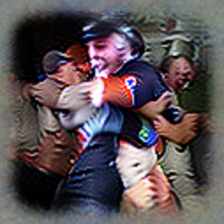
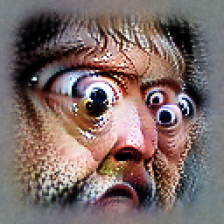
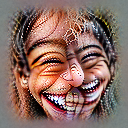
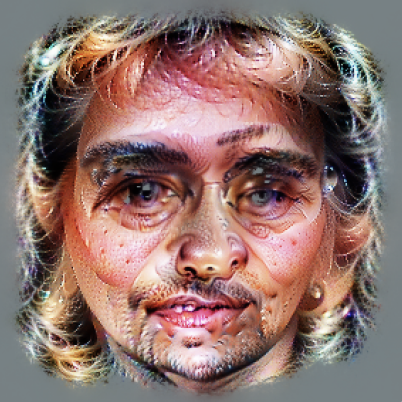
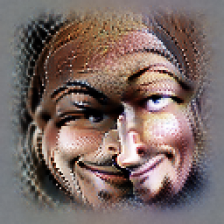
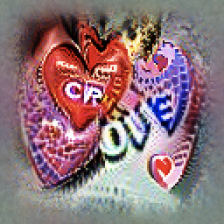
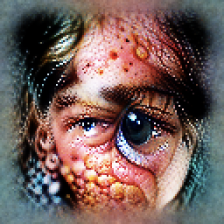
Probing how CLIP understands words, it appears to the model that the word “surprised” implies some not just some measure of shock, but a shock of a very specific kind, one combined perhaps with delight or wonder. “Intimate” consists of a soft smile and hearts, but not sickness. We note that this reveals a reductive understanding of the the full human experience of intimacy-the subtraction of illness precludes, for example, intimate moments with loved ones who are sick. We find many such omissions when probing CLIP’s understanding of language.
Fallacies of abstraction
The degree of abstraction in CLIP surfaces a new vector of attack that we believe has not manifested in previous systems. Like many deep networks, the representations at the highest layers of the model are completely dominated by such high-level abstractions. What distinguishes CLIP, however, is a matter of degree—CLIP’s multimodal neurons generalize across the literal and the iconic, which may be a double-edged sword.
Through a series of carefully-constructed experiments, we demonstrate that we can exploit this reductive behavior to fool the model into making absurd classifications. We have observed that the excitations of the neurons in CLIP are often controllable by its response to images of text, providing a simple vector of attacking the model.
The finance neuron [1330], for example, responds to images of piggy banks, but also responds to the string “$$$”. By forcing the finance neuron to fire, we can fool our model into classifying a dog as a piggy bank.
Attacks in the wild
We refer to these attacks as typographic attacks. We believe attacks such as those described above are far from simply an academic concern. By exploiting the model’s ability to read text robustly, we find that even photographs of hand-written text can often fool the model. Like the Adversarial Patch, this attack works in the wild; but unlike such attacks, it requires no more technology than pen and paper.
We also believe that these attacks may also take a more subtle, less conspicuous form. An image, given to CLIP, is abstracted in many subtle and sophisticated ways, and these abstractions may over-abstract common patterns—oversimplifying and, by virtue of that, overgeneralizing.
Bias and overgeneralization
Our model, despite being trained on a curated subset of the internet, still inherits its many unchecked biases and associations. Many associations we have discovered appear to be benign, but yet we have discovered several cases where CLIP holds associations that could result in representational harm, such as denigration of certain individuals or groups.
We have observed, for example, a “Middle East” neuron [1895] with an association with terrorism; and an “immigration” neuron [395] that responds to Latin America. We have even found a neuron that fires for both dark-skinned people and gorillas [1257], mirroring earlier photo tagging incidents in other models we consider unacceptable.
These associations present obvious challenges to applications of such powerful visual systems.[1] Whether fine-tuned or used zero-shot, it is likely that these biases and associations will remain in the system, with their effects manifesting in both visible and nearly invisible ways during deployment. Many biased behaviors may be difficult anticipate a priori, making their measurement and correction difficult. We believe that these tools of interpretability may aid practitioners the ability to preempt potential problems, by discovering some of these associations and ambigiuities ahead of time.
Our own understanding of CLIP is still evolving, and we are still determining if and how we would release large versions of CLIP. We hope that further community exploration of the released versions as well as the tools we are announcing today will help advance general understanding of multimodal systems, as well as inform our own decision-making.
Conclusion
Alongside the publication of “Multimodal Neurons in Artificial Neural Networks,” we are also releasing some of the tools we have ourselves used to understand CLIP—the OpenAI Microscope catalog has been updated with feature visualizations, dataset examples, and text feature visualizations for every neuron in CLIP RN50x4. We are also releasing the weights of CLIP RN50x4 and RN101 to further accommodate such research. We believe these investigations of CLIP only scratch the surface in understanding CLIP’s behavior, and we invite the research community to in improving our understanding of CLIP and models like it.
OpenAI