This is the second in a two part series on Amazon Comprehend custom classification models. In Part 1 of this series, we looked at how to build an AWS Step Functions workflow to automatically build, test, and deploy Amazon Comprehend custom classification models and endpoints. In Part 2, we look at real-time classification APIs, feedback loops, and human review workflows that help with continuous model training to keep the model up-to-date with new data and patterns. You can find Part 1 here.
The Amazon Comprehend custom classification API enables you to easily build custom text classification models using your business-specific labels without learning machine learning (ML). For example, your customer support organization can use custom classification to automatically categorize inbound requests by problem type based on how the customer described the issue. You can use custom classifiers to automatically label support emails with appropriate issue types, thereby routing customer phone calls to the right agents and categorizing social media posts into user segments.
In Part 1 of this series, we looked at how to build an AWS Step Functions workflow to automatically build, test, and deploy Amazon Comprehend custom classification models and endpoints. In this post, we cover the real-time classification APIs, feedback loops, and human review workflows that help with continuous model training to keep it up to date with new data and patterns.
Solution architecture
This post describes a reference architecture for retraining custom classification models. The architecture comprises real-time classification, feedback pipelines, human review workflows using Amazon Augmented AI (Amazon A2I) , preparing new training data from the human review data, and triggering the model building flow that we covered in Part 1 of this series.
The following diagram illustrates this architecture covering the last three components. In the following sections, we walk you through each step in the workflow.
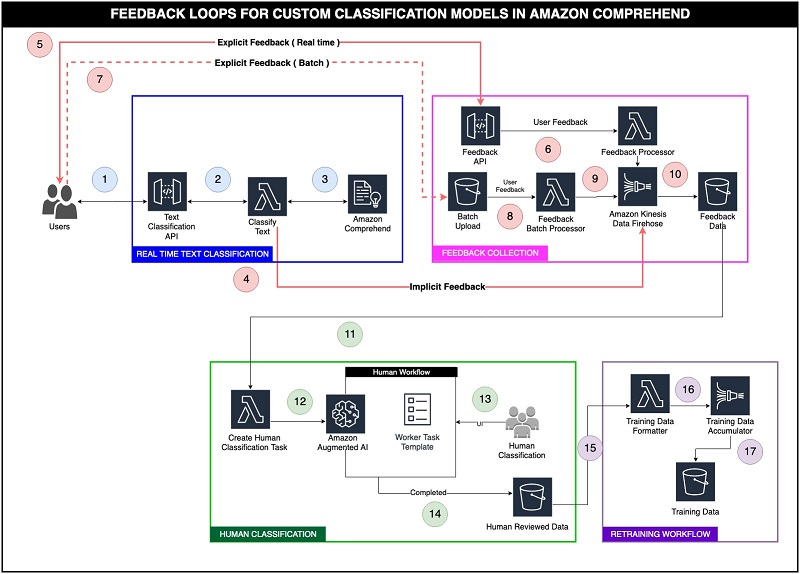
Real-time classification
To use custom classification in Amazon Comprehend in real time, you need to create an API that calls the custom classification model endpoint with the text that needs to be classified. This stage is represented by Steps 1–3 in the preceding architecture:
- The end user application calls an Amazon API Gateway endpoint with a text that needs to be classified.
- The API Gateway endpoint then calls an AWS Lambda function configured to call an Amazon Comprehend endpoint.
- The Lambda function calls the Amazon Comprehend endpoint, which returns the unlabeled text classification and a confidence score.
Feedback collection
When the endpoint returns the classification and the confidence score during the real-time classification, you can send instances with low confidence scores to human review. This type of feedback is called implicit feedback.
- The Lambda function sends the implicit feedback to an Amazon Kinesis Data Firehose delivery stream.
The other type of feedback is called explicit feedback, and comes from the application’s end users that use the custom classification feature. This type of feedback comprises the instances of text where the user wasn’t happy with the prediction. You can send explicit feedback either in real time through an API or a batch process.
- End users of the application submit explicit real-time feedback through an API Gateway endpoint.
- The Lambda function backing the API endpoint transforms the data into a standard feedback format and writes it to the Kinesis Data Firehose delivery stream.
- End users of the application can also submit explicit feedback as a batch file by uploading it to an S3 bucket.
- A trigger configured on the S3 bucket triggers a Lambda function.
- The Lambda function transforms the data into a standard feedback format and writes it to the delivery stream.
- Both the implicit and explicit feedback data get sent to a delivery stream in a standard format. All this data is buffered and written to an S3 bucket.
Human classification
The human classification stage includes the following steps:
- A trigger configured on the feedback bucket in Step 10 invokes a Lambda function.
- The Lambda function creates Amazon A2I human review tasks for all the feedback data received.
- Workers assigned to the classification jobs log in to the human review portal and either approve the classification by the model or classify the text with the right labels.
- After the human review, all these instances are stored in an S3 bucket and used for retraining the models.
Retraining workflow
The retraining workflow stage includes the following steps:
- A trigger configured on the human-reviewed data bucket in Step 14 invokes a Lambda function.
- The function transforms the human-reviewed data payload to a comma-separated training data format, required by Amazon Comprehend custom classification models. After transformation, this data is written to a Firehose delivery stream, which acts as an accumulator.
- Depending on the time frame set for retraining models, the delivery stream flushes the data into the training bucket that was created in Part 1 of this series. For this post, we set the buffer conditions to 1 MiB or 60 seconds. For your own use case, you might want to adjust these settings so model retraining occurs according to your time or size requirements. This completes the active learning loop, and starts the Step Functions workflow for retraining models.
Solution overview
The next few sections of the post go over how to set up this architecture in your AWS account. We classify news into four categories: World, Sports, Business, and Sci/Tech, using the AG News dataset for custom classification, and set up the implicit and explicit feedback loop. You need to complete two manual steps:
- Create an Amazon Comprehend custom classifier and an endpoint.
- Create an Amazon SageMaker private workforce, worker task template, and human review workflow.
After this, you run the provided AWS CloudFormation template to set up the rest of the architecture.
Prerequisites
If you’re continuing from Part 1 of this series, you can skip to the step Create a private workforce, worker task template, and human review workflow.
Create a custom classifier and an endpoint
Before you get started, download the dataset and upload it to Amazon S3. This dataset comprises a collection of news articles and their corresponding category labels. We have created a training dataset called train.csv
from the original dataset and made it available for download.
The following screenshot shows a sample of the train.csv
file.

After you download the train.csv
file, upload it to an S3 bucket in your account for reference during training. For more information about uploading files, see How do I upload files and folders to an S3 bucket?
To create your classifier for classifying news, complete the following steps:
- On the Amazon Comprehend console, choose Custom Classification.
- Choose Train classifier.
- For Name, enter
news-classifier-demo
.
- Select Using Multi-class mode.
- For Training data S3 location, enter the path for
train.csv
in your S3 bucket, for example, s3://
<your-bucketname>/train.csv
.
- For Output data S3 location, enter the S3 bucket path where you want the output, such as
s3://
<your-bucketname
>/
.
- For IAM role, select Create an IAM role.
- For Permissions to access, choose Input and output (if specified) S3 bucket.
- For Name suffix, enter
ComprehendCustom
.
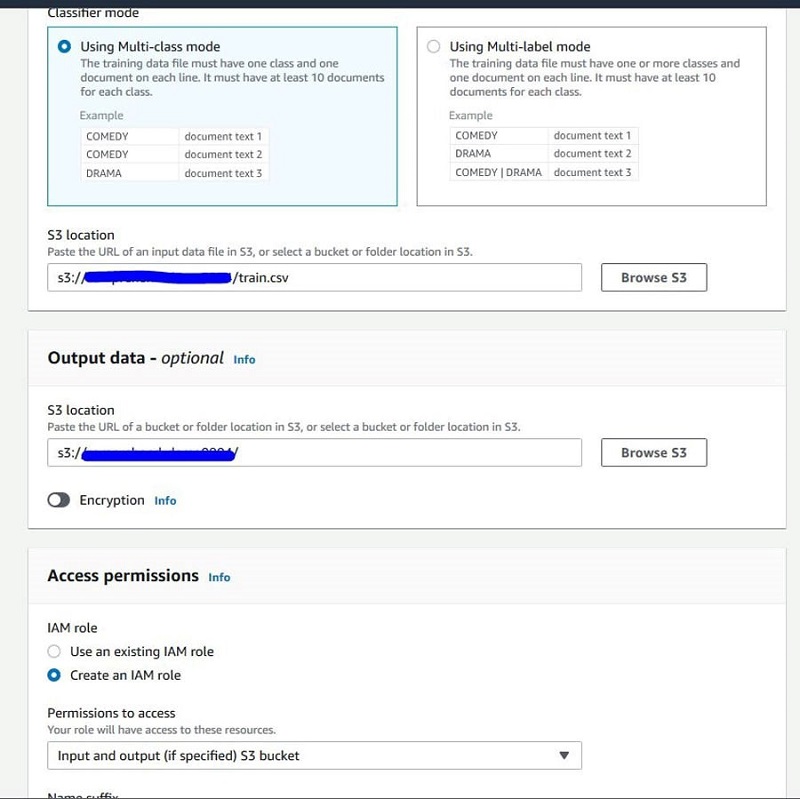
- Scroll down and choose Train Classifier to start the training process.
The training takes some time to complete. You can either wait to create an endpoint or come back to this step later after finishing the steps in the section Create a private workforce, worker task template, and human review workflow.
Create a custom classifier real-time endpoint
To create your endpoint, complete the following steps:
- On the Amazon Comprehend console, choose Custom Classification.
- From the Classifiers list, choose the name of the custom model for which you want to create the endpoint and select your model
news-classifier-demo
.
- On the Actions drop-down menu, choose Create endpoint.
- For Endpoint name, enter
classify-news-endpoint
and give it one inference unit.
- Choose Create endpoint.
- Copy the endpoint ARN as shown in the following screenshot. You use it when running the CloudFormation template in a future step.
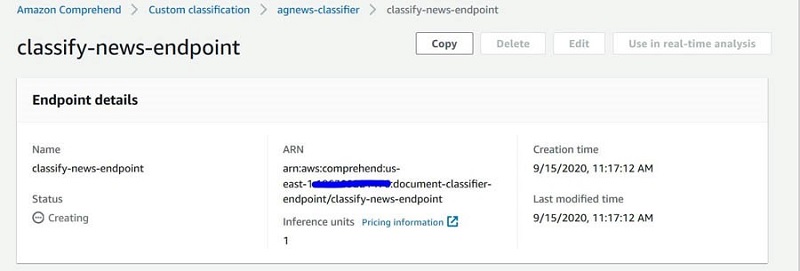
Create a private workforce, worker task template, and human review workflow
This section walks you through creating a private workforce in SageMaker, a worker task template, and your human review workflow.
Create a labeling workforce
For this post, you create a private work team and add only one user (you) to it. For instructions, see Create a Private Workforce (Amazon SageMaker Console).
After the user accepts the invitation, you add them to the workforce. For instructions, see the Add a Worker to a Work Team section the Manage a Workforce (Amazon SageMaker Console).
Create a worker task template
To create a worker task template, complete the following steps:
- On the Amazon A2I console, choose Worker task templates.
- Choose to Create a template.
- For Template name, enter
custom-classification-template
.
- For Template type, choose Custom,
- In the Template editor, enter the following GitHub UI template code.
- Choose Create.
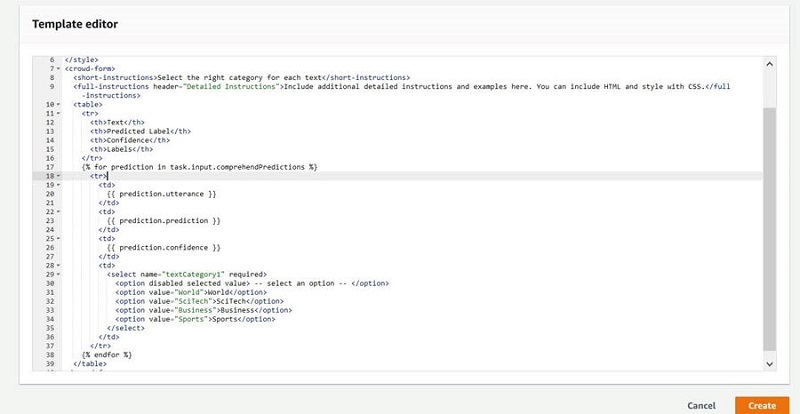
Create a human review workflow
To create your human review workflow, complete the following steps:
- On the Amazon A2I console, choose Human review workflows.
- Choose Create human review workflow.
- For Name, enter
classify-workflow
.
- Create a S3 bucket to store the human review output. Make a note of this bucket, because we use this in the later part of the post.
- Specify an S3 bucket to store output:
s3://
<your bucketname>/
. Use the bucket created earlier.
- For IAM role, select Create a new role.
- For Task type, choose Custom.
- Under Worker task template creation, select the custom classification template you created.
- For Task description, enter
Read the instructions and review the document
.
- Under Workers, select Private.
- Use the drop-down list to choose the private team that you created.
- Choose Create.
- Copy the workflow ARN (see the following screenshot). You will use it when initializing the CloudFormation template parameters in a later step.
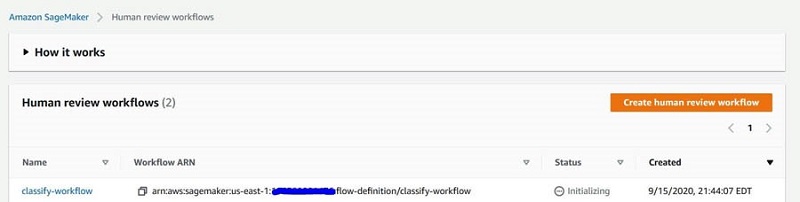
Deploy the CloudFormation template to set up active learning feedback
Now that you have completed the manual steps, you can run the CloudFormation template to set up this architecture’s building blocks, including the real-time classification, feedback collection, and the human classification.
Before deploying the CloudFormation template, make sure you have the following to pass as parameters:
- Custom classifier endpoint ARN
- Amazon A2I workflow ARN
- Choose Launch Stack:

- You must set this parameter, only if you’re continuing from Part 1 of this series. For BucketFromBlogPart1, enter the bucket name that was created for storing training data in Part 1 of this blog series.
- You must set this parameter, only if you’re continuing from Part 1 of this series. For ComprehendEndpointParameterKey, enter
/
<<StackName of Part1 Blog>>/CURRENT_CLASSIFIER_ENDPOINT
. This parameter can be found in the Parameter Store section of the Systems Manager.
- You’re not required to set this parameter if you’re continuing from Part 1.For ComprehendEndpointARN, enter the endpoint ARN of your Amazon Comprehend custom classification model.
- For HumanReviewWorkflowARN, enter the workflow ARN you copied.
- For ComrehendClassificationScoreThreshold, enter
0.5
, which means a 50% threshold for low confidence scores.
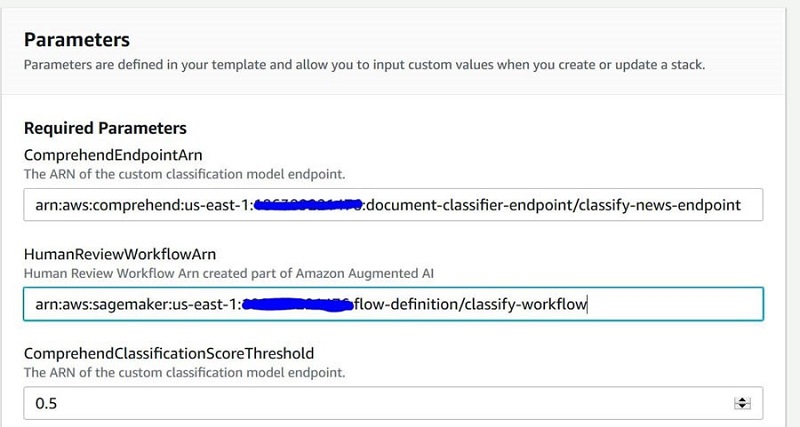
- Choose Next until the Capabilities
- Select the check box to provide acknowledgment to AWS CloudFormation to create AWS Identity and Access Management (IAM) resources and expand the template.
For more information about these resources, see AWS IAM resources.
- Choose Create stack.
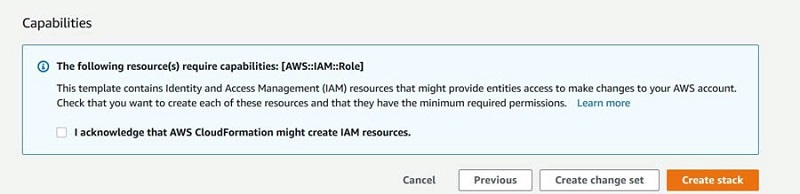
Wait until the status of the stack changes from CREATE_IN_PROGRESS
to CREATE_COMPLETE
.
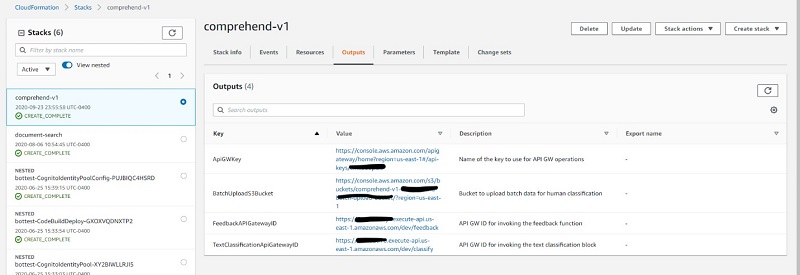
- On the Outputs tab of the stack (see the following screenshot), copy the value for
BatchUploadS3Bucket
, FeedbackAPIGatewayID,
and TextClassificationAPIGatewayID
to interact with the feedback loop.
Both the TextClassificationAPI
and FeedbackAPI
require an API key to interact with them. The CloudFormation stack output ApiGWKey
refers to the name of the API key. As of this writing, this API key is associated with a usage plan that allows 2,000 requests per month.
- On the API Gateway console, choose either the
TextClassificationAPI
or the FeedbackAPI
.
- In the navigation pane, choose API Keys.
- Expand the API key section and copy the value.
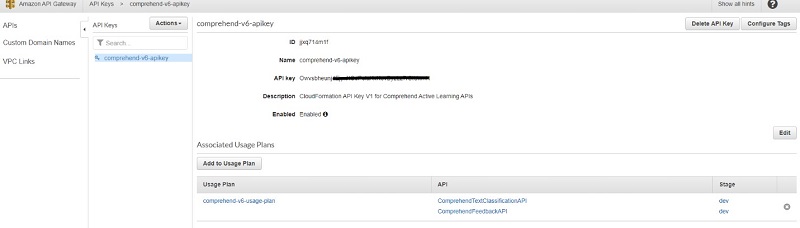
You can manage the usage plan by following the instructions on Create, configure, and test usage plans with the API Gateway console.
You can also add fine-grained authentication and authorization to your APIs. For more information on securing your APIs, see Controlling and managing access to a REST API in API Gateway.
Enable the trigger to start the retraining workflow
The last step of the process is to add a trigger to the S3 bucket that we created earlier to store the human-reviewed output. The trigger invokes the Lambda function that begins the payload transformation from the Amazon A2I human review output format to a CSV format required for training Amazon Comprehend custom classification models.
- Open the Lambda function
HumanReviewTrainingDataTransformerFunction
, created by running the CloudFormation template.
- In the Trigger configuration section, choose S3.
- For Bucket, enter the bucket you created earlier in the step 4 of Create a human review workflow section.
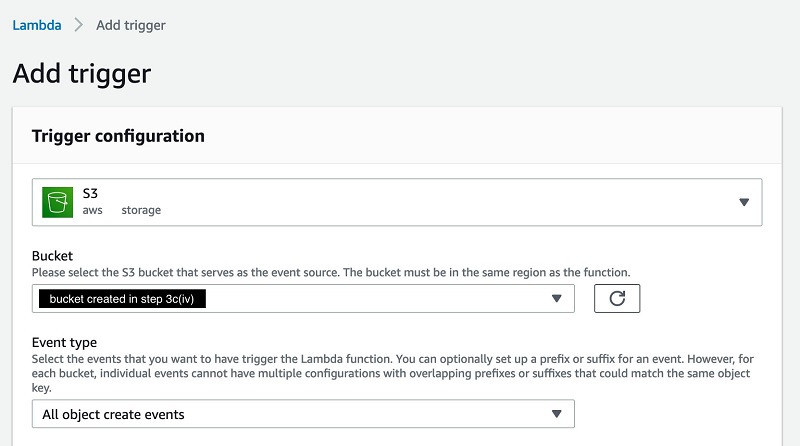
Test the feedback loop
In this section, we walk you through testing your feedback loop, including real-time classification, implicit and explicit feedback, and human review tasks.
Real-time classification
To interact and test these APIs, you need to download Postman.
The API Gateway endpoint receives an unlabeled text document from a client application and internally calls the custom classification endpoint, which returns the predicted label and a confidence score.
- Open Postman and enter the
TextClassificationAPIGateway
URL in POST method.
- In the Headers section, configure the API key: x-api-key : << Your API key >>.
- In the text field, enter the following JSON code (make sure you have JSON selected and enable raw):
{"classifier":"<your custom classifier name>", "sentence":"MS Dhoni retires and a billion people had mixed feelings."}
- Choose Send.
You get a response back with a confidence score and class, as seen in the following screenshot.
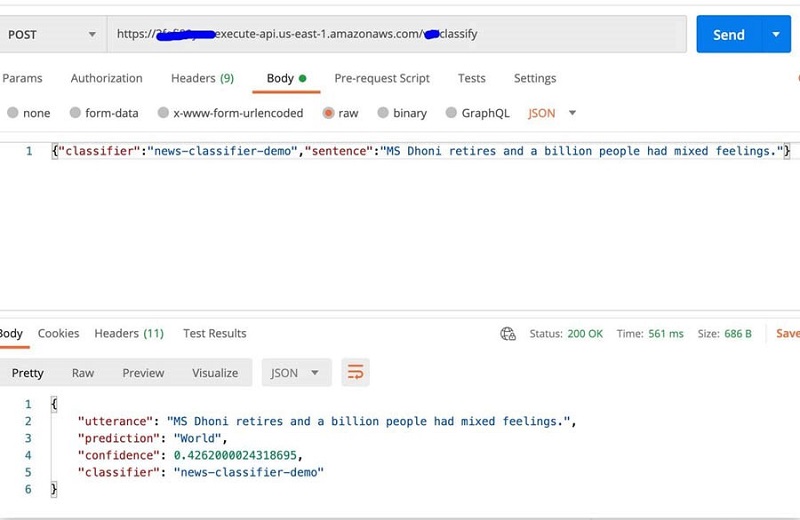
Implicit feedback
When the endpoint returns the classification and the confidence score during the real-time classification, you can route all the instances where the confidence score doesn’t meet the threshold to human review. This type of feedback is called implicit feedback. For this post, we set the threshold as 0.5 as an input to the CloudFormation stack parameter.
You can change this threshold when deploying the CloudFormation template based on your needs.
Explicit feedback
The explicit feedback comes from the end users of the application that uses the custom classification feature. This type of feedback comprises the instances of text where the user wasn’t happy with the prediction. You can send the predicted label by the model’s explicit feedback through the following methods:
- Real time through an API, which is usually triggered through a like/dislike button on a UI
- Batch process, where a file with a collection of misclassified utterances is put together based on a user survey conducted by the customer outreach team
Invoke the explicit real-time feedback loop
To test the Feedback API, complete the following steps:
- Open Postman and enter the
FeedbackAPIGatewayID
value from your CloudFormation stack output in POST method.
- In the Headers section, configure the API key:
x-api-key
: << Your API key >>.
- In the text field, enter the following JSON code (for
classifier
, enter the classifier you created, such as news-classifier-demo
, and make sure you have JSON selected and enable raw):
{"classifier":"<your custom classifier name>","sentence":"Sachin is Indian Cricketer."}
- Choose Send.
We recommend that you submit at least four test samples that will result in a confidence score lesser than your set threshold.
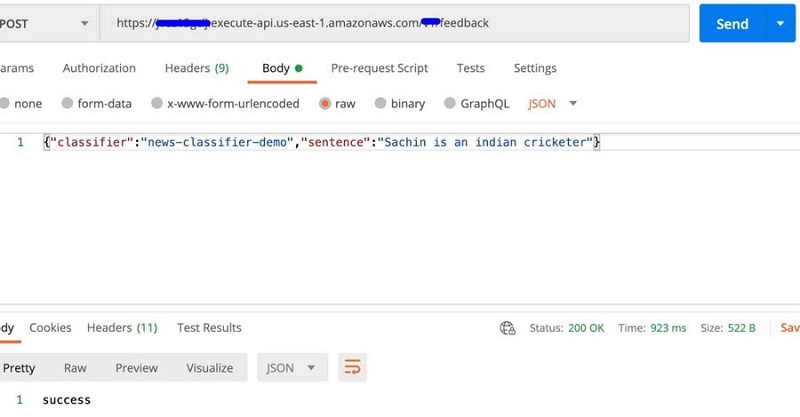
Submit explicit feedback as a batch file
Download the following test feedback JSON file, populate it with your data, and upload it into the BatchUploadS3Bucket created when you deployed your CloudFormation template. We recommend that you submit at least four feedback entries in this file. The following code shows some sample data in the file:
{
"classifier":"news-classifier-demo",
"sentences":[
"US music firms take legal action against 754 computer users alleged to illegally swap music online.",
"A gamer spends $26,500 on a virtual island that exists only in a PC role-playing game."
]
}
Uploading the file triggers the Lambda function that starts your human review loop.
Human review tasks
All the feedback collected through the implicit and explicit methods is sent for human classification. The labeling workforce can include Amazon Mechanical Turk, private teams, or AWS Marketplace vendors. For this post, we create a private workforce. The URL to the labeling portal is located on the SageMaker console, on the Labeling workforces page, on the Private tab.
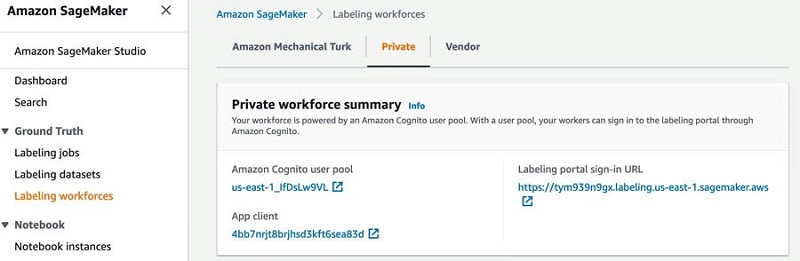
After you log in, you can see the human review tasks assigned to you. Select the task to complete and choose Start working.
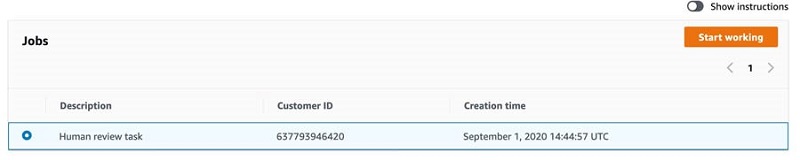
You see the tasks displayed based on the worker template used when creating the human workflow.

After you complete the human classification and submit the tasks, the human-reviewed data is stored in the S3 bucket you configured when creating the human review workflow. This bucket is located under Output location on the workflow details page.
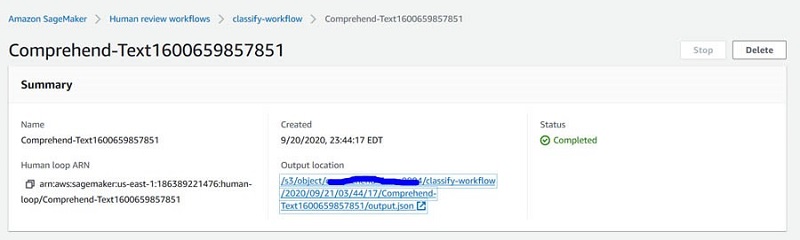
This human-reviewed data is used to retrain the custom classification model to learn newer patterns and improve its overall accuracy. The following screenshot shows the human-annotated output file output.json
in the S3 bucket.

This human-reviewed data is then converted to a custom classification model training data format, and transferred to the training bucket that was created in Part 1 of this series, which starts the Step Functions workflow for retraining models. The process of retraining the models with human-reviewed data, selecting the best model, and automatically deploying the new endpoints completes the active learning workflow.
Cleanup
To remove all resources created throughout this process and prevent additional costs, complete the following steps:
- On the Amazon S3 console, delete the S3 bucket that contains the training dataset.
- On the Amazon Comprehend console, delete the endpoint and the classifier.
- On the Amazon A2I console, delete the human review workflow, worker template, and private workforce.
- On the AWS CloudFormation console, delete the stack you created. (This removes the resources the CloudFormation template created.)
Conclusion
Amazon Comprehend helps you build scalable and accurate natural language processing capabilities without any ML experience. This post provides a reusable pattern and infrastructure for active learning workflows for custom classification models. The feedback pipelines and human review workflow help the custom classifier learn new data patterns continuously. To learn more about automatic model building, selection, and deployment of custom classification models, you can refer to Active learning workflow for Amazon Comprehend custom classification models – Part 1.
For more information, see Custom Classification. You can discover other Amazon Comprehend features and get inspiration from other AWS blog posts about how to use Amazon Comprehend beyond classification.
About the Authors
Shanthan Kesharaju is a Senior Architect in the AWS ProServe team. He helps our customers with AI/ML strategy, architecture, and developing products with a purpose. Shanthan has an MBA in Marketing from Duke University and an MS in Management Information Systems from Oklahoma State University.
Mona Mona is an AI/ML Specialist Solutions Architect based out of Arlington, VA. She works with the World Wide Public Sector team and helps customers adopt machine learning on a large scale. She is passionate about NLP and ML explainability areas in AI/ML.
Joyson Neville Lewis obtained his masters in Information Technology from Rutgers University in 2018. He has worked as a Software/Data engineer before diving into the conversational AI domain in 2019, where he works with companies to connect the dots between business and AI using voice and chatbot solutions. Joyson joined Amazon Web Services in February of 2018 as a Big Data Consultant for the AWS Professional Services team in NYC.
Read More