Companies that operate and maintain a broad range of industrial machinery such as generators, compressors, and turbines are constantly working to improve operational efficiency and avoid unplanned downtime due to component failure. They invest heavily in physical sensors (tags), data connectivity, data storage, and data visualization to monitor the condition of their equipment and get real-time alerts for predictive maintenance.
With machine learning (ML), more powerful technologies have become available that can provide data-driven models that learn from an equipment’s historical data. However, implementing such ML solutions is time-consuming and expensive because it involves managing and setting up complex infrastructure and having the right ML skills. Furthermore, ML applications need human oversight to ensure accuracy with sensitive data, help provide continuous improvements, and retrain models with updated predictions. However, you’re often forced to choose between an ML-only or human-only system. Companies are looking for the best of both worlds—integrating ML systems into your workflow while keeping a human eye on the results to achieve higher precision.
In this post, we show you how you can set up Amazon Lookout for Equipment to train an abnormal behavior detection model using a wind turbine dataset for predictive maintenance, use a human in the loop workflow to review the predictions using Amazon Augmented AI (Amazon A2I), and augment the dataset and retrain the model.
Solution overview
Amazon Lookout for Equipment analyzes the data from your sensors, such as pressure, flow rate, RPMs, temperature, and power, to automatically train a specific ML model based on your data, for your equipment, with no ML expertise required. Amazon Lookout for Equipment uses your unique ML model to analyze incoming sensor data in near-real time and accurately identify early warning signs that could lead to machine failures. This means you can detect equipment abnormalities with speed and precision, quickly diagnose issues, take action to reduce expensive downtime, and reduce false alerts.
Amazon A2I is an ML service that makes it easy to build the workflows required for human review. Amazon A2I brings human review to all developers, removing the undifferentiated heavy lifting associated with building human review systems or managing large numbers of human reviewers, whether running on AWS or not.
To get started with Amazon Lookout for Equipment, we create a dataset, ingest data, train a model, and run inference by setting up a scheduler. After going through these steps, we show you how you can quickly set up a human review process using Amazon A2I and retrain your model with augmented or human reviewed datasets.
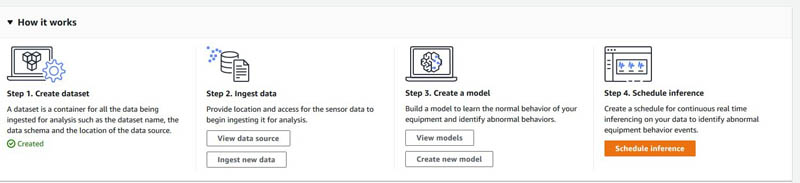
In the accompanying Jupyter notebook, we walk you through the following steps:
- Create a dataset in Amazon Lookout for Equipment.
- Ingest data into the Amazon Lookout for Equipment dataset.
- Train a model in Amazon Lookout for Equipment.
- Run diagnostics on the trained model.
- Create an inference scheduler in Amazon Lookout for Equipment to send a simulated stream of real-time requests.
- Set up an Amazon A2I private human loop and review the predictions from Amazon Lookout for Equipment.
- Retrain your model based on augmented datasets from Amazon A2I.
Architecture overview
The following diagram illustrates our solution architecture.
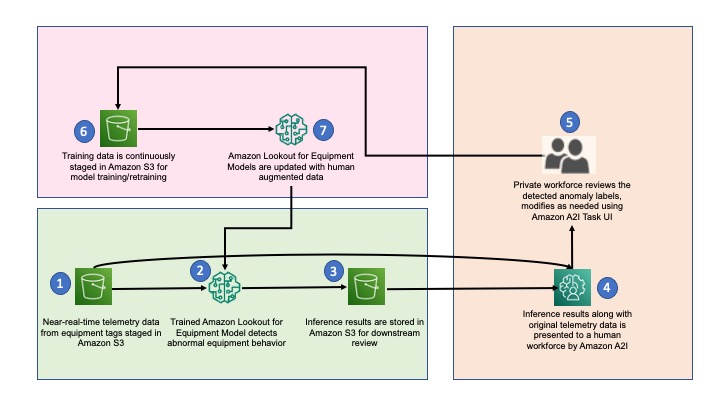
The workflow contains the following steps:
- The architecture assumes that the inference pipeline is built and sensor data is periodically stored in the S3 path for inference inputs. These inputs are stored in CSV format with corresponding timestamps in the file name.
- Amazon Lookout for Equipment wakes up at a prescribed frequency and processes the most recent file from the inference inputs Amazon Simple Storage Service (Amazon S3) path.
- Inference results are stored in the inference outputs S3 path in JSON lines file format. The outputs also contain event diagnostics, which are used for root cause analysis.
- When Amazon Lookout for Equipment detects an anomaly, the inference input and outputs are presented to the private workforce for validation via Amazon A2I.
- A private workforce investigates and validates the detected anomalies and provides new anomaly labels. These labels are stored in a new S3 path.
- Training data is also updated, along with the corresponding new labels, and is staged for subsequent model retraining.
- After enough new labels are collected, a new Amazon Lookout for Equipment model is created, trained, and deployed. The retraining cycle can be repeated for continuous model retraining.
Prerequisites
Before you get started, complete the following steps to set up the Jupyter notebook:
- Create a notebook instance in Amazon SageMaker.
Make sure your SageMaker notebook has the necessary AWS Identity and Access Management (IAM) roles and permissions mentioned in the prerequisite section of the notebook.
- When the notebook is active, choose Open Jupyter.
- On the Jupyter dashboard, choose New, and choose Terminal.
- In the terminal, enter the following code:
cd SageMaker
git clone https://github.com/aws-samples/lookout-for-equipment-demo
- First run the data preparation notebook –
1_data_preparation.ipynb
- Then open the notebook for this blog –
3_integrate_l4e_and_a2i.ipynb
You’re now ready to run the following steps through the notebook cells. Run the setup environment step to set up the necessary Python SDKs and libraries that we use throughout the notebook.
- Provide an AWS Region, create an S3 bucket, and provide details of the bucket in the following code cell:
REGION_NAME = '<your region>'
BUCKET = '<your bucket name>'
PREFIX = 'data/wind-turbine'
Analyze the dataset and create component metadata
In this section, we walk you through how you can preprocess the existing wind turbine data and ingest it for Amazon Lookout for Equipment. Please make sure to run the data preparation notebook prior to running the accompanying notebook for the blog to follow through all the steps in this post. You need a data schema for using your existing historical data with Amazon Lookout for Equipment. The data schema tells Amazon Lookout for Equipment what the data means. Because a data schema describes the data, its structure mirrors that of the data files of the components it describes.
All components must be described in the data schema. The data for each component is contained in a separate CSV file structured as shown in the data schema.
You store the data for each asset’s component in a separate CSV file using the following folder structure:
S3 bucket > Asset_name > Component 1 > Component1.csv
Go to the notebook section Pre-process and Load Datasets and run the following cell to inspect the data:
import pandas as pd
turbine_id = 'R80711'
df = pd.read_csv(f'../data/wind-turbine/interim/{turbine_id}.csv', index_col = 'Timestamp')
df.head()
The following screenshot shows our output.
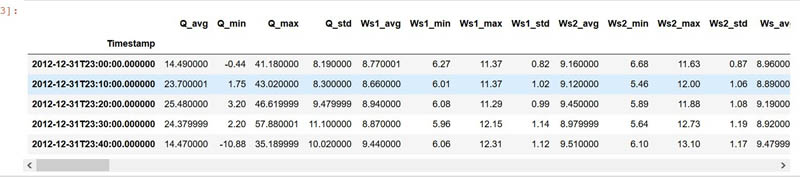
Now we create components map to create a dataset expected by Amazon Lookout for Equipment for ingest. Run the notebook cells under the section Create the Dataset Component Map to create a component map and generate a CSV file for ingest.
Create the Amazon Lookout for Equipment dataset
We use Amazon Lookout for Equipment Create Dataset APIs to create a dataset and provide the component map we created in the previous step as an input. Run the following notebook cell to create a dataset:
ROLE_ARN = sagemaker.get_execution_role()
# REGION_NAME = boto3.session.Session().region_name
DATASET_NAME = 'wind-turbine-train-dsv2-PR'
MODEL_NAME = 'wind-turbine-PR-v1'
lookout_dataset = lookout.LookoutEquipmentDataset(
dataset_name=DATASET_NAME,
component_fields_map=DATASET_COMPONENT_FIELDS_MAP,
region_name=REGION_NAME,
access_role_arn=ROLE_ARN
)
pp = pprint.PrettyPrinter(depth=5)
pp.pprint(eval(lookout_dataset.dataset_schema))
lookout_dataset.create()
You get the following output:
Dataset "wind-turbine-train-dsv2-PR" does not exist, creating it...
{'DatasetName': 'wind-turbine-train-dsv2-PR',
'DatasetArn': 'arn:aws:lookoutequipment:ap-northeast-2:<aws-account>:dataset/wind-turbine-train-dsv2-PR/8325802a-9bb7-48fb-804b-ab9f5b79f49d',
'Status': 'CREATED',
'ResponseMetadata': {'RequestId': '52dc754c-84da-4a8c-aaef-1908e4348837',
'HTTPStatusCode': 200,
'HTTPHeaders': {'x-amzn-requestid': '52dc754c-84da-4a8c-aaef-1908e4348837',
'content-type': 'application/x-amz-json-1.0',
'content-length': '203',
'date': 'Thu, 25 Mar 2021 21:18:29 GMT'},
'RetryAttempts': 0}}
Alternatively, you can go to the Amazon Lookout for Equipment console to view the dataset.
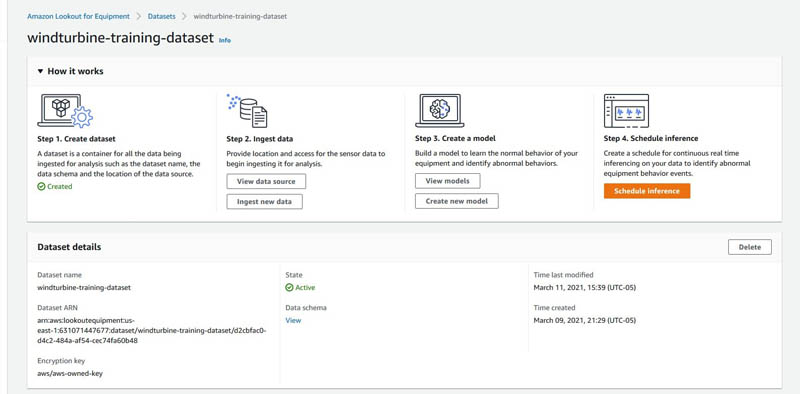
You can choose View under Data schema to view the schema of the dataset. You can choose Ingest new data to start ingesting data through the console, or you can use the APIs shown in the notebook to do the same using Python Boto3 APIs.
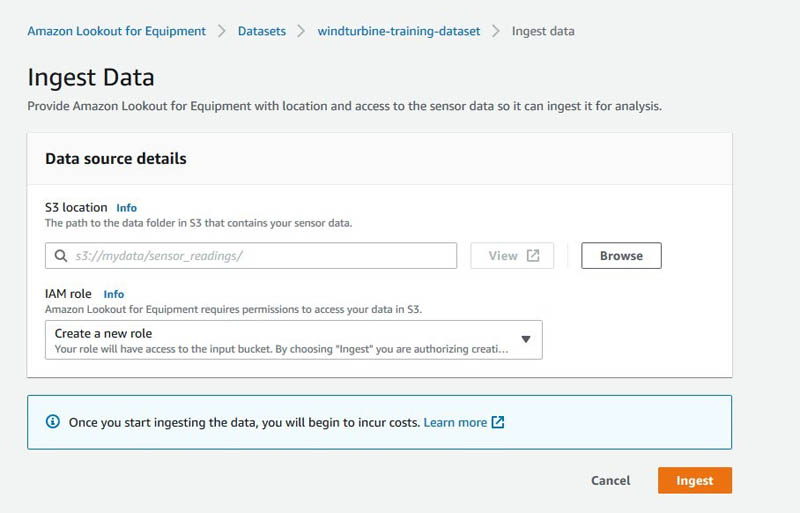
Run the notebook cells to ingest the data. When ingestion is complete, you get the following response:
=====Polling Data Ingestion Status=====
2021-03-25 21:18:45 | IN_PROGRESS
2021-03-25 21:19:46 | IN_PROGRESS
2021-03-25 21:20:46 | IN_PROGRESS
2021-03-25 21:21:46 | IN_PROGRESS
2021-03-25 21:22:46 | SUCCESS
Now that we have preprocessed the data and ingested the data into Amazon Lookout for Equipment, we move on to the training steps. Alternatively, you can choose Ingest data.
Label your dataset using the SageMaker labeling workforce
If you don’t have an existing labeled dataset available to directly use with Amazon Lookout for Equipment, create a custom labeling workflow. This may be relevant in a use case in which, for example, a company wants to build a remote operating facility where alerts from various operations are sent to the central facility for the SMEs to review and update. For a sample crowd HTML template for your labeling UI, refer to our GitHub repository.
The following screenshot shows an example of what the sample labeling UI looks like.
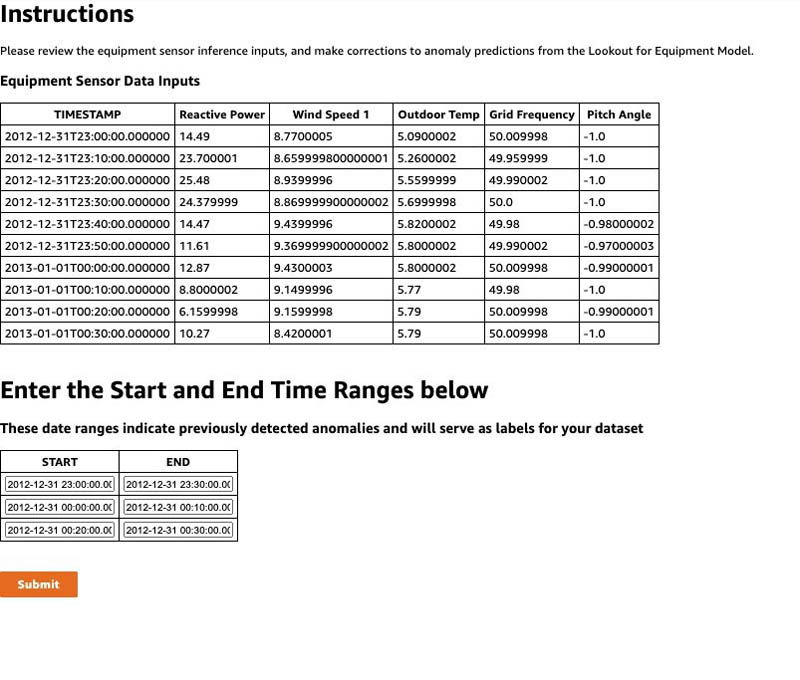
For this post, we use the labels that came with the dataset for training. If you want to use the label file you created for your actual training in the next step, you need to copy the label file to an S3 bucket and provide the location in the training configuration.
Create a model in Amazon Lookout for Equipment
We walk you through the following steps in this section:
- Prepare the model parameters and split data into test and train sets
- Train the model using Amazon Lookout for Equipment APIs
- Get diagnostics for the trained model
Prepare the model parameters and split the data
In this step, we split the datasets into test and train, prepare labels, and start the training using the notebook. Run the notebook code Split train and test to split the dataset into an 80/20 split for training and testing, respectively. Then run the prepare labels code and move on to setting up training config, as shown in the following code:
# Prepare the model parameters:
lookout_model = lookout.LookoutEquipmentModel(model_name=MODEL_NAME,
dataset_name=DATASET_NAME,
region_name=REGION_NAME)
# Set the training / evaluation split date:
lookout_model.set_time_periods(evaluation_start,
evaluation_end,
training_start,
training_end)
# Set the label data location:
lookout_model.set_label_data(bucket=BUCKET,
prefix=PREFIX+'/labelled_data/',
access_role_arn=ROLE_ARN)
# This sets up the rate the service will resample the data before
# training:
lookout_model.set_target_sampling_rate(sampling_rate='PT10M')
In the preceding code, we set up model training parameters such as time periods, label data, and target sampling rate for our model. For more information about these parameters, see CreateModel.
Train model
After setting these model parameters, you need to run the following train model API to start training your model with your dataset and the training parameters:
You get the following response:
{'ModelArn': 'arn:aws:lookoutequipment:ap-northeast-2:<accountid>:model/wind-turbine-PR-v1/fac217a9-8855-4931-95f9-dd47f0af1ec5',
'Status': 'IN_PROGRESS',
'ResponseMetadata': {'RequestId': '3d385895-c62e-4126-9622-38f0ebed9715',
'HTTPStatusCode': 200,
'HTTPHeaders': {'x-amzn-requestid': '3d385895-c62e-4126-9622-38f0ebed9715',
'content-type': 'application/x-amz-json-1.0',
'content-length': '152',
'date': 'Thu, 25 Mar 2021 21:27:05 GMT'},
'RetryAttempts': 0}}
Alternatively, you can go to the Amazon Lookout for Equipment console and monitor the training after you create the model.
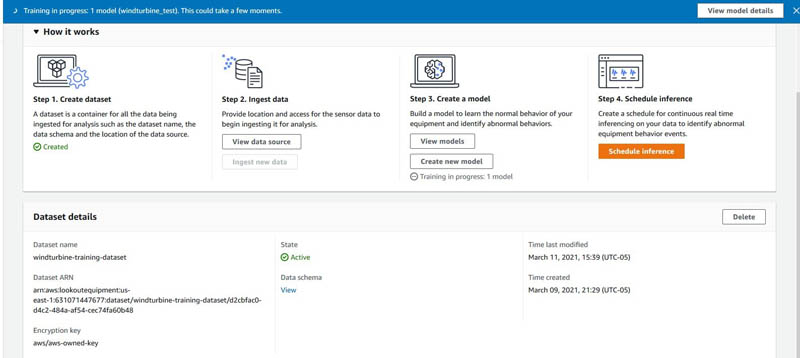
The sample turbine dataset we provide in our example has millions of data points. Training takes approximately 2.5 hours.
Evaluate the trained model
After a model is trained, Amazon Lookout for Equipment evaluates its performance and displays the results. It provides an overview of the performance and detailed information about the abnormal equipment behavior events and how well the model performed when detecting those. With the data and failure labels that you provided for training and evaluating the model, Amazon Lookout for Equipment reports how many times the model’s predictions were true positives. It also reports the average forewarning time across all true positives. Additionally, it reports the false positive results generated by the model, along with the duration of the non-event.
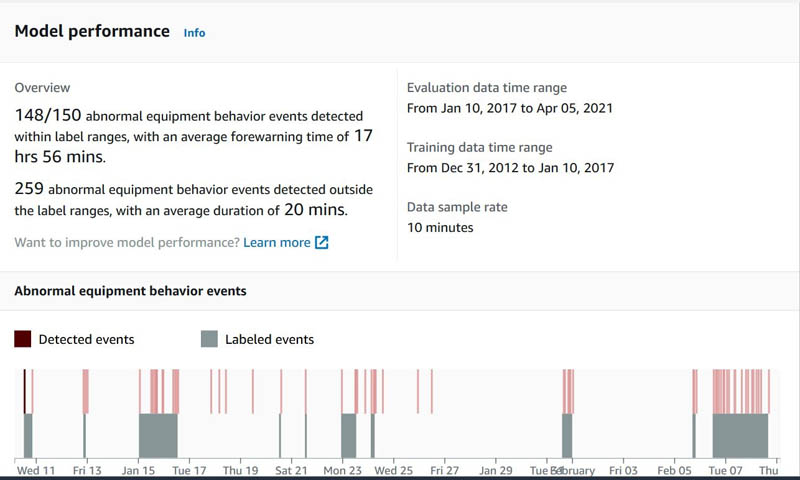
For more information about performance evaluation, see Evaluating the output.
Review training diagnostics
Run the following code to generate the training diagnostics. Refer to the accompanying notebook for the complete code block to run for this step.
LookoutDiagnostics = lookout.LookoutEquipmentAnalysis(model_name=MODEL_NAME, tags_df=df, region_name=REGION_NAME)
LookoutDiagnostics.set_time_periods(evaluation_start, evaluation_end, training_start, training_end)
predicted_ranges = LookoutDiagnostics.get_predictions()
The results returned show the percentage contribution of each feature towards the abnormal equipment prediction for the corresponding date range.
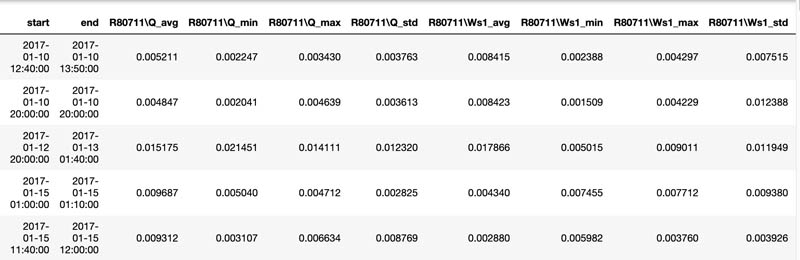
Create an inference scheduler in Amazon Lookout for Equipment
In this step, we show you how the CreateInferenceScheduler API creates a scheduler and starts it—this starts costing you right away. Scheduling an inference is setting up a continuous real-time inference plan to analyze new measurement data. When setting up the scheduler, you provide an S3 bucket location for the input data, assign it a delimiter between separate entries in the data, set an offset delay if desired, and set the frequency of inference. You must also provide an S3 bucket location for the output data. Run the following notebook section to run inference on the model to create an inference scheduler:
scheduler = lookout.LookoutEquipmentScheduler(
scheduler_name=INFERENCE_SCHEDULER_NAME,
model_name=MODEL_NAME_FOR_CREATING_INFERENCE_SCHEDULER,
region_name=REGION_NAME
)
scheduler_params = {
'input_bucket': INFERENCE_DATA_SOURCE_BUCKET,
'input_prefix': INFERENCE_DATA_SOURCE_PREFIX,
'output_bucket': INFERENCE_DATA_OUTPUT_BUCKET,
'output_prefix': INFERENCE_DATA_OUTPUT_PREFIX,
'role_arn': ROLE_ARN_FOR_INFERENCE,
'upload_frequency': DATA_UPLOAD_FREQUENCY,
'delay_offset': DATA_DELAY_OFFSET_IN_MINUTES,
'timezone_offset': INPUT_TIMEZONE_OFFSET,
'component_delimiter': COMPONENT_TIMESTAMP_DELIMITER,
'timestamp_format': TIMESTAMP_FORMAT
}
scheduler.set_parameters(**scheduler_params)
After you create an inference scheduler, the next step is to create some sample datasets for inference.
Prepare the inference data
Run through the notebook steps to prepare the inference data. Let’s load the tags description; this dataset comes with a data description file. From here, we can collect the list of components (subsystem column) if required. We use the tag metadata from the data descriptions as a point of reference for our interpretation. We use the tag names to construct a list that Amazon A2I uses. For more details, refer to the section Set up Amazon A2I to review predictions from Amazon Lookout for Equipment in this post.
To build our sample inference dataset, we extract the last 2 hours of data from the evaluation period of the original time series. Specifically, we create three CSV files containing simulated real-time tags for our turbine 10 minutes apart. These are all stored in Amazon S3 in the inference-a2i folder
. Now that we’ve prepared the data, create the scheduler by running the following code:
create_scheduler_response = scheduler.create()
You get the following response:
===== Polling Inference Scheduler Status =====
Scheduler Status: PENDING
Scheduler Status: RUNNING
===== End of Polling Inference Scheduler Status =====
Alternatively, on the Amazon Lookout for Equipment console, go to the Inference schedule settings section of your trained model and set up a scheduler by providing the necessary parameters.
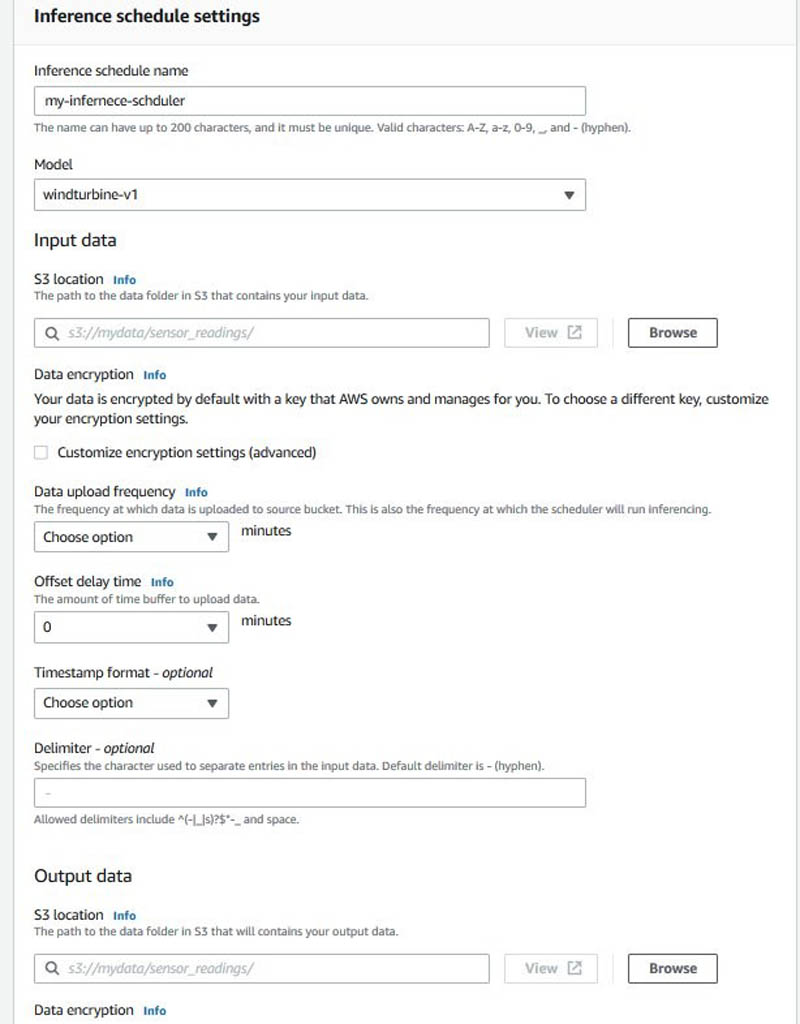
Get inference results
Run through the notebook steps List inference executions to get the run details from the schedule you created in the previous step. Wait 5–15 minutes for the scheduler to run its first inference. When it’s complete, we can use the ListInferenceExecution API for our current inference scheduler. The only mandatory parameter is the scheduler name.
You can also choose a time period for which you want to query inference runs. If you don’t specify it, all runs for an inference scheduler are listed. If you want to specify the time range, you can use the following code:
START_TIME_FOR_INFERENCE_EXECUTIONS = datetime.datetime(2010,1,3,0,0,0)END_TIME_FOR_INFERENCE_EXECUTIONS = datetime.datetime(2010,1,5,0,0,0)
This code means that the runs after 2010-01-03 00:00:00
and before 2010-01-05 00:00:00
are listed.
You can also choose to query for runs in a particular status, such as IN_PROGRESS
, SUCCESS
, and FAILED
:
START_TIME_FOR_INFERENCE_EXECUTIONS = None
END_TIME_FOR_INFERENCE_EXECUTIONS = None
EXECUTION_STATUS = None
execution_summaries = []
while len(execution_summaries) == 0:
execution_summaries = scheduler.list_inference_executions(
start_time=START_TIME_FOR_INFERENCE_EXECUTIONS,
end_time=END_TIME_FOR_INFERENCE_EXECUTIONS,
execution_status=EXECUTION_STATUS
)
if len(execution_summaries) == 0:
print('WAITING FOR THE FIRST INFERENCE EXECUTION')
time.sleep(60)
else:
print('FIRST INFERENCE EXECUTEDn')
break
execution_summaries
You get the following response:
{'ModelName': 'wind-turbine-PR-v1',
'ModelArn': 'arn:aws:lookoutequipment:ap-northeast-2:<aws-account>:model/wind-turbine-PR-v1/fac217a9-8855-4931-95f9-dd47f0af1ec5',
'InferenceSchedulerName': 'wind-turbine-scheduler-a2i-PR-v10',
'InferenceSchedulerArn': 'arn:aws:lookoutequipment:ap-northeast-2:<aws-account>:inference-scheduler/wind-turbine-scheduler-a2i-PR-v10/e633c39d-a4f9-49f6-8248-7594349db2d0',
'ScheduledStartTime': datetime.datetime(2021, 3, 29, 15, 35, tzinfo=tzlocal()),
'DataStartTime': datetime.datetime(2021, 3, 29, 15, 30, tzinfo=tzlocal()),
'DataEndTime': datetime.datetime(2021, 3, 29, 15, 35, tzinfo=tzlocal()),
'DataInputConfiguration': {'S3InputConfiguration': {'Bucket': '<your s3 bucket>',
'Prefix': 'data/wind-turbine/inference-a2i/input/'}},
'DataOutputConfiguration': {'S3OutputConfiguration': {'Bucket': '<your s3 bucket>',
'Prefix': 'data/wind-turbine/inference-a2i/output/'}},
'CustomerResultObject': {'Bucket': '<your s3 bucket>',
'Key': 'data/wind-turbine/inference-a2i/output/2021-03-29T15:30:00Z/results.jsonl'},
'Status': 'SUCCESS'}]
Get actual prediction results
After each successful inference, a JSON file is created in the output location of your bucket. Each inference creates a new folder with a single results.jsonl
file in it. You can run through this section in the notebook to read these files and display their content.
The following screenshot shows the results.
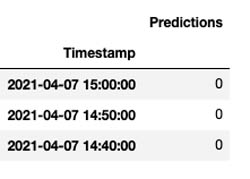
Stop the inference scheduler
Make sure to stop the inference scheduler; we don’t need it for the rest of the steps in this post. However, as part of your solution, the inference scheduler should be running to ensure real-time inference for your equipment continues. Run through this notebook section to stop the inference scheduler.
Set up Amazon A2I to review predictions from Amazon Lookout for Equipment
Now that inference is complete, let’s understand how to set up a UI to review the inference results and update it, so we can send it back to Amazon Lookout for Equipment for retraining the model. In this section, we show how to use the Amazon A2I custom task type to integrate with Amazon Lookout for Equipment through the walkthrough notebook to set up a human in the loop process. It includes the following steps:
- Create a human task UI
- Create a workflow definition
- Send predictions to Amazon A2I human loops
- Sign in to the worker portal and annotate Amazon Lookout for Equipment inference predictions
Follow the steps provided in the notebook to initialize Amazon A2I APIs. Make sure to set up the bucket name in the initialization block where you want your Amazon A2I output:
a2ibucket = '<your bucket>'
You also need to create a private workforce and provide a work team ARN in the initialize step.
On the SageMaker console, create a private workforce. After you create the private workforce, find the workforce ARN and enter the ARN in the notebook:
WORKTEAM_ARN = 'your private workforce team ARN'
Create the human task UI
You now create a human task UI resource, giving a UI template in liquid HTML. You can download the provided template and customize it. This template is rendered to the human workers whenever a human loop is required. For over 70 pre-built UIs, see the amazon-a2i-sample-task-uis GitHub repo. We also provide this template in our GitHub repo.
You can use this template to create a task UI either via the console or by running the following code in the notebook:
def create_task_ui():
response = sagemaker_client.create_human_task_ui(
HumanTaskUiName=taskUIName,
UiTemplate={'Content': template})
return response
Create a human review workflow definition
Workflow definitions allow you to specify the following:
- The worker template or human task UI you created in the previous step.
- The workforce that your tasks are sent to. For this post, it’s the private workforce you created in the prerequisite steps.
- The instructions that your workforce receives.
This post uses the Create Flow Definition
API to create a workflow definition. Run the following cell in the notebook:
create_workflow_definition_response = sagemaker_client.create_flow_definition(
FlowDefinitionName= flowDefinitionName,
RoleArn=role,
HumanLoopConfig= {
"WorkteamArn": WORKTEAM_ARN,
"HumanTaskUiArn": humanTaskUiArn,
"TaskCount": 1,
"TaskDescription": "Review the contents and select correct values as indicated",
"TaskTitle": "Equipment Condition Review"
},
OutputConfig={
"S3OutputPath" : OUTPUT_PATH
}
)
flowDefinitionArn = create_workflow_definition_response['FlowDefinitionArn']
Send predictions to Amazon A2I human loops
We create an item list from the Pandas DataFrame where we have the Amazon Lookout for Equipement output saved. Run the following notebook cell to create a list of items to send for review:
NUM_TO_REVIEW = 5 # number of line items to review
dftimestamp = sig_full_df['Timestamp'].astype(str).to_list()
dfsig001 = sig_full_df['Q_avg'].astype(str).to_list()
dfsig002 = sig_full_df['Ws1_avg'].astype(str).to_list()
dfsig003 = sig_full_df['Ot_avg'].astype(str).to_list()
dfsig004 = sig_full_df['Nf_avg'].astype(str).to_list()
dfsig046 = sig_full_df['Ba_avg'].astype(str).to_list()
sig_list = [{'timestamp': dftimestamp[x], 'reactive_power': dfsig001[x], 'wind_speed_1': dfsig002[x], 'outdoor_temp': dfsig003[x], 'grid_frequency': dfsig004[x], 'pitch_angle': dfsig046[x]} for x in range(NUM_TO_REVIEW)]
sig_list
Run the following code to create a JSON input for the Amazon A2I loop. This contains the lists that are sent as input to the Amazon A2I UI displayed to the human reviewers.
ip_content = {"signal": sig_list,
'anomaly': ano_list
}
Run the following notebook cell to call the Amazon A2I API to start the human loop:
import json
humanLoopName = str(uuid.uuid4())
start_loop_response = a2i.start_human_loop(
HumanLoopName=humanLoopName,
FlowDefinitionArn=flowDefinitionArn,
HumanLoopInput={
"InputContent": json.dumps(ip_content)
}
)
You can check the status of human loop by running the next cell in the notebook.
Annotate the results via the worker portal
Run the following notebook cell to get a login link to navigate to the private workforce portal:
workteamName = WORKTEAM_ARN[WORKTEAM_ARN.rfind('/') + 1:]
print("Navigate to the private worker portal and do the tasks. Make sure you've invited yourself to your workteam!")
print('https://' + sagemaker_client.describe_workteam(WorkteamName=workteamName)['Workteam']['SubDomain'])
You’re redirected to the Amazon A2I console. Select the human review job and choose Start working. After you review the changes and make corrections, choose Submit.
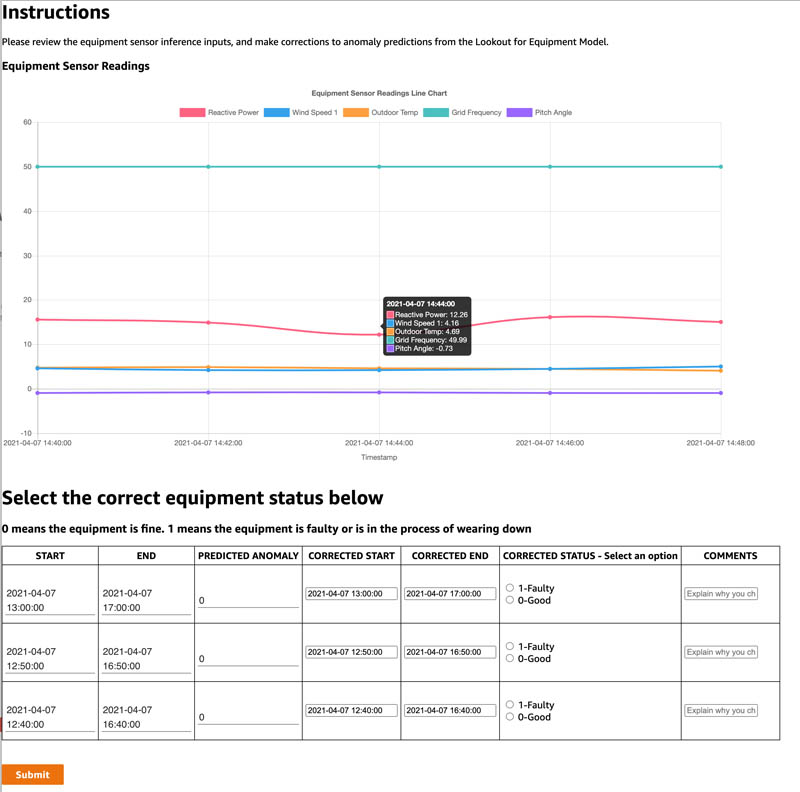
You can evaluate the results store in Amazon S3.
Evaluate the results
When the labeling work is complete, your results should be available in the S3 output path specified in the human review workflow definition. The human answers are returned and saved in the JSON file. Run the notebook cell to get the results from Amazon S3:
import re
import pprint
pp = pprint.PrettyPrinter(indent=4)
json_output = ''
for resp in completed_human_loops:
splitted_string = re.split('s3://' + a2ibucket + '/', resp['HumanLoopOutput']['OutputS3Uri'])
print(splitted_string[1])
output_bucket_key = splitted_string[1]
response = s3.get_object(Bucket=a2ibucket, Key=output_bucket_key)
content = response["Body"].read()
json_output = json.loads(content)
pp.pprint(json_output)
print('n')
You get a response with human reviewed answers and flow-definition. Refer to the notebook to get the complete response.
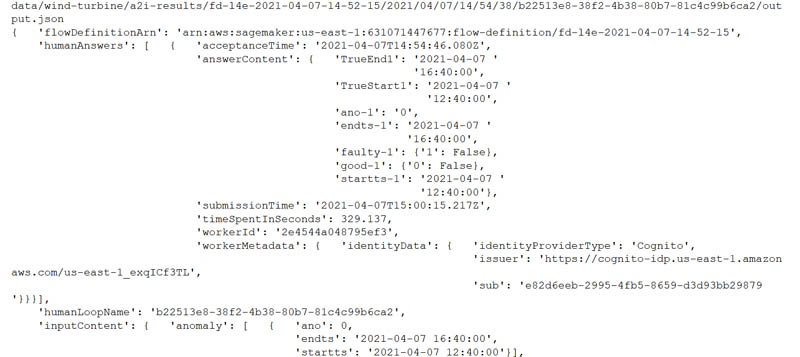
Model retraining based on augmented datasets from Amazon A2I
Now we take the Amazon A2I output, process it, and send it back to Amazon Lookout for Equipment to retrain our model based on the human corrections. Refer to the accompanying notebook for all the steps to complete in this section. Let’s look at the last few entries of our original label file:
labels_df = pd.read_csv(os.path.join(LABEL_DATA, 'labels.csv'), header=None)
labels_df[0] = pd.to_datetime(labels_df[0])
labels_df[1] = pd.to_datetime(labels_df[1])
labels_df.columns = ['start', 'end']
labels_df.tail()
The following screenshot shows the labels file.
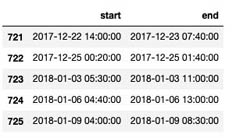
Update labels with new date ranges
Now let’s update our existing labels dataset with the new labels we received from the Amazon A2I human review process:
faulty = False
a2i_lbl_df = labels_df
x = json_output['humanAnswers'][0]
row_df = pd.DataFrame(columns=['rownr'])
tslist = {}
# Let's first check if the users mark equipment as faulty and if so get those row numbers into a dataframe
for i in json_output['humanAnswers']:
print("checking equipment review...")
x = i['answerContent']
for idx, key in enumerate(x):
if "faulty" in key:
if str(x.get(key)).split(':')[1].lstrip().strip('}') == "True": # faulty equipment selected
faulty = True
row_df.loc[len(row_df.index)] = [key.split('-')[1]]
print("found faulty equipment in row: " + key.split('-')[1])
# Now we will get the date ranges for the faulty choices
for idx,k in row_df.iterrows():
x = json_output['humanAnswers'][0]
strchk = "TrueStart"+k['rownr']
endchk = "TrueEnd"+k['rownr']
for i in x['answerContent']:
if i == strchk:
tslist[i] = x['answerContent'].get(i)
if i == endchk:
tslist[i] = x['answerContent'].get(i)
# And finally let's add it to our new a2i labels dataset
for idx,k in row_df.iterrows():
x = json_output['humanAnswers'][0]
strchk = "TrueStart"+k['rownr']
endchk = "TrueEnd"+k['rownr']
a2i_lbl_df.loc[len(a2i_lbl_df.index)] = [tslist[strchk], tslist[endchk]]
You get the following response:
checking equipment review...
found faulty equipment in row: 1
found faulty equipment in row: 2
The following screenshot shows the updated labels file.
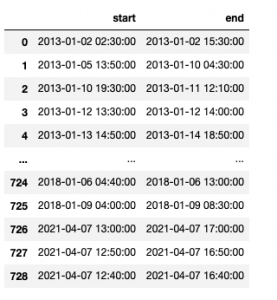
Let’s upload the updated labels data to a new augmented labels file:
a2i_label_s3_dest_path = f's3://{BUCKET}/{PREFIX}/augmented-labelled-data/labels.csv'
!aws s3 cp $a2i_label_src_fname $a2i_label_s3_dest_path
Update the training dataset with new measurements
We now update our original training dataset with the new measurement range based on what we got back from Amazon A2I. Run the following code to load the original dataset to a new DataFrame that we use to append our augmented data. Refer to the accompanying notebook for all the steps required.
turbine_id = 'R80711'
file = '../data/wind-turbine/final/training-data/'+turbine_id+'/'+turbine_id+'.csv'
newdf = pd.read_csv(file, index_col='Timestamp')
newdf.head()
The following screenshot shows our original training dataset snapshot.
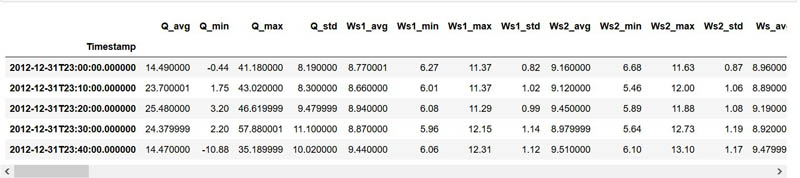
Now we use the updated training dataset with the simulated inference data we created earlier, in which the human reviewers indicated that they found faulty equipment when running the inference. Run the following code to modify the index of the simulated inference dataset to reflect a 10-minute duration for each reading:
sig_full_df = sig_full_df.set_index('Timestamp')
tm = pd.to_datetime('2021-04-05 20:30:00')
print(tm)
new_index = pd.date_range(
start=tm,
periods=sig_full_df.shape[0],
freq='10min'
)
sig_full_df.index = new_index
sig_full_df.index.name = 'Timestamp'
sig_full_df = sig_full_df.reset_index()
sig_full_df['Timestamp'] = pd.to_datetime(sig_full_df['Timestamp'], errors='coerce')
Run the following code to append the simulated inference dataset to the original training dataset:
newdf = newdf.reset_index()
newdf = pd.concat([newdf,sig_full_df])
The simulated inference data with the recent timestamp is appended to the end of the training dataset. Now let’s create a CSV file and copy the data to the training channel in Amazon S3:
TRAIN_DATA_AUGMENTED = os.path.join(TRAIN_DATA,'augmented')
os.makedirs(TRAIN_DATA_AUGMENTED, exist_ok=True)
newdf.to_csv('../data/wind-turbine/final/training-data/augmented/'+turbine_id+'.csv')
!aws s3 sync $TRAIN_DATA_AUGMENTED s3://$BUCKET/$PREFIX/training_data/augmented
Now we update the components map with this augmented dataset, reload the data into Amazon Lookout for Equipment, and retrain this training model with this dataset. Refer to the accompanying notebook for the detailed steps to retrain the model.
Conclusion
In this post, we walked you through how to use Amazon Lookout for Equipment to train a model to detect abnormal equipment behavior with a wind turbine dataset, review diagnostics from the trained model, review the predictions from the model with a human in the loop using Amazon A2I, augment our original training dataset, and retrain our model with the feedback from the human reviews.
With Amazon Lookout for Equipment and Amazon A2I, you can set up a continuous prediction, review, train, and feedback loop to audit predictions and improve the accuracy of your models.
Please let us know what you think of this solution and how it applies to your industrial use case. Check out the GitHub repo for full resources to this post. Visit the webpages to learn more about Amazon Lookout for Equipment and Amazon Augmented AI. We look forward to hearing from you. Happy experimentation!
About the Authors
Dastan Aitzhanov is a Solutions Architect in Applied AI with Amazon Web Services. He specializes in architecting and building scalable cloud-based platforms with an emphasis on machine learning, internet of things, and big data-driven applications. When not working, he enjoys going camping, skiing, and spending time in the great outdoors with his family
Prem Ranga is an Enterprise Solutions Architect based out of Atlanta, GA. He is part of the Machine Learning Technical Field Community and loves working with customers on their ML and AI journey. Prem is passionate about robotics, is an Autonomous Vehicles researcher, and also built the Alexa-controlled Beer Pours in Houston and other locations.
Mona Mona is a Senior AI/ML Specialist Solutions Architect based out of Arlington, VA. She works with public sector customers, and helps them adopt machine learning on a large scale. She is passionate about NLP and ML explainability areas in AI/ML.
Baris Yasin is a Solutions Architect at AWS. He’s passionate about AI/ML & Analytics technologies and helping startup customers solve challenging business and technical problems with AWS.
Read More