This blog post was co-authored by Arbi Tamrazian, Director of Data Science and Machine Learning at Fox Sports
FOX Sports is the sports television arm of FOX Network. The company used machine learning (ML) and Amazon SageMaker to streamline the production of relevant in-game storylines for commentators to use during live broadcasts.
“We collaborated with the Amazon Machine Learning Solutions Lab to build a natural language generation (NLG) engine that automatically produces sports narratives for commentators to use during games. Leveraging Amazon SageMaker, the Amazon Machine Learning Solutions Lab developed a model pipeline that generates natural-sounding sports narratives from a ML model trained on billions of English texts and sports stats snippets. In just a few short weeks, the NLG solution achieved BLEU scores above 99% on unseen Fox Sports testing dataset, significantly improving the readability of narratives compared to test benchmarks. Standardizing our ML workloads on Amazon SageMaker will enable our broadcasters to engage fans with pertinent gameday stories, in real-time.” – Arbi Tamrazian, Director of Data Science and Machine Learning, Fox Sports
Objectives
As viewers may have noticed, sports broadcasters are increasingly sharing statistical insights throughout the game to tell a richer story for the audience. Thanks to an abundance of data and advanced stats such as NFL Next Gen Stats powered by AWS, broadcasters can quickly tell stories and make comparisons between teams and players to keep viewers engaged.
Due to the fast-paced nature of many games, broadcasters rely on template-generated narratives to speak about in-game statistics in real time. These rule-based templates “stitch” tabular information and create narratives with fixed sentence structures that sometimes sound rigid and are hard to understand. It’s also becoming harder to build and maintain templates to keep up the pace with the introduction of new statistics.
To improve the broadcasting experience, Fox Sports turns to AWS and its artificial intelligence technologies to convert their real-time data into easy-to-understand narratives for commentators and audiences. The Amazon ML Solutions Lab partnered with Fox Sports to design and implement an end-to-end ML system using natural language generation (NLG), a technique to generate natural language descriptions from structured data. The objective of the partnership is to produce more natural-sounding narratives compared to the rule-based templates in a scalable fashion. The system enables Fox Sports to expand their rule-based generation engine into an ML solution. The model is trained to understand the semantic meaning of inputs, and can be expanded to new statistics and other sports by fine-tuning with a few hundred sample narratives.
In this post, we walk you through how to fine-tune a pretrained language model to generate sentences similar to those from rule-based templates. In addition, we show how to use different NLG techniques to make the sentences sound more natural, which leads to improved fan experiences and reduced cost in building and maintaining templates.
Template for an ML approach
The first phase of the NLG-based narrative generation solution relies on tabular features, including player and team names, metrics, and game situations. These features are paired with their target sequences, which are generated using predefined rule-based templates. The goal here is to use NLG to take the tabular features and generate candidate narratives containing all the relevant information.
Dataset
To train this model, we use a dataset synthetically generated by Fox Sports using the current rule-based methodology. The dataset is generated by permuting different statistics, feature values, and team and player names, and includes more than 57,000 samples of 8 features. For each sample, we have the narrative generated from a rule-based template as our target. We randomly shuffle and divide the dataset into training, validation, and testing sets based on an 80/10/10 split for training and fine-tuning our models.
The following table shows examples of the raw data used in this experiment—each row represents a record, and each column represents the relevant information associated with the record, including the statistic, values for the statistic, situation that the statistic is calculated upon, and more. For this post, we replace actual team and players names with generic names: team Bobcats and player John Peccy.
Statistic |
Situation |
Value |
Time frame |
Rank |
Rank Order |
Population |
Team name / Player name |
rec_td |
stadium_retractable_dome |
5 |
season |
7 |
True |
32 |
Bobcats |
qbkd |
score_differential_trailing |
3 |
season |
2 |
False |
190 |
John Peccy |
For each row, the raw tabular features are concatenated to form a text sequence. The following table shows examples of the text sequences used as input and the associated narrative from the rule-based template as output.
Template input |
Template output |
rec_td stadium_retractable_dome 5 season 7 TRUE 32 Bobcats |
Bobcats’ 5 caught passes for touchdowns when playing in a retractable roof is the 7th highest out of 32 in the NFL this season. |
qbkd score_differential_trailing 3 season 2 FALSE 190 John Peccy |
John Peccy’s 3 credited QB knockdowns when trailing is the 2nd lowest out of 190 in the NFL this season. |
Methods and metrics
The task of translating tabular features to natural sentences is a subtask of natural language generation. Because transfer learning has proved effective at this task, we utilize a language model called T5 (Text-To-Text Transfer Transformer), which was pretrained on the open-source dataset C4 (Colossal Clean Crawled Corpus). T5 achieves state-of-the-art results on many NLP benchmarks and is flexible to be fine-tuned to different NLP tasks. To fine-tune the T5 model for Fox Sports, we concatenate the tabular features into a single sequence of text as our training input. Then we use the template-generated statements as labels. For example, the following table is translated into the text sequence Team Bobcats, prss, 4, score_differential_leading, 7.
Team name |
Metric |
Value |
Situation |
Rank |
Bobcats |
prss |
4 |
score_differential_leading |
7 |
The corresponding template statement – The Bobcats’ 4 total times of pressuring the quarterback when leading is the 7th highest in the NFL this season” – is passed in as the target output. After fine-tuning the T5 model with thousands of such examples, the model is able to generate statements similar to the template. It even works for previously unseen input, making it extensible to fresh players and newly created metrics.
We use the BLEU (Bilingual Evaluation Understudy) performance metric to quantitatively measure model performance. BLEU measures the matching quality of a generated sentence to a ground truth sentence by assigning a score from 0–100, with 100 being a perfect match to the ground truth. After fine-tuning on a few thousand sentences, the T5 model is able to achieve a BLEU score of above 99 on the test set, an indication that most of the generated sentences are identical to template-generated sentences. It also echoes the usefulness of using pretrained models on abundantly available unlabeled text for different downstream tasks.
Improving comprehensibility
The template-generated narratives capture core details, but are repetitive and sometimes difficult to read because they follow the same predefined sentence structure. This leads to confusion for the broadcasters and fans. To address this drawback, we include a second phase of modeling, which employs language models to enhance the readability and comprehensibility of the fine-tuned T5 model’s generated narratives. This step’s objective is to make the narratives sound more natural, allowing commentators to easily communicate the information during live broadcasting.
Language processing methods
One way to replace unnatural words in sentences is through back translation. Back translation is a two-step translation method. It first translates a sentence into another language, and then translates the sentence back to its original language. It’s a technique used mostly for text data augmentation, namely, increasing the variety of original text. For this use case, we find that translation models trained on a large text corpus can help fix mistakes in the original sentence. During back translation, a singular noun may be corrected to a plural. The model may also choose more natural-sounding language. This approach gives us an automatic way to improve readability for our generated sentences.
An alternative natural language processing (NLP) approach to back translation is called paraphrasing—a technique that aims to express semantically similar narratives in different forms. We employ a pretrained T5 model, which is fine-tuned for paraphrasing purposes using the open-sourced paraphraser dataset PAWS. Our paraphrasing model generates several candidates for a given narrative with slightly different content. One major advantage of using this technique is that it offers several narratives per input. This gives us several candidate sentences, from which we can choose the version that best fits Fox Sports’s business needs. An example of the paraphrasing output against a sample sentence is shown in the following table.
Type |
Sentence |
Original |
The Bobcats’ 4 total times of pressuring the quarterback when leading is the 7th highest out of 32 in the NFL this season. |
Paraphrased 1 |
The Bobcats pressing the quarterback 4 times when leading this season is the 7th best out of 32 in the NFL. |
Paraphrased 2 |
The Bobcats’ 4 total times of pressuring quarterback in leading is the 7th highest out of 32 in the NFL this season. |
Paraphrased 3 |
The Bobcats have pressured the quarterback 4 times total when leading—the 7th highest out of 32 in the NFL this season. |
Model evaluation
Quantitatively evaluating how natural a sentence sounds is an ongoing challenge in the NLP community. For this project, we use an existing metric called perplexity. Perplexity is a proxy measure of how “surprised” a language model is at sentences. In other words, it measures how common an evaluation sentence is among text corpus used to train a language model, which can be used to compare the quality of different sentences. For language models such as GPT2, it typically assigns a low perplexity score to real and syntactically correct sentences and high score to fake, incorrect, or highly infrequent sentences. For example, GPT2 assigns a lower score to sentences like “Can you do it?” and a higher score to sentences like “Can you does it?” With this, we can compare the quality of generated sentences sharing similar semantic meanings and output the one with the lowest perplexity score.
Architecture
Our final product is an end-to-end ML workflow using SageMaker. To meet Fox Sports’ needs, the workflow ensures that the following two criteria are satisfied:
- The end-to-end results must include all the required features defined by a user
- The final narrative output of the models shouldn’t be harder to read than the original rule-based template narrative
Our solution consists of two major components:
- Replace the current ruled-based approach with the fine-tuned T5 model
- Enhance the generated narratives through a multi-step ML-based approach
As illustrated in the following figure, the fine-tuned T5 ML model generates the narratives (green blocks). Next, the narratives are passed through the back translation model as an attempt to produce enhanced narratives. If the back translated results include the necessary keywords and their perplexity scores are lower compared to the T5 model outputs, they’re used as the final outputs. Otherwise, we pass the T5 model outputs through the paraphrasing model and apply the same condition check. If none of our enhancement models reduce the perplexity score, we simply output the T5 model outputs. Through this workflow, we ensure all the required features are captured and improve the readability of the sentence when appropriate, maximizing the benefit ML can bring to the existing solution.
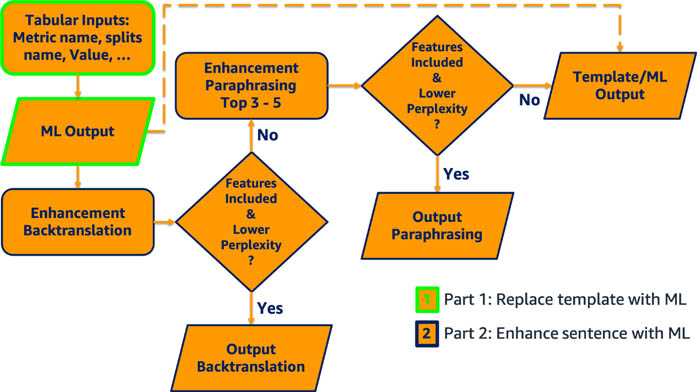
Results
With models combined to form the preceding architecture, the output narrative has on average 13% lower perplexity compared to original rule-based, template-generated narratives, and all the information is maintained. Fox Sports can display the narratives to broadcasters and sports fans for more exciting viewing experiences!
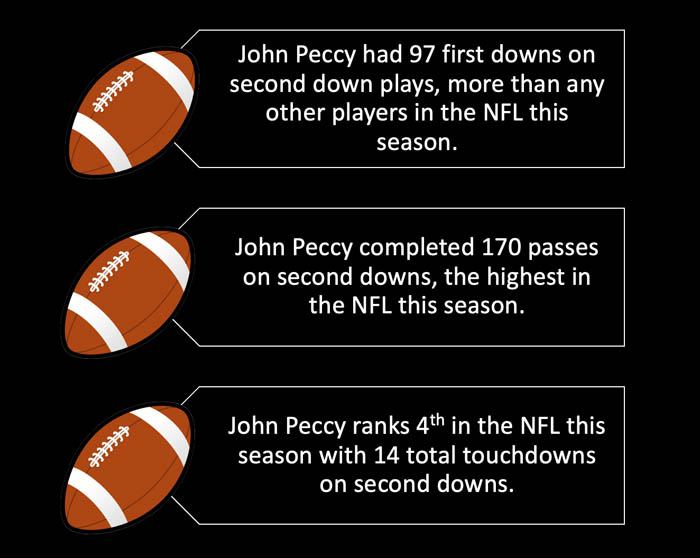
Conclusion
The ML Solutions Lab and Fox Sports ML team worked closely to build an end-to-end ML solution that converts in-game tabular stats into natural-sounding narratives. Because the solution is built on top of language models pretrained on a huge text corpus, additional metrics and game situations can be passed in directly to generate the desired outputs. The extensibility also enables the solution to be transferred to other sports by simply fine-tuning the model with sample narratives. These capabilities allow the model to scale and adapt to future business needs.
Around the world, many sports leagues and sports networks like Fox Sports are transforming the fan experience with AWS technology. AWS is helping bring fans closer to the game through partnering with Bundesliga, F1, NFL, NHL, NASCAR, and many others. Visit AWS Sports for more details.
If you’d like help accelerating your use of ML in your products and processes, please contact the ML Solutions Lab program.
About the Authors
Henry Wang is a Data Scientist at Amazon Machine Learning Solutions Lab. Prior to joining AWS, he was a graduate student at Harvard in Computational Science and Engineering, where he worked on healthcare research with reinforcement learning. In his spare time, he enjoys playing tennis and golf, reading, and watching StarCraft II tournaments.
Saman Sarraf is a Data Scientist at the Amazon ML Solutions Lab. His background is in applied machine learning including deep learning, computer vision, and time series data prediction.
Arbi Tamrazian is the Director of Data Science and Machine Learning at FOX where he focuses on building scalable machine learning solutions that can be applied to real-time data feeds and media assets. His main areas of interest are Deep Learning, Computer Vision and Reinforcement Learning.
Read More