The post Risk-driven backbone management during COVID-19 and beyond appeared first on Facebook Research.
Three’s Company: NVIDIA Studio 3D Showcase at SIGGRAPH Spotlights NVIDIA Omniverse Update, New NVIDIA RTX A2000 Desktop GPU, August Studio Driver
The future of 3D graphics is on display at the SIGGRAPH 2021 virtual conference, where NVIDIA Studio is leading the way, showcasing exclusive benefits that NVIDIA RTX technologies bring to creators working with 3D workflows.
It starts with NVIDIA Omniverse, an immersive and connected shared virtual world where artists create one-of-a-kind digital scenes, perfect 3D models, design beautiful buildings and more with endless creative possibilities. The Omniverse platform continues to expand, gaining Blender USD support, a new Adobe Substance 3D plugin, and a new extension, GANverse3D — designed to make 3D modeling easier with AI.
Omniverse is currently in open beta and free for NVIDIA RTX and GeForce RTX GPU users. With today’s launch of the NVIDIA RTX A2000 GPU, millions more 3D artists and content creators will have the opportunity to explore the platform’s capabilities.
The latest creative app updates, along with Omniverse and RTX A2000 GPUs, gain improved levels of support in the August NVIDIA Studio Driver, available for download today.
Omniverse Expands the 3D Metaverse at SIGGRAPH
NVIDIA announced that Blender, the world’s leading open-source 3D animation application, will include support for Pixar’s Universal Scene Description (USD) in the Blender 3.0 release, enabling artists to use the application with Omniverse production pipelines.
The open-source 3D file framework gives software partners and artists multiple ways to extend and connect to Omniverse through USD adoption, building a plugin, or an Omniverse Connector, extension or app.
NVIDIA also unveiled an experimental Blender alpha 3.0 USD branch that includes more advanced USD and material support, which will be available soon for Blender users everywhere.
In addition, NVIDIA and Adobe are collaborating on a new Substance 3D plugin that will enable Substance Material support in Omniverse.
With the plugin, materials created in Adobe Substance 3D or imported from the Substance 3D Asset Library can be adjusted directly in Omniverse. 3D artists will save valuable time when making changes as they don’t need to export and reupload assets from Substance 3D Designer and Substance 3D Sampler.
We’re also releasing a new Omniverse extension, GANverse3D – Image2Car, which makes 3D modeling easier with AI. It’s the first of a collection of extensions that will comprise the Omniverse AI Toy Box.
GANverse3D was built on a generative adversarial network trained on 2D photos, synthesizing multiple views of thousands of objects to predict 3D geometry, texture and part segmentation labels. This process could turn a single photo of a car into a 3D model that can drive around a virtual scene, complete with realistic headlights, blinkers and wheels.
The AI Toy Box extension allows inexperienced 3D artists to easily create scenes, and experienced artists to bring new enhancements to their multi-app workflows.
Here’s GANverse3D in action with an Omniverse-connected workflow featuring Omniverse Create, Reallusion Character Creator 3 and Adobe Photoshop.
For a further dive into the latest innovations in 3D, including Omniverse, watch the NVIDIA special address at SIGGRAPH on demand.
Omniverse plays a critical role in many creative projects, like the GTC keynote with NVIDIA CEO Jensen Huang.
Get a sneak peek of how a small team of artists was able to blur the line between real and rendered.
The full documentary releases alongside the NVIDIA SIGGRAPH panel on Wednesday, August 11, at 11 a.m. PT.
The world’s leading artists use NVIDIA RTX and Omniverse to create beautiful work and stunning worlds. Hear from them directly in the second edition of NVIDIA’s RTX All Stars, a free e-book that spotlights creative professionals.
More RTX, More 3D Creative Freedom
NVIDIA RTX A2000 joins the RTX lineup as the most powerful, low-profile, dual-slot GPU for 3D creators. The new desktop GPU encompasses the latest RTX technologies in the NVIDIA Ampere architecture, including:
- 2nd-gen RT Cores for real-time ray tracing with up to 5x performance from last gen with RTX ON.
- 3rd-gen Tensor Cores to power and accelerate creative AI features.
- 2x PCIe Gen 4 accelerating data paths in and out of the GPU and up to 6GB of GPU ECC memory for rendering and exporting large files.
RTX A2000-based systems will be available starting in October.
For on-the-go creators, the NVIDIA RTX A2000 laptop GPU — available in Studio laptops shipping today like the Lenovo ThinkPad P17 Gen 2 — is the most power-efficient, professional RTX laptop GPU bringing ray tracing and AI capabilities to thin and light mobile workstations.
The NVIDIA RTX A2000 GPUs support a wealth of creative workflows, including 3D modeling and Omniverse, whether behind a desktop or anywhere a laptop may travel.
August Brings Creative App Updates and Latest Studio Driver
Several exciting updates to cutting-edge creative apps shipped recently. The August Studio Driver, available today, sharpens support for all of them.
Topaz Sharpen AI v3.2 offers refinements to AI models accelerated by RTX GPUs and Tensor Cores, adding 1.5x motion blur and Too Soft/Very Blurry features further reducing artifacts.
In-app masking has also been improved with real-time processing of mask strokes and customization controls for the overlay display.
Reallusion Character Creator v3.43, the first third-party app with Audio2Face integration, now allows artists to export characters from Character Creator to Omniverse as USD files with Audio2Face-compliant meshes. This allows facial and lip animations to be completely AI-driven solely from voice input, regardless of language, simplifying the process of animating a 3D character.
Capture One 21 v14.3.0 adds a new Magic Brush tool to create complex masks for layer editing based on image content in a split second, working on an underlying processed image from the raw file. This process is hardware accelerated and is up to 3x faster when using the GPU compared to the CPU.
Support for these app updates, plus the new features in Omniverse, are only a click away. Download the August Studio Driver.
Best Studio Laptops for 3D Workflows
3D workflows range from modeling scenes in real time with complex lights and shadows, to visualizing architectural marvels, in or out of Omniverse, with massive exports. These necessitate major computational power, requiring advanced NVIDIA RTX and GeForce RTX GPUs to get jobs done quickly.
These Studio laptops are built to handle demanding 3D creative workflows:
- Lenovo P1 Gen 4 is stylish and lightweight, at less than 4 pounds. It comes in a ton of configurations, including GeForce RTX 3070 and 3080, plus RTX A4000 and A5000 laptop GPUs.
- Dell Precision 7760 is their thinnest, smallest and lightest 17-inch mobile workstation. With up to an RTX A5000 and 16GB of video memory, it’s great for working with massive 3D models or in multi-app workflows.
- Acer ConceptD 7 Ezel features their patented Ezel Hinge with a 15.6-inch, 4K PANTONE-validated touchscreen display. Available later this month, it also comes with up to a GeForce RTX 3080 laptop GPU and 16GB of video memory.
Set to make a splash later this year is the HP Zbook Studio G8. Engineered for heavy 3D work, it comes well-equipped with up to an RTX A5000 or GeForce RTX 3080 laptop GPU, perfect for on-the-go creativity.
Browse the NVIDIA Studio Shop for more great options.
Stay up to date on all things Studio by subscribing to the NVIDIA Studio newsletter and following us on Facebook, Twitter and Instagram.
The post Three’s Company: NVIDIA Studio 3D Showcase at SIGGRAPH Spotlights NVIDIA Omniverse Update, New NVIDIA RTX A2000 Desktop GPU, August Studio Driver appeared first on The Official NVIDIA Blog.
What Is the Metaverse?
What is the metaverse? The metaverse is a shared virtual 3D world, or worlds, that are interactive, immersive, and collaborative.
Just as the physical universe is a collection of worlds that are connected in space, the metaverse can be thought of as a bunch of worlds, too.
Massive online social games, like battle royale juggernaut Fortnite and user-created virtual worlds like Minecraft and Roblox, reflect some elements of the idea.
Video-conferencing tools, which link far-flung colleagues together amidst the global COVID pandemic, are another hint at what’s to come.
But the vision laid out by Neal Stephenson’s 1992 classic novel “Snow Crash” goes well beyond any single game or video-conferencing app.
The metaverse will become a platform that’s not tied to any one app or any single place — digital or real, explains Rev Lebaredian, vice president of simulation technology at NVIDIA.
And just as virtual places will be persistent, so will the objects and identities of those moving through them, allowing digital goods and identities to move from one virtual world to another, and even into our world, with augmented reality.
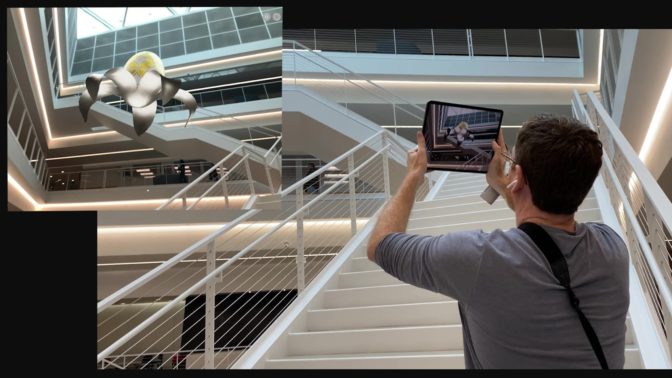
“Ultimately we’re talking about creating another reality, another world, that’s as rich as the real world,” Lebaredian says.
Those ideas are already being put to work with NVIDIA Omniverse, which, simply put, is a platform for connecting 3D worlds into a shared virtual universe.
Omniverse is in use across a growing number of industries for projects such as design collaboration and creating “digital twins,” simulations of real-world buildings and factories.
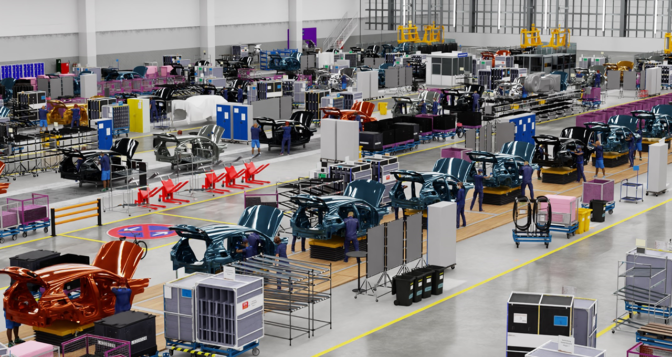
How NVIDIA Omniverse Creates, Connects Worlds Within the Metaverse
So how does Omniverse work? We can break it down into three big parts.
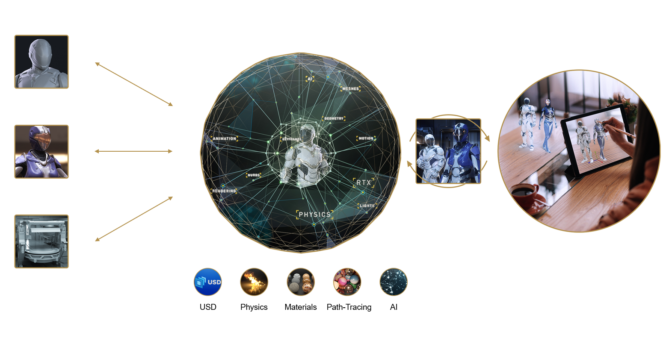
The first is Omniverse Nucleus. It’s a database engine that connects users and enables the interchange of 3D assets and scene descriptions.
Once connected, designers doing modeling, layout, shading, animation, lighting, special effects or rendering can collaborate to create a scene.
Omniverse Nucleus relies on USD, or Universal Scene Description, an interchange framework invented by Pixar in 2012.
Released as open-source software in 2016, USD provides a rich, common language for defining, packaging, assembling and editing 3D data for a growing array of industries and applications.
Lebardian and others say USD is to the emerging metaverse what hyper-text markup language, or HTML, was to the web — a common language that can be used, and advanced, to support the metaverse.
Multiple users can connect to Nucleus, transmitting and receiving changes to their world as USD snippets.
The second part of Omniverse is the composition, rendering and animation engine — the simulation of the virtual world.
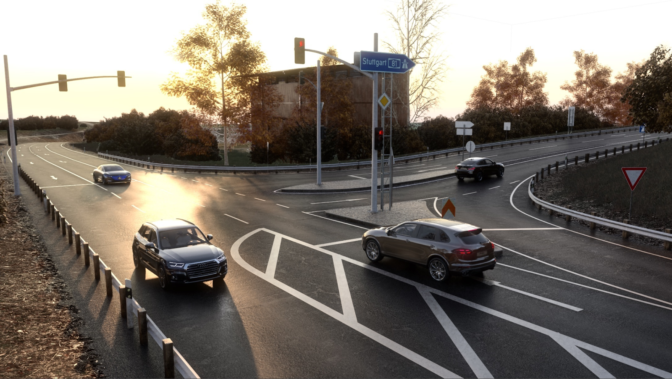
Omniverse is a platform built from the ground up to be physically based. Thanks to NVIDIA RTX graphics technologies, it is fully path traced, simulating how each ray of light bounces around a virtual world in real-time.
Omniverse simulates physics with NVIDIA PhysX. It simulates materials with NVIDIA MDL, or material definition language.
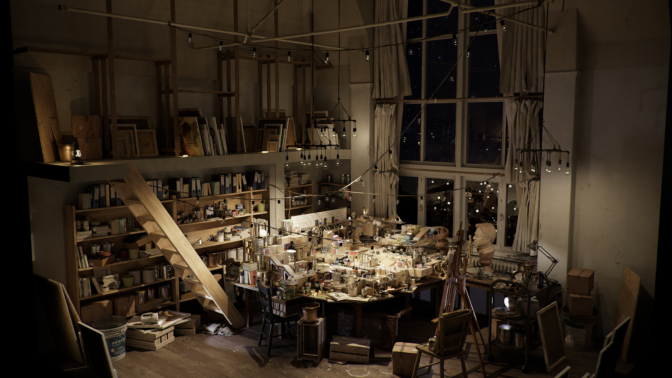
And Omniverse is fully integrated with NVIDIA AI (which is key to advancing robotics, more on that later).
Omniverse is cloud-native, scales across multiple GPUs, runs on any RTX platform and streams remotely to any device.
The third part is NVIDIA CloudXR, which includes client and server software for streaming extended reality content from OpenVR applications to Android and Windows devices, allowing users to portal into and out of Omniverse.
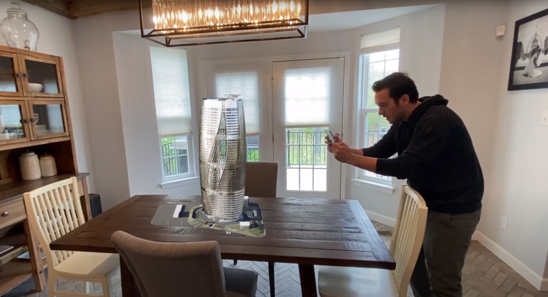
You can teleport into Omniverse with virtual reality, and AIs can teleport out of Omniverse with augmented reality.
Metaverses Made Real
NVIDIA released Omniverse to open beta in December, and NVIDIA Omniverse Enterprise in April. Professionals in a wide variety of industries quickly put it to work.
At Foster + Partners, the legendary design and architecture firm that designed Apple’s headquarters and London’s famed 30 St Mary Axe office tower — better known as “the Gherkin” — designers in 14 countries worldwide create buildings together in their Omniverse shared virtual space.
Visual effects pioneer Industrial Light & Magic is testing Omniverse to bring together internal and external tool pipelines from multiple studios. Omniverse lets them collaborate, render final shots in real-time and create massive virtual sets like holodecks.
Multinational networking and telecommunications company Ericsson uses Omniverse to simulate 5G wave propagation in real-time, minimizing multi-path interference in dense city environments.
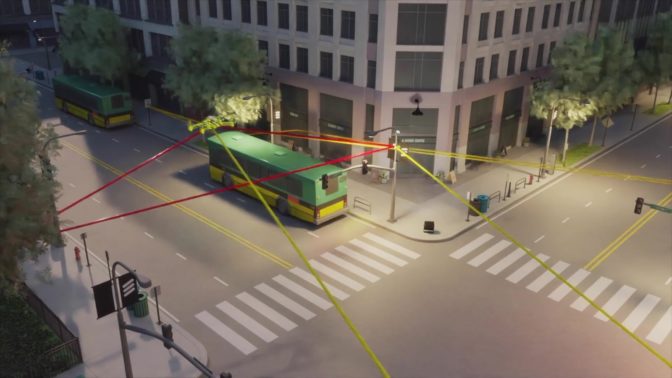
Infrastructure engineering software company Bentley Systems is using Omniverse to create a suite of applications on the platform. Bentley’s iTwin platform creates a 4D infrastructure digital twin to simulate an infrastructure asset’s construction, then monitor and optimize its performance throughout its lifecycle.
The Metaverse Can Help Humans and Robots Collaborate
These virtual worlds are ideal for training robots.
One of the essential features of NVIDIA Omniverse is that it obeys the laws of physics. Omniverse can simulate particles and fluids, materials and even machines, right down to their springs and cables.
Modeling the natural world in a virtual one is a fundamental capability for robotics.
It allows users to create a virtual world where robots — powered by AI brains that can learn from their real or digital environments — can train.
Once the minds of these robots are trained in the Omniverse, roboticists can load those brains onto a NVIDIA Jetson, and connect it to a real robot.
Those robots will come in all sizes and shapes — box movers, pick-and-place arms, forklifts, cars, trucks and even buildings.
In the future, a factory will be a robot, orchestrating many robots inside, building cars that are robots themselves.
How the Metaverse, and NVIDIA Omniverse, Enable Digital Twins
NVIDIA Omniverse provides a description for these shared worlds that people and robots can connect to — and collaborate in — to better work together.
It’s an idea that automaker BMW Group is already putting to work.
The automaker produces more than 2 million cars a year. In its most advanced factory, the company makes a car every minute. And each vehicle is customized differently.
BMW Group is using NVIDIA Omniverse to create a future factory, a perfect “digital twin.” It’s designed entirely in digital and simulated from beginning to end in Omniverse.
The Omniverse-enabled factory can connect to enterprise resource planning systems, simulating the factory’s throughput. It can simulate new plant layouts. It can even become the dashboard for factory employees, who can uplink into a robot to teleoperate it.
The AI and software that run the virtual factory are the same as what will run the physical one. In other words, the virtual and physical factories and their robots will operate in a loop. They’re twins.
No Longer Science Fiction
Omniverse is the “plumbing,” on which metaverses can be built.
It’s an open platform with USD universal 3D interchange, connecting them into a large network of users. NVIDIA has 12 Omniverse Connectors to major design tools already, with another 40 on the way. The Omniverse Connector SDK sample code, for developers to write their own Connectors, is available for download now.
The most important design tool platforms are signed up. NVIDIA has already enlisted partners from the world’s largest industries — media and entertainment; gaming; architecture, engineering and construction; manufacturing; telecommunications; infrastructure; and automotive.
And the hardware needed to run it is here now.
Computer makers worldwide are building NVIDIA-Certified workstations, notebooks and servers, which all have been validated for running GPU-accelerated workloads with optimum performance, reliability and scale. And starting later this year, Omniverse Enterprise will be available for enterprise license via subscription from the NVIDIA Partner Network.
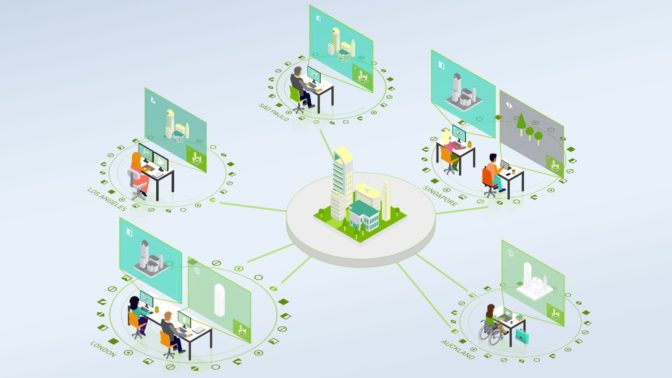
Thanks to NVIDIA Omniverse, the metaverse is no longer science fiction.
Back to the Future
So what’s next?
Humans have been exploiting how we perceive the world for thousands of years, NVIDIA’s Lebaredian points out. We’ve been hacking our senses to construct virtual realities through music, art and literature for millennia.
Next, add interactivity and the ability to collaborate, he says. Better screens, head-mounted displays like the Oculus Quest, and mixed-reality devices like Microsoft’s Hololens are all steps toward fuller immersion.
All these pieces will evolve. But the most important one is here already: a high-fidelity simulation of our virtual world to feed the display. That’s NVIDIA Omniverse.
To steal a line from science-fiction master William Gibson: the future is already here; it’s just not very evenly distributed.
The metaverse is the means through which we can distribute those experiences more evenly. Brought to life by NVIDIA Omniverse, the metaverse promises to weave humans, AI and robots together in fantastic new worlds.
The post What Is the Metaverse? appeared first on The Official NVIDIA Blog.
NVIDIA Makes RTX Technology Accessible to More Professionals
With its powerful real-time ray tracing and AI acceleration capabilities, NVIDIA RTX technology has transformed design and visualization workflows for the most complex tasks, like designing airplanes and automobiles, visual effects in movies and large-scale architectural design.
The new NVIDIA RTX A2000 — our most compact, power-efficient GPU for a wide range of standard and small-form-factor workstations — makes it easier to access RTX from anywhere.
The RTX A2000 is designed for everyday workflows, so professionals can develop photorealistic renderings, build physically accurate simulations and use AI-accelerated tools. With it, artists can create beautiful 3D worlds, architects can design and virtually explore the next generation of smart buildings and homes, and engineers can create energy-efficient and autonomous vehicles that will drive us into the future.
The GPU has 6GB of memory capacity with error correction code (ECC) to maintain data integrity for uncompromised computing accuracy and reliability, which especially benefits the healthcare and financial services fields.
With remote work part of the new normal, simultaneous collaboration with colleagues on projects across the globe is critical. NVIDIA RTX technology powers Omniverse, our collaboration and simulation platform that enables teams to iterate together on a single 3D design in real time while working across different software applications. The A2000 will serve as a portal into this world for millions of designers.
Customer Adoption
Among the first to tap into the RTX A2000 are Avid, Cuhaci & Peterson and Gilbane Building Company.
“The A2000 from NVIDIA has made our modeling flow faster and more efficient. No longer are we sitting and wasting valuable time for graphics to render, and panning around complex geometry has become smoother,” said Connor Reddington, mechanical engineer and certified SOLIDWORKS professional at Avid Product Development, a Lubrizol Company.
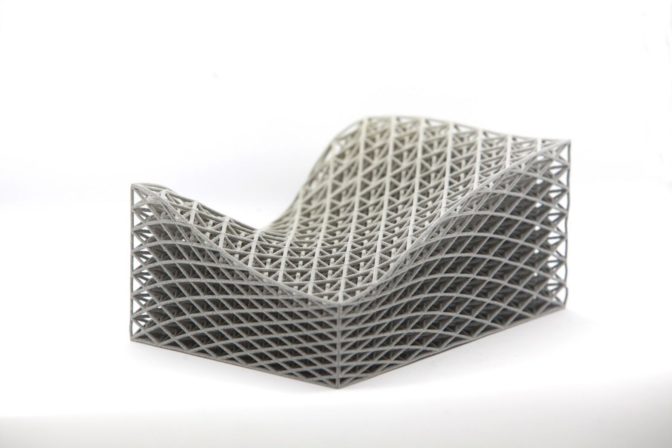
“Introducing RT Cores into the NVIDIA RTX A2000 has resulted in impressive rendering speedups for photorealistic visualization compared to the previous generation GPUs,” said Steven Blevins, director of Digital Practice at Cuhaci & Peterson.
“The small form factor and low power usage of the NVIDIA RTX A2000 is extraordinary and ensures fitment in just about any existing workstation chassis,” said Ken Grothman, virtual design and construction manager at Gilbane Building Company.
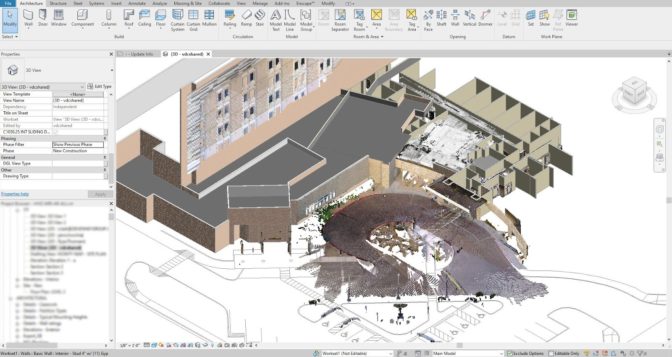
Next-Generation RTX Technology
The NVIDIA RTX A2000 is the most powerful low-profile, dual-slot GPU for professionals. It combines the latest-generation RT Cores, Tensor Cores and CUDA cores with 6GB of ECC graphics memory in a compact form factor to fit a wide range of systems.
The NVIDIA RTX A2000 features the latest technologies in the NVIDIA Ampere architecture:
- Second-Generation RT Cores: Real-time ray tracing for all professional workflows. Up to 5x the rendering performance from the previous generation with RTX on.
- Third-Generation Tensor Cores: Available in the GPU architecture to enable AI-augmented tools and applications.
- CUDA Cores: Up to 2x the FP32 throughput of the previous generation for significant increases in graphics and compute workloads.
- Up to 6GB of GPU Memory: Supports ECC memory, the first time that NVIDIA has enabled ECC memory in its 2000 series GPUs, for error-free computing.
- PCIe Gen 4: Double the throughput with more than 40 percent bandwidth improvement from the previous generation for accelerating data paths in and out of the GPU.
Availability
The NVIDIA RTX A2000 desktop GPU will be available in workstations from manufacturers including ASUS, BOXX Technologies, Dell Technologies, HP and Lenovo as well as NVIDIA’s global distribution partners starting in October.
Learn more about NVIDIA at SIGGRAPH.
The post NVIDIA Makes RTX Technology Accessible to More Professionals appeared first on The Official NVIDIA Blog.
A Code for the Code: Simulations Obey Laws of Physics with USD
Life in the metaverse is getting more real.
Starting today, developers can create and share realistic simulations in a standard way. Apple, NVIDIA and Pixar Animation Studios have defined a common approach for expressing physically accurate models in Universal Scene Description (USD), the common language of virtual 3D worlds.
Pixar released USD and described it in 2016 at SIGGRAPH. It was originally designed so artists could work together, creating virtual characters and environments in a movie with the tools of their choice.
Fast forward, and USD is now pervasive in animation and special effects. USD is spreading to other professions like architects who can benefit from their tools to design and test everything from skyscrapers to sports cars and smart cities.
Playing on the Big Screen
To serve the needs of this expanding community, USD needs to stretch in many directions. The good news is Pixar designed USD to be open and flexible.
So, it’s fitting the SIGGRAPH 2021 keynote provides a stage to describe USD’s latest extension. In technical terms, it’s a new schema for rigid-body physics, the math that describes how solids behave in the real world.
For example, when you’re simulating a game where marbles roll down ramps, you want them to respond just as you would expect when they hit each other. To do that, developers need physical details like the weight of the marbles and the smoothness of the ramp. That’s what this new extension supplies.
USD Keeps Getting Better
The initial HTML 1.0 standard, circa 1993, defined how web pages used text and graphics. Fifteen years later HTML5 extended the definition to include video so any user on any device could watch videos and movies.
Apple and NVIDIA were both independently working on ways to describe physics in simulations. As members of the SIGGRAPH community, we came together with Pixar to define a single approach as a new addition to USD.
In the spirit of flexibility, the extension lets developers choose whatever solvers they prefer as they can all be driven from the same set of USD-data. This presents a unified set of data suitable for off-line simulation for film, to games, to augmented reality.
That’s important because solvers for real-time uses like gaming prioritize speed over accuracy, while architects, for example, want solvers that put accuracy ahead of speed.
An Advance That Benefits All
Together the three companies wrote a white paper describing their combined proposal and shared it with the USD community. The reviews are in and it’s a hit. Now the extension is part of the standard USD distribution, freely available for all developers.
The list of companies that stand to benefit reads like credits for an epic movie. It includes architects, building managers, product designers and manufacturers of all sorts, companies that design games — even cellular providers optimizing layouts of next-generation networks. And, of course, all the vendors that provide the digital tools to do the work.
“USD is a major force in our industry because it allows for a powerful and consistent representation of complex, 3D scene data across workflows,” said Steve May, Chief Technology Officer at Pixar.
“Working with NVIDIA and Apple, we have developed a new physics extension that makes USD even more expressive and will have major implications for entertainment and other industries,” he added.
Making a Metaverse Together
It’s a big community we aim to serve with NVIDIA Omniverse, a collaboration environment that’s been described as an operating system for creatives or “like Google Docs for 3D graphics.”
We want to make it easy for any company to create lifelike simulations with the tools of their choice. It’s a goal shared by dozens of organizations now evaluating Omniverse Enterprise, and close to 400 companies and tens of thousands of individual creators who have downloaded Omniverse open beta since its release in December 2020.
We envision a world of interconnected virtual worlds — a metaverse — where someday anyone can share their life’s work.
Making that virtual universe real will take a lot of hard work. USD will need to be extended in many dimensions to accommodate the community’s diverse needs.
A Virtual Invitation
To get a taste of what’s possible, watch a panel discussion from GTC (free with registration), where 3D experts from nine companies including Pixar, BMW Group, Bentley Systems, Adobe and Foster + Partners talked about the opportunities and challenges ahead.
We’re happy we could collaborate with engineers and designers at Apple and Pixar on this latest USD extension. We’re already thinking about a sequel for soft-body physics and so much more.
Together we can build a metaverse where every tool is available for every job.
For more details, watch a talk on the USD physics extension from NVIDIA’s Adam Moravanszky and attend a USD birds-of-a-feather session hosted by Pixar.
The post A Code for the Code: Simulations Obey Laws of Physics with USD appeared first on The Official NVIDIA Blog.
Amazon Robotics names initial fellowship program recipients
The fellowships are aimed at helping students from underrepresented backgrounds establish careers in robotics, engineering, computer science, and related fields.Read More
Attendee matchmaking at virtual events with Amazon Personalize
Amazon Personalize enables developers to build applications with the same machine learning (ML) technology used by Amazon.com for real-time personalized recommendations—no ML expertise required. Amazon Personalize makes it easy for developers to build applications capable of delivering a wide array of personalization experiences, including specific product recommendations, personalized product re-ranking, and customized direct marketing. Besides applications in retail and ecommerce, other common use cases for Amazon Personalize include recommending videos, blog posts, or newsfeeds based on users’ activity history.
What if you wanted to recommend users of common interest to connect with each other? As the pandemic pushes many of our normal activities virtual, connecting with people is a greater challenge than ever before. This post discusses how 6Connex turned this challenge into an opportunity by harnessing Amazon Personalize to elevate their “user matchmaking” feature.
6Connex and event AI
6Connex is an enterprise virtual venue and hybrid events system. Their cloud-based product portfolio includes virtual environments, learning management, and webinars. Attendee experience is one of the most important metrics for their success.
Attendees have better experiences when they are engaged not only with the event’s content, organizers, and sponsors, but also when making connections with other attendees. Engagement metrics are measured and reported for each attendee activity on the platform, as well as feedback from post-event surveys. The goal is to make the events system more attendee-centric by not only providing personalized content and activity recommendations, but also making matchmaking suggestions for attendees based on similar interests and activity history. By adding event AI features to their platform, 6Connex fosters more meaningful connections between attendees, and keeps their attendees more engaged with a personalized event journey.
Implementation and solution architecture
6Connex built their matchmaking solution using the related items recipe (SIMS) of Amazon Personalize. The SIMS algorithm uses collaborative filtering to recommend items that are similar to a given item. The novelty of 6Connex’s approach lies in the reverse mapping of users and items. In this solution, event attendees are items in Amazon Personalize terms, and content, meeting rooms, and so on are users’ in Amazon Personalize terms.
When a platform user joins a meeting room or views a piece of content, an interaction is created. To increase the accuracy of interaction types, also known as event_type, you can add logic to only count as an interaction when a user stays in a meeting room for at least a certain amount of time. This eliminates accidental clicks and cases when users join but quickly leave a room due to lack of interest.
As many users interact with the platform during a live event, interactions are streamed in real time from the platform via Amazon Kinesis Data Streams. AWS Lambda functions are used for data transformation before streaming data directly to Amazon Personalize through an event tracker. This mechanism also enables Amazon Personalize to adjust to changing user interest over time, allowing recommendations to adapt in real time.
After a model is trained in Amazon Personalize, a fully managed inference endpoint (campaign) is created to serve real-time recommendations for 6Connex’s platform. To answer the question “for each attendee, who are similar attendees?”, 6Connex’s client-side application queries the GetRecommendations API with a current user (represented as an itemId
). The API response provides recommended connections because they have been identified as similar by the Amazon Personalize.
Due to its deep learning capabilities, Amazon Personalize requires at least 1,000 interaction data points before training the model. At the start of a live event, there aren’t enough interactions, therefore a rules engine is used at the beginning of an event to provide the initial recommendations prior to gathering 1000 data points. The following table shows the three main phases of an event where connection recommendations are generated.
Rule-based recommendations |
|
Amazon Personalize real-time recommendations during live sessions |
|
Amazon Personalize batch recommendations for on-demand users |
|
For a high-level example architecture, see the following diagram.
The following are the steps involved in the solution architecture:
- 6Connex web application calls the
GetRecommendations
API to retrieve recommended connections. - A matchmaking Lambda function retrieves recommendations.
- Until the training threshold of 1,000 interaction data points is reached, the matchmaking function uses a simple rules engine to provide recommendations.
- Recommendations are generated from Amazon Personalize and stored in Amazon ElastiCache. The reason for caching recommendations is to improve response performance while reducing the number of queries on the Amazon Personalize API. When new recommendations are requested, or when the cache expires (expiration is set to every 15 minutes), recommendations are pulled from Amazon Personalize.
- New user interactions are ingested in real time via Kinesis Data Streams.
- A Lambda function consumes data from the data stream, performs data transformation, persists the transformed data to Amazon Simple Storage Service (Amazon S3) and related metadata to Amazon DynamoDB, and sends the records to Amazon Personalize via the
PutEvents
API. - AWS Step Functions orchestrates the process for creating solutions, training, retraining, and several other workflows. More details on the Step Functions workflow are in the next section.
- Amazon EventBridge schedules regular retraining events during the virtual events. We also use EventBridge to trigger batch recommendations after the virtual events are over and when the contents are served to end users on demand.
- Recommendations are stored in DynamoDB for use during the on-demand period and also for future analysis.
Adoption of MLOps
It was crucial for 6Connex to quickly shift from a rules-based recommender engine to personalized recommendations using Amazon Personalize. To accelerate this shift and hydrate the interactions dataset, 6Connex infers interactions not only from content engagement, but also from other sources such as pre-events questionnaires. This is an important development that increased the speed to when users start receiving ML-based recommendations.
More importantly, the adoption of Amazon Personalize MLOps enabled 6Connex to automate and accelerate the transition from rule-based recommendations to personalized recommendations using Amazon Personalize. After the minimum threshold for data is met, Step Functions loads data into Amazon Personalize and manages the training process.
The following diagram shows the MLOps pipeline for the initial loading of data, training solutions, and deploying campaigns.
6Connex created their MLOps solution based on the Amazon Personalize MLOps reference solution to automate this process. There are several Step Functions workflows that offload long-running processes such loading batch recommendations in DynamoDB, retraining Amazon Personalize solutions, and cleaning up after an event is complete.
With Amazon Personalize and MLOps pipelines, 6Connex brought an AI solution to market in less than half the time it would have taken to develop and deploy their own ML infrastructure. Moreover, these solutions reduced the cost of acquiring data science and ML expertise. As a result, 6Connex realized a competitive advantage through AI-based personalized recommendations for each individual user.
Based on the success of this engagement, 6Connex plans to expand its usage of Amazon Personalize to provide content-based recommendations in the near future. 6Connex is looking forward to expanding the partnership not only in ML, but also in data analytics and business intelligence to serve the fast-growing hybrid event market.
Conclusion
With a well-designed MLOps pipeline and some creativity, 6Connex built a robust recommendation engine using Amazon Personalize in a short amount of time.
Do you have a use case for a recommendation engine but are short on time or ML expertise? You can get started with Amazon Personalize using the Developer Guide, as well as a myriad of hands-on resources such as the Amazon Personalize Samples GitHub repo.
If you have any questions on this matchmaking solution, please leave a comment!
About the Author
Shu Jackson is a Senior Solutions Architect with AWS. Shu works with startup customers helping them design and build solutions in the cloud, with a focus on AI/ML.
Luis Lopez Soria is a Sr AI/ML specialist solutions architect working with the Amazon Machine Learning team. He works with AWS customers to help them adopt machine learning on a large scale. He enjoys playing sports, traveling around the world, and exploring new foods and cultures.
Accurately predicting future sales at Clearly using Amazon Forecast
This post was cowritten by Ziv Pollak, Machine Learning Team Lead, and Alex Thoreux, Web Analyst at Clearly.
A pioneer in online shopping, Clearly launched their first site in 2000. Since then, they’ve grown to become one of the biggest online eyewear retailers in the world, providing customers across Canada, the US, Australia and New Zealand with glasses, sunglasses, contact lenses, and other eye health products. Through their Mission to eliminate poor vision, Clearly strives to make eyewear affordable and accessible for everyone. Creating an optimized platform is a key part of this wider vision.
Predicting future sales is one of the biggest challenges every retail organization has – but it’s also one of the most important pieces of insight. Having a clear and reliable picture of predicted sales for the next day or week allows your company to adjust its strategy and increase the chances of meeting its sales and revenue goals.
We’ll talk about how Clearly built an automated and orchestrated forecasting pipeline using AWS Step Functions, and used Amazon Forecast APIs to train a machine learning (ML) model and predict sales on a daily basis for the upcoming weeks and months.
With a solution that also collects metrics and logs, provides auditing, and is invoked automatically, Clearly was able to create a serverless, well-architected solution in just a few weeks.
The challenge: Detailed sales forecasting
With a reliable sales forecast, we can improve our marketing strategy, decision-making process, and spend, to ensure successful operations of the business.
In addition, when a diversion between the predicted sales numbers and the actual sales number occurs, it’s a clear indicator that something is wrong, such as an issue with the website or promotions that may not be working properly. From there, we can problem solve the issues and address them in a timely manner.
For forecasting sales, our existing solution was based on senior members of the marketing team building manual predictions. Historical data was loaded into an Excel sheet and predictions were made using basic forecasting functionality and macros. These manual predictions were nearly 90% accurate and took 4–8 hours to complete, which was a good starting point, but still not accurate enough to confidently guide the marketing team’s next steps.
In addition, testing “what-if” future scenarios was difficult to implement because we could only perform the predictions for the following months, without further granularity such as weeks and days.
Having a detailed sales forecast allows us to identify situations where money is being lost due to outages or other technical or business issues. With a reliable and accurate forecast, when we see that actual sales aren’t meeting expected sales, we know there is an issue.
Another major challenge we faced was the lack of a tenured ML team – all members had been with the company less than a year when the project kicked off.
Overview of solution: Forecast
Amazon Forecast is a fully managed service that uses ML to deliver highly accurate forecasts. After we provided the data, Forecast automatically examined it, identified what was meaningful, and produced a forecasting model capable of making predictions on our different lines of products and geographical locations to deliver the most accurate daily forecasts. The following diagram illustrates our forecasting pipeline.
To operationalize the flow, we applied the following workflow:
- Amazon EventBridge calls the orchestration pipeline daily to retrieve the predictions.
- Step Functions help manage the orchestration pipeline.
- An AWS Lambda function calls Amazon Athena APIs to retrieve and prepare the training data, stored on Amazon Simple Storage Service (Amazon S3).
- An orchestrated pipeline of Lambda functions uses Forecast to create the datasets, train the predictors, and generate the forecasted revenue. The forecasted data is saved in an S3 bucket.
- Amazon Simple Notification Service (Amazon SNS) notifies users when a problem occurs during the forecasting process or when the process completes successfully.
- Business analysts build dashboards on Amazon QuickSight, which queries the forecast data from Amazon S3 using Athena.
We chose to work with Forecast for a few reasons:
- Forecast is based on the same technology used at Amazon.com, so we have a lot of confidence in the tool’s capabilities.
- The ease of use and implementation allowed us to quickly confirm we have the needed dataset to produce accurate results.
- Because the Clearly ML team was less than 1 year old, a fully managed service allowed us to deliver this project without needing deep technical ML skills and knowledge.
Data sources
Finding the data to use for this forecast, while making sure it was clear and reliable, was the most important element in our ability to generate accurate predictions. We ended up using the following datasets, training the model on 3 years of daily data:
- Web traffic.
- Number of orders.
- Average order value.
- Conversion rate.
- New customer revenue.
- Marketing spend.
- Marketing return on advertisement spend.
- Promotions.
To create the dataset, we went through many iterations, changing the number of data sources until the predictions reach our benchmark of at least 95% accuracy.
Dashboard and results
Writing the prediction results into our existing data lake allows us to use QuickSight to build metrics and dashboards for the senior-level managers. This enables them to understand and use these results when making decisions on the next steps needed to meet our monthly marketing targets.
We were able to present the forecast results on two levels, starting with overall business performance and then going deeper into performance per each line of business (contacts and glasses). For those three cases (overall, contacts, glasses) we presented the following information:
- Predicted revenue vs. target – This allows the marketing team to understand how we’re expected to perform this month, compared to our target, if they take no additional actions. For example, if we see that the projected sales don’t meet our marketing goals, we need to launch a new marketing campaign. The following screenshot shows an example analysis with a value of -17.47%, representing the expected total monthly revenue vs. the target.
- Revenue performance compared to predictions over the last month – This graph shows that the predicted revenue is within the forecasted range, which means that the predictions are accurate. The following example graph shows high bound, revenue, and low bound values.
- Month to date revenue compared to weekly and monthly forecasts – The following example screenshot shows text automatically generated by QuickSight that indicates revenue-related KPIs.
Thanks to Forecast, Clearly now has an automated pipeline that generates forecasts for daily and weekly scenarios, reaching or surpassing our benchmarks of 97%, which is an increase of 7.78% from a process that was done manually and was limited to longer periods.
Now, daily forecasts for weekly and monthly revenue take only 15 minutes in data gathering and preparation, with the forecasting process taking close to 15 minutes to complete on a daily basis. This is a huge improvement from 4-8 hours with the manual process, which could only perform predictions for the whole month.
With more granularity and better accuracy, our marketing team has better tools to act faster on discrepancies and create prediction scenarios on campaigns could achieve better revenue results.
Conclusion
Effective and accurate prediction of customer future behavior is one of the biggest challenges in ML in retail today, and having a good understanding of our customers and their behavior is vital for business success. Forecast provided a fully managed ML solution to easily create an accurate and reliable prediction with minimal overhead. The biggest benefit we get with these predictions is that we have accurate visibility of what the future will look like and can change it if it doesn’t meet our targets.
In addition, Forecast allows us to predict what-if scenarios and their impact on revenue. For example, we can project the overall revenue until the end of the month, and with some data manipulation we can also predict what will happen if we launch a BOGO (buy one, get one free) campaign next Tuesday.
“With leading ecommerce tools like Virtual Try On, combined with our unparalleled customer service, we strive to help everyone see clearly in an affordable and effortless manner—which means constantly looking for ways to innovate, improve, and streamline processes. Effective and accurate prediction of customer future behavior is one of the biggest challenges in machine learning in retail today. In just a few weeks, Amazon Forecast helped us accurately and reliably forecast sales for the upcoming week with over 97% accuracy, and with over 90% accuracy when predicting sales for the following month.”
– Dr. Ziv Pollak, Machine Learning Team Leader.
For more information about how to get started building your own MLOps pipelines with Forecast, see Building AI-powered forecasting automation with Amazon Forecast by applying MLOps, and for other use cases, visit the AWS Machine Leaning Blog.
The content and opinions in this post are those of the third-party author and AWS is not responsible for the content or accuracy of this post.
About the Author
Dr Ziv Pollak is an experienced technical leader who transforms the way organizations use machine learning to increase revenue, reduce costs, improve customer service, and ensure business success. He is currently leading the Machine Learning team at Clearly.
Alex Thoreux is a Jr Web Analyst at Clearly who built the forecasting pipeline, as well as other ML applications for Clearly.
Fernando Rocha is a Specialist SA. As Clearly’s Solutions Architect, he helps them build analytics and machine learning solutions on AWS.
Announcing model improvements and lower annotation limits for Amazon Comprehend custom entity recognition
Amazon Comprehend is a natural language processing (NLP) service that provides APIs to extract key phrases, contextual entities, events, sentiment from unstructured text, and more. Entities refer to things in your document such as people, places, organizations, credit card numbers, and so on. But what if you want to add entity types unique to your business, like proprietary part codes or industry-specific terms? Custom entity recognition (CER) in Amazon Comprehend enables you to train models with entities that are unique to your business in just a few easy steps. You can identify almost any kind of entity, simply by providing a sufficient number of details to train your model effectively.
Training an entity recognizer from the ground up requires extensive knowledge of machine learning (ML) and a complex process for model optimization. Amazon Comprehend makes this easy for you using a technique called transfer learning to help build your custom model. Internally, Amazon Comprehend uses base models that have been trained on data collected by Amazon Comprehend and optimized for the purposes of entity recognition. With this in place, all you need to supply is the data. ML model accuracy is typically dependent on both the volume and quality of data. Getting good quality annotation data is a laborious process.
Until today, you could train an Amazon Comprehend custom entity recognizer with only 1,000 documents and 200 annotations per entity. Today, we’re announcing that we have improved underlying models for the Amazon Comprehend custom entity API by reducing the minimum requirements to train the model. Now, with as few as 250 documents and 100 annotations per entity (also referred to as shots), you can train Amazon Comprehend CER models to predict entities with greater accuracy. To take advantage of the updated performance offered by the new CER model framework, you can simply retrain and deploy improved models.
To illustrate the model improvements, we compare the result of previous models with that of the new release. We selected a diverse set of entity recognition datasets across different domains and languages from the open-source domain to showcase the model improvements. In this post, we walk you through the results from our training and inference process between the previous CER model version and the new CER model.
Datasets
When you train an Amazon Comprehend CER model, you provide the entities that you want the custom model to recognize, and the documents with text containing these entities. You can train Amazon Comprehend CER models using entity lists or annotations. Entity lists are CSV files that contain the text (a word or words) of an entity example from the training document along with a label, which is the entity type that the text is categorized as. With annotations, you can provide the positional offset of entities in a sentence along with the entity type being represented. When you use the entire sentence, you’re providing the contextual reference for the entities, which increases the accuracy of the model you’re training.
We selected the annotations option for labeling our entities because the datasets we selected already contained the annotations for each of the entity types represented. In this section, we discuss the datasets we selected and what they describe.
CoNLL
The Conference on Computational Natural Language Learning (CoNLL) provides datasets for language-independent (doesn’t use language-specific resources for performing the task) named entity recognition with entities provided in English, Spanish, and German. Four types of named entities are provided in the dataset: persons, locations, organizations, and names of miscellaneous entities that don’t belong to the previous three types.
We used the CoNLL-2003 dataset for English, and the CoNLL-2002 dataset for Spanish languages for our entity recognition training. We ran some basic transformations to convert the annotations data to a format that is required by Amazon Comprehend CER. We converted the entity types from their semantic notation to actual words they represent, such as person
, organization
, location
, and miscellaneous
.
SNIPS
The SNIPS dataset was created in 2017 as part of benchmarking tests for natural language understanding (NLU) by Snips. The results from these tests are available in the 2018 paper “Snips Voice Platform: an embedded Spoken Language Understanding system for private-by-design voice interfaces” by Coucke, et al. We used the GetWeather
and the AddToPlaylist
datasets for our experiments. The entities for the GetWeather
dataset we considered are timerange
, city
, state
, condition_description
, country
, and condition_temperature
. For AddToPlaylist
, we considered the entities artist
, playlist_owner
, playlist
, music_item
, and entity_name
.
Sampling configuration
The following table represents the dataset configuration for our tests. Each row represents an Amazon Comprehend CER model that was trained, deployed, and used for entity prediction with our test dataset.
Dataset | Published year | Language | Number of documents sampled for training | Number of entities sampled | Number of annotations per entity (shots) | Number of documents sampled for blind test inference (never seen during training) |
SNIPS-AddToPlaylist | 2017 | English | 254 | 5 | artist – 101 playlist_owner – 148 playlist – 254 music_item – 100 entity_name – 100 |
100 |
SNIPS-GetWeather | 2017 | English | 600 | 6 | timeRange – 281 city – 211 state – 111 condition_description – 121 country – 117 condition_temperature – 115 |
200 |
SNIPS-GetWeather | 2017 | English | 1000 | 6 | timeRange -544 city – 428 state -248 condition_description -241 country -230 condition_temperature – 228 |
200 |
SNIPS-GetWeather | 2017 | English | 2000 | 6 | timeRange -939 city -770 state – 436 condition_description – 401 country – 451 condition_temperature – 431 |
200 |
CoNLL | 2003 | English | 350 | 3 | Location – 183 Organization – 111 Person – 229 |
200 |
CoNLL | 2003 | English | 600 | 3 | Location – 384 Organization – 210 Person – 422 |
200 |
CoNLL | 2003 | English | 1000 | 4 | Location – 581 Miscellaneous – 185 Organization – 375 Person – 658 |
200 |
CoNLL | 2003 | English | 2000 | 4 | Location – 1133 Miscellaneous – 499 Organization – 696 Person – 1131 |
200 |
CoNLL | 2002 | Spanish | 380 | 4 | Location – 208 Miscellaneous – 103 Organization – 404 Person – 207 |
200 |
CoNLL | 2002 | Spanish | 600 | 4 | Location – 433 Miscellaneous – 220 Organization – 746 Person – 436 |
200 |
CoNLL | 2002 | Spanish | 1000 | 4 | Location – 578 Miscellaneous – 266 Organization – 929 Person – 538 |
200 |
CoNLL | 2002 | Spanish | 2000 | 4 | Location – 1184 Miscellaneous – 490 Organization – 1726 Person – 945 |
200 |
For more details on how to format data to create annotations and entity lists for Amazon Comprehend CER, see Training Custom Entity Recognizers. We created a benchmarking approach based on the sampling configuration for our tests, and we discuss the results in the following sections.
Benchmarking process
As shown in the sampling configuration in the preceding section, we trained a total of 12 models, with four models each for CoNLL English and Spanish datasets with varying document and annotation configurations, three models for the SNIPS-GetWeather
dataset, again with varying document and annotation configurations, and one model with the SNIPS-AddToPlaylist
dataset, primarily to test the new minimums of 250 documents and 100 annotations per entity.
Two inputs are required to train an Amazon CER model: entity representations and the documents containing these entities. For an example of how to train your own CER model, refer to Setting up human review of your NLP-based entity recognition models with Amazon SageMaker Ground Truth, Amazon Comprehend, and Amazon A2I. We measure the accuracy of our models using metrics such as F1 score, precision, and recall for the test set at training and the blind test set at inference. We run subsequent inference on these models using a blind test dataset of documents that we set aside from our original datasets.
Precision indicates how many times the model makes a correct entity identification compared to the number of attempted identifications. Recall indicates how many times the model makes a correct entity identification compared to the number of instances of that the entity is actually present, as defined by the total number of correct identifications (true positives) and missed identifications (false negatives). F1 score indicates a combination of the precision and recall metrics, which measures the overall accuracy of the model for custom entity recognition. To learn more about these metrics, refer to Custom Entity Recognizer Metrics.
Amazon Comprehend CER provides support for both real-time endpoints and batch inference requirements. We used the asynchronous batch inference API for our experiments. Finally, we calculated the F1 score, precision, and recall for the inference by comparing what the model predicted with what was originally annotated for the test documents. The metrics are calculated by doing a strict match for the span offsets, and a partial match isn’t considered nor given partial credit.
Results
The following tables document the results from our experiments we ran using the sampling configuration and the benchmarking process we explained previously.
Previous limits vs. new limits
The limits have reduced from 1,000 documents and 200 annotations per entity for CER training in the previous model to 250 documents and 100 annotations per entity in the improved model.
The following table shows the absolute improvement in F1 scores measured at training, between the old and new models. The new model improves the accuracy of your entity recognition models even when you have a lower count of training documents.
Model | Previous F1 during training | New F1 during training | F1 point gains |
CoNLL-2003-EN-600 | 85 | 96.2 | 11.2 |
CoNLL-2003-EN-1000 | 80.8 | 91.5 | 10.7 |
CoNLL-2003-EN-2000 | 92.2 | 94.1 | 1.9 |
CoNLL-2003-ES-600 | 81.3 | 86.5 | 5.2 |
CoNLL-2003-ES-1000 | 85.3 | 92.7 | 7.4 |
CoNLL-2003-ES-2000 | 86.1 | 87.2 | 1.1 |
SNIPS-Weather-600 | 74.7 | 92.1 | 17.4 |
SNIPS-Weather-1000 | 93.1 | 94.8 | 1.7 |
SNIPS-Weather-2000 | 92.1 | 95.9 | 3.8 |
Next, we report the evaluation on a blind test set that was split before the training process from the dataset.
Previous model with at least 200 annotations | New (improved) model with approximately 100 annotations | |||||
Dataset | Number of entities | F1 | Blind test set F1 | F1 | Blind test set F1 | F1 point gains on blind test set |
CoNLL-2003 – English | 3 | 84.9 | 79.4 | 90.2 | 87.9 | 8.5 |
CoNLL-2003 – Spanish | 4 | 85.8 | 76.3 | 90.4 | 81.8 | 5.5 |
SNIPS-Weather | 6 | 74.74 | 80.64 | 92.14 | 93.6 | 12.96 |
Overall, we observe an improvement in F1 scores with the new model even with half the number of annotations provided, as seen in the preceding table.
Continued improvement with more data
In addition to the improved F1 scores at lower limits, we noticed a trend where the new model’s accuracy measured with the blind test dataset continued to improve as we trained with increased annotations. For this test, we considered the SNIPS GetWeather
and AddToPlaylist
datasets.
The following graph shows a distribution of absolute blind test F1 scores for models trained with different datasets and annotation counts.
We generated the following metrics during training and inference for the SNIPS-AddToPlaylist
model trained with 250 documents in the new Amazon Comprehend CER model.
SNIPS-AddToPlaylist metrics at training time
SNIPS-AddToPlaylist inference metrics with blind test dataset
Conclusion
In our experiments with the model improvements in Amazon Comprehend CER, we observe accuracy improvements with fewer annotations and lower document volumes. Now, we consistently see increased accuracy across multiple datasets even with half the number of data samples. We continue to see improvements to the F1 score as we trained models with different dataset sampling configurations, including multi-lingual models. With this updated model, Amazon Comprehend makes it easy to train custom entity recognition models. Limits have been lowered to 100 annotations per entity and 250 documents for training while offering improved accuracy with your models. You can start training custom entity models on the Amazon Comprehend console or through the API.
About the Authors
Prem Ranga is an Enterprise Solutions Architect based out of Houston, Texas. He is part of the Machine Learning Technical Field Community and loves working with customers on their ML and AI journey. Prem is passionate about robotics, is an Autonomous Vehicles researcher, and also built the Alexa-controlled Beer Pours in Houston and other locations.
Chethan Krishna is a Senior Partner Solutions Architect in India. He works with Strategic AWS Partners for establishing a robust cloud competency, adopting AWS best practices and solving customer challenges. He is a builder and enjoys experimenting with AI/ML, IoT and Analytics.
Mona Mona is an AI/ML Specialist Solutions Architect based out of Arlington, VA. She helps customers adopt machine learning on a large scale. She is passionate about NLP and ML Explainability areas in AI/ML.
A Dataset Exploration Case Study with Know Your Data
Posted by Mark Díaz and Emily Denton, Research Scientists, Google Research, Ethical AI Team
Data underlies much of machine learning (ML) research and development, helping to structure what a machine learning algorithm learns and how models are evaluated and benchmarked. However, data collection and labeling can be complicated by unconscious biases, data access limitations and privacy concerns, among other challenges. As a result, machine learning datasets can reflect unfair social biases along dimensions of race, gender, age, and more.
Methods of examining datasets that can surface information about how different social groups are represented within are a key component of ensuring development of ML models and datasets is aligned with our AI Principles. Such methods can inform the responsible use of ML datasets and point toward potential mitigations of unfair outcomes. For example, prior research has demonstrated that some object recognition datasets are biased toward images sourced from North America and Western Europe, prompting Google’s Crowdsource effort to balance out image representations in other parts of the world.
Today, we demonstrate some of the functionality of a dataset exploration tool, Know Your Data (KYD), recently introduced at Google I/O, using the COCO Captions dataset as a case study. Using this tool, we find a range of gender and age biases in COCO Captions — biases that can be traced to both dataset collection and annotation practices. KYD is a dataset analysis tool that complements the growing suite of responsible AI tools being developed across Google and the broader research community. Currently, KYD only supports analysis of a small set of image datasets, but we’re working hard to make the tool accessible beyond this set.
Introducing Know Your Data
Know Your Data helps ML research, product and compliance teams understand datasets, with the goal of improving data quality, and thus helping to mitigate fairness and bias issues. KYD offers a range of features that allow users to explore and examine machine learning datasets — users can filter, group, and study correlations based on annotations already present in a given dataset. KYD also presents automatically computed labels from Google’s Cloud Vision API, providing users with a simple way to explore their data based on signals that weren’t originally present in the dataset.
A KYD Case Study
As a case study, we explore some of these features using the COCO Captions dataset, an image dataset that contains five human-generated captions for each of over 300k images. Given the rich annotations provided by free-form text, we focus our analysis on signals already present within the dataset.
Exploring Gender Bias
Previous research has demonstrated undesirable gender biases within computer vision datasets, including pornographic imagery of women and image label correlations that align with harmful gender stereotypes. We use KYD to explore gender biases within COCO Captions by examining gendered correlations within the image captions. We find a gender bias in the depiction of different activities across the images in the dataset, as well as biases relating to how people of different genders are described by annotators.
The first part of our analysis aimed to surface gender biases with respect to different activities depicted in the dataset. We examined images captioned with words describing different activities and analyzed their relation to gendered caption words, such as “man” or “woman”. The KYD Relations tab makes it easy to examine the relation between two different signals in a dataset by visualizing the extent to which two signals co-occur more (or less) than would be expected by chance. Each cell indicates either a positive (blue color) or negative (orange color) correlation between two specific signal values along with the strength of that correlation.
KYD also allows users to filter rows of a relations table based on substring matching. Using this functionality, we initially probed for caption words containing “-ing”, as a simple way to filter by verbs. We immediately saw strong gendered correlations:
![]() |
Using KYD to analyze the relationship between any word and gendered words. Each cell shows if the two respective words co-occur in the same caption more (up arrow) or less often (down arrow) than pure chance. |
Digging further into these correlations, we found that several activities stereotypically associated with women, such as “shopping” and “cooking”, co-occur with images captioned with “women” or “woman” at a higher rate than with images captioned with “men” or “man”. In contrast captions describing many physically intensive activities, such as “skateboarding”, “surfing”, and “snowboarding”, co-occur with images captioned with “man” or “men” at higher rates.
While individual image captions may not use stereotypical or derogatory language, such as with the example below, if certain gender groups are over (or under) represented within a particular activity across the whole dataset, models developed from the dataset risk learning stereotypical associations. KYD makes it easy to surface, quantify, and make plans to mitigate this risk.
![]() |
An image with one of the captions: “Two women cooking in a beige and white kitchen.” Image licensed under CC-BY 2.0. |
In addition to examining biases with respect to the social groups depicted with different activities, we also explored biases in how annotators described the appearance of people they perceived as male or female. Inspired by media scholars who have examined the “male gaze” embedded in other forms of visual media, we examined the frequency with which individuals perceived as women in COCO are described using adjectives that position them as an object of desire. KYD allowed us to easily examine co-occurrences between words associated with binary gender (e.g. “female/girl/woman” vs. “male/man/boy”) and words associated with evaluating physical attractiveness. Importantly, these are captions written by human annotators, who are making subjective assessments about the gender of people in the image and choosing a descriptor for attractiveness. We see that the words “attractive”, “beautiful”, “pretty”, and “sexy” are overrepresented in describing people perceived as women as compared to those perceived as men, confirming what prior work has said about how gender is viewed in visual media.
![]() |
A screenshot from KYD showing the relationship between words that describe attractiveness and gendered words. For example, “attractive” and “male/man/boy” co-occur 12 times, but we expect ~60 times by chance (the ratio is 0.2x). On the other hand, “attractive” and “female/woman/girl” co-occur 2.62 times more than chance. |
KYD also allows us to manually inspect images for each relation by clicking on the relation in question. For example, we can see images whose captions include female terms (e.g. “woman”) and the word “beautiful”.
Exploring Age Bias
Adults older than 65 have been shown to be underrepresented in datasets relative to their presence in the general population — a first step toward improving age representation is to allow developers to assess it in their datasets. By looking at caption words describing different activities and analyzing their relation to caption words describing age, KYD helped us to assess the range of example captions depicting older adults. Having example captions of adults in a range of environments and activities is important for a variety of tasks, such as image captioning or pedestrian detection.
The first trend that KYD made clear is how rarely annotators described people as older adults in captions detailing different activities. The relations tab also shows a trend wherein “elderly”, “old”, and “older” tend not to occur with verbs that describe a variety of physical activities that might be important for a system to be able to detect. Important to note is that, relative to “young”, “old” is more often used to describe things other than people, such as belongings or clothing, so these relations are also capturing some uses that don’t describe people.
![]() |
The relationship between words associated with age and movement from a screenshot of KYD. |
The underrepresentation of captions containing the references to older adults that we examined here could be rooted in a relative lack of images depicting older adults as well as in a tendency for annotators to omit older age-related terms when describing people in images. While manual inspection of the intersection of “old” and “running” shows a negative relation, we notice that it shows no older people and a number of locomotives. KYD makes it easy to quantitatively and qualitatively inspect relations to identify dataset strengths and areas for improvement.
Conclusion
Understanding the contents of ML datasets is a critical first step to developing suitable strategies to mitigate the downstream impact of unfair dataset bias. The above analysis points towards several potential mitigations. For example, correlations between certain activities and social groups, which can lead trained models to reproduce social stereotypes, can be potentially mitigated by “dataset balancing” — increasing the representation of under-represented group/activity combinations. However, mitigations focused exclusively on dataset balancing are not sufficient, as our analysis of how different genders are described by annotators demonstrated. We found annotators’ subjective judgements of people portrayed in images were reflected within the final dataset, suggesting a deeper look at methods of image annotations are needed. One solution for data practitioners who are developing image captioning datasets is to consider integrating guidelines that have been developed for writing image descriptions that are sensitive to race, gender, and other identity categories.
The above case studies highlight only some of the KYD features. For example, Cloud Vision API signals are also integrated into KYD and can be used to infer signals that annotators haven’t labeled directly. We encourage the broader ML community to perform their own KYD case studies and share their findings.
KYD complements other dataset analysis tools being developed across the ML community, including Google’s growing Responsible AI toolkit. We look forward to ML practitioners using KYD to better understand their datasets and mitigate potential bias and fairness concerns. If you have feedback on KYD, please write to knowyourdata-feedback@google.com.
Acknowledgements
The analysis and write-up in this post were conducted with equal contribution by Emily Denton, Mark Díaz, and Alex Hanna. We thank Marie Pellat, Ludovic Peran, Daniel Smilkov, Nikhil Thorat and Tsung-Yi for their contributions to and reviews of this post.