Participating teams reported their progress at a workshop earlier this month.Read More
How AI is making information more useful
Today, there’s more information accessible at people’s fingertips than at any point in human history. And advances in artificial intelligence will radically transform the way we use that information, with the ability to uncover new insights that can help us both in our daily lives and in the ways we are able to tackle complex global challenges.
At our Search On livestream event today, we shared how we’re bringing the latest in AI to Google’s products, giving people new ways to search and explore information in more natural and intuitive ways.
Making multimodal search possible with MUM
Earlier this year at Google I/O, we announced we’ve reached a critical milestone for understanding information with Multitask Unified Model, or MUM for short.
We’ve been experimenting with using MUM’s capabilities to make our products more helpful and enable entirely new ways to search. Today, we’re sharing an early look at what will be possible with MUM.
In the coming months, we’ll introduce a new way to search visually, with the ability to ask questions about what you see. Here are a couple of examples of what will be possible with MUM.
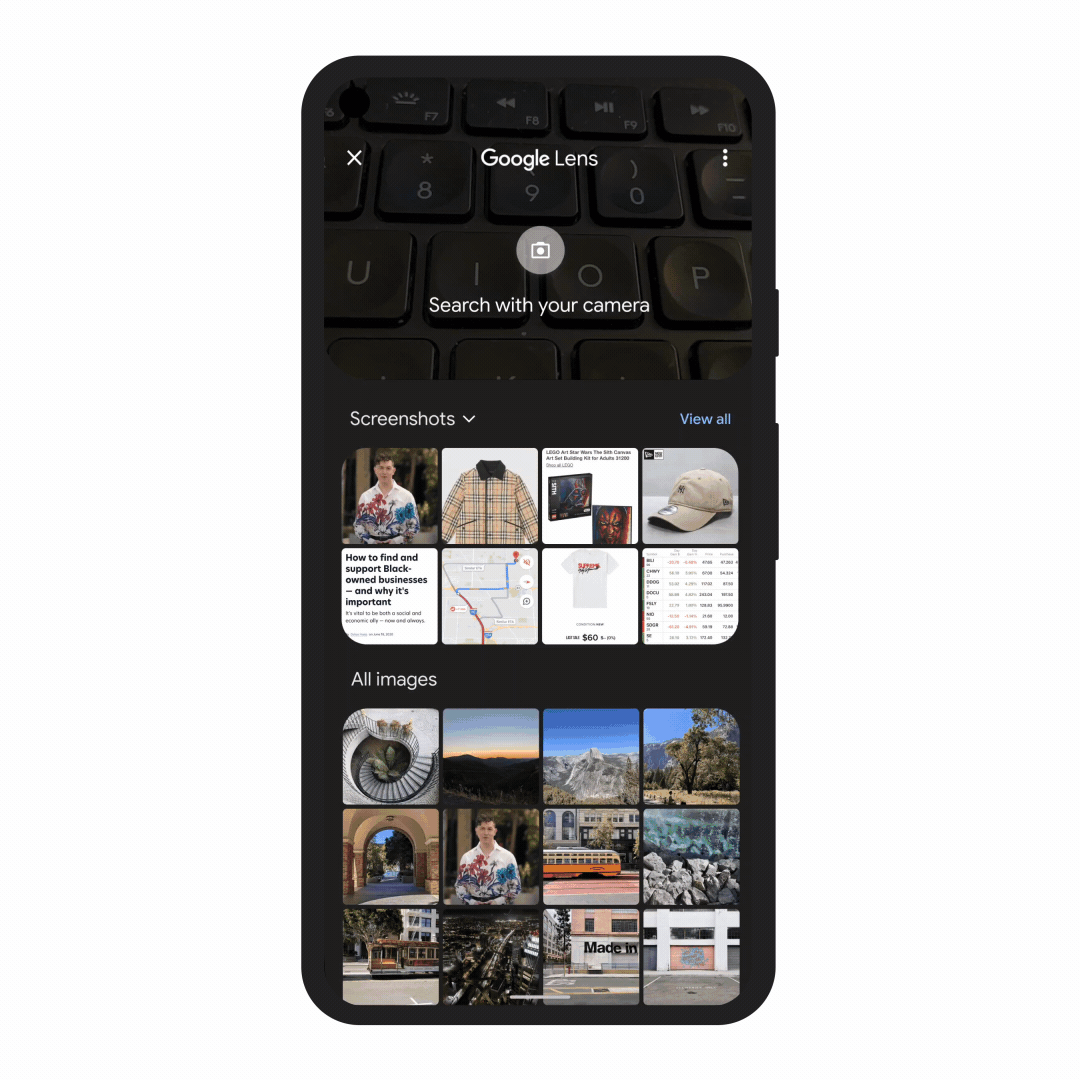
With this new capability, you can tap on the Lens icon when you’re looking at a picture of a shirt, and ask Google to find you the same pattern — but on another article of clothing, like socks. This helps when you’re looking for something that might be difficult to describe accurately with words alone. You could type “white floral Victorian socks,” but you might not find the exact pattern you’re looking for. By combining images and text into a single query, we’re making it easier to search visually and express your questions in more natural ways.
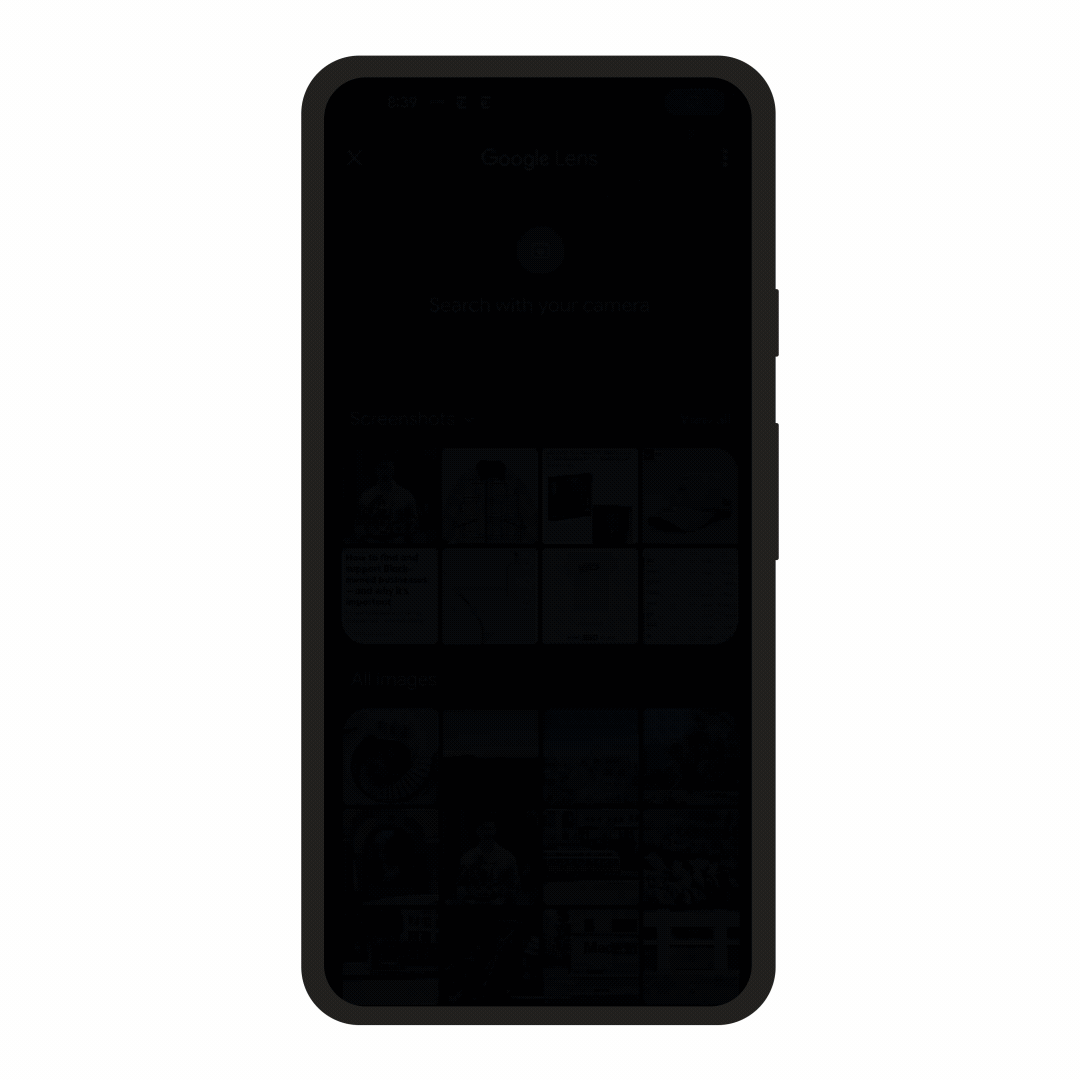
Some questions are even trickier: Your bike has a broken thingamajig, and you need some guidance on how to fix it. Instead of poring over catalogs of parts and then looking for a tutorial, the point-and-ask mode of searching will make it easier to find the exact moment in a video that can help.
Helping you explore with a redesigned Search page
We’re also announcing how we’re applying AI advances like MUM to redesign Google Search. These new features are the latest steps we’re taking to make searching more natural and intuitive.
First, we’re making it easier to explore and understand new topics with “Things to know.” Let’s say you want to decorate your apartment, and you’re interested in learning more about creating acrylic paintings.
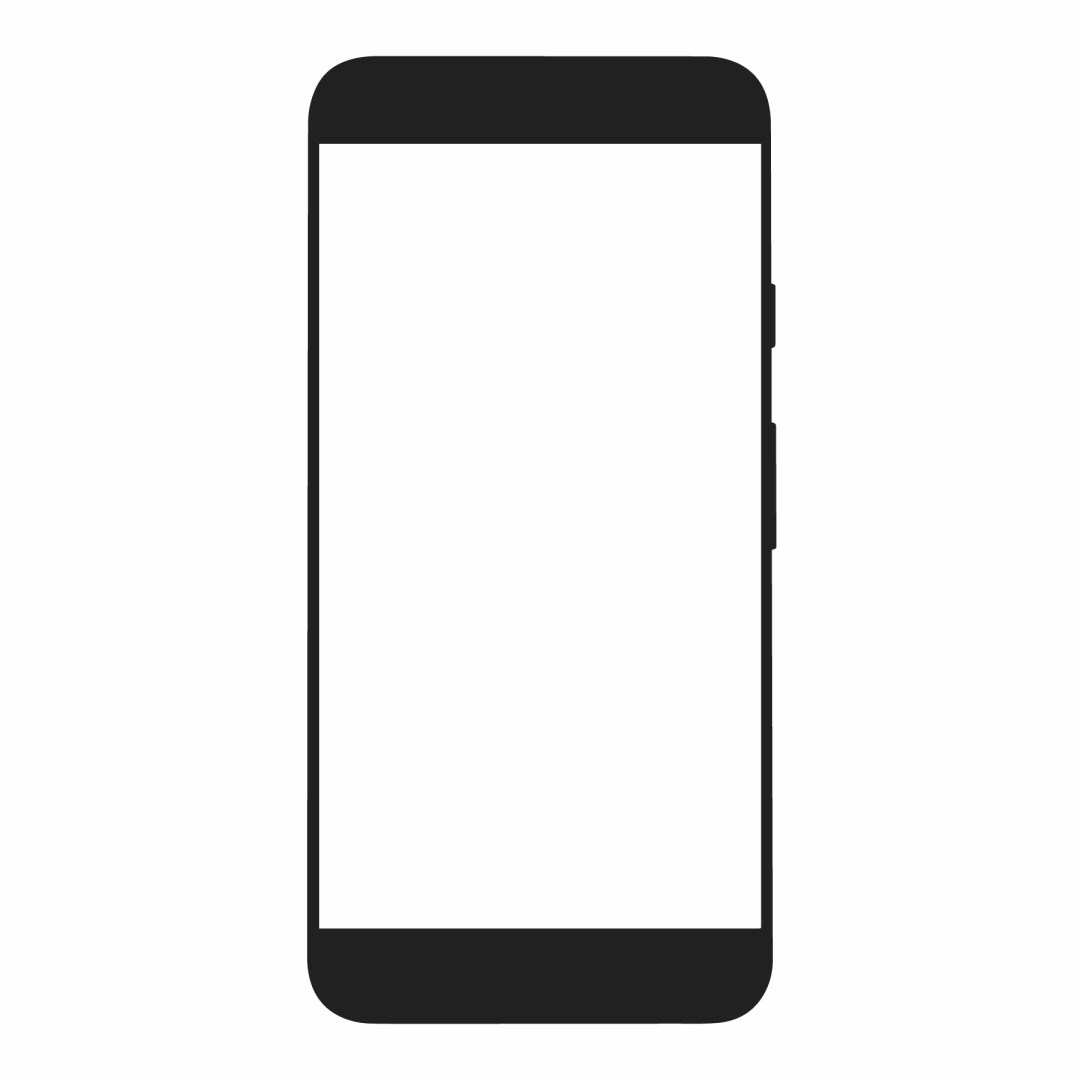
If you search for “acrylic painting,” Google understands how people typically explore this topic, and shows the aspects people are likely to look at first. For example, we can identify more than 350 topics related to acrylic painting, and help you find the right path to take.
We’ll be launching this feature in the coming months. In the future, MUM will unlock deeper insights you might not have known to search for — like “how to make acrylic paintings with household items” — and connect you with content on the web that you wouldn’t have otherwise found.
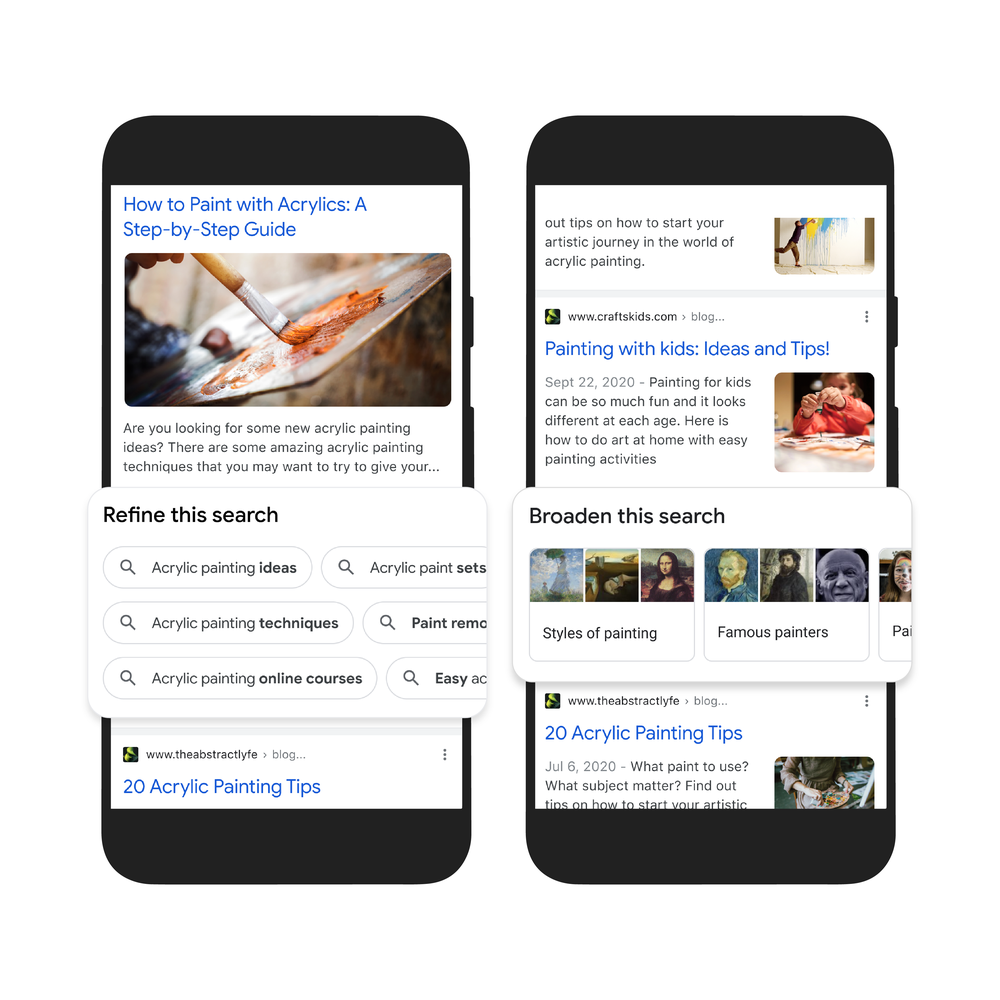
Second, to help you further explore ideas, we’re making it easy to zoom in and out of a topic with new features to refine and broaden searches.
In this case, you can learn more about specific techniques, like puddle pouring, or art classes you can take. You can also broaden your search to see other related topics, like other painting methods and famous painters. These features will launch in the coming months.
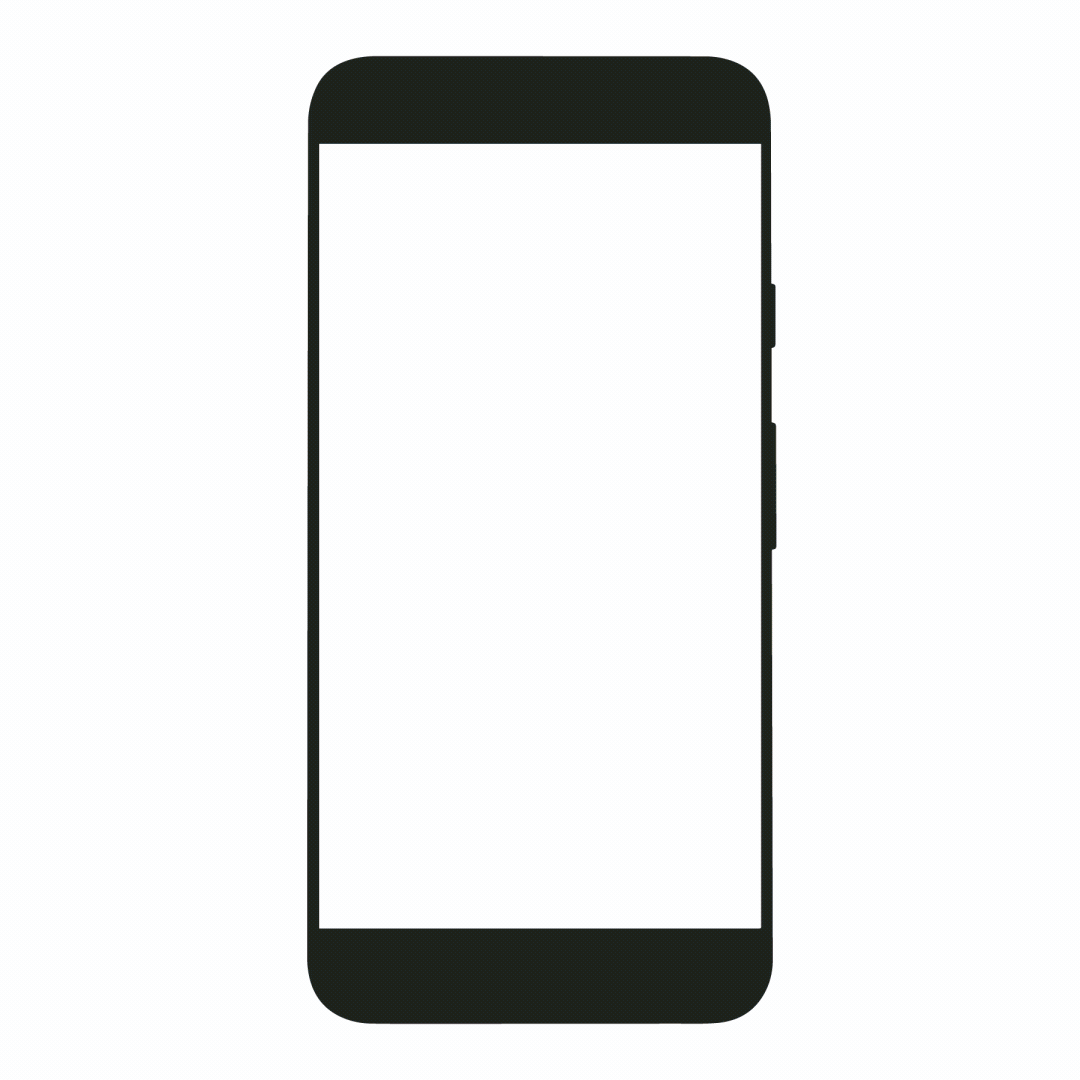
Third, we’re making it easier to find visual inspiration with a newly designed, browsable results page. If puddle pouring caught your eye, just search for “pour painting ideas” to see a visually rich page full of ideas from across the web, with articles, images, videos and more that you can easily scroll through.
This new visual results page is designed for searches that are looking for inspiration, like “Halloween decorating ideas” or “indoor vertical garden ideas,” and you can try it today.
Get more from videos
We already use advanced AI systems to identify key moments in videos, like the winning shot in a basketball game, or steps in a recipe. Today, we’re taking this a step further, introducing a new experience that identifies related topics in a video, with links to easily dig deeper and learn more.
Using MUM, we can even show related topics that aren’t explicitly mentioned in the video, based on our advanced understanding of information in the video. In this example, while the video doesn’t say the words “macaroni penguin’s life story,” our systems understand that topics contained in the video relate to this topic, like how macaroni penguins find their family members and navigate predators. The first version of this feature will roll out in the coming weeks, and we’ll add more visual enhancements in the coming months.
Across all these MUM experiences, we look forward to helping people discover more web pages, videos, images and ideas that they may not have come across or otherwise searched for.
A more helpful Google
The updates we’re announcing today don’t end with MUM, though. We’re also making it easier to shop from the widest range of merchants, big and small, no matter what you’re looking for. And we’re helping people better evaluate the credibility of information they find online. Plus, for the moments that matter most, we’re finding new ways to help people get access to information and insights.
All this work not only helps people around the world, but creators, publishers and businesses as well. Every day, we send visitors to well over 100 million different websites, and every month, Google connects people with more than 120 million businesses that don’t have websites, by enabling phone calls, driving directions and local foot traffic.
As we continue to build more useful products and push the boundaries of what it means to search, we look forward to helping people find the answers they’re looking for, and inspiring more questions along the way.
A more useful way to measure robotic localization error
Measuring the displacement between location estimates derived from different camera views can help enforce the local consistency vital to navigation.Read More
Nowcasting the next hour of rain
Our lives are dependent on the weather. At any moment in the UK, according to one study, one third of the country has talked about the weather in the past hour, reflecting the importance of weather in daily life. Amongst weather phenomena, rain is especially important because of its influence on our everyday decisions. Should I take an umbrella? How should we route vehicles experiencing heavy rain? What safety measures do we take for outdoor events? Will there be a flood? Our latest research and state-of-the-art model advances the science of Precipitation Nowcasting, which is the prediction of rain (and other precipitation phenomena) within the next 1-2 hours. In a paper written in collaboration with the Met Office and published in Nature, we directly tackle this important grand challenge in weather prediction. This collaboration between environmental science and AI focuses on value for decision-makers, opening up new avenues for the nowcasting of rain, and points to the opportunities for AI in supporting our response to the challenges of decision-making in an environment under constant change.Read More
Nowcasting the Next Hour of Rain
Our latest research and state-of-the-art model advances the science of Precipitation Nowcasting.Read More
Astro’s Intelligent Motion brings state-of-the-art navigation to the home
“Body language” and an awareness of social norms help Amazon’s new household robot integrate gracefully into the home.Read More
The science behind visual ID
A new opt-in feature for Echo Show and Astro provides more-personalized content and experiences for customers who choose to enroll.Read More
How Amazon is using self-service to democratize AI
Preference teaching for Alexa, Alexa Custom Sound Event Detection, and Ring Custom Event Alerts let customers configure machine learning models.Read More
Making roadway spending more sustainable
The share of federal spending on infrastructure has reached an all-time low, falling from 30 percent in 1960 to just 12 percent in 2018.
While the nation’s ailing infrastructure will require more funding to reach its full potential, recent MIT research finds that more sustainable and higher performing roads are still possible even with today’s limited budgets.
The research, conducted by a team of current and former MIT Concrete Sustainability Hub (MIT CSHub) scientists and published in Transportation Research D, finds that a set of innovative planning strategies could improve pavement network environmental and performance outcomes even if budgets don’t increase.
The paper presents a novel budget allocation tool and pairs it with three innovative strategies for managing pavement networks: a mix of paving materials, a mix of short- and long-term paving actions, and a long evaluation period for those actions.
This novel approach offers numerous benefits. When applied to a 30-year case study of the Iowa U.S. Route network, the MIT CSHub model and management strategies cut emissions by 20 percent while sustaining current levels of road quality. Achieving this with a conventional planning approach would require the state to spend 32 percent more than it does today. The key to its success is the consideration of a fundamental — but fraught — aspect of pavement asset management: uncertainty.
Predicting unpredictability
The average road must last many years and support the traffic of thousands — if not millions — of vehicles. Over that time, a lot can change. Material prices may fluctuate, budgets may tighten, and traffic levels may intensify. Climate (and climate change), too, can hasten unexpected repairs.
Managing these uncertainties effectively means looking long into the future and anticipating possible changes.
“Capturing the impacts of uncertainty is essential for making effective paving decisions,” explains Fengdi Guo, the paper’s lead author and a departing CSHub research assistant.
“Yet, measuring and relating these uncertainties to outcomes is also computationally intensive and expensive. Consequently, many DOTs [departments of transportation] are forced to simplify their analysis to plan maintenance — often resulting in suboptimal spending and outcomes.”
To give DOTs accessible tools to factor uncertainties into their planning, CSHub researchers have developed a streamlined planning approach. It offers greater specificity and is paired with several new pavement management strategies.
The planning approach, known as Probabilistic Treatment Path Dependence (PTPD), is based on machine learning and was devised by Guo.
“Our PTPD model is composed of four steps,” he explains. “These steps are, in order, pavement damage prediction; treatment cost prediction; budget allocation; and pavement network condition evaluation.”
The model begins by investigating every segment in an entire pavement network and predicting future possibilities for pavement deterioration, cost, and traffic.
“We [then] run thousands of simulations for each segment in the network to determine the likely cost and performance outcomes for each initial and subsequent sequence, or ‘path,’ of treatment actions,” says Guo. “The treatment paths with the best cost and performance outcomes are selected for each segment, and then across the network.”
The PTPD model not only seeks to minimize costs to agencies but also to users — in this case, drivers. These user costs can come primarily in the form of excess fuel consumption due to poor road quality.
“One improvement in our analysis is the incorporation of electric vehicle uptake into our cost and environmental impact predictions,” Randolph Kirchain, a principal research scientist at MIT CSHub and MIT Materials Research Laboratory (MRL) and one of the paper’s co-authors. “Since the vehicle fleet will change over the next several decades due to electric vehicle adoption, we made sure to consider how these changes might impact our predictions of excess energy consumption.”
After developing the PTPD model, Guo wanted to see how the efficacy of various pavement management strategies might differ. To do this, he developed a sophisticated deterioration prediction model.
A novel aspect of this deterioration model is its treatment of multiple deterioration metrics simultaneously. Using a multi-output neural network, a tool of artificial intelligence, the model can predict several forms of pavement deterioration simultaneously, thereby, accounting for their correlations among one another.
The MIT team selected two key metrics to compare the effectiveness of various treatment paths: pavement quality and greenhouse gas emissions. These metrics were then calculated for all pavement segments in the Iowa network.
Improvement through variation
The MIT model can help DOTs make better decisions, but that decision-making is ultimately constrained by the potential options considered.
Guo and his colleagues, therefore, sought to expand current decision-making paradigms by exploring a broad set of network management strategies and evaluating them with their PTPD approach. Based on that evaluation, the team discovered that networks had the best outcomes when the management strategy includes using a mix of paving materials, a variety of long- and short-term paving repair actions (treatments), and longer time periods on which to base paving decisions.
They then compared this proposed approach with a baseline management approach that reflects current, widespread practices: the use of solely asphalt materials, short-term treatments, and a five-year period for evaluating the outcomes of paving actions.
With these two approaches established, the team used them to plan 30 years of maintenance across the Iowa U.S. Route network. They then measured the subsequent road quality and emissions.
Their case study found that the MIT approach offered substantial benefits. Pavement-related greenhouse gas emissions would fall by around 20 percent across the network over the whole period. Pavement performance improved as well. To achieve the same level of road quality as the MIT approach, the baseline approach would need a 32 percent greater budget.
“It’s worth noting,” says Guo, “that since conventional practices employ less effective allocation tools, the difference between them and the CSHub approach should be even larger in practice.”
Much of the improvement derived from the precision of the CSHub planning model. But the three treatment strategies also play a key role.
“We’ve found that a mix of asphalt and concrete paving materials allows DOTs to not only find materials best-suited to certain projects, but also mitigates the risk of material price volatility over time,” says Kirchain.
It’s a similar story with a mix of paving actions. Employing a mix of short- and long-term fixes gives DOTs the flexibility to choose the right action for the right project.
The final strategy, a long-term evaluation period, enables DOTs to see the entire scope of their choices. If the ramifications of a decision are predicted over only five years, many long-term implications won’t be considered. Expanding the window for planning, then, can introduce beneficial, long-term options.
It’s not surprising that paving decisions are daunting to make; their impacts on the environment, driver safety, and budget levels are long-lasting. But rather than simplify this fraught process, the CSHub method aims to reflect its complexity. The result is an approach that provides DOTs with the tools to do more with less.
This research was supported through the MIT Concrete Sustainability Hub by the Portland Cement Association and the Ready Mixed Concrete Research and Education Foundation.
Optical character recognition with TensorFlow Lite: A new example app
Posted by Wei Wei, TensorFlow Developer Advocate
As the old adage goes, “a picture is worth a thousand words.” Images are rich in visual information, but sometimes the key is with the text within. While it is easy for literate human beings to read words embedded in images, how do we use computer vision and machine learning to teach computers to do so?
Today, we are going to show you how to use TensorFlow Lite to extract text from images on Android devices. We will walk you through the key steps of the Optical Character Recognition (OCR) Android app that we recently open sourced here, which you can refer to for the complete code. You can see how the app extracts the product names from three Google product logos in the animation below.
The process of recognizing text from images is called Optical Character Recognition and is widely used in many domains. For example, Google Maps uses OCR technology to automatically extract information from the geo-located imagery to improve Google Maps.
Generally speaking, OCR is a pipeline with multiple steps. Usually they consist of text detection and text recognition:
- Use a text detection model to find out bounding boxes around text;
- Do some post-processing to transform the bounding boxes;
- Transform the images within those bounding boxes into grayscale, so that a text recognition model can map out the words and numbers.
In our case, we are going to leverage the text detection and text recognition models from TensorFlow Hub. There are several different model versions for speed / accuracy tradeoffs; we use the float16 quantized models here. For more information on model quantization, please refer to the TensorFlow Lite quantization section. We also use OpenCV, which is a widely used computer vision library for Non-Maximum Suppression (NMS) and perspective transformation (we’ll expand on this later) to post-process detection results. In addition, we use the TFLite Support Library to grayscale and normalize the images.
![]() |
OCR pipeline from text detection, perspective transformation, to recognition. |
For text detection, since the detection model accepts a fixed size of 320×320, we use the TFLite Support Library to resize and normalize the input image:
val imageProcessor =
ImageProcessor.Builder()
.add(ResizeOp(height, width, ResizeOp.ResizeMethod.BILINEAR))
.add(NormalizeOp(means, stds))
.build()
var tensorImage = TensorImage(DataType.FLOAT32)
tensorImage.load(bitmapIn)
tensorImage = imageProcessor.process(tensorImage)
Then we use TFLite to run the detection model:
detectionInterpreter.runForMultipleInputsOutputs(detectionInputs, detectionOutputs)
The output of the detection model is a number of rotated bounding boxes which contain the text in the image. We run Non-Maximum Suppression to identify one bounding box for each text block with OpenCV:
NMSBoxesRotated(
boundingBoxesMat,
detectedConfidencesMat,
detectionConfidenceThreshold.toFloat(),
detectionNMSThreshold.toFloat(),
indicesMat
)
Sometimes texts inside images are distorted (e.g., the ‘kubernetes’ sticker on my laptop) with a perspective angle:
![]() |
Perspective transformation demo |
If we just feed the raw rotated bounding box into the recognition model, the model is unlikely to correctly identify the characters. In this case, we need to use OpenCV to do perspective transformation:
val rotationMatrix = getPerspectiveTransform(srcPtsMat, targetPtsMat)
warpPerspective(
srcBitmapMat,
recognitionBitmapMat,
rotationMatrix,
Size(recognitionImageWidth.toDouble(), recognitionImageHeight.toDouble())
)
After that, we use the TFLite Support Library again to resize, grayscale, and normalize the transformed images inside the bounding boxes:
val imageProcessor =
ImageProcessor.Builder()
.add(ResizeOp(height, width, ResizeOp.ResizeMethod.BILINEAR))
.add(TransformToGrayscaleOp())
.add(NormalizeOp(mean, std))
.build()
Finally, we run the text recognition model, map out the characters and numbers from the model output, and update the app UI:
recognitionInterpreter.run(recognitionTensorImage.buffer, recognitionResult)
var recognizedText = ""
for (k in 0 until recognitionModelOutputSize) {
var alphabetIndex = recognitionResult.getInt(k * 8)
if (alphabetIndex in 0..alphabets.length - 1)
recognizedText = recognizedText + alphabets[alphabetIndex]
}
Log.d("Recognition result:", recognizedText)
if (recognizedText != "") {
ocrResults.put(recognizedText, getRandomColor())
}
That’s it. We are now able to extract text from input images using TFLite within our app.
Finally, if you just want a ready-to-use OCR SDK, Google also offers on-device OCR functionality through ML Kit, which uses TFLite underneath and should be sufficient for most OCR use cases. There are some situations where you may want to build your own OCR solution with TFLite such as:
- You have your own text detection / recognition TFLite models that you would like to use;
- You have special business requirements (e.g. recognizing upside-down text) and need to customize the OCR pipeline;
- You want to support languages not covered by ML Kit;
- Your target user devices that don’t necessarily have Google Play services installed;
- You want to have control over hardware backends (CPU / GPU / etc.) used to run your models.
In these cases, I hope that this tutorial and our example implementation can help you get started on building your own OCR functionality in your app.
You can learn more about OCR with the resources below.
- OpenCV text detection / recognition example
- OCR TFLite community project by @Tulasi123789 and @risingsayak
- OpenCV Text Detection (EAST text detector)
- Keras OCR
- Deep Learning based Text Detection Using OpenCV
- OCR model for reading Captchas with Keras
Acknowledgements
The author would like to thank Tian Lin for the helpful feedback and community contributors @Tulasi123789 and @risingsayak for their prior work on OCR using TFLite (creating and uploading the models to TF Hub, providing accompanying notebooks, and etc.).