Cresta Intelligence, a California-based AI startup, makes businesses radically more productive by using Expertise AI to help sales and service teams unlock their full potential. Cresta is bringing together world-renowned AI thought-leaders, engineers, and investors to create a real-time coaching and management solution that transforms sales and increases service productivity, weeks after application deployment. Cresta enables customers such as Intuit, Cox Communications, and Porsche to realize a 20% improvement in sales conversion rate, 25% greater average order value, and millions of dollars in additional annual revenue.
This post discusses Cresta’s journey as they moved from a multi-cloud environment to consolidating their machine learning (ML) workloads on AWS. It also gives a high-level view of their legacy and current training and inference architectures. Cresta chose to migrate to using Meta’s PyTorch ML framework due to its ease of use, efficiency, and enterprise adoption. This includes their use of TorchServe for ML inference in production.
Machine learning at Cresta
Cresta uses multiple natural language processing (NLP) models in their production applications. The Suggestions model monitors the conversation between the call center agent and the customer and generates a full form response, which the agent can use to respond to the customer. A second model called Smart Compose predicts the next few words to auto-complete the agent’s response while typing. Cresta also uses other ML models for intent classification and named entity recognition.
Cresta was born in the cloud and initially used multiple public clouds to build architectures to store, manage, and process datasets, and to train and deploy ML models. As Cresta’s development and production workloads grew in size, managing resources, moving data, and maintaining ML pipelines across multiple clouds became increasingly tedious, time-consuming to manage, and added to operational costs. As a result, Cresta took a holistic view of their siloed ML pipelines and chose AWS to host all their ML training and inference workloads.
“Using multiple cloud providers required us to effectively double our efforts on security and compliance, as each cloud provider needed similar effort to ensure strict security limitations,” says Jack Lindamood, Head of Infrastructure at Cresta. “It also split our infrastructure expertise as we needed to become experts in services provided by multiple clouds. We chose to consolidate ML workloads on AWS because of our trust in their commitment to backward-compatibility, history of service availability, and strong customer support on both the account and technical side.”
Multi-cloud environments and workload consolidation
At a high level, the following diagram captures Cresta’s previous architecture spanning two public cloud service providers. The main datasets were hosted and maintained on Amazon Aurora, and training was performed outside AWS, on another cloud service provider, using custom chips. Based on training requirements, a subset of the data would be curated from Aurora, copied to Amazon Simple Storage Service (Amazon S3), then exported out of AWS into the other cloud where Cresta trained their NLP models. The size of data moved each time ranged from 1–100 GB. The ML training pipeline was built around Argo Workflows, which is an open-source workflow engine where each step in a workflow is implemented in a container. Once trained, the models were automatically evaluated for accuracy before manual checks. The models that passed this validation were imported back to AWS, containerized, and deployed into production using Amazon Elastic Kubernetes Service (Amazon EKS). Cresta’s production inference was hosted on AWS.
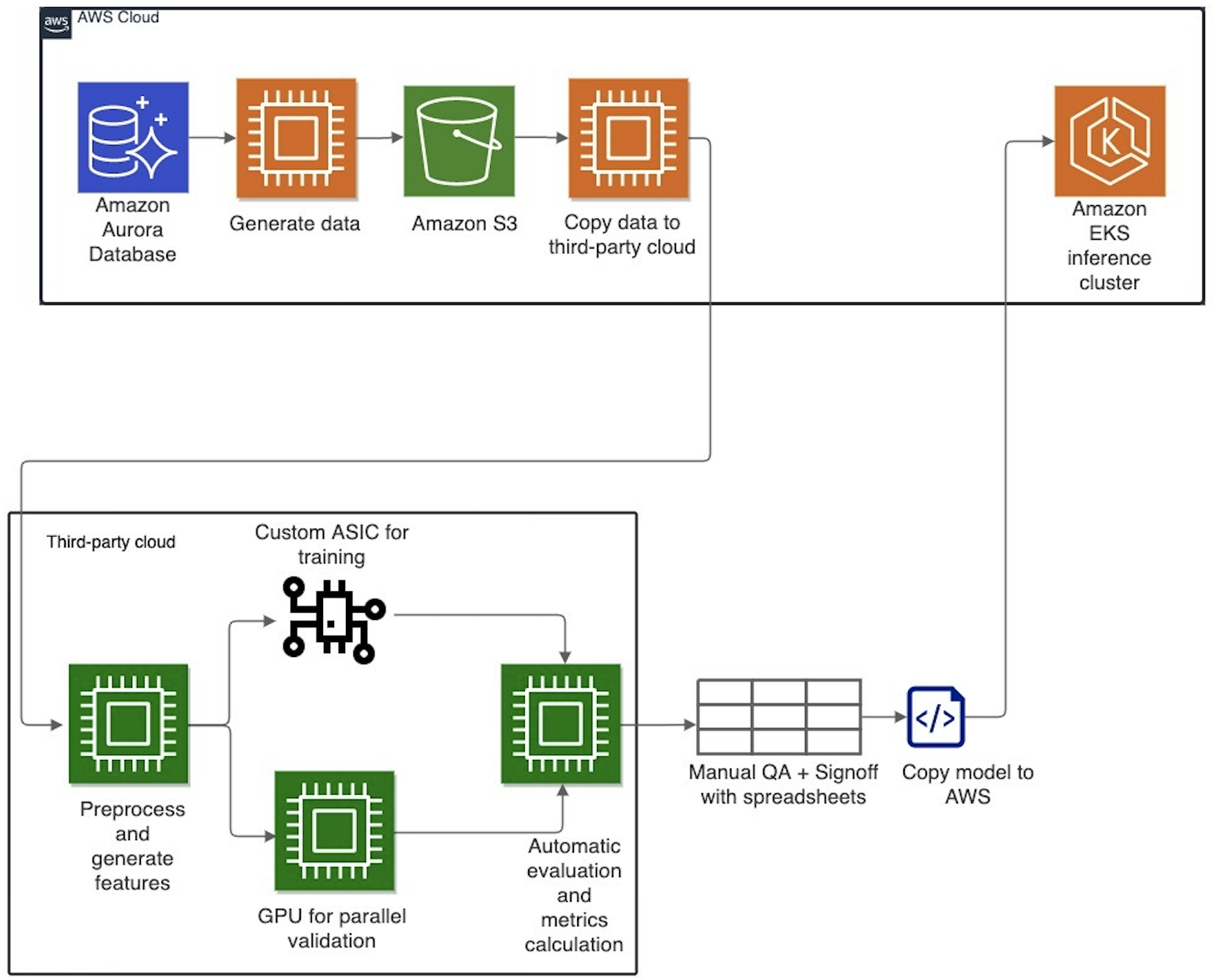
This approach initially worked well for Cresta when the number of datasets and models were limited and performance requirements were low. As the complexity of their applications grew over time, Cresta faced multiple challenges in managing environments on two cloud providers. Security audits had to be performed on both cloud environments, which prolonged release cycles. Keeping the datasets current while moving large amounts of data and trained models between environments was challenging. It also became increasingly difficult to maintain the system’s architecture—the workflow often broke at the cloud boundaries, and resource partitioning between clouds was difficult to optimize. This multi-cloud complexity prevented Cresta from scaling faster and cost-effectively.
To overcome these challenges, Cresta decided to consolidate all their ML workloads on AWS. The key drivers to choosing AWS for all development and production ML workloads were AWS’s breadth of feature-rich services like Amazon Elastic Compute Cloud (Amazon EC2), Amazon S3, Amazon EKS, EC2 Spot Instances, and databases, the built-in cost-optimization features in these services, native support for ML frameworks like PyTorch, and superior technical support. The AWS team worked closely with Cresta to architect the ML training pipeline with Amazon EKS and Spot Instances, and optimized the model training and inference performance. In addition to developing custom ML models, Cresta uses NLP models from Hugging Face, which are supported on AWS GPU instances out of the box for training and inference. To train these models on AWS, Cresta used P3 instances (based on NVIDIA V100 GPUs) of varying sizes.
As a result of this migration, the teams at Cresta no longer had to worry about managing ML pipelines across separate clouds, thereby significantly improving productivity. The Amazon Aurora PostgreSQL database was integrated into the development pipeline, removing the need to use an intermediate storage system to save results or to export datasets externally. Dataset generation, model training, and inferencing are now all performed on the same cloud environment, which has simplified operations, improved reliability, and reduced the complexity of the build and deploy toolchain.
Model training and validation on AWS
The following figure represents the development and training pipeline after the migration to AWS. The pipeline uses Argo Workflows, an open-source container-native workflow engine for orchestrating parallel jobs in Kubernetes. Argo Workflows is deployed on Amazon EKS in a Multi-AZ model.
For the Suggestions model use case, Cresta uses chat data for training, and these datasets are stored in the Aurora database. When a model is ready to be trained, data generation scripts query the database, identify the datasets, and develop a snapshot of the dataset for training. C5.4xlarge instances are used to handle these operations. The preprocessing step converts the dataset to a low level where it is ready to be fed to the model. Training language models requires two preprocessing steps: serialization and tokenization. Structured data is converted to a single stream of characters, finalizing the string representation of the data. This is followed by the tokenization step, where the serial string representation is converted to a vector of integers. Preprocessing data helps accelerate the training process and hyperparameter sweeps. To train the Suggestions models, Cresta serializes data during preprocessing. Tokenization is handled during the training phase.
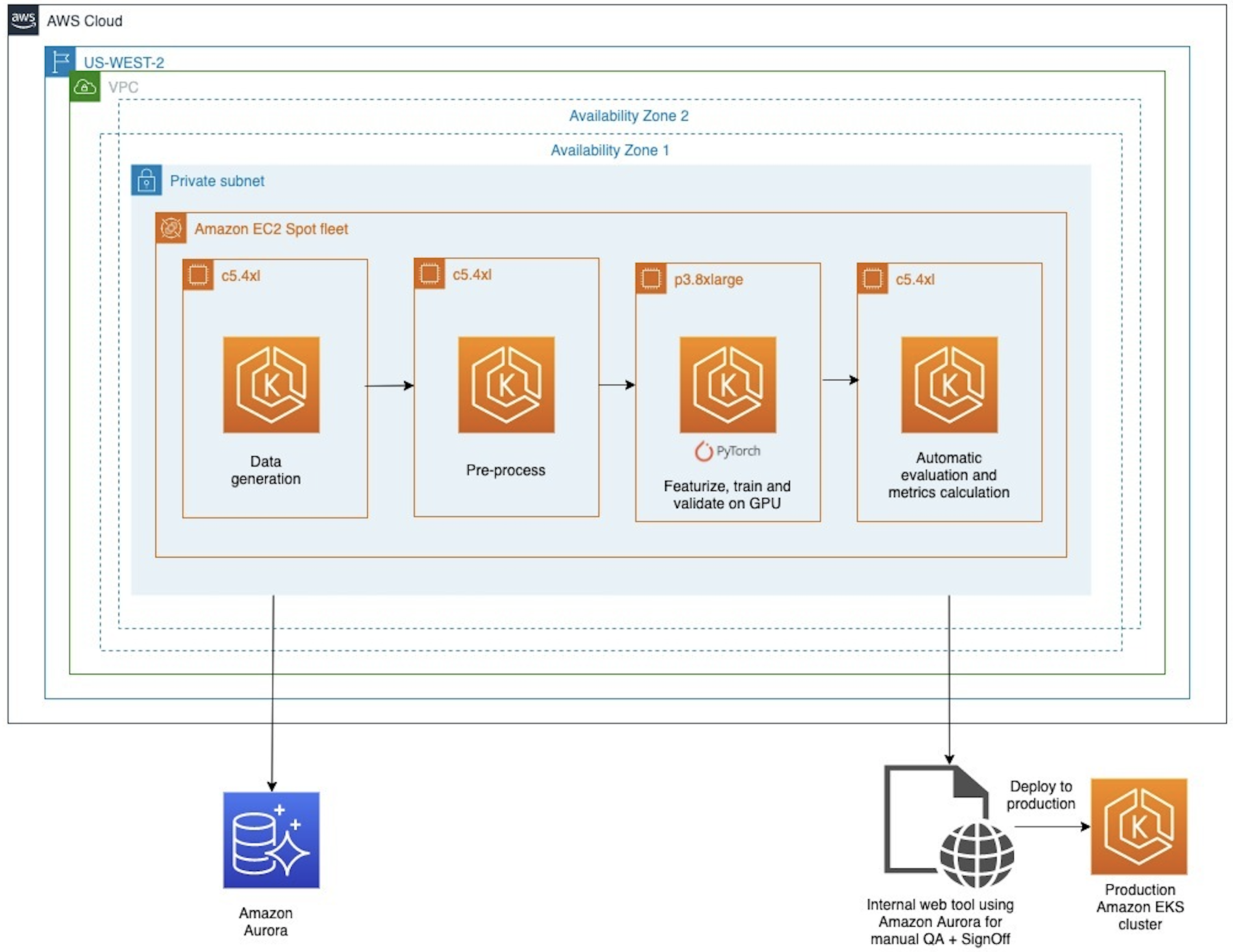
During training, a blind validation of the model is performed over a huge dataset of past chats during the epochal training. The epochal training continues only when the model shows improvement, otherwise the training step is stopped early, thereby preserving compute resources.
In the legacy architecture, model training was performed on a custom training chip followed by a large model validation step to check for accuracy improvement at the end of each epoch. Because the validation dataset was large, model validation couldn’t be performed on the same custom training chip, and had to be performed across multiple GPUs. This approach had single points of failure that could stall the training job. This was because the process required the launch of asynchronous threads to monitor the validation process and periodically poll to check for completion. Using the same hardware accelerator for both training and validation allows for seamless management of this process. After the training and validation steps are performed, manual verification of the training results is performed before deploying the model to the production environment.
To optimize for compute costs for the training process, Cresta used EC2 Spot Instances, which is spare Amazon EC2 capacity available at up to 90% discount compared to On-Demand pricing. For production inference workloads, Cresta uses G4dn instances, which are the industry’s most cost-effective and versatile GPU instances for deploying ML models such as image classification, object detection, and speech recognition. To minimize interruptions, Cresta uses a launch template that specifies multiple instance sizes, including g4dn.xlarge and g4dn.2xlarge. Cresta uses checkpoints and dataset loading from Amazon S3 to allow for model training to be restarted from the point of interruption. This makes it possible to train models efficiently with EC2 Spot Instances, which can be reclaimed with a 2-minute notice.
Model inference on AWS
The trained models are stored on Amazon S3 and are served using PyTorch TorchServe on an Amazon EKS cluster using G4dn instances (NVIDIA T4 GPUs) instances. The cluster is deployed across multiple Availability Zones, and the node groups include GPUs to enable high throughput and low-latency inferences. The model server pods are deployed on these nodes and are horizontally scaled to meet the throughput requirements of any given customer. As the models get retrained, the pods are restarted to pick up and serve the latest models. One Amazon EKS cluster serves all the customers, and customers are logically separated based on the Kubernetes namespace.
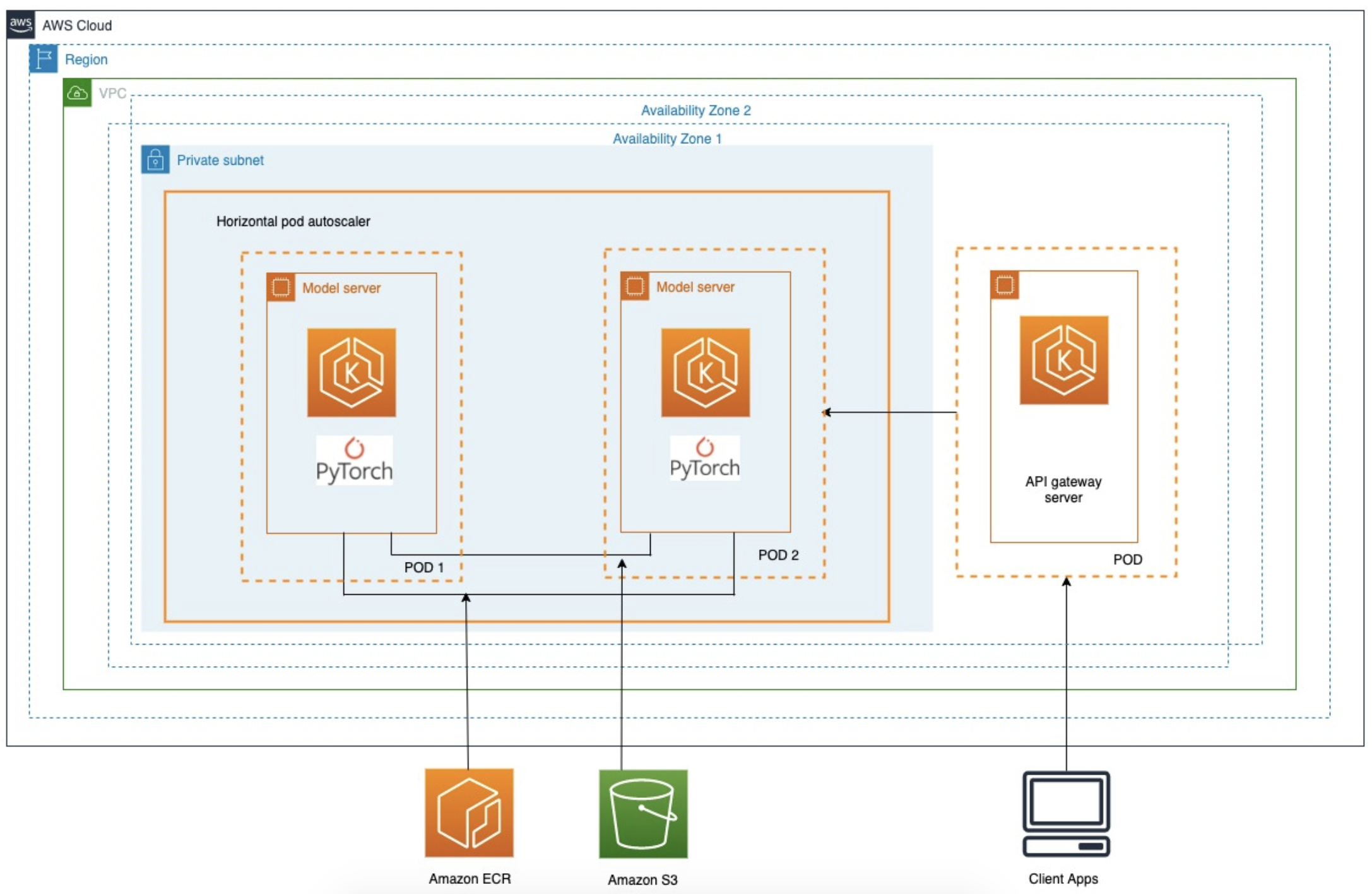
Migration to PyTorch
To support the growing capabilities of their products, Cresta needed to use and fine-tune newer NLP models faster. PyTorch, being popular among the research community, drives much of the innovation in NLP and natural language understanding (NLU) areas. Cresta handpicks NLP models from Hugging Face to retool and fine-tune for reuse, and most models available are based on PyTorch. Lastly, Cresta’s ML teams found PyTorch to be simpler than other frameworks to learn, ramp up, and build on.
“We are moving to PyTorch because most research in the NLP world is migrating to PyTorch,” says Saurabh Misra, AI Lead at Cresta. “A large ecosystem around PyTorch, like the Hugging Face library, enables us to quickly utilize the latest advancements in NLP without rewriting code. PyTorch is also very developer friendly and allows us to develop new models quickly with its ease of use, model debuggability, and support for efficient deployments.”
Because of these reasons, Cresta has chosen to migrate all their ML workloads to use PyTorch for model training and inference, aligning with the ongoing industry trend. Specifically, Cresta uses parallel training on 4 GPUs using torch.nn.DataParallel provided with the Hugging Face Trainer. Before using PyTorch, Cresta had to develop custom implementations of parallel training. This requirement was eliminated with the use of PyTorch, because PyTorch enables the implementation of a variety of training backends and methods, essentially for free. For large-scale inference in production, Cresta uses TorchServe as a model server because of its ease of use and out-of-the-box monitoring of the model, which helps with auto scaling the deployment according to the traffic.
Conclusion and next steps
In this post, we discussed how Cresta moved from a multi-cloud environment to consolidating their ML workloads on AWS. By moving all development and production ML workloads to AWS, Cresta is able to streamline efforts, better optimize for cost, and take advantage of the breadth and depth of AWS services. To further improve performance and cost-effectiveness, Cresta is investigating the following topics:
- Pack multiple models into a single chip using bin-packing for optimal use of resources (memory and compute). This also helps with A/B tests on model performance.
- Deploy models for inference using AWS Inferentia as a way to improve inference performance while keeping costs low.
- Investigate different ways of static compilation of model graphs to reduce the compute required during inference. This will further improve the cost-effectiveness of Cresta’s deployments.
To dive deeper into developing scalable ML architectures with EKS, please refer these two reference architectures – distributed training with TorchElastic and serving 3000 models on EKS with AWS Inferentia.
The content and opinions in this post are those of the third-party author and AWS is not responsible for the content or accuracy of this post.
About the Authors
Jaganath Achari is a Sr. Startup Solutions Architect at Amazon Web Services based out of San Francisco. He focuses on providing technical guidance to startup customers, helping them architect and build secure and scalable solutions on AWS. Outside of work, Jaganath is an amateur astronomer with an interest in deep sky astrophotography.
Sundar Ranganathan is the Head of Business Development, ML Frameworks on the Amazon EC2 team. He focuses on large-scale ML workloads across AWS services like Amazon EKS, Amazon ECS, Elastic Fabric Adapter, AWS Batch, and Amazon SageMaker. His experience includes leadership roles in product management and product development at NetApp, Micron Technology, Qualcomm, and Mentor Graphics.
Mahadevan Balasubramaniam is a Principal Solutions Architect for Autonomous Computing with nearly 20 years of experience in the area of physics-infused deep learning, building, and deploying digital twins for industrial systems at scale. Mahadevan obtained his PhD in Mechanical Engineering from the Massachusetts Institute of Technology and has over 25 patents and publications to his credit.
Saurabh Misra is a Staff Machine Learning Engineer at Cresta. He currently works on creating conversational technologies to make customer care organizations highly effective and efficient. Outside of work, he loves to play the drums and read books.
Jack Lindamood is the Head of Infrastructure at Cresta. In his spare time, he enjoys basketball and watching Esports.
Read More