Step inside an auto assembly plant. See workers ratcheting down nuts to bolts. Hear the whirring of air tools. Watch pristine car bodies gliding along the line and robots rolling up with parts.
Now, fire up its digital twin in 3D online. See animated digital humans at work in the exact same, but digital version of the plant. Drag and drop in robots to move heavy materials, and run simulations for optimizations, taking in real-time factory floor data for improvements. That’s a digital twin.
A digital twin is a virtual representation — a true-to-reality simulation of physics and materials — of a real-world physical asset or system, which is continuously updated.
Digital twins aren’t just for inanimate objects and people. They can be a virtual representation of computer networking architecture used as a sandbox for cyberattack simulations. They can replicate a fulfillment center process to test out human-robot interactions before activating certain robot functions in live environments. The applications are as wide as the imagination.
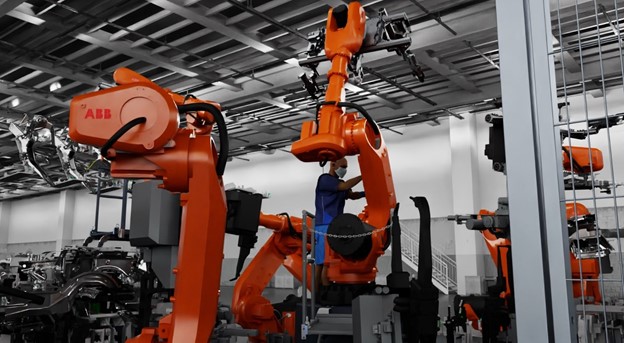
Digital twins are shaking up operations of businesses. The worldwide market for digital twin platforms is forecast to reach $86 billion by 2028, according to Grand View Research. Its report cites COVID-19 as a catalyst for the adoption of digital twins in specific industries.
What’s Driving Digital Twins?
The Internet of Things is revving up digital twins.
IoT is helping to enable connected machines and devices to share data with their digital twins and vice versa. That’s because digital twins are always on and up-to-date computer-simulated versions of real-world IoT-connected physical things or processes they represent.
Digital twins are virtual representations that can capture the physics of structures and changing conditions internally and externally, as measured by myriad connected sensors driven by edge computing. They can also run simulations within the virtualizations to test for problems and seek improvements through service updates.
Robotics development and autonomous vehicles are just a couple of the growing number of examples used in digital twins to mimic physical equipment and environments.
“Autonomous vehicles at a very simple level are robots that operate in the open world, striving to avoid contact with anything,” said Rev Lebaredian, vice president of Omniverse and Simulation Technology at NVIDIA. “Eventually we’ll have sophisticated autonomous robots working alongside humans in settings like kitchens — manipulating knives and other dangerous tools. We need digital twins of the worlds they are going to be operating in, so we can teach them safely in the virtual world before transferring their intelligence into the real world.”
Digital Twins in 3D Virtual Environments
Shared virtual 3D worlds are bringing people together to collaborate on digital twins.
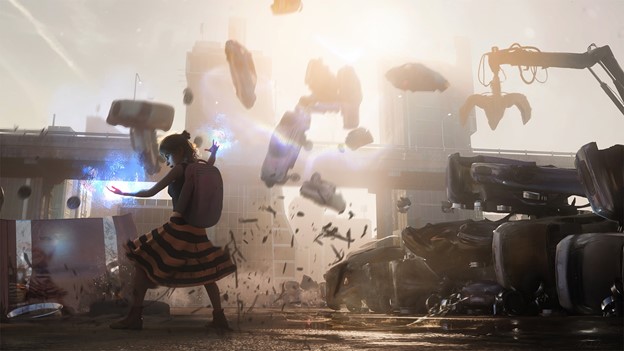
The interactive 3D virtual universe is evident in gaming. Online social games such as Fortnite and the user-generated virtual world of Roblox offer a glimpse of the potential of interactions.
Video conferencing calls in VR, with participants existing as avatars of themselves in a shared virtual conference room, are a step toward realizing the possibilities for the enterprise.
Today the tools exist to develop each of these shared virtual worlds in a shared virtual collaboration platform within this environment.
Omniverse Replicator for Digital Twin Simulations
At GTC, NVIDIA unveiled Omniverse Replicator to help develop digital twins. It’s a synthetic-data-generation engine that produces physically simulated data for training deep neural networks.
Along with this, the company introduced two implementations of the engine for applications that generate synthetic data: NVIDIA DRIVE Sim, a virtual world for hosting the digital twin of autonomous vehicles, and NVIDIA Isaac Sim, a virtual world for the digital twin of manipulation robots.
Autonomous vehicles and robots developed using this data can master skills across an array of virtual environments before applying them in the real world.
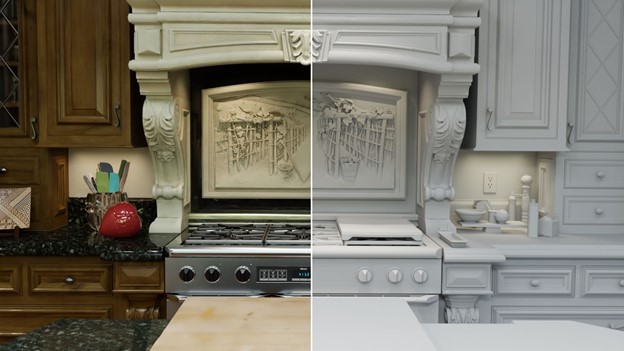
Based on Pixar’s Universal Scene Description and NVIDIA RTX technology, NVIDIA Omniverse is the world’s first scalable, multi-GPU physically accurate world simulation platform.
Omniverse offers users the ability to connect to multiple software ecosystems — including Epic Games Unreal Engine, Reallusion, OnShape, Blender and Adobe — that can assist millions of users.
The reference development platform is modular and can be extended easily. Teams across NVIDIA have enlisted the platform to build core simulation apps such as the previously mentioned NVIDIA Isaac Sim for robotics and synthetic data generation, and NVIDIA DRIVE Sim.
DRIVE Sim enables recreating real-world driving scenarios in a virtual environment to enable testing and development of rare and dangerous use cases. In addition, because the simulator has a perfect understanding of the ground-truth in any scene, the data from the simulator can be used for training the deep neural networks used in autonomous vehicle perception.
As shown in BMW Group’s factory of the future, Omniverse’s modularity and openness allows it to utilize several other NVIDIA platforms such as the NVIDIA Isaac platform for robotics, NVIDIA Metropolis for intelligent video analytics, and the NVIDIA Aerial software development kit, which brings GPU-accelerated, software-defined 5G wireless radio access networks to environments as well as third-party software for users and companies to continue to use their own tools.
How Are Digital Twins Coming Online?
When building a digital twin and deploying its features, corralling AI resources is necessary.
NVIDIA Base Command Platform enables enterprises to deploy large-scale AI infrastructure. It optimizes resources for users and teams, and it can monitor the workflow from early development to production.
Base Command was developed to help support NVIDIA’s in-house research team with AI resources. It helps manage the available GPU resources, select databases, workspaces and container images available.
It manages the lifecycle of AI development, including workload management and resource sharing, providing both a graphical user interface and a command line interface, and integrated monitoring and reporting dashboards. It delivers the latest NVIDIA updates directly into your AI workflows.
Think of it as the compute engine of AI.
How Are Digital Twins Managed?
NVIDIA Fleet Command provides remote AI management.
Implementing AI from digital twins into the real world requires a deployment platform to handle the updates to the thousands, or even millions, of machines and devices of the edge.
NVIDIA Fleet Command is a cloud-based service accessible from the NVIDIA NGC hub of GPU-accelerated software to securely deploy, manage and scale AI applications across edge-connected systems and devices.
Fleet Command enables fulfillment centers, manufacturing facilities, retailers and many others to remotely implement AI updates.
How Are Digital Twins Advancing?
Digital twins enable the autonomy of things. They can be used to control a physical counterpart autonomously.
An electric vehicle maker, for example, might use a digital twin of a sedan to run simulations on software updates. And when the simulations show improvements to the car’s performance or solve a problem, those software updates can be dispatched over the air to the physical vehicle.
Siemens Energy is creating digital twins to support predictive maintenance of power plants. A digital twin of this scale promises to reduce downtime and help save utility providers an estimated $1.7 billion a year, according to the company.
Passive Logic, a startup based in Salt Lake City, offers an AI platform to engineer and autonomously operate the IoT components of buildings. Its AI engine understands how building components work together, down to the physics, and can run simulations of building systems.
The platform can take in multiple data points and make control decisions to optimize operations autonomously. It compares this optimal control path to actual sensor data, applies machine learning and learns improvements about operating the building over time.
Trains are on a fast track to autonomy as well, and digital twins are being developed to help get there. They’re being used in simulations for features such as automated braking and collision detection systems, enabled by AI run on NVIDIA GPUs.
What Is the History of Digital Twins?
By many accounts, NASA was the first to introduce the notion of the digital twin. While clearly not connected in the Internet of Things way, NASA’s early twin concept and its usage share many similarities with today’s digital twins.
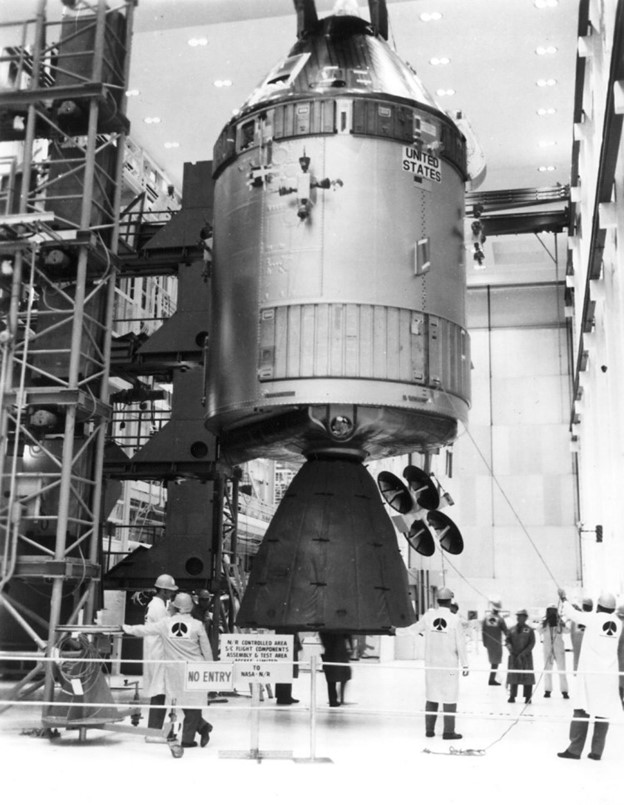
NASA began with the digital twin idea as early as the 1960s. The space agency illustrated its enormous potential in the Apollo 13 moon mission. NASA had set up simulators of systems on the Apollo 13 spacecraft, which could get updates from the real ship in outer space via telecommunications. This allowed NASA engineers to run situation simulations between astronauts and engineers ahead of departure, and it came in handy when things went awry on that mission in 1970.
Engineers on the ground were able to troubleshoot with the astronauts in space, referring to the models on Earth and saving the mission from disaster.
What Types of Digital Twins Are There?
Smart Cities Sims
Smart cities are popping up everywhere. Using video cameras, edge computing and AI, cities are able to understand everything from parking to traffic flow to crime patterns. Urban planners can study the data to help draw up and improve city designs.
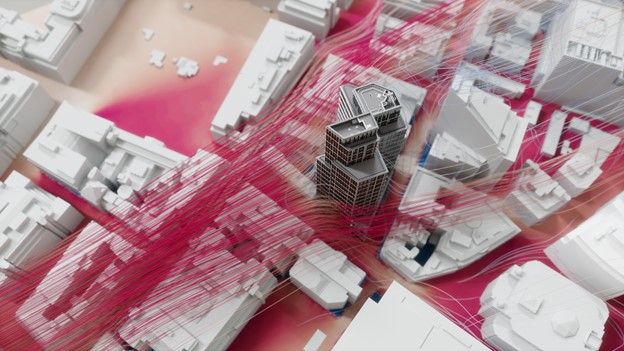
Digital twins of smart cities can enable better planning of construction as well as constant improvements in municipalities. Smart cities are building 3D replicas of themselves to run simulations. These digital twins help optimize traffic flow, parking, street lighting and many other aspects to make life better in cities, and these improvements can be implemented in the real world.
Dassault Systèmes has helped build digital twins around the world. In Hong Kong, the company presented examples for a walkability study, using a 3D simulation of the city for visualization.
NVIDIA Metropolis is an application framework, a set of developer tools and a large ecosystem of specialist partners that help developers and service providers better instrument physical space and build smarter infrastructure and spaces through AI-enabled vision. The platform spans AI training to inference, facilitating edge-to-cloud deployment, and it includes enterprise management tools like Fleet Command to better manage fleets of edge nodes.
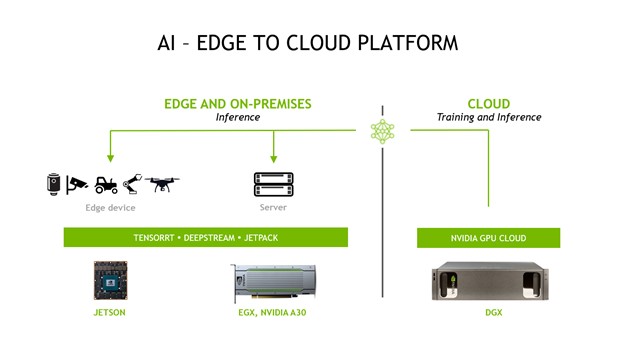
Earth Simulation Twins
Digital twins are even being applied to climate modeling.
NVIDIA CEO Jensen Huang disclosed plans to build the world’s most powerful AI supercomputer dedicated to predicting climate change.
Named Earth-2, or E-2, the system would create a digital twin of Earth in Omniverse.
Separately, the European Union has launched Destination Earth, an effort to build a digital simulation of the planet. The plan is to help scientists accurately map climate development as well as extreme weather.
Supporting an EU mandate for achieving climate neutrality by 2050, the digital twin effort would be rendered at one-kilometer scale and based on continuously updated observational data from climate, atmospheric and meteorological sensors. It also plans to take into account measurements of the environmental impacts of human activities.
It is predicted that the Destination Earth digital twin project would require a system with 20,000 GPUs to operate at full scale, according to a paper published in Nature Computational Science. Simulation insights can enable scientists to develop and test scenarios. This can help inform policy decisions and sustainable development planning.
Such work can help assess drought risk, monitor rising sea levels and track changes in the polar regions. It can also be used for planning on food and water issues, and renewable energy such as wind farms and solar plants. The goal is for the main digital modeling platform to be operating by 2023, with the digital twin live by 2027.
Data Center Networking Simulation
Networking is an area where digital twins are reducing downtime for data centers.
Over time, networks have become more complicated. The scale of networks, the number of nodes and the interoperability between components add to their complexity, affecting preproduction and staging operations.
Network digital twins speed up initial deployments by pretesting routing, security, automation and monitoring in simulation. They also enhance ongoing operations, including validating network change requests in simulation, which reduces maintenance times.
Networking operations have also evolved to more advanced capabilities with the use of APIs and automation. And streaming telemetry — think IoT-connected sensors for devices and machines — allows for constant collection of data and analytics on the network for visibility into problems and issues.
The NVIDIA Air infrastructure simulation platform enables network engineers to host digital twins of data center networks.
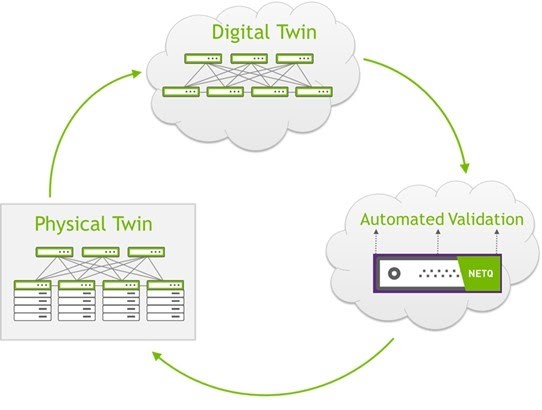
Ericsson, a maker of telecommunications equipment, is combining decades of radio network simulation expertise with NVIDIA Omniverse Enterprise.
The Stockholm-based company is building city-scale digital twins in NVIDIA Omniverse to help accurately simulate the interplay between 5G cells and the environment to maximize performance and coverage.
Automotive Manufacturing Twins
BMW Group, which has 31 factories around the world, is collaborating with NVIDIA on digital twins. The German automaker is relying on NVIDIA Omniverse Enterprise to run factory simulations to optimize its operations.
Its factories provide more than 100 options for each car, and more than 40 BMW models, offering 2,100 possible configurations of a new vehicle. Some 99 percent of the vehicles produced in BMW factories are custom configurations, which creates challenges for keeping materials stocked on the assembly line.
To help maintain the flow of materials for its factories, BMW Group is also harnessing the NVIDIA Isaac robotics platform to deploy a fleet of robots for logistics to improve the distribution of materials in its production environment. These human-assisting robots, which are put into simulation scenarios with digital humans in pre-production, enable the company to safely test out robot applications on the factory floor of the digital twin before launching into production.
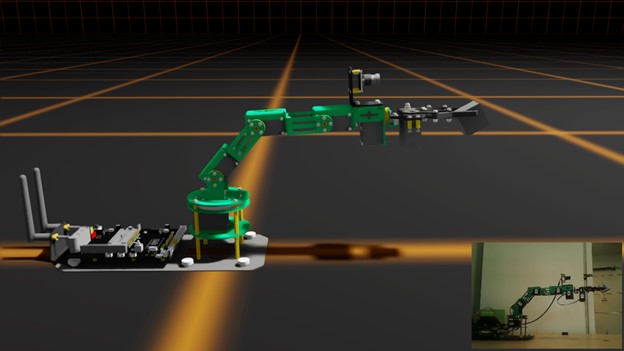
Virtual simulations also enable the company to optimize the assembly line as well as worker ergonomics and safety. Planning experts from different regions can connect virtually with NVIDIA Omniverse, which lets global 3D design teams work together simultaneously across multiple software suites in a shared virtual space.
NVIDIA Omniverse Enterprise is enabling digital twins for many different industrial applications.
Architecture, Engineering and Construction
Building design teams face a growing demand for efficient collaboration, faster iteration on renderings, and expectations for accurate simulation and photorealism.
These demands can become even more challenging when teams are dispersed worldwide.
Creating digital twins in Omniverse for architects, engineers and construction teams to assess designs together can quicken the pace of development, helping contracts run on time.
Teams on Omniverse can be brought together virtually in a single, interactive platform — even when simultaneously working in different software applications — to rapidly develop architectural models as if they are in the same room and simulate with full physical accuracy and fidelity.
Retail and Fulfillment
Logistics for order fulfillment is a massive industry of moving parts. Fulfillment centers now are aided by robots to help workers avoid injury and boost their efficiency. It’s an environment filled with cameras driven by AI and edge computing to help rapidly pick, pull and pack products. It’s how one-day deliveries arrive at our doors.
The use of digital twins means that much of this can be created in a virtual environment, and simulations can be run to eliminate bottlenecks and other problems.
Kinetic Vision is reinventing intelligent fulfillment and distribution centers with digital twins through digitization and AI. Successfully implementing a network of intelligent stores and fulfillment centers needs robust information, data, and operational technologies to enable innovative edge computing and AI solutions like real-time product recognition. This drives faster, more agile product inspections and order fulfillments.
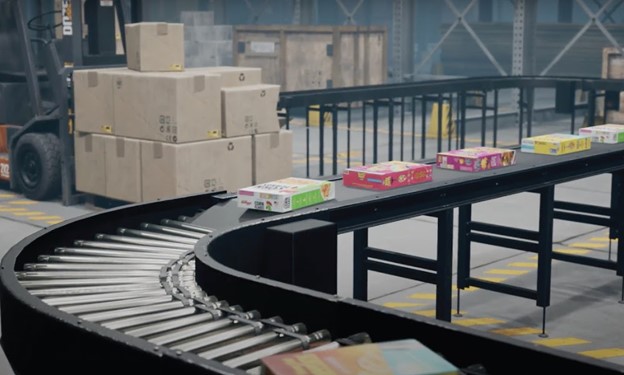
Energy Industry Twins
Siemens Energy is relying on the NVIDIA Omniverse platform to create digital twins to support predictive maintenance of power plants.
Using NVIDIA Modulus software frameworks, running on NVIDIA A100 Tensor Core GPUs, Siemens Energy can simulate the corrosive effects of heat, water and other conditions on metal over time to fine-tune maintenance needs.
Hydrocarbon Exploration
Oil companies face huge risks in seeking to tap new reservoirs or reassess production stage fields with the least financial and environmental downside. Drilling can cost hundreds of millions of dollars. After locating hydrocarbons, these energy companies need to quickly figure out the most profitable strategies for new or ongoing production
Digital twins for reservoir simulations can save many millions of dollars and avoid environmental problems. Using technical software applications, these companies can model how water and hydrocarbons flow under the ground amid wells. This allows them to evaluate potentially problematic situations and virtual production strategies on supercomputers.
Having assessed the risks beforehand, in the digital twins, these exploration companies can minimize losses when committing to new projects. Real-world versions in production can also be optimized for better output based on analytics from their digital doppelganger.
Airport Efficiencies
Digital twins can enable airports to improve customer experiences. For instance, video cameras could monitor the Transportation Security Administration, or TSA, and apply AI to look for ways to analyze bottlenecks at peak hours. Those could be addressed in digital models, and then moved into production to reduce missed flights. Baggage handling video can be assessed to improve ways in the digital environment to ensure luggage arrives on time.
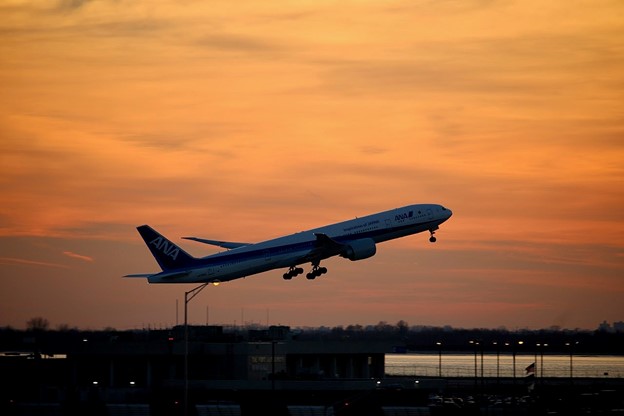
Airplane turnarounds can benefit, too. Many vendors service arriving planes to get them turned around and back on the runway for departures. Video can help airlines track these vendors to ensure timely turnarounds. Digital twins can also analyze the services coordination to optimize workflows before changing things up.
Airlines can then hold their vendors accountable to quickly carrying out services. Caterers, cleaners, refueling, trash and waste removal and other service providers all have what’s known as service-level agreements with airlines to help keep the planes running on time. All of these activities can be run in simulations in the digital world and then applied to scheduling in production for real-world results to help reduce departure delays.
NVIDIA Metropolis helps to process massive amounts of video from the edge so that airports and other industries can analyze operations in real time and derive insights from analytics.
What’s the Future for Digital Twins?
Digital twin simulations have been simmering for half a century. But the past decade’s advances in GPUs, AI and software platforms are heating up their adoption amid this higher-fidelity era of more immersive experiences.
Increasing penetration of virtual reality and augmented reality will accelerate this work.
Worldwide sales of VR headsets are expected to increase from roughly 7 million in 2021 to more than 28 million in 2025, according to analyst firm IDC.
That’s a lot more headset-connected, content-consuming eyeballs for virtual environments.
And all those in it will be able to access the NVIDIA Omniverse platform for AI, human and robot interactions, and infinite simulations, driving a wild ride of advances from digital twins.
“There has been talk of virtual worlds and digital twins for years. We’re right at the beginning of this transition into reality, much as AI became viable and created an explosion of possibilities,” said NVIDIA’s Lebaredian.
Buckle up for the adventure.