As more organizations move to machine learning (ML) to drive deeper insights, two key stumbling blocks they run into are labeling and lifecycle management. Labeling is the identification of data and adding labels to provide context so an ML model can learn from it. Labels might indicate a phrase in an audio file, a car in a photograph, or an organ in an MRI. Data labeling is necessary to enable ML models to work against the data. Lifecycle management has to do with the process of setting up an ML experiment and documenting the dataset, library, version, and model used to get results. A team might run hundreds of experiments before settling on one approach. Going back and recreating that approach can be difficult without records of the elements of that experiment.
Many ML examples and tutorials start with a dataset that includes a target value. However, real-world data doesn’t always have such a target value. For example, in sentiment analysis, a person can usually make a judgment on whether a review is positive, negative, or mixed. But reviews are made up of a collection of text with no judgment value attached to it. In order to create a supervised learning model to solve this problem, a high-quality labeled dataset is essential. Amazon SageMaker Ground Truth is a fully managed data labeling service that makes it easy to build highly accurate training datasets for ML.
For organizations that use Databricks as their data and analytics platform on AWS to perform extract, transform, and load (ETL) tasks, the ultimate goal is often training a supervised learning model. In this post, we show how Databricks integrates with Ground Truth and Amazon SageMaker for data labeling and model distribution.
Solution overview
Ground Truth is a fully managed data labeling service that makes it easy to build highly accurate training datasets for ML. Through the Ground Truth console, we can create custom or built-in data labeling workflows in minutes. These workflows support a variety of use cases, including 3D point clouds, video, images, and text. In addition, Ground Truth offers automatic data labeling, which uses an ML model to label our data.
We train our model on the publicly available Amazon Customer Reviews dataset. At a high level, the steps are as follows:
- Extract a raw dataset to be labeled and move it to Amazon Simple Storage Service (Amazon S3).
- Perform labeling by creating a labeling job in SageMaker.
- Build and train a simple Scikit-learn linear learner model to classify the sentiment of the review text on the Databricks platform using a sample notebook.
- Use MLflow components to create and perform MLOps and save the model artifacts.
- Deploy the model as a SageMaker endpoint using the MLflow SageMaker library for real-time inference.
The following diagram illustrates the labeling and ML journey using Ground Truth and MLflow.
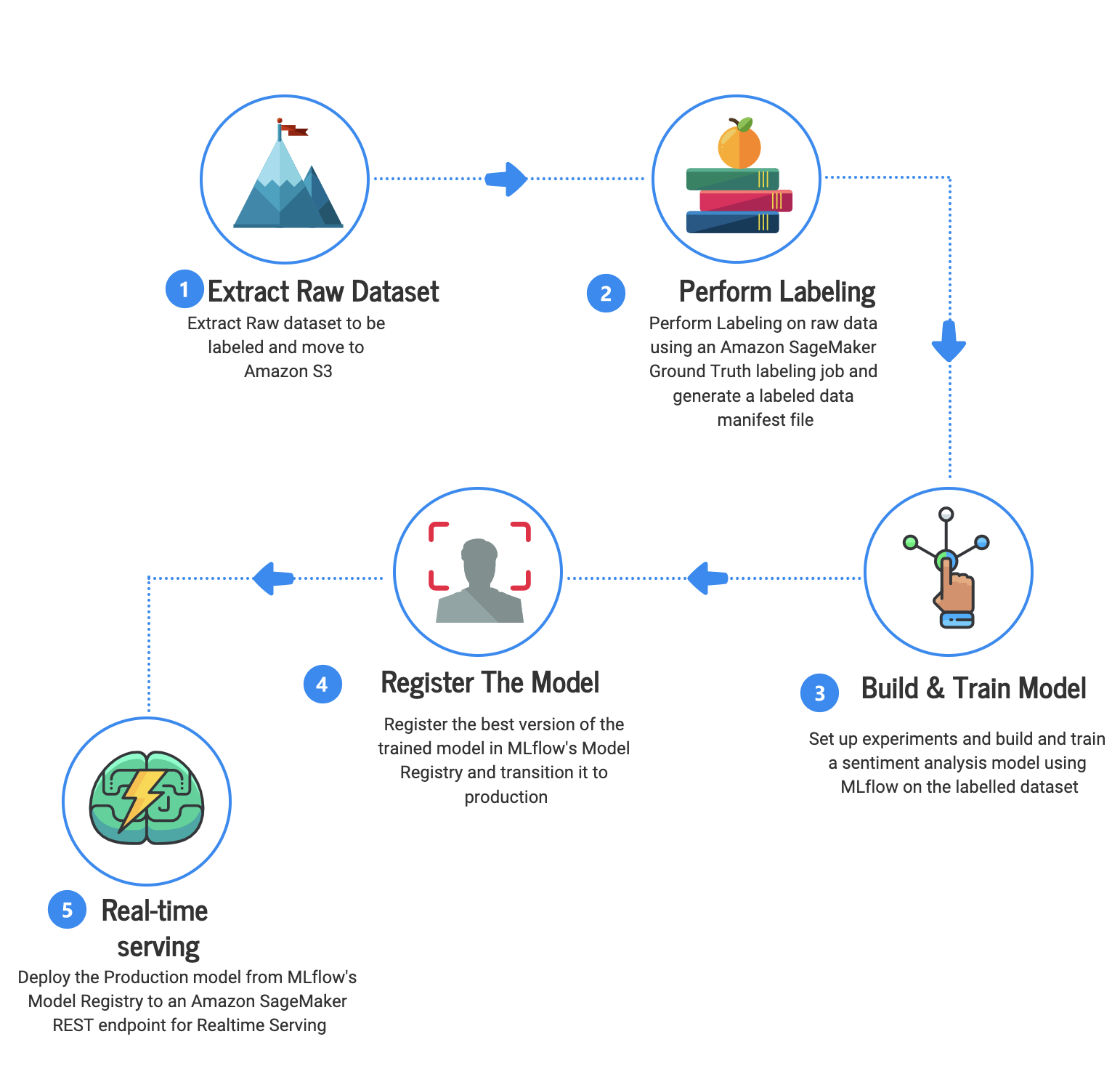
Create a labeling job in SageMaker
From the Amazon Customer Reviews dataset, we extract the text portions only, because we’re building a sentiment analysis model. Once extracted, we put the text in an S3 bucket and then create a Ground Truth labeling job via the SageMaker console.
On the Create labeling job page, fill out all required fields. As a part of step on this page, Ground Truth allows you to generate the job manifest file. Ground Truth uses the input manifest file to identify the number of files or objects in the labeling job so that the right number of tasks are created and sent to human (or machine) labelers. The file is automatically saved in the S3 bucket. The next step is to specify the task category and task selection. In this use case, we choose Text as the task category, and Text Classification with a single label for task selection, which means a review text will have a single sentiment: positive, negative, or neutral.
Finally, we write simple but concise instructions for labelers on how to label the text data. The instructions are displayed on the labeling tool and you can optionally review the annotator’s view at this time. Finally, we submit the job and monitor the progress on the console.
While the labeling job is in progress, we can also look at the labeled data on the Output tab. We can monitor each review text and label, and if the job was done by a human or machine. We can select 100% of the labeling jobs to be done by humans or choose machine annotation, which speeds up the job and reduces labor costs.
When the job is complete, the labeling job summary contains links to the output manifest and the labeled dataset. We can also go to Amazon S3 and download both from our S3 bucket folder.
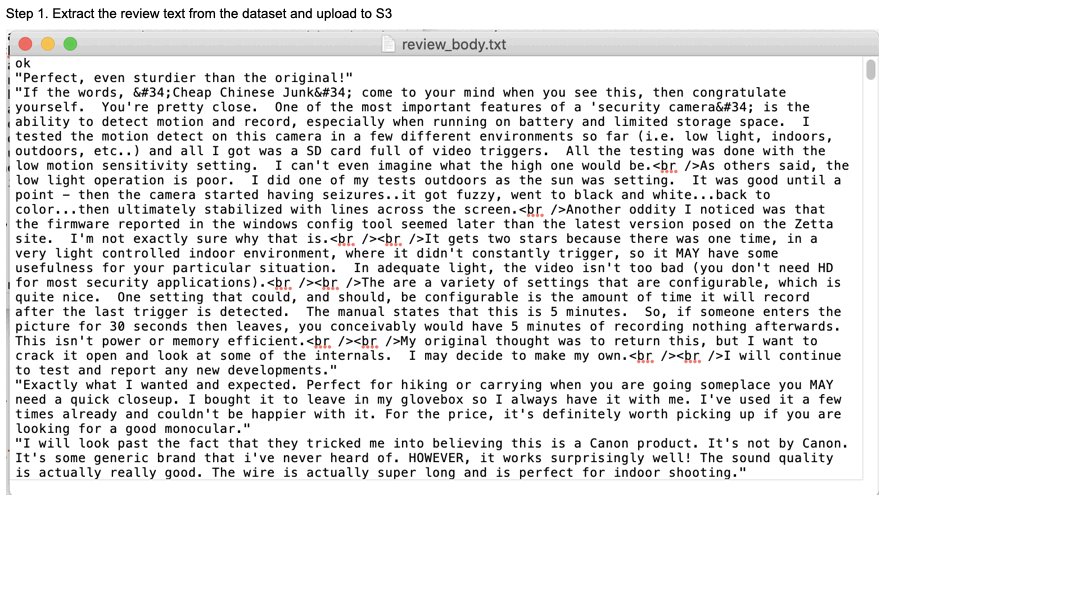
In the next steps, we use a Databricks notebook, MLflow, and datasets labeled by Ground Truth to build a Scikit-learn model.
Download a labeled dataset from Amazon S3
We start by downloading the labeled dataset from Amazon S3. The manifest is saved in JSON format and we load it into a Spark DataFrame in Databricks. For training the sentiment analysis model, we only need the review text and sentiment that was annotated by the Ground Truth labeling job. We use select() to extract those two features. Then we convert the dataset from a PySpark DataFrame to a Pandas DataFrame, because the Scikit-learn algorithm requires Pandas DataFrame format.
Next, we use Scikit-learn CountVectorizer
to transform the review text into a bigram vector by setting the ngram_range
max value to 2. CountVectorizer
converts text into a matrix of token counts. Then we use TfidfTransformer
to transform the bigram vector into a term frequency-inverse document frequency (TF-IDF) format.
We compare the accuracy scores for training done with a bigram vector vs. bigram with TF-IDF. TF-IDF is a statistical measure that evaluates how relevant a word is to a document in a collection of documents. Because the review text tends to be relatively short, we can observe how TF-IDF affects the performance of the predictive model.
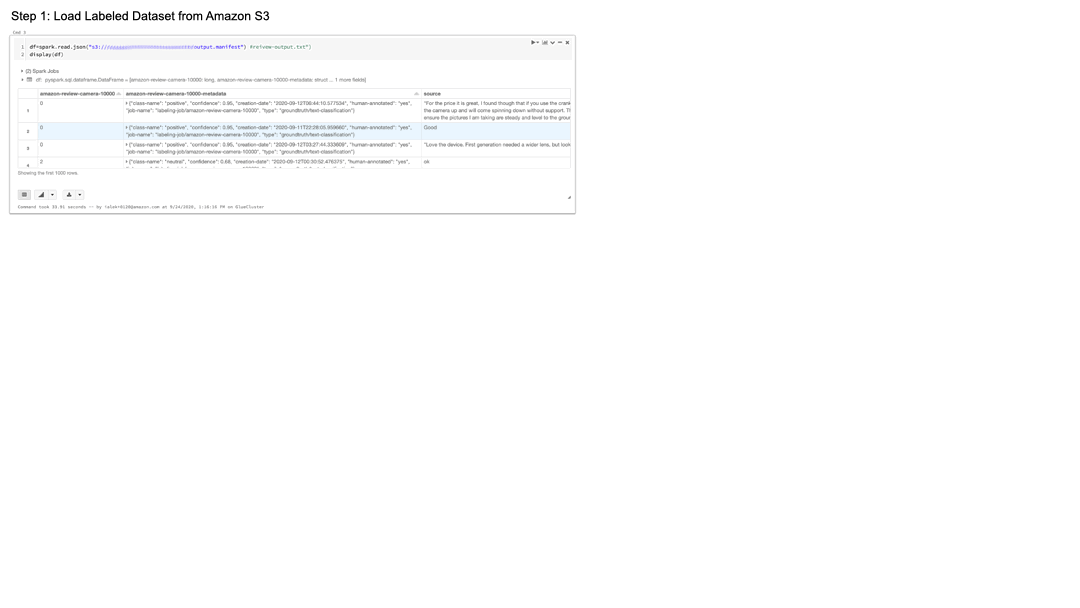
Set up an MLflow experiment
MLflow was developed by Databricks and is now an open-source project. MLflow manages the ML lifecycle, so you can track, recreate, and publish experiments easily.
To set up MLflow experiments, we use mlflow.sklearn.autolog()
to enable auto logging of hyperparameters, metrics, and model artifacts whenever estimator.fit()
, estimator.fit_predict()
, and estimator.fit_transform()
are called. Alternatively, you can do this manually by calling mlflow.log_param()
and mlflow.log_metric()
.
We fit the transformed dataset to a linear classifier with Stochastic Gradient Descent (SGD) learning. With SGD, the gradient of the loss is estimated one sample at a time and the model is updated along the way with a decreasing strength schedule.
Those two datasets we prepared earlier are passed to the train_and_show_scores()
function for training. After training, we need to register a model and save its artifacts. We use mlflow.sklearn.log_model()
to do so.
Before deploying, we look at the experiment’s results and choose two experiments (one for bigram and the other for bigram with TF-IDF) to compare. In our use case, the second model trained with bigram TF-IDF performed slightly better, so we pick that model to deploy. After the model is registered, we deploy the model, changing the model stage to production. We can accomplish this on the MLflow UI, or in the code using transition_model_version_stage()
.
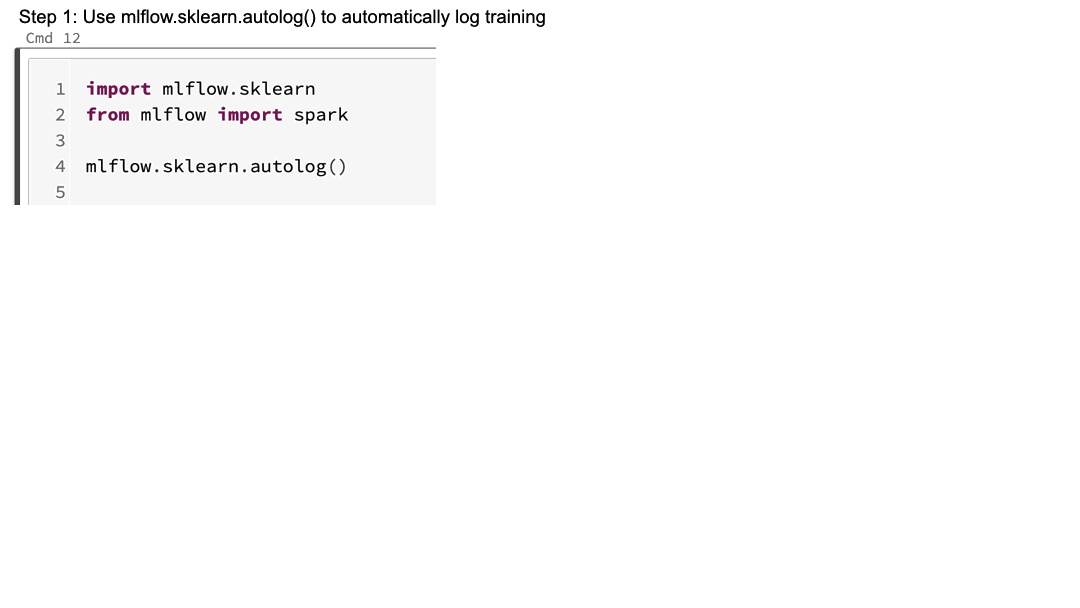
Deploy and test the model as a SageMaker endpoint
Before we deploy the trained model, we need to build a Docker container to host the model in SageMaker. We do this by running a simple MLflow command that builds and pushes the container to Amazon Elastic Container Registry (Amazon ECR) in our AWS account.
We can now find the image URI on the Amazon ECR console. We pass the image URI as an image_url
parameter, and use DEPLOYMENT_MODE_CREATE
for the mode parameter if this is a new deployment. If updating an existing endpoint with a new version, use DEPLOYMENT_MODE_REPLACE
.
To test the SageMaker endpoint, we create a function that takes the endpoint name and input data as its parameters.
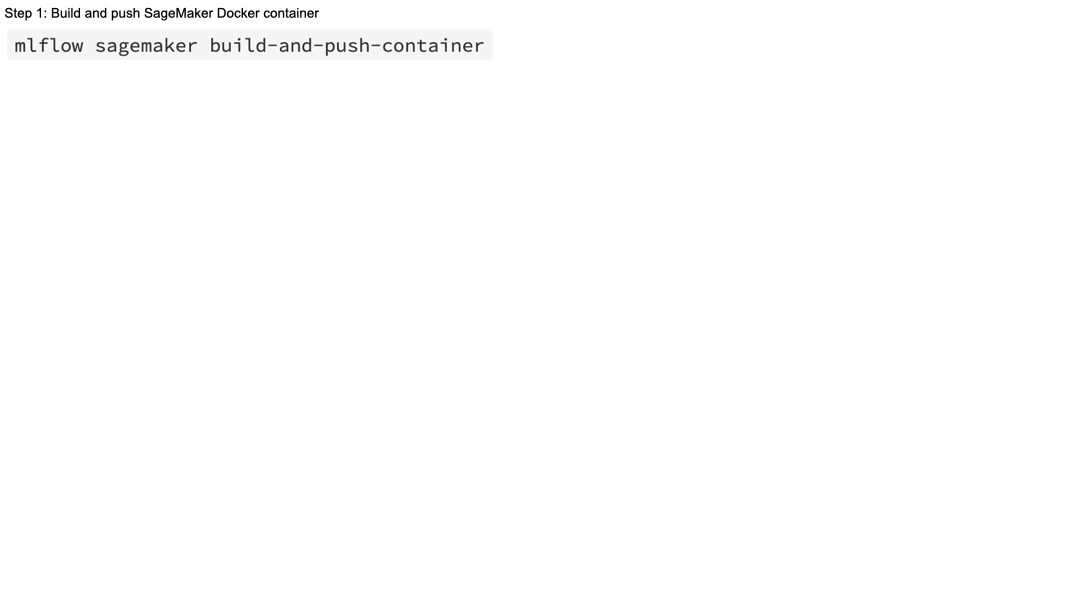
Conclusion
In this post, we showed you how to use Ground Truth to label a raw dataset, and the use the labeled data to train a simple linear classifier using Scikit-learn. In this example, we use MLflow to track hyperparameters and metrics, register a production-grade model, and deploy the trained model to SageMaker as an endpoint. Along with Databricks to process the data, you can automate this whole use case, so as new data is introduced, it can be labeled and processed into the model. By automating these pipelines and models, data science teams can focus on new use cases and uncover more insights instead of spending their time managing data updates on a day-to-day basis.
To get started, check out Use Amazon SageMaker Ground Truth to Label Data and sign up for a 14-day free trial of Databricks on AWS. To learn more about how Databricks integrates with SageMaker, as well as other AWS services like AWS Glue and Amazon Redshift, visit Databricks on AWS.
Additionally, check out the following resources used in this post:
Use the following notebook to get started.
About the Authors
Rumi Olsen is a Solutions Architect in the AWS Partner Program. She specializes in serverless and machine learning solutions in her current role, and has a background in natural language processing technologies. She spends most of her spare time with her daughter exploring the nature of Pacific Northwest.
Igor Alekseev is a Partner Solution Architect at AWS in Data and Analytics. Igor works with strategic partners helping them build complex, AWS-optimized architectures. Prior joining AWS, as a Data/Solution Architect, he implemented many projects in Big Data, including several data lakes in the Hadoop ecosystem. As a Data Engineer, he was involved in applying AI/ML to fraud detection and office automation. Igor’s projects were in a variety of industries including communications, finance, public safety, manufacturing, and healthcare. Earlier, Igor worked as full stack engineer/tech lead.
Naseer Ahmed is a Sr. Partner Solutions Architect at Databricks supporting its AWS business. Naseer specializes in Data Warehousing, Business Intelligence, App development, Container, Serverless, Machine Learning Architectures on AWS. He was voted 2021 SME of the year at Databricks and is an avid crypto enthusiast.
Read More