AWS CodeArtifact allows developers to connect internal code repositories to upstream code repositories like Pypi, Maven, or NPM. AWS CodeArtifact is a powerful addition to CI/CD workflows on AWS, but it is similarly effective for code-bases hosted on a Jupyter notebook. This is a common development paradigm for Machine Learning developers that build and train ML models regularly.
In this post, we demonstrate how to securely connect to AWS CodeArtifact from an Internet-disabled SageMaker Notebook Instance. This post is for network and security architects that support decentralized data science teams on AWS.
In another post, we discussed how to create an Internet-disabled notebook in a private subnet of an Amazon VPC while maintaining connectivity to AWS services via AWS Private Link endpoints. The examples in this post will connect an Internet-disabled notebook instance to AWS CodeArtifact and download open-source code packages without needing to traverse the public internet.
Solution overview
The following diagram describes the solution we will implement. We create a SageMaker notebook instance in a private subnet of a VPC. We also create an AWS CodeArtifact domain and a repository. Access to the repository will be controlled by CodeArtifact repository policies and PrivateLink access policies.
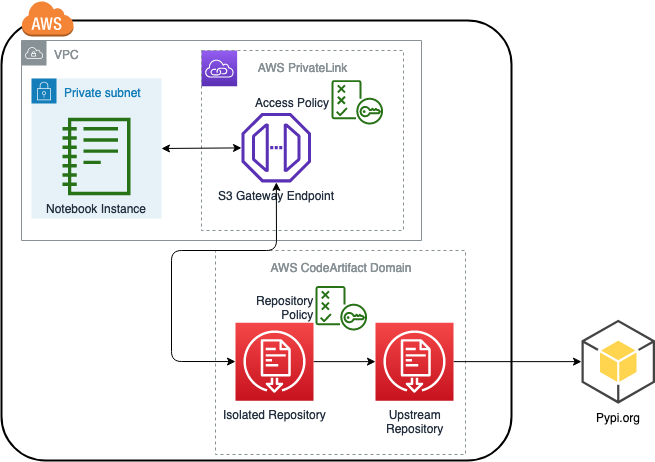
The architecture allows our Internet-disabled SageMaker notebook instance to access CodeArtifact repositories without traversing the public internet. Because the network traffic doesn’t traverse the public internet, we improve the security posture of the notebook instance by ensuring that only users with the expected network access can access the notebook instance. Furthermore, this paradigm allows security administrators to restrict library consumption to only “approved” distributions of code packages. By combining network security with secure package management, security engineers can transparently manage open-source libraries for data scientists without impeding their ability to work.
Prerequisites
For this post, we need an Internet-disabled SageMaker notebook instance, and a VPC with a private subnet. Visit this link to create a private subnet in a VPC, as well as the previous post in this series to get started with these prerequisites.
We also need a CodeArtifact domain in the AWS Region where you created your Internet-disabled SageMaker notebook instance. The domain is a way to organize repositories logically in the CodeArtifact service. Name the domain, select AWS managed key for encryption, and create the domain. This link discusses how to create an AWS CodeArtifact Domain.
Configure AWS CodeArtifact
To configure AWS CodeArtifact, we create a repository in the domain. Before proceeding, be sure to select the same region where the notebook instance has been deployed. Perform the following steps to configure AWS CodeArtifact:
- On the AWS CodeArtifact console, choose Create repository.
- Give the repository a name and description. Select the public upstream repository you want to use. We use the pypi-store in this post.
- Choose Next.
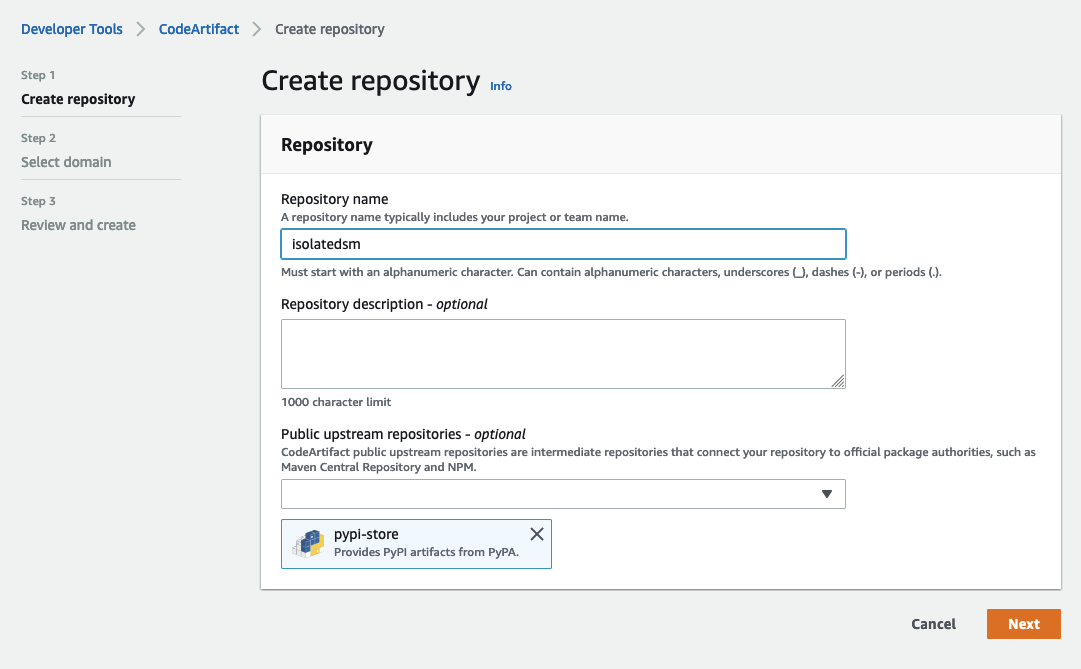
- Choose the AWS account you are working in and the AWS CodeArtifact domain you use for this account.
- Choose Next.
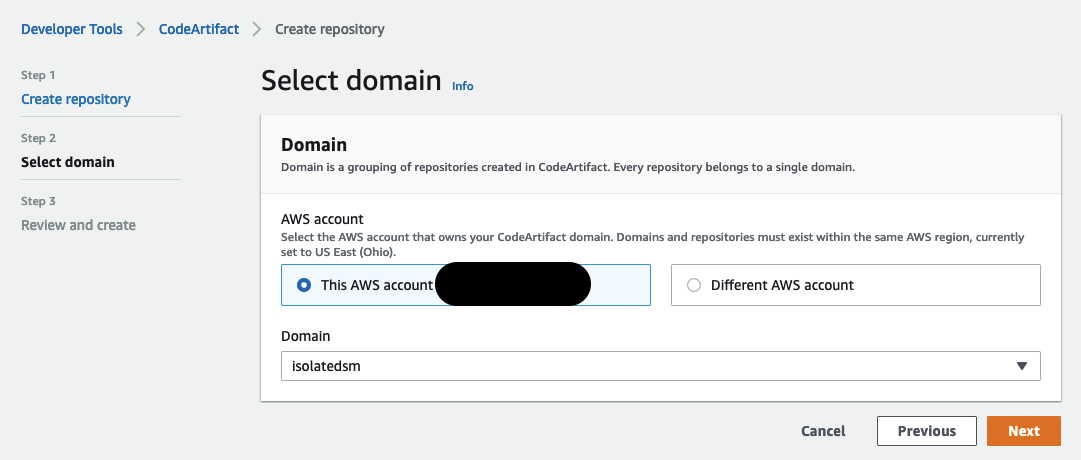
- Review the repository information summary and choose Create repository.
- Note: In the review screen section marked “Package flow” there is a flowchart describing the flow of dependencies from external connections into the domain managed by AWS CodeArtifact.
- This flowchart describes what happens when we create our repository. We are actually creating two repositories. The first is the “pypi-store” repository that connects to the externally hosted pypi repository. This repository was created by AWS CodeArtifact and is used to stage connections to the upstream repository. The second repository, “isolatedsm”, connects to the “pypi-store” repository. This transitive repository lets us combine external connections and stage third-party libraries before using them.
- This form of transitive repository management allows us to enforce least privileged access on the libraries we use for data science workloads.
Alternatively, we can perform these steps with the AWS CLI using the following command:
aws codeartifact create-repository --domain <domain_name> --domain-owner <account_number> --repository <repo_name> --description <repo_description> --region <region_name>
The result at the end of this process is two repositories in our domain.
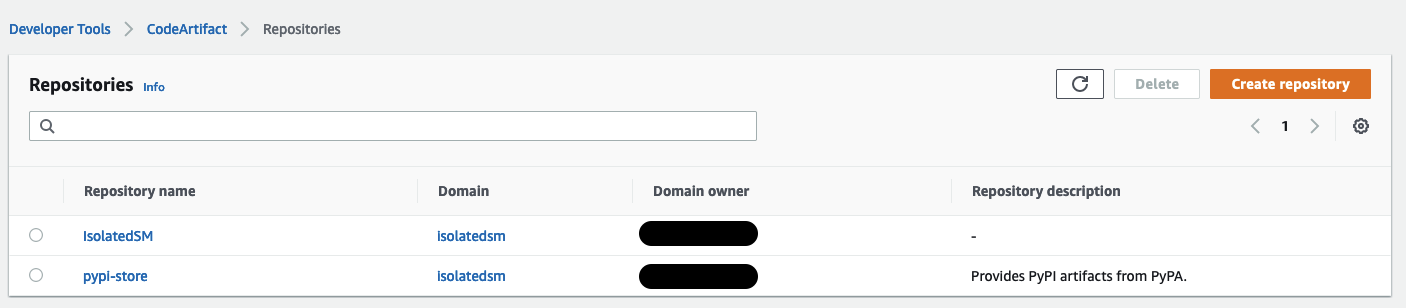
CodeArtifact Repository Policy
For brevity, we will grant a relatively open policy for our IsolatedSM repository allowing any of our developers to access the CodeArtifact repository. This policy should be modified for production use cases. Later in the post, we will discuss how to implement least-privilege access at the role level using an IAM policy attached to the notebook instance role. For now, navigate to the repository in the AWS Management Console and expand the Details section of the repository configuration page. Choose Apply a repository policy under Repository policy.
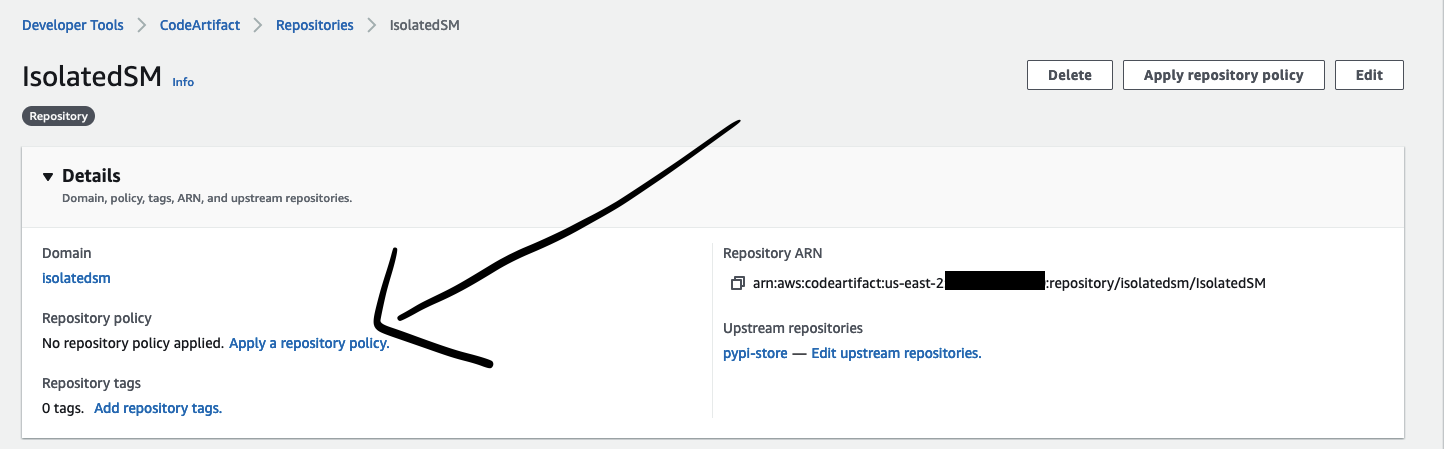
On the next screen, paste the following policy document in the text field marked Edit repository policy and then choose Save:
{
"Version": "2012-10-17",
"Statement": [
{
"Action": [
"codeartifact:AssociateExternalConnection",
"codeartifact:CopyPackageVersions",
"codeartifact:DeletePackageVersions",
"codeartifact:DeleteRepository",
"codeartifact:DeleteRepositoryPermissionsPolicy",
"codeartifact:DescribePackageVersion",
"codeartifact:DescribeRepository",
"codeartifact:DisassociateExternalConnection",
"codeartifact:DisposePackageVersions",
"codeartifact:GetPackageVersionReadme",
"codeartifact:GetRepositoryEndpoint",
"codeartifact:ListPackageVersionAssets",
"codeartifact:ListPackageVersionDependencies",
"codeartifact:ListPackageVersions",
"codeartifact:ListPackages",
"codeartifact:PublishPackageVersion",
"codeartifact:PutPackageMetadata",
"codeartifact:PutRepositoryPermissionsPolicy",
"codeartifact:ReadFromRepository",
"codeartifact:UpdatePackageVersionsStatus",
"codeartifact:UpdateRepository"
],
"Effect": "Allow",
"Resource": "*",
"Principal": {
"AWS": "arn:aws:iam::329542461890:role/GeneralIsolatedNotebook"
}
}
]
}
This policy provides full access to the repository for the role attached to the isolated notebook instance. This policy is a sample policy enables developer access to CodeArtifact. For more information on policy definitions for CodeArtifact repositories (especially if you need more restrictive role-based access), see the CodeArtifact User Guide. To configure the same repository policy using the AWS CLI, save the preceding policy document as policy.json
and run the following command:
aws codeartifact put-repository-permissions-policy --domain <domain_name> --domain-owner <account_number> --repository <repo_name> --policy-document file:///PATH/TO/policy.json --region <region_name>
Configure access to AWS CodeArtifact
Connecting to a CodeArtifact repository requires logging into the repository as a user. By navigating into a repository and choosing View connection instructions, we can select the appropriate connection instructions for our package manager of choice. We will use pip in this post; from the drop-down, select pip and copy the connection instructions.
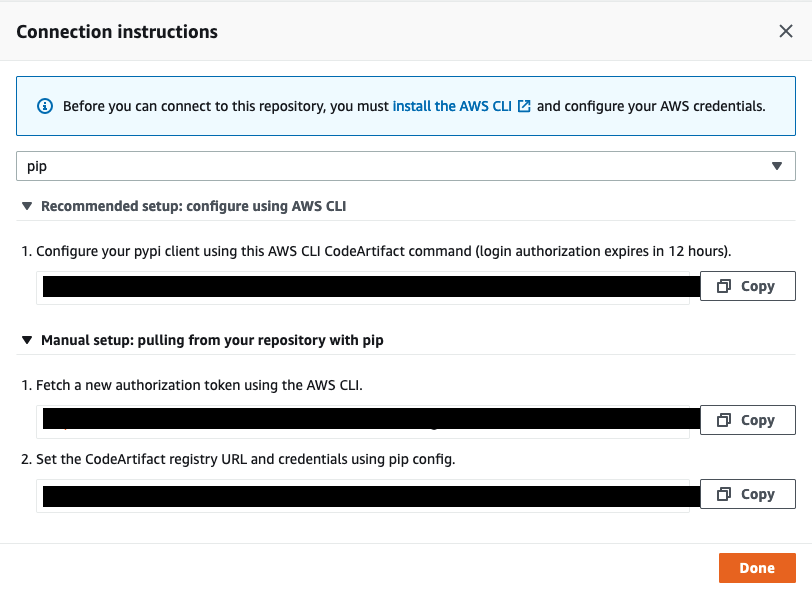
The AWS CLI command to use should be similar to the following:
aws codeartifact login --tool pip --repository <repo_name> --domain <domain_name> --domain-owner <account_number> --region <region_name>
This command is a CodeArtifact API call that returns an authentication cookie for the role that requested access to this repository. This command can be run in a Jupyter notebook to authenticate access to the CodeArtifact repository and will configure package managers to install libraries from that upstream repository. We can test this in our Internet-disabled SageMaker notebook instance.
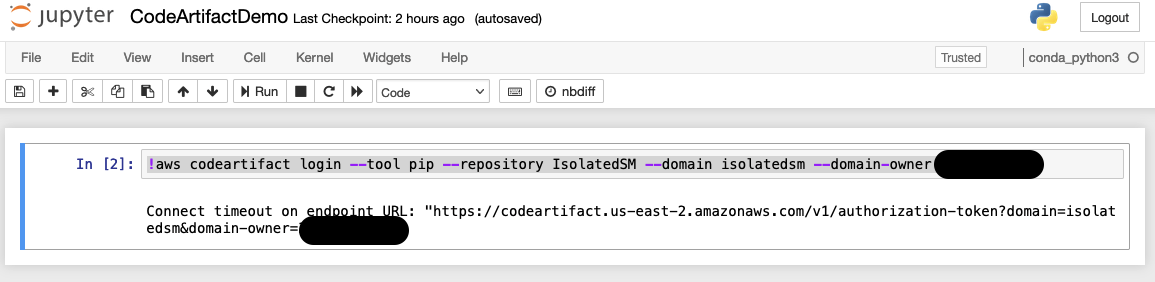
When running the login command in our isolated notebook instance, nothing happens for some time. After a while (approximately 300 seconds), Jupyter will output a connection timeout error. This is because our notebook instance lives in an isolated network subnet. This is expected behavior, it confirms our notebook instance is Internet-disabled. We need to provision network access between this subnet and our CodeArtifact repository.
Create A PrivateLink connection between the Notebook and CodeArtifact
AWS PrivateLink is a networking service that creates VPC endpoints in your VPC for other AWS services like Amazon Elastic Compute Cloud (Amazon EC2), Amazon S3, and Amazon Simple Notification Service (Amazon SNS). Private endpoints facilitate API requests to other AWS services through your VPC instead of through the public internet. This is the crucial component that lets our solution privately and securely access the CodeArtifact repository we’ve created.
Before we create our PrivateLink endpoints, we must create a security group to associate with the endpoints. Before proceeding, make sure you are in the same region as the Internet-disabled SageMaker notebook instance.
- On the Amazon VPC console, choose Security Groups.
- Choose Create security group.
- Give the group an appropriate name and description.
- Select the VPC that you will deploy the PrivateLink endpoints to. This should be the same VPC that hosts the isolated SageMaker notebook.
- Under Inbound Rules, choose Add Rule and then permit All Traffic from the security group hosting the isolated SageMaker notebook.
-
Outbound Rules should remain the default. Create the security group.
You can replicate these steps in the CLI with the following:
> aws ec2 create-security-group --group-name endpoint-sec-group --description "group for endpoint security" --vpc-id vpc_id --region region
> {
"GroupId": endpoint-sec-group-id
}
> aws ec2 authorize-security-group-ingress --group-id endpoint-sec-group-id --protocol all --port -1 --source-group isolated-SM-sec-group-id --region region

For this next step, we recommend that customers use the AWS CLI for simplicity. First, save the following policy document as policy.json
in your local file system.
{
"Statement": [
{
"Action": "codeartifact:*",
"Effect": "Allow",
"Resource": "*",
"Principal": "*"
},
{
"Effect": "Allow",
"Action": "sts:GetServiceBearerToken",
"Resource": "*",
"Principal": "*"
},
{
"Action": [
"codeartifact:CreateDomain",
"codeartifact:CreateRepository",
"codeartifact:DeleteDomain",
"codeartifact:DeleteDomainPermissionsPolicy",
"codeartifact:DeletePackageVersions",
"codeartifact:DeleteRepository",
"codeartifact:DeleteRepositoryPermissionsPolicy",
"codeartifact:PutDomainPermissionsPolicy",
"codeartifact:PutRepositoryPermissionsPolicy",
"codeartifact:UpdateRepository"
],
"Effect": "Deny",
"Resource": "*",
"Principal": "*"
}
]
}
Then, run the following commands using the AWS CLI to create the PrivateLink endpoints for CodeArtifact. This first command creates the VPC Endpoint for CodeArtifact repository API commands.
> aws ec2 create-vpc-endpoint
--vpc-endpoint-type Interface
--vpc-id vpc-id
--service-name com.amazonaws.region.codeartifact.repositories
--subnet-ids [list-of-subnet-ids]
-–security-group-ids endpoint-sec-group-id
–-private-dns-enabled
--policy-document file:///PATH/TO/policy.json
--region region
This second commands creates the VPC Endpoint for CodeArtifact non-repository API commands. Note in this command, we do not enable private DNS for the endpoint. Note the output of this command as we will use it to enable private DNS in a subsequent CLI command.
> aws ec2 create-vpc-endpoint
--vpc-endpoint-type Interface
--vpc-id vpc-id
--service-name com.amazonaws.region.codeartifact.api
--subnet-ids [list-of-subnet-ids]
-–security-group-ids endpoint-sec-group-id
–-no-private-dns-enabled
--policy-document file:///PATH/TO/policy.json
--region region
{
"VpcEndpoint": {
"VpcEndpointId": "vpc-endpoint-id",
...
}
}
Once this VPC endpoint has been created, enable private DNS for the endpoint by running the following, final command:
> aws ec2 modify-vpc-endpoint
--vpc-endpoint-id vpc-endpoint-id
--private-dns-enabled
--region region
This policy document permits common CodeArtifact operations performed by developers to be permitted over this PrivateLink endpoint. This is acceptable for our use case because CodeArtifact lets us define access policies on the repositories themselves. We are only blocking CodeArtifact administrative commands from being sent over this endpoint. We block administrative commands because we do not want developers to perform administrative commands on the repository.
The following screenshot shows the API endpoint and the security group it belongs to.
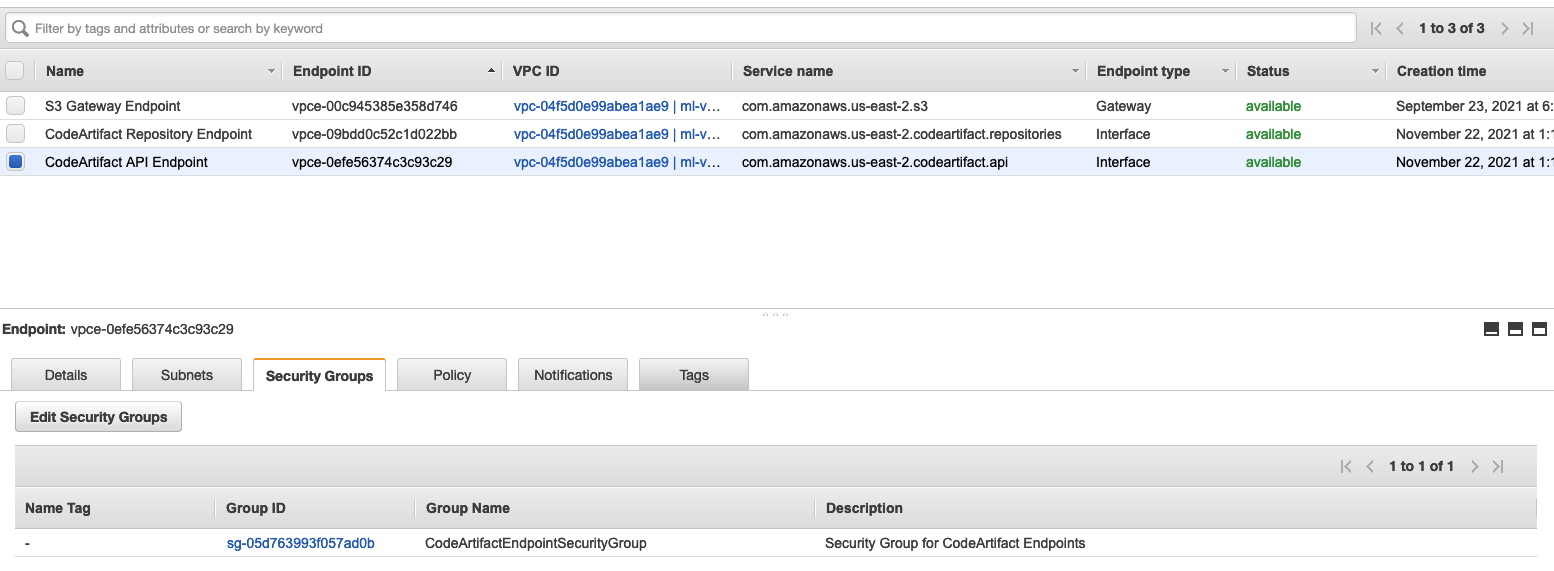
The inbound rules on this security group should list one inbound rule, allowing All traffic from the isolated SageMaker notebook’s security group.
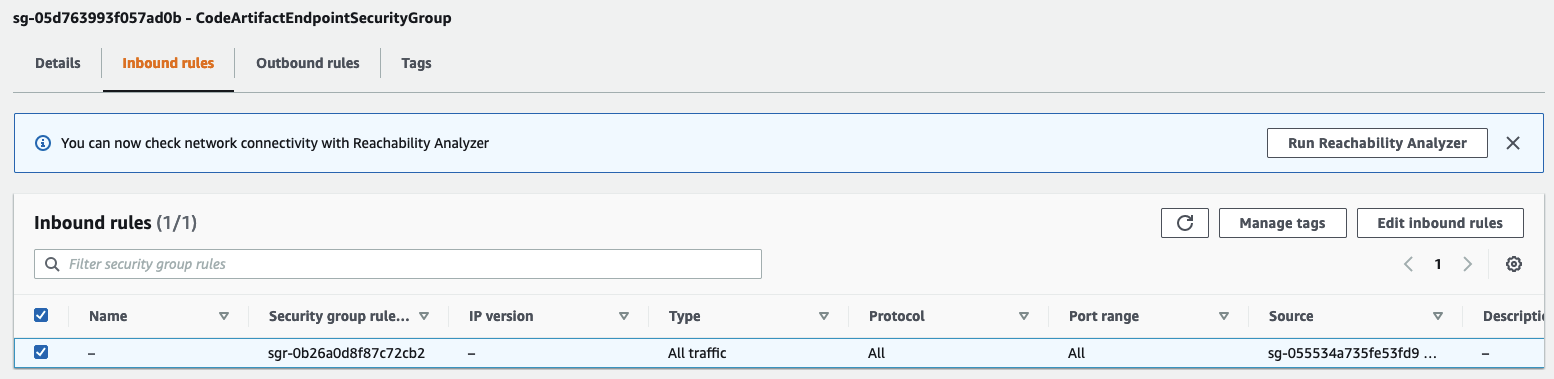
Network test
Once the security groups have been configured and the PrivateLink endpoints have been created, open a Jupyter notebook on the isolated SageMaker notebook instance. In a cell, run the connection instructions for the CodeArtifact repository we created earlier. Instead of a long pause with an eventual timeout error, we now get an AccessDenied exception.

Recall that CodeArtifact connection instructions can be found in the AWS Management Console by navigating to the CodeArtifact repository and selecting View connection instructions. For this post, select the connection instructions for pip.
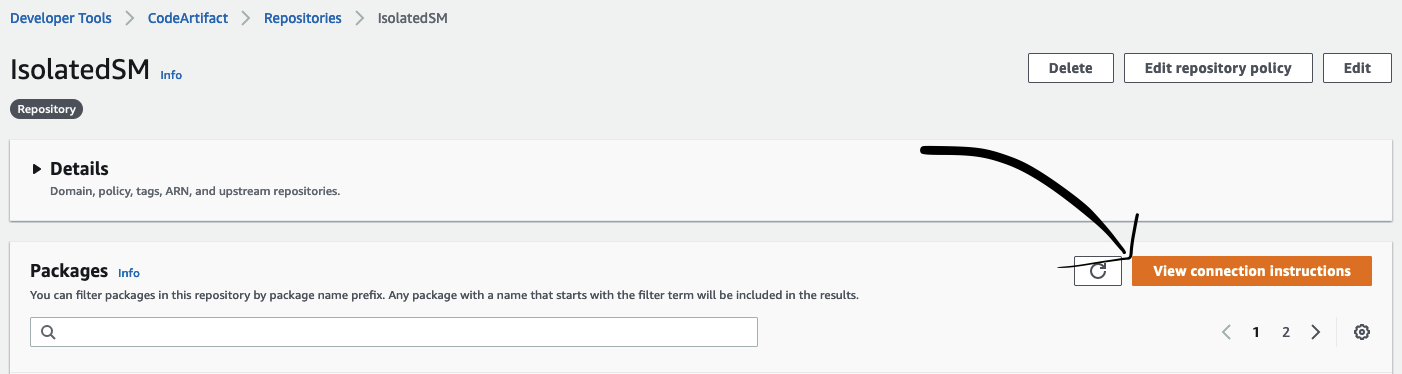
At this point, our isolated SageMaker notebook instance can connect to the CodeArtifact service via PrivateLink. We now need to give our notebook instance’s role the relevant permissions required to interact with the service from a Jupyter notebook.
Modify notebook permissions
Once our CodeArtifact repository is configured, we need to modify the permissions on our isolated notebook instance role to allow our notebook to read from the artifact repository. In the AWS Management Console, navigate to the IAM service and, under Policies, choose Create Policy. Choose the JSON tab and paste the following JSON document in the text window:
{
"Version": "2012-10-17",
"Statement": [
{
"Effect": "Allow",
"Action": "codeartifact:GetAuthorizationToken",
"Resource": "arn:aws:codeartifact:<region>:<account_no>:domain/<domain_name>"
},
{
"Effect": "Allow",
"Action": "sts:GetServiceBearerToken",
"Resource": "*"
}
]
}
To create this policy document in the CLI, save this JSON as policy.json
and run the following command:
aws iam create-policy --policy-name <policy_name> --policy-document file:///PATH/TO/policy.json
When attached to a role, this policy document permits the retrieval of an authorization token from the CodeArtifact service. Attach this policy to our notebook instance role by navigating to the IAM service in the AWS Management Console. Choose the notebook instance role you are using and attach this policy directly to the instance role. This can be done with the AWS CLI by running the following command:
aws iam attach-role-policy --role-name <role_name> --policy-arn <policy_arn>
Equipped with a role that allows authentication to CodeArtifact, we can now continue testing.
Permissions Test
In the AWS Management Console, navigate to the SageMaker service and open a Jupyter notebook from the Internet-disabled notebook instance. In the notebook cell, attempt to log into the CodeArtifact repository using the same command from the network test (found in the Network Test section).
Instead of an access denied exception, the output should show a successful authentication to the repository with an expiration on the token. Continue testing by using pip to download, install, and uninstall packages. These commands are authorized based on the policy attached to the CodeArtifact repository. If you want to restrict access to the repository based on the user, for example, restricting the ability to uninstall a package, modify the CodeArtifact repository policy.
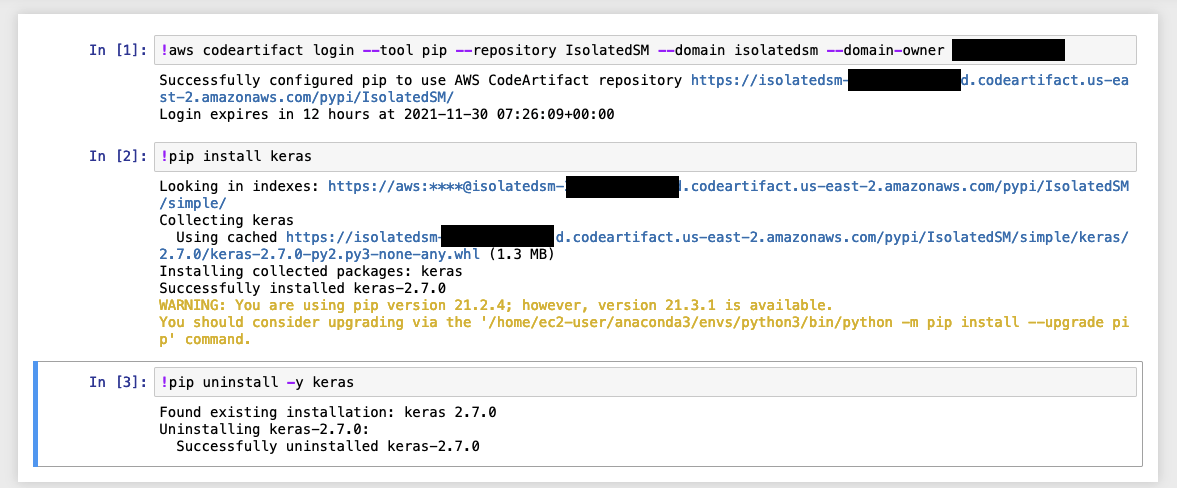
We can confirm that the packages are installed by navigating to the repository in the AWS Management Console and searching for the installed package.
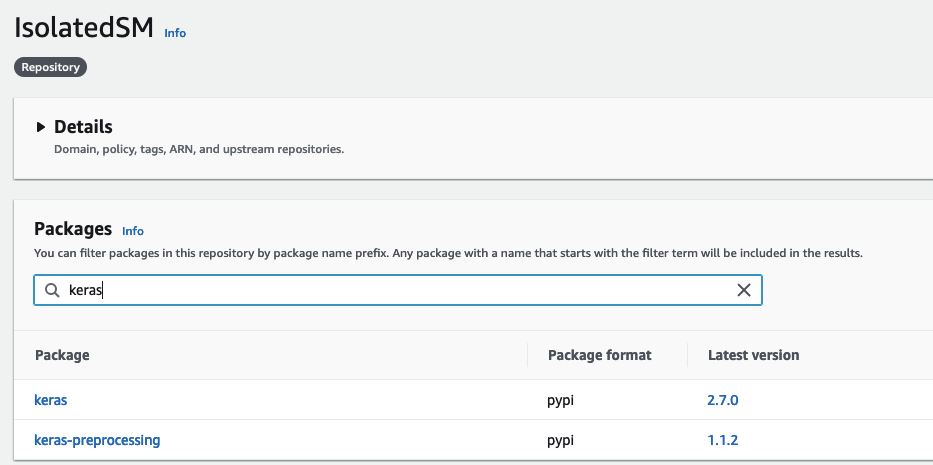
Clean up
When you destroy the VPC endpoints, the notebook instance loses access to the CodeArtifact repository. This reintroduces the timeout error from earlier in this post. This is expected behavior. Additionally, you may also delete the CodeArtifact repository, which charges customers based on the number of GBs of data stored per month.
Conclusion
By combining VPC endpoints with SageMaker notebooks, we can extend the availability of other AWS services to Internet-disabled private notebook instances. This allows us to improve the security posture of our development environment, without sacrificing developer productivity.
About the Author
Dan Ferguson is a Solutions Architect at Amazon Web Services, focusing primarily on Private Equity & Growth Equity investments into late-stage startups.
Read More