Subtitle creation on video content poses challenges no matter how big or small the organization. To address those challenges, Amazon Transcribe has a helpful feature that enables subtitle creation directly within the service. There is no machine learning (ML) or code writing required to get started. This post walks you through setting up a no-code workflow for creating video subtitles using Amazon Transcribe within your Amazon Web Services account.
Subtitles vs. closed captions
The terms subtitles and closed captions are commonly used interchangeably, and both refer to spoken text displayed on the screen. However, a primary difference between subtitles and closed captions (based on industry and accessibility definitions) is that closed captions contain both the transcription of the spoken word as well as a description of background music or sounds occurring within the audio track for a richer accessibility experience. This post only focuses on the creation of transcribed spoken word subtitle files using automatic speech recognition (ASR) technology that don’t contain speaker identification, sound effects, or music descriptions. Amazon Transcribe supports the industry standard SubRip Text (*.srt) and Web Video Text Tracks (*.vtt) formats for subtitle creation.
The following image shows an example of subtitles toggled on within a web video player.
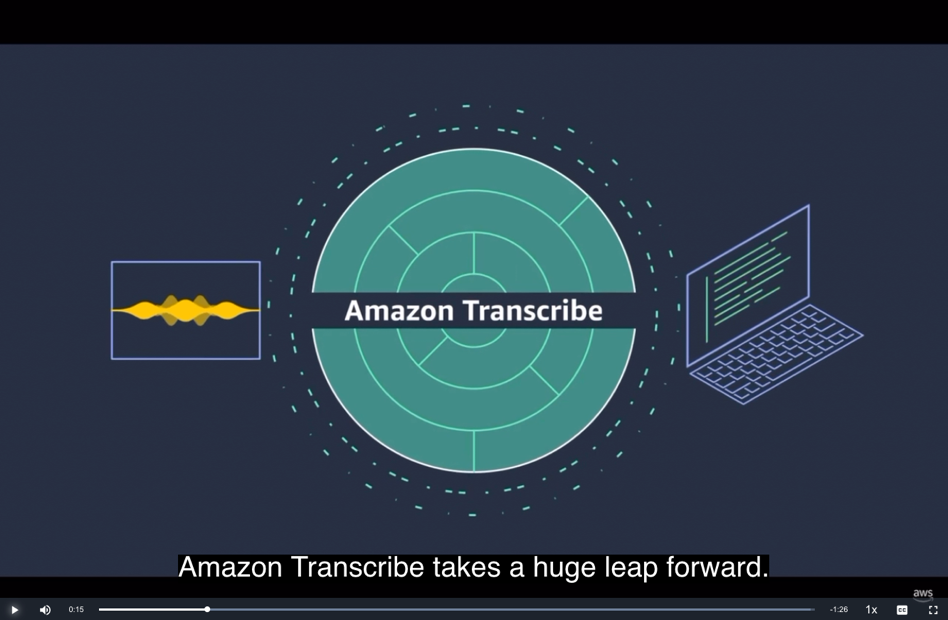
Subtitles benefit video creators by extending both the reach and inclusivity of their video content. By displaying the spoken audio portion of a video on the screen, subtitles make audio/video content accessible to a larger audience, including those that are non-native language speakers and those that are in an environment where sound is inaudible.
Although the benefits of subtitles are clear, video creators have traditionally faced obstacles in the creation of subtitles. Obstacles arise due to the time-consuming and resource-intensive requirements of the traditional creation process that heavily rely on manual effort. Traditional subtitling methods are manual and can take days to weeks to complete, and therefore may not be compatible with all production schedules. Likewise, many companies utilize manual transcription services, but these processes often don’t scale and are expensive to maintain. Amazon Transcribe makes it easy for you to convert speech to text using ML-based technologies and helps video creators address these issues.
Solution overview
This post walks through a no-code workflow for generating subtitles using Amazon Simple Storage Service (Amazon S3) and Amazon Transcribe.
Amazon S3 is object storage built to store and retrieve any amount of data from anywhere. This post walks through the process to create an S3 bucket and upload an audio file. When users store data in Amazon S3, they work with resources known as buckets and objects. A bucket is a container for objects. An object is a file and any metadata that describes that file.
Amazon Transcribe is an ASR service that uses fully managed and continuously trained ML models to convert audio/video files to text. Amazon Transcribe inputs and outputs are stored in Amazon S3. Amazon Transcribe takes audio data, either a media file in an Amazon S3 bucket or a media stream, and converts it to text data. Amazon Transcribe allows you to ingest audio input, produce easy-to-read transcripts with a high degree of accuracy, customize your output for domain specific vocabulary using custom language models (CLM) and custom vocabularies, and filter content to ensure customer privacy. Customers can choose to use Amazon Transcribe for a variety of business applications, including transcription of voice-based customer service calls, generation of subtitles on audio/video content, and conduct (text based) content analysis on audio/video content. For this post, we demonstrate creating a transcription job and reviewing the job output.
If you prefer a video walkthrough, refer to the Amazon Transcribe video snacks episode Creating video subtitles without writing any code.
Prerequisites
To walk through the solution, you must have the following prerequisites:
If you don’t already have a sample audio/video file, you can create one using a video recording application on your computer or smartphone. Make sure you’re speaking clearly into the microphone to ensure the highest level of transcription quality when recording. Another option is to find a freely available download featuring spoken word, such as a podcast, or the video walkthrough provided in this post, that can be ingested by Amazon Transcribe. The recorded or downloaded file needs to be accessible on your desktop for upload to your AWS account.
Before you get started, review the Amazon Transcribe and Amazon S3 pricing pages for service pricing.
Create the S3 buckets
For this post, we create two S3 buckets to keep the input and output separated.
- On the Amazon S3 console, choose Create bucket.
- Give each bucket a globally unique name.
- Use the default settings to ensure compliance with the policies of your organization.
- Enable bucket versioning and default server-side encryption (recommended).
- Choose Create bucket.
The following screenshot shows the configuration for the input bucket.
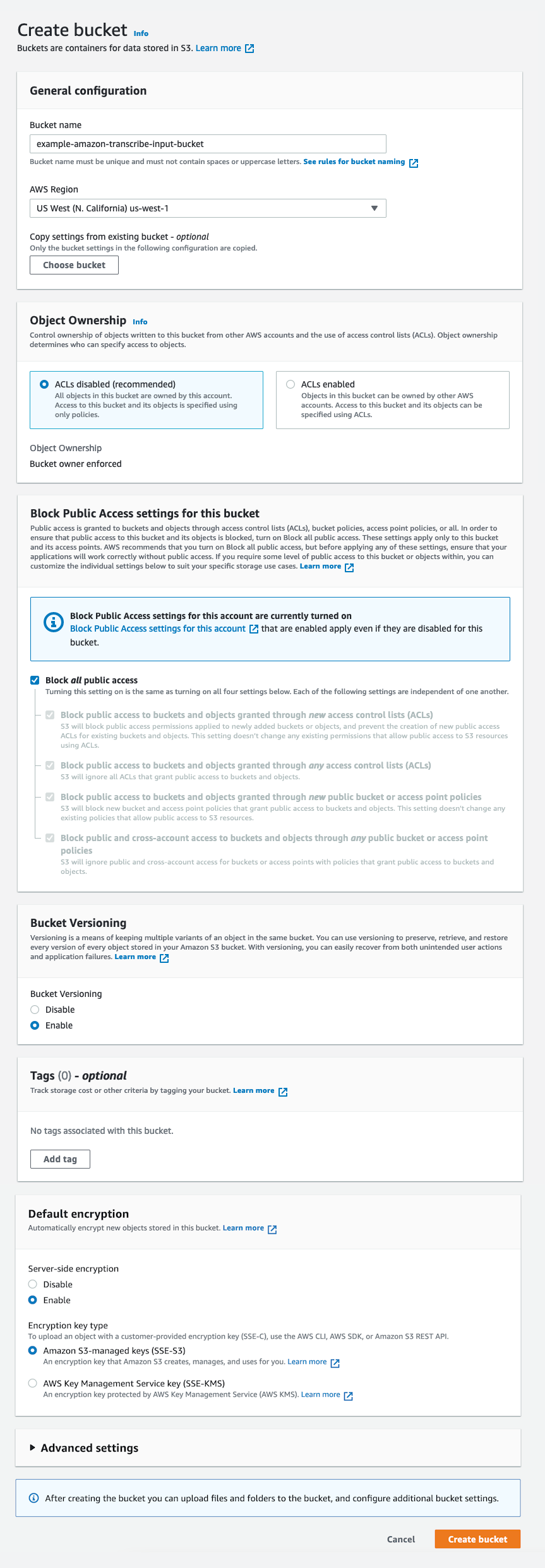
The S3 bucket for input is now ready to have the audio/video file uploaded. At the time of this publication, the maximum input size for Amazon Transcribe is 2 GB. If the video file exceeds that amount or is in a format that is not natively supported by Amazon Transcribe, consider using AWS Elemental MediaConvert to create an audio-only output. This is beneficial because audio files are typically much smaller than video files and Amazon Transcribe only requires the audio track, and not the video track, to generate transcriptions and subtitles.
Upload the source file to the S3 bucket
To upload your source file, complete the following steps:
- On the Amazon S3 console, select your input bucket.
- Choose Upload.
- Choose the file from your desktop.
- Accept the default storage class and encryption settings or modify them based on the policies of your organization.
- Choose Upload.
Create a transcription job
With the input file ready in Amazon S3, we now create a transcription job in Amazon Transcribe.
- On the Amazon Transcribe console, choose Transcription jobs in the navigation pane.
- Choose Create job.
This walkthrough largely uses default options; however, you should choose the configuration best suited to the requirements of your organization.
- For Name, enter in a name for this job and resulting file.
- For Language settings, select Specific language.
- For Language, choose the source language of the input file.
- For Model type¸ select General model.
We use the general model for this demo, but we encourage you to explore training and using custom language models for improved accuracy for specific use cases such as industry-specific terms or acronyms. For a deeper dive into custom language models, watch the Amazon Transcribe video snack Using Custom Language Models (CLM) to supercharge transcription accuracy.
- For Input file location on S3, choose Browse S3.
- Choose the input bucket and audio/video file to be transcribed.
- For Output data location type info, select Customer specified S3 bucket.
- For Output file destination on S3, choose Browse S3.
- Choose the newly created output bucket.
The Subtitle file format section provides the two most essential options of this entire post. You can select the *.srt and *.vtt formatted outputs as part of the Amazon Transcribe transcription job. At the time of this writing, selecting one or both doesn’t add any additional cost to the Amazon Transcribe job.
- For this post, select both SRT and VTT.
- For Specify the start index, choose 0 or 1.
This value refers to the starting number of the first subtitle in sequence. If you’re unsure which value to choose, 1 is the most common.
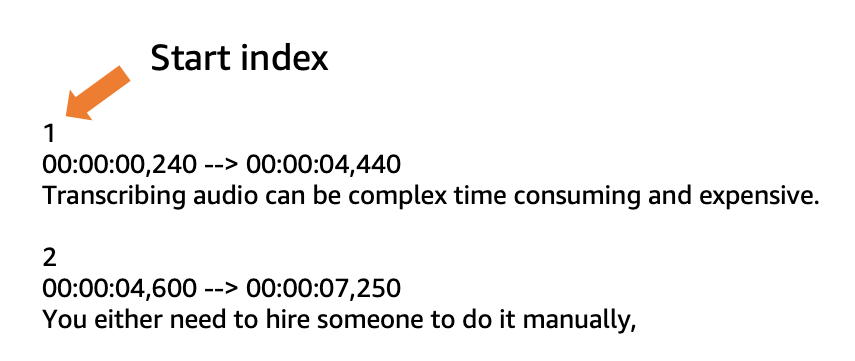
- When the settings are in place, choose Next.
- Configure any optional settings as per your needs.
Amazon Transcribe presents options for audio identification for channels or speakers, alternative results, PII redaction, vocabulary filtering, and custom vocabulary. For this particular post, you can skip these configuration options. For a deeper dive into job configuration options, watch the Amazon Transcribe video snacks episodes for custom vocabulary, custom language models, and vocabulary filtering.
- Choose Create job.
Review the job output
The transcription job to create your video subtitles starts. The job status, as shown in the following screenshot, is displayed in the job details panel. When the job is complete, choose the output data location to locate the newly created subtitles in the S3 bucket.
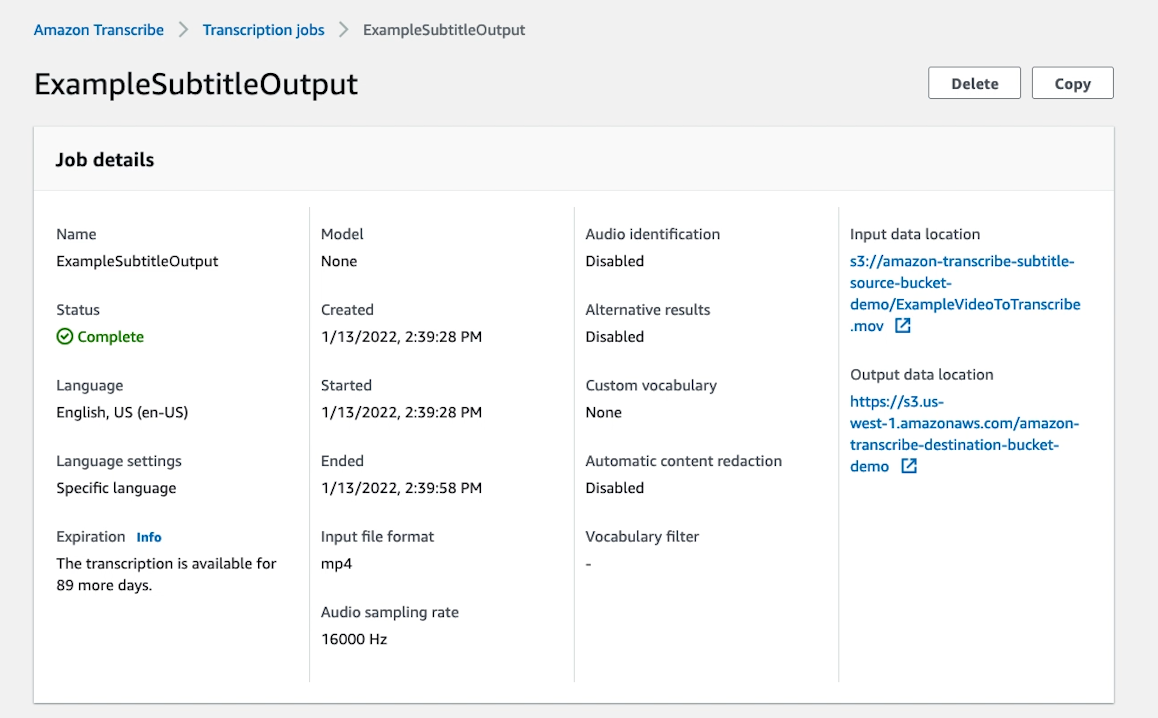
Subtitles are identified by the *.srt or *.vtt extensions. When you select the object in the S3 bucket, you have the option to download the file.
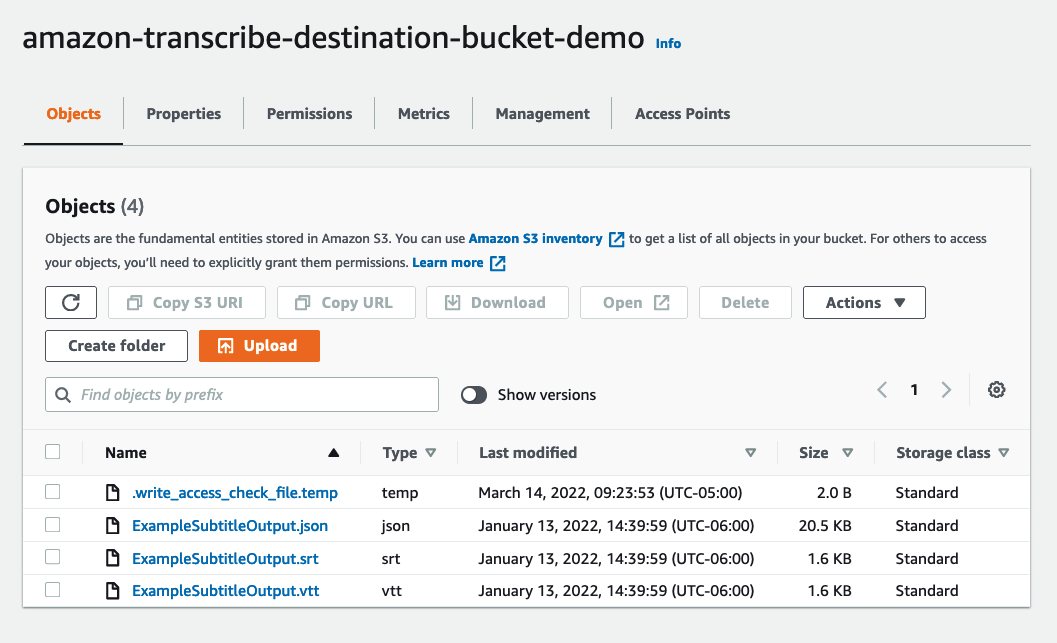
Because these subtitles are in plain text format, any text editor can view and edit the resulting transcription. Comparing the *.srt and *.vtt files reveals many similarities, with subtle differences.
The following is an example of *.srt format:
1
00:00:00,240 --> 00:00:04,440
Transcribing audio can be complex, time consuming and expensive.
2
00:00:04,600 --> 00:00:07,250
You either need to hire someone to do it manually,
3
00:00:07,490 --> 00:00:10,790
implement applications that are difficult to maintain, or use
4
00:00:10,790 --> 00:00:13,920
hard to integrate services that yield poor results.
5
00:00:14,540 --> 00:00:17,290
Amazon Transcribe takes a huge leap forward.
The following is an example of *.vtt format:
WEBVTT
1
00:00:00.240 --> 00:00:04.440
Transcribing audio can be complex, time consuming and expensive.
2
00:00:04.600 --> 00:00:07.250
You either need to hire someone to do it manually,
3
00:00:07.490 --> 00:00:10.790
implement applications that are difficult to maintain, or use
4
00:00:10.790 --> 00:00:13.920
hard to integrate services that yield poor results.
5
00:00:14.540 --> 00:00:17.290
Amazon Transcribe takes a huge leap forward.
The numbers indicate the order the subtitle is displayed. The timecode indicates when the subtitle is displayed. The text is the subtitle text itself.
Any changes or revisions are now possible directly within the text editor and remain compatible when saved with the *.srt or *.vtt extension. You can also preview changes on the video platform itself, inside a video editing application, or within a video player.
VLC is a popular open-source and cross-platform video player that supports *.srt and *.vtt subtitles. To automatically play subtitles over a video within VLC, place both the original video and the subtitle file in the same directory with the exact same file name before the file extension.

Now when you open the video file within VLC, the subtitle file should automatically detect and play back within the video player window.
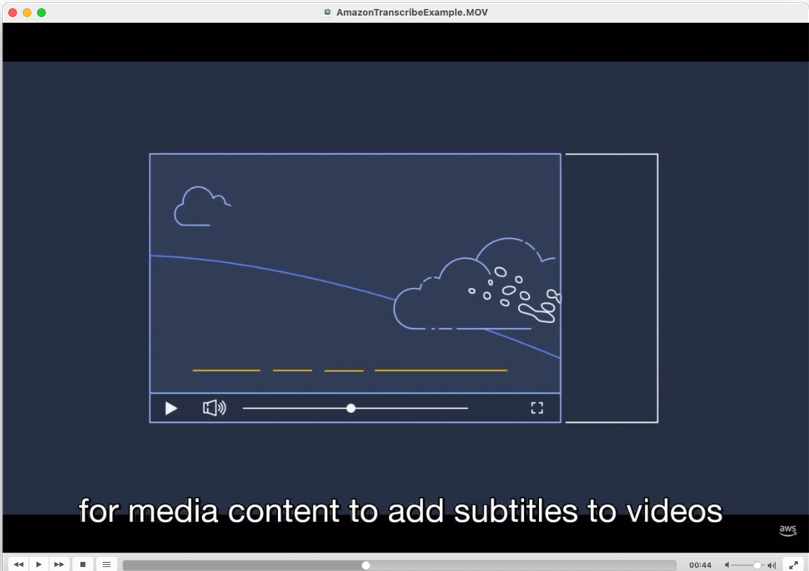
Clean up
To avoid incurring future charges, empty and delete the S3 buckets used for input and output. Ensure that you have all necessary files stored as this will permanently remove all objects contained within the buckets. On the Transcribe console, select and delete any jobs that are no longer needed.
Conclusion
You have now created a complete end-to-end subtitle creation workflow to augment and accelerate your video subtitle creation process, and all without writing any code. In a manner of minutes, you created S3 storage buckets, uploaded a file to Amazon S3, and used Amazon Transcribe for subtitle creation. You can then download the resulting *.srt and *.vtt subtitle files for review, and upload them to the destination platform.
This workflow focused on audio/video subtitles created using the automatic speech recognition (ASR) technology in Amazon Transcribe specifically for video workflows. This workflow alone is not a substitute for a human-based closed captioning process, which is able to meet higher standards for accessibility, including speaker identification, sound effects, music description, and copyediting review for accuracy. You can utilize the text editing method described in this post to add these elements after the initial Amazon Transcribe job is complete. Furthermore, for more advanced browser-based subtitle creation, preview, and copyediting, you can explore deploying the Content Localization on AWS solution that is vetted by AWS Solution Architects and includes an implementation guide. This solution offers additional features such as in-browser preview and editing of subtitles, subtitle translation powered by Amazon Translate, and computer vision capabilities offered by Amazon Rekognition.
If you enjoyed this demonstration of Amazon Transcribe’s capability to create subtitles, consider taking a deeper dive into additional features and capabilities to accelerate your audio/video workflows. For additional details and code samples to support automating and scaling subtitle creation, refer to Creating video subtitles. Good luck in your exploration and developing your subtitle creation workflow.
About the Author
Jason O’Malley is a Sr. Partner Solutions Architect at AWS supporting partners architecting media, communications, and technology industry solutions. Before joining AWS, Jason spent 13 years in the media and entertainment industry at companies including Conan O’Brien’s Team Coco, WarnerMedia, and Media.Monks. Jason started his career in television production and post-production before building media workloads on AWS. When Jason isn’t creating solutions for partners and customers, he can be found adventuring with his wife and son, or reading about sustainability.
Read More