Amazon’s director of applied science in Adelaide, Australia, believes the economic value of computer vision has “gone through the roof”.Read More
The King’s Swedish: AI Rewrites the Book in Scandinavia
If the King of Sweden wants help drafting his annual Christmas speech this year, he could ask the same AI model that’s available to his 10 million subjects.
As a test, researchers prompted the model, called GPT-SW3, to draft one of the royal messages, and it did a pretty good job, according to Magnus Sahlgren, who heads research in natural language understanding at AI Sweden, a consortium kickstarting the country’s journey into the machine learning era.
“Later, our minister of digitalization visited us and asked the model to generate arguments for political positions and it came up with some really clever ones — and he intuitively understood how to prompt the model to generate good text,” Sahlgren said.
Early successes inspired work on an even larger and more powerful version of the language model they hope will serve any citizen, company or government agency in Scandinavia.
A Multilingual Model
The current version packs 3.6 billion parameters and is smart enough to do a few cool things in Swedish. Sahlgren’s team aims to train a state-of-the-art model with a whopping 175 billion parameters that can handle all sorts of language tasks in the Nordic languages of Swedish, Danish, Norwegian and, it hopes, Icelandic, too.
For example, a startup can use it to automatically generate product descriptions for an e-commerce website given only the products’ names. Government agencies can use it to quickly classify and route questions from citizens.
Companies can ask it to rapidly summarize reports so they can react fast. Hospitals can run distilled versions of the model privately on their own systems to improve patient care.
“It’s a foundational model we will provide as a service for whatever tasks people want to solve,” said Sahlgren, who’s been working at the intersection of language and machine learning since he earned his Ph.D. in computational linguistics in 2006.
Permission to Speak Freely
It’s a capability increasingly seen as a strategic asset, a keystone of digital sovereignty in a world that speaks thousands of languages across nearly 200 countries.
Most language services today focus on Chinese or English, the world’s two most-spoken tongues. They’re typically created in China or the U.S., and they aren’t free.
“It’s important for us to have models built in Sweden for Sweden,” Sahlgren said.
Small Team, Super System
“We’re a small country and a core team of about six people, yet we can build a state-of-the-art resource like this for people to use,” he added.
That’s because Sweden has a powerful engine in BerzeLiUs, a 300-petaflops AI supercomputer at Linköping University. It trained the initial GPT-SW3 model using just 16 of the 60 nodes in the NVIDIA DGX SuperPOD.
The next model may exercise all the system’s nodes. Such super-sized jobs require super software like the NVIDIA NeMo Megatron framework.
“It lets us scale our training up to the full supercomputer, and we’ve been lucky enough to have access to experts in the NeMo development team — without NVIDIA it would have been so much more complicated to come this far,” he said.
A Workflow for Any Language
NVIDIA’s engineers created a recipe based on NeMo and an emerging process called p-tuning that optimizes massive models fast, and it’s geared to work with any language.
In one early test, a model nearly doubled its accuracy after NVIDIA engineers applied the techniques.
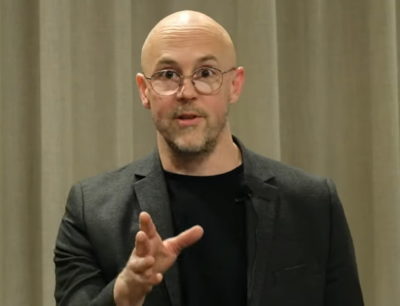
What’s more, it requires one-tenth the data, slashing the need for tens of thousands of hand-labeled records. That opens the door for users to fine-tune a model with the relatively small, industry-specific datasets they have at hand.
“We hope to inspire a lot of entrepreneurship in industry, startups and the public using our technology to develop their own apps and services,” said Sahlgren.
Writing the Next Chapter
Meanwhile, NVIDIA’s developers are already working on ways to make the enabling software better.
One test shows great promise for training new capabilities using widely available English datasets into models designed for any language. In another effort, they’re using the p-tuning techniques in inference jobs so models can learn on the fly.
Zenodia Charpy, a senior solutions architect at NVIDIA based in Gothenburg, shares the enthusiasm of the AI Sweden team she supports. “We’ve only just begun trying new and better methods to tackle these large language challenges — there’s much more to come,” she said.
The GPT-SW3 model will be made available by the end of year via an early access program. To apply, contact francisca.hoyer@ai.se.
The post The King’s Swedish: AI Rewrites the Book in Scandinavia appeared first on NVIDIA Blog.
BYOL-Explore: Exploration with Bootstrapped Prediction
We present BYOL-Explore, a conceptually simple yet general approach for curiosity-driven exploration in visually-complex environments. BYOL-Explore learns a world representation, the world dynamics, and an exploration policy all-together by optimizing a single prediction loss in the latent space with no additional auxiliary objective. We show that BYOL-Explore is effective in DM-HARD-8, a challenging partially-observable continuous-action hard-exploration benchmark with visually-rich 3-D environments.Read More
BYOL-Explore: Exploration with Bootstrapped Prediction
We present BYOL-Explore, a conceptually simple yet general approach for curiosity-driven exploration in visually-complex environments. BYOL-Explore learns a world representation, the world dynamics, and an exploration policy all-together by optimizing a single prediction loss in the latent space with no additional auxiliary objective. We show that BYOL-Explore is effective in DM-HARD-8, a challenging partially-observable continuous-action hard-exploration benchmark with visually-rich 3-D environments.Read More
Seeing the whole from some of the parts
Upon looking at photographs and drawing on their past experiences, humans can often perceive depth in pictures that are, themselves, perfectly flat. However, getting computers to do the same thing has proved quite challenging.
The problem is difficult for several reasons, one being that information is inevitably lost when a scene that takes place in three dimensions is reduced to a two-dimensional (2D) representation. There are some well-established strategies for recovering 3D information from multiple 2D images, but they each have some limitations. A new approach called “virtual correspondence,” which was developed by researchers at MIT and other institutions, can get around some of these shortcomings and succeed in cases where conventional methodology falters.
The standard approach, called “structure from motion,” is modeled on a key aspect of human vision. Because our eyes are separated from each other, they each offer slightly different views of an object. A triangle can be formed whose sides consist of the line segment connecting the two eyes, plus the line segments connecting each eye to a common point on the object in question. Knowing the angles in the triangle and the distance between the eyes, it’s possible to determine the distance to that point using elementary geometry — although the human visual system, of course, can make rough judgments about distance without having to go through arduous trigonometric calculations. This same basic idea — of triangulation or parallax views — has been exploited by astronomers for centuries to calculate the distance to faraway stars.
Triangulation is a key element of structure from motion. Suppose you have two pictures of an object — a sculpted figure of a rabbit, for instance — one taken from the left side of the figure and the other from the right. The first step would be to find points or pixels on the rabbit’s surface that both images share. A researcher could go from there to determine the “poses” of the two cameras — the positions where the photos were taken from and the direction each camera was facing. Knowing the distance between the cameras and the way they were oriented, one could then triangulate to work out the distance to a selected point on the rabbit. And if enough common points are identified, it might be possible to obtain a detailed sense of the object’s (or “rabbit’s”) overall shape.
Considerable progress has been made with this technique, comments Wei-Chiu Ma, a PhD student in MIT’s Department of Electrical Engineering and Computer Science (EECS), “and people are now matching pixels with greater and greater accuracy. So long as we can observe the same point, or points, across different images, we can use existing algorithms to determine the relative positions between cameras.” But the approach only works if the two images have a large overlap. If the input images have very different viewpoints — and hence contain few, if any, points in common — he adds, “the system may fail.”
During summer 2020, Ma came up with a novel way of doing things that could greatly expand the reach of structure from motion. MIT was closed at the time due to the pandemic, and Ma was home in Taiwan, relaxing on the couch. While looking at the palm of his hand and his fingertips in particular, it occurred to him that he could clearly picture his fingernails, even though they were not visible to him.
That was the inspiration for the notion of virtual correspondence, which Ma has subsequently pursued with his advisor, Antonio Torralba, an EECS professor and investigator at the Computer Science and Artificial Intelligence Laboratory, along with Anqi Joyce Yang and Raquel Urtasun of the University of Toronto and Shenlong Wang of the University of Illinois. “We want to incorporate human knowledge and reasoning into our existing 3D algorithms” Ma says, the same reasoning that enabled him to look at his fingertips and conjure up fingernails on the other side — the side he could not see.
Structure from motion works when two images have points in common, because that means a triangle can always be drawn connecting the cameras to the common point, and depth information can thereby be gleaned from that. Virtual correspondence offers a way to carry things further. Suppose, once again, that one photo is taken from the left side of a rabbit and another photo is taken from the right side. The first photo might reveal a spot on the rabbit’s left leg. But since light travels in a straight line, one could use general knowledge of the rabbit’s anatomy to know where a light ray going from the camera to the leg would emerge on the rabbit’s other side. That point may be visible in the other image (taken from the right-hand side) and, if so, it could be used via triangulation to compute distances in the third dimension.
Virtual correspondence, in other words, allows one to take a point from the first image on the rabbit’s left flank and connect it with a point on the rabbit’s unseen right flank. “The advantage here is that you don’t need overlapping images to proceed,” Ma notes. “By looking through the object and coming out the other end, this technique provides points in common to work with that weren’t initially available.” And in that way, the constraints imposed on the conventional method can be circumvented.
One might inquire as to how much prior knowledge is needed for this to work, because if you had to know the shape of everything in the image from the outset, no calculations would be required. The trick that Ma and his colleagues employ is to use certain familiar objects in an image — such as the human form — to serve as a kind of “anchor,” and they’ve devised methods for using our knowledge of the human shape to help pin down the camera poses and, in some cases, infer depth within the image. In addition, Ma explains, “the prior knowledge and common sense that is built into our algorithms is first captured and encoded by neural networks.”
The team’s ultimate goal is far more ambitious, Ma says. “We want to make computers that can understand the three-dimensional world just like humans do.” That objective is still far from realization, he acknowledges. “But to go beyond where we are today, and build a system that acts like humans, we need a more challenging setting. In other words, we need to develop computers that can not only interpret still images but can also understand short video clips and eventually full-length movies.”
A scene in the film “Good Will Hunting” demonstrates what he has in mind. The audience sees Matt Damon and Robin Williams from behind, sitting on a bench that overlooks a pond in Boston’s Public Garden. The next shot, taken from the opposite side, offers frontal (though fully clothed) views of Damon and Williams with an entirely different background. Everyone watching the movie immediately knows they’re watching the same two people, even though the two shots have nothing in common. Computers can’t make that conceptual leap yet, but Ma and his colleagues are working hard to make these machines more adept and — at least when it comes to vision — more like us.
The team’s work will be presented next week at the Conference on Computer Vision and Pattern Recognition.
Calculating the differential cost of code changes
Automated-reasoning method enables the calculation of tight bounds on the use of resources — such as computation or memory — that results from code changes.Read More
Announcing the winners of the 2022 Silent Data Corruptions at Scale request for proposals
Announcing the winners of the 2022 Silent Data Corruptions at Scale request for proposalsRead More
Evolution through large models
OpenAI Blog
Unlocking High-Accuracy Differentially Private Image Classification through Scale
According to empirical evidence from prior works, utility degradation in DP-SGD becomes more severe on larger neural network models – including the ones regularly used to achieve the best performance on challenging image classification benchmarks. Our work investigates this phenomenon and proposes a series of simple modifications to both the training procedure and model architecture, yielding a significant improvement on the accuracy of DP training on standard image classification benchmarks.Read More
Unlocking High-Accuracy Differentially Private Image Classification through Scale
According to empirical evidence from prior works, utility degradation in DP-SGD becomes more severe on larger neural network models – including the ones regularly used to achieve the best performance on challenging image classification benchmarks. Our work investigates this phenomenon and proposes a series of simple modifications to both the training procedure and model architecture, yielding a significant improvement on the accuracy of DP training on standard image classification benchmarks.Read More