The post Microsoft’s framework for building AI systems responsibly appeared first on The AI Blog.
Swin Transformer supports 3-billion-parameter vision models that can train with higher-resolution images for greater task applicability
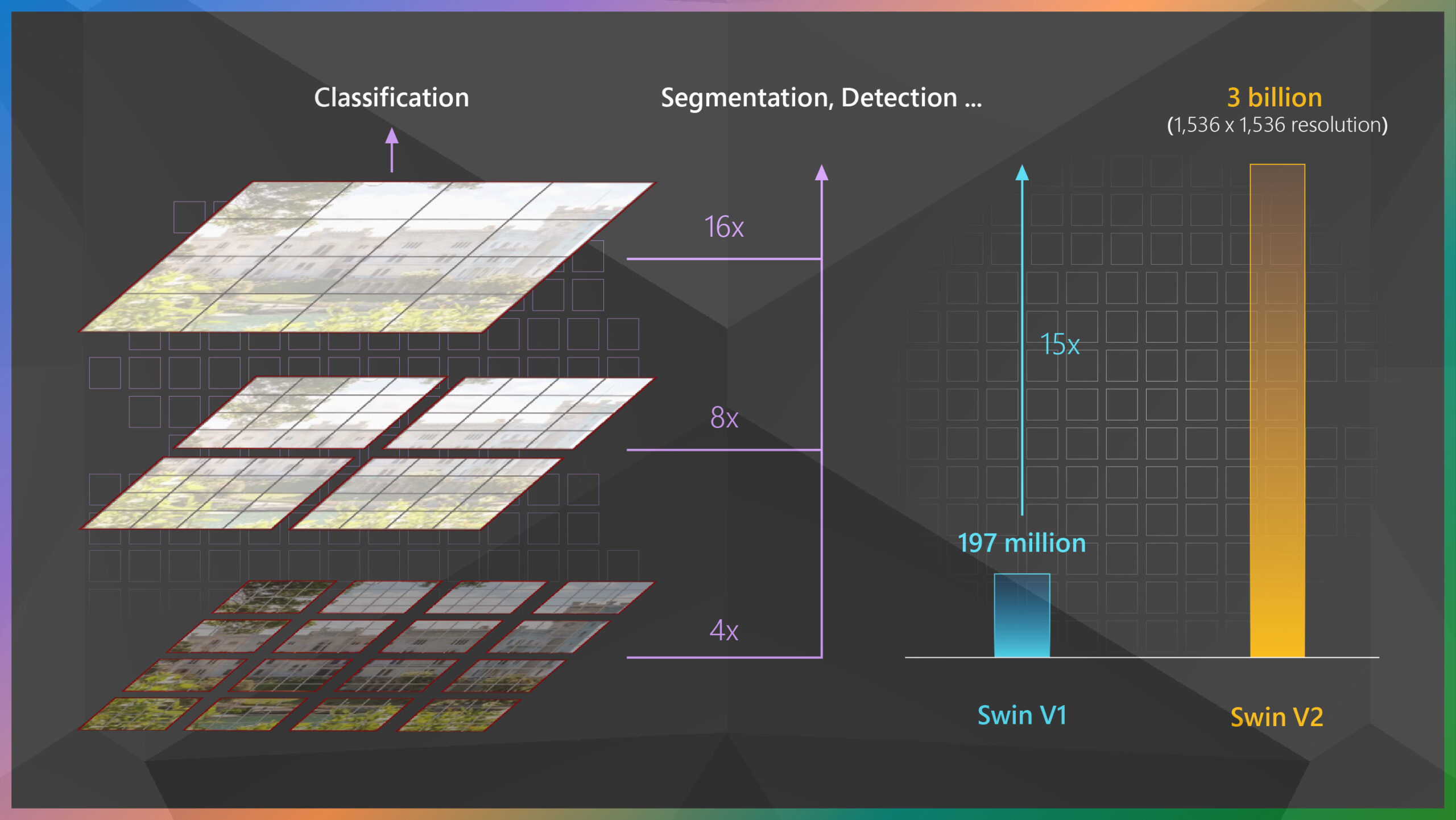
Early last year, our research team from the Visual Computing Group introduced Swin Transformer, a Transformer-based general-purpose computer vision architecture that for the first time beat convolutional neural networks on the important vision benchmark of COCO object detection and did so by a large margin. Convolutional neural networks (CNNs) have long been the architecture of choice for classifying images and detecting objects within them, among other key computer vision tasks. Swin Transformer offers an alternative. Leveraging the Transformer architecture’s adaptive computing capability, Swin can achieve higher accuracy. More importantly, Swin Transformer provides an opportunity to unify the architectures in computer vision and natural language processing (NLP), where the Transformer has been the dominant architecture for years and has benefited the field because of its ability to be scaled up.
So far, Swin Transformer has shown early signs of its potential as a strong backbone architecture for a variety of computer vision problems, powering the top entries of many important vision benchmarks such as COCO object detection, ADE20K semantic segmentation, and CelebA-HQ image generation. It has also been well-received by the computer vision research community, garnering the Marr Prize for best paper at the 2021 International Conference on Computer Vision (ICCV). Together with works such as CSWin, Focal Transformer, and CvT, also from teams within Microsoft, Swin is helping to demonstrate the Transformer architecture as a viable option for many vision challenges. However, we believe there’s much work ahead, and we’re on an adventurous journey to explore the full potential of Swin Transformer.
In the past few years, one of the most important discoveries in the field of NLP has been that scaling up model capacity can continually push the state of the art for various NLP tasks, and the larger the model, the better its ability to adapt to new tasks with very little or no training data. Can the same be achieved in computer vision, and if so, how?
In pursuit of answers, we scaled up Swin Transformer to 3 billion parameters, the largest and most effective dense vision model to date. There have been successfully trained vision models with up to 1.8 billion parameters. However, these vision models require billions of labeled images to be trained well and are applicable to only image classification. With our model, SwinV2-G, we address a common obstacle when increasing model size in the computer vision space—training instability—to support more parameters, and thanks to a technique we developed to address the resolution gap that exists between pretraining and fine-tuning tasks, SwinV2-G marks the first time that a billion-scale vision model has been applied to a broader set of vision tasks. Additionally, leveraging a self-supervised pretraining approach we call SimMIM, SwinV2-G uses 40 times less labeled data and 40 times less training time than previous works to drive the learning of billion-scale vision models.
SwinV2-G achieved state-of-the-art accuracy on four representative benchmarks when it was released in November: ImageNetV2 image classification, COCO object detection, ADE20K semantic segmentation, and Kinetics-400 video action classification.
Our experience and lessons learned in exploring the training and application of large vision models are described in two papers—“Swin Transformer V2: Scaling Up Capacity and Resolution” and “SimMIM: A Simple Framework for Masked Image Modeling”—both of which are being presented at the 2022 Computer Vision and Pattern Recognition Conference (CVPR). The code for Swin Transformer and the code for SimMIM are both available on GitHub. (For the purposes of this blog and our paper, the upgraded Swin Transformer architecture resulting from this work is referred to as V2.)
Improving training stability
The first issue we faced when training large models was the problem of training instability. We observed that as models get larger, it becomes very easy for them to crash. After checking the feature values of each layer of the models we trained in scaling up Swin Transformer to 3 billion parameters, we found the cause of the instability: large feature variance discrepancy between different layers.
As shown in Figure 1, the average feature variance in the deep layers of the original Swin Transformer model increases significantly as the model grows larger. With a 200-million-parameter Swin-L model, the discrepancy between layers with the highest and lowest average feature variance has reached an extreme value of 10^4. Crashing occurs during training when the model capacity is further scaled to 658 million parameters (Swin-H).
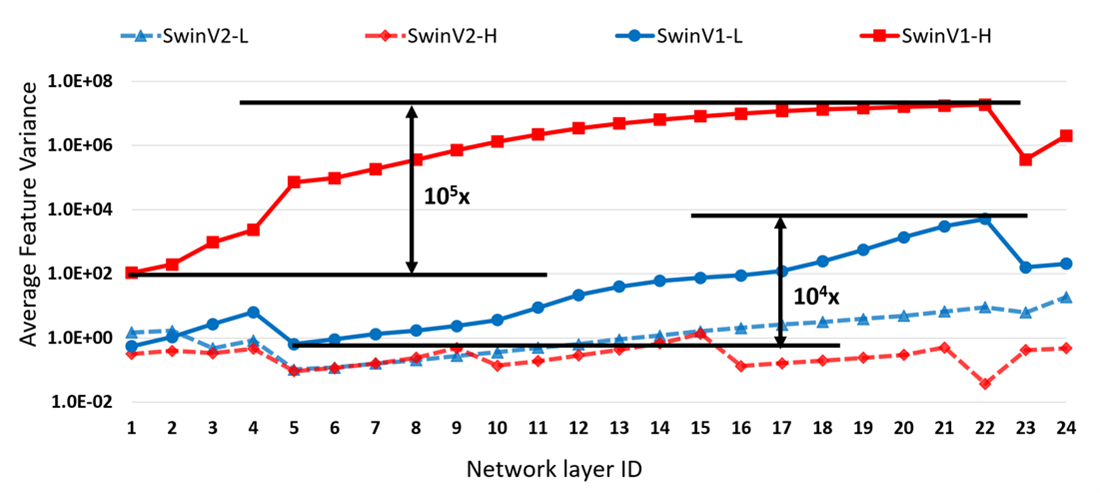
Looking closely at the architecture of the original Swin Transformer, we found that this was due to the output of the residual branch being added directly back to the main branch without normalization. In other words, the unconstrained output feature values could be very large compared to the input. As illustrated in Figure 2 (left), after one Transformer block, the feature values of the output can increase to 61 times larger than that of the input. To alleviate this problem, we propose a new normalization method called residual-post-normalization. As shown in Figure 2 (right), this method moves the normalization layer from the beginning to the end of each residual branch so that the output of each residual branch is normalized before being merged back into the main branch. In this way, the average feature variance of the main branch doesn’t increase significantly as the layers deepen. Experiments have shown that this new normalization method moderates the average feature variance of each layer in the model (see the dashed lines in Figure 1; the SwinV2 models have the same respective number of parameters as the SwinV1 models: 200 million [L] and 658 million [H]).
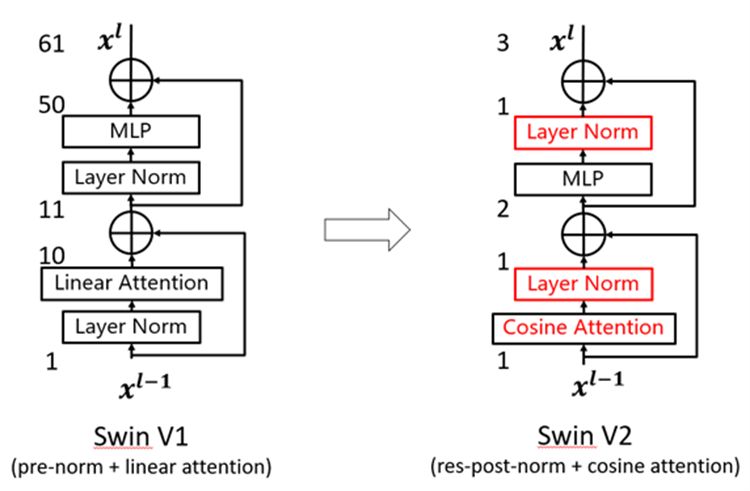
In addition, we also found that as the model becomes larger, the attention weights of certain layers tend to be dominated by a few specific points in the original self-attention computation, especially when residual-post-normalization is used. To tackle this problem, our team further proposes the scaled cosine attention mechanism (see Figure 2 right) to replace the common dot-product linear attention unit. In the new scaled cosine attention unit, the computation of self-attention is independent of the input magnitude, resulting in less saturated attention weights.
Experiments have shown that residual-post-normalization and the scaled cosine attention mechanism not only stabilize the training dynamics of large models but also improve accuracy.
Addressing large resolution gaps between pretraining and fine-tuning tasks
Another difficulty with large vision models is that the image resolution discrepancy between pretraining and fine-tuning can be large: pretraining is typically carried out at low resolutions, while many downstream vision tasks require high-resolution input images or attention windows, as shown in Figure 3.
In Swin Transformer, there’s a term of relative position bias in the attention unit to represent the impact of one image patch on another based on the relative position between them. This term is learned in pretraining. However, since the relative position range at fine-tuning has been changed significantly compared to that in pretraining, we need techniques to initiate the biases at new relative positions not seen in pretraining. While the original Swin Transformer architecture uses a handcrafted bicubic interpolation method to transfer the old relative position biases to the new resolution, we find it’s not that effective when the resolution discrepancy between pretraining and fine-tuning is very large.
To resolve this problem, we propose a log-spaced continuous position bias approach (Log-spaced CPB). By applying a small meta-network to the relative position coordinates in log space, Log-spaced CPB can generate position bias for any coordinate range. Since the meta-network can take arbitrary coordinates as input, a pretrained model can freely transfer between different window sizes by sharing the weights of a meta-network. Moreover, by converting the coordinates to a log space, the extrapolation rate required to transfer between different window resolutions is much smaller than with using the original linear space coordinates.
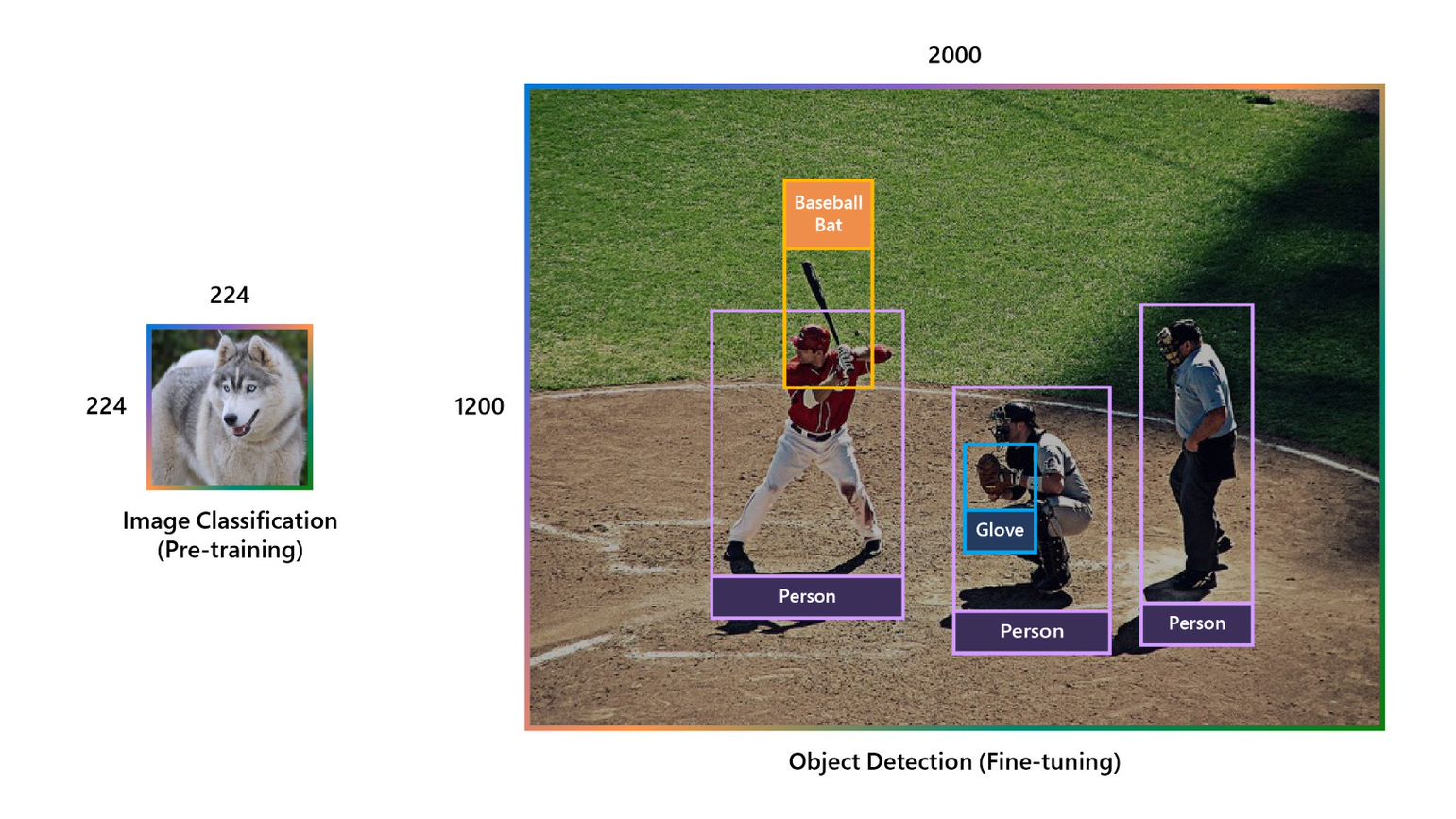
Using Log-spaced CPB, Swin Transformer V2 achieves smooth transferring between different resolutions, enabling us to use a smaller image resolution—192 × 192—with no accuracy loss on downstream tasks compared with the standard 224 × 224 resolution used in pretraining. This speeds up training by 50 percent.
Scaling model capacity and resolution results in excessive GPU memory consumption for existing vision models. To address the memory issue, we combined several crucial techniques, including Zero-Redundancy Optimizer (ZeRO), activation checkpointing, and a new sequential self-attention implementation. With these techniques, GPU memory consumption is significantly reduced for large-scale models and large resolutions with little impact to training speed. The GPU savings also allows us to train the 3-billion-parameter SwinV2-G model on images with resolutions of up to 1,536 × 1,536 using the 40-gigabyte A100 GPU, making it applicable to a range of vision tasks requiring high resolution, such as object detection.
Tackling the data starvation problem for large vision models
Training larger models often requires more labeled data; however, the computer vision field lacks such labeled data at scale because of the high cost of human-annotated data. This has compelled the vision field to explore the training of large models with smaller amounts of labeled data. To this aim, we introduce the self-supervised pretraining approach SimMIM, short for Simple Framework for Masked Image Modeling.
As shown in Figure 4, SimMIM learns image representation by masked image modeling, a pretext task in which a portion of an input image is masked and then the model predicts the RGB values of the masked area given the other visible parts. By this approach, the rich information contained in each image is better exploited, which allows us to use less data to drive the training of large models. With SimMIM, we were able to train the SwinV2-G model by using only 70 million labeled images, which is 40 times less than that used by previous billion-scale vision models.
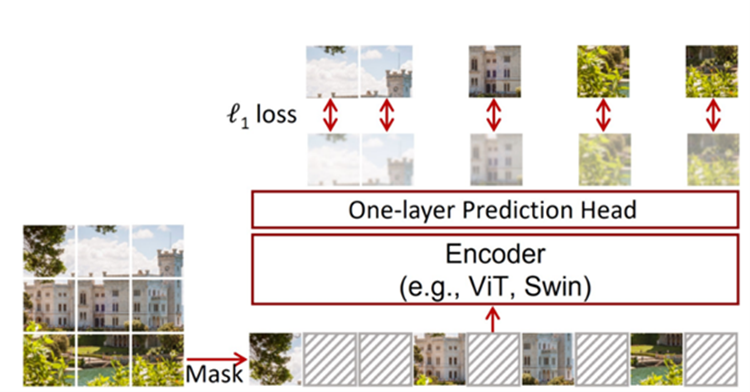
Setting new records on four representative vision benchmarks
By scaling up model capacity and resolution, Swin Transformer V2 set new records on four representative vision benchmarks when it was introduced in November: 84.0 percent top-1 accuracy on ImageNetV2 image classification; 63.1 / 54.4 box / mask mean average precision (mAP) on COCO object detection; 59.9 mean Intersection-over-Union (mIoU) on ADE20K semantic segmentation; and 86.8 percent top-1 accuracy on Kinetics-400 video action classification.
Benchmark | ImageNetV2 | COCO test-dev | ADE20K val | Kinetics-400 |
---|---|---|---|---|
Swin V1 | 77.5 | 58.7 | 53.5 | 84.9 |
Previous state of the art | 83.3 (Google, July 2021) | 61.3 (Microsoft, July 2021) | 58.4 (Microsoft, October 2021) | 85.4 (Google, October 2021) |
Swin V2 (November 2021) | 84.0 (+0.7) | 63.1 (+1.8) | 59.9 (+1.5) | 86.8 (+1.4) |
We hope this strong performance on a variety of vision tasks can encourage the field to invest more in scaling up vision models and that the provided training “recipe” can facilitate future research in this direction.
To learn more about the Swin Transformer journey, check out our Tech Minutes video.
Swin Transformer research team
(In alphabetical order) Yue Cao, Li Dong, Baining Guo, Han Hu, Stephen Lin, Yutong Lin, Ze Liu, Jia Ning, Furu Wei, Yixuan Wei, Zhenda Xie, Zhuliang Yao, and Zheng Zhang
The post Swin Transformer supports 3-billion-parameter vision models that can train with higher-resolution images for greater task applicability appeared first on Microsoft Research.
Researchers release open-source photorealistic simulator for autonomous driving
Hyper-realistic virtual worlds have been heralded as the best driving schools for autonomous vehicles (AVs), since they’ve proven fruitful test beds for safely trying out dangerous driving scenarios. Tesla, Waymo, and other self-driving companies all rely heavily on data to enable expensive and proprietary photorealistic simulators, since testing and gathering nuanced I-almost-crashed data usually isn’t the most easy or desirable to recreate.
To that end, scientists from MIT’s Computer Science and Artificial Intelligence Laboratory (CSAIL) created “VISTA 2.0,” a data-driven simulation engine where vehicles can learn to drive in the real world and recover from near-crash scenarios. What’s more, all of the code is being open-sourced to the public.
“Today, only companies have software like the type of simulation environments and capabilities of VISTA 2.0, and this software is proprietary. With this release, the research community will have access to a powerful new tool for accelerating the research and development of adaptive robust control for autonomous driving,” says MIT Professor and CSAIL Director Daniela Rus, senior author on a paper about the research.
VISTA 2.0 builds off of the team’s previous model, VISTA, and it’s fundamentally different from existing AV simulators since it’s data-driven — meaning it was built and photorealistically rendered from real-world data — thereby enabling direct transfer to reality. While the initial iteration supported only single car lane-following with one camera sensor, achieving high-fidelity data-driven simulation required rethinking the foundations of how different sensors and behavioral interactions can be synthesized.
Enter VISTA 2.0: a data-driven system that can simulate complex sensor types and massively interactive scenarios and intersections at scale. With much less data than previous models, the team was able to train autonomous vehicles that could be substantially more robust than those trained on large amounts of real-world data.
“This is a massive jump in capabilities of data-driven simulation for autonomous vehicles, as well as the increase of scale and ability to handle greater driving complexity,” says Alexander Amini, CSAIL PhD student and co-lead author on two new papers, together with fellow PhD student Tsun-Hsuan Wang. “VISTA 2.0 demonstrates the ability to simulate sensor data far beyond 2D RGB cameras, but also extremely high dimensional 3D lidars with millions of points, irregularly timed event-based cameras, and even interactive and dynamic scenarios with other vehicles as well.”
The team was able to scale the complexity of the interactive driving tasks for things like overtaking, following, and negotiating, including multiagent scenarios in highly photorealistic environments.
Training AI models for autonomous vehicles involves hard-to-secure fodder of different varieties of edge cases and strange, dangerous scenarios, because most of our data (thankfully) is just run-of-the-mill, day-to-day driving. Logically, we can’t just crash into other cars just to teach a neural network how to not crash into other cars.
Recently, there’s been a shift away from more classic, human-designed simulation environments to those built up from real-world data. The latter have immense photorealism, but the former can easily model virtual cameras and lidars. With this paradigm shift, a key question has emerged: Can the richness and complexity of all of the sensors that autonomous vehicles need, such as lidar and event-based cameras that are more sparse, accurately be synthesized?
Lidar sensor data is much harder to interpret in a data-driven world — you’re effectively trying to generate brand-new 3D point clouds with millions of points, only from sparse views of the world. To synthesize 3D lidar point clouds, the team used the data that the car collected, projected it into a 3D space coming from the lidar data, and then let a new virtual vehicle drive around locally from where that original vehicle was. Finally, they projected all of that sensory information back into the frame of view of this new virtual vehicle, with the help of neural networks.
Together with the simulation of event-based cameras, which operate at speeds greater than thousands of events per second, the simulator was capable of not only simulating this multimodal information, but also doing so all in real time — making it possible to train neural nets offline, but also test online on the car in augmented reality setups for safe evaluations. “The question of if multisensor simulation at this scale of complexity and photorealism was possible in the realm of data-driven simulation was very much an open question,” says Amini.
With that, the driving school becomes a party. In the simulation, you can move around, have different types of controllers, simulate different types of events, create interactive scenarios, and just drop in brand new vehicles that weren’t even in the original data. They tested for lane following, lane turning, car following, and more dicey scenarios like static and dynamic overtaking (seeing obstacles and moving around so you don’t collide). With the multi-agency, both real and simulated agents interact, and new agents can be dropped into the scene and controlled any which way.
Taking their full-scale car out into the “wild” — a.k.a. Devens, Massachusetts — the team saw immediate transferability of results, with both failures and successes. They were also able to demonstrate the bodacious, magic word of self-driving car models: “robust.” They showed that AVs, trained entirely in VISTA 2.0, were so robust in the real world that they could handle that elusive tail of challenging failures.
Now, one guardrail humans rely on that can’t yet be simulated is human emotion. It’s the friendly wave, nod, or blinker switch of acknowledgement, which are the type of nuances the team wants to implement in future work.
“The central algorithm of this research is how we can take a dataset and build a completely synthetic world for learning and autonomy,” says Amini. “It’s a platform that I believe one day could extend in many different axes across robotics. Not just autonomous driving, but many areas that rely on vision and complex behaviors. We’re excited to release VISTA 2.0 to help enable the community to collect their own datasets and convert them into virtual worlds where they can directly simulate their own virtual autonomous vehicles, drive around these virtual terrains, train autonomous vehicles in these worlds, and then can directly transfer them to full-sized, real self-driving cars.”
Amini and Wang wrote the paper alongside Zhijian Liu, MIT CSAIL PhD student; Igor Gilitschenski, assistant professor in computer science at the University of Toronto; Wilko Schwarting, AI research scientist and MIT CSAIL PhD ’20; Song Han, associate professor at MIT’s Department of Electrical Engineering and Computer Science; Sertac Karaman, associate professor of aeronautics and astronautics at MIT; and Daniela Rus, MIT professor and CSAIL director. The researchers presented the work at the IEEE International Conference on Robotics and Automation (ICRA) in Philadelphia.
This work was supported by the National Science Foundation and Toyota Research Institute. The team acknowledges the support of NVIDIA with the donation of the Drive AGX Pegasus.
Google at CVPR 2022
This week marks the beginning of the premier annual Computer Vision and Pattern Recognition conference (CVPR 2022), held both in-person in New Orleans, LA and virtually. As a leader in computer vision research and a Platinum Sponsor, Google will have a strong presence across CVPR 2022 with over 80 papers being presented at the main conference and active involvement in a number of conference workshops and tutorials.
If you are attending CVPR this year, please stop by our booth and chat with our researchers who are actively exploring the latest machine learning techniques for application to various areas of machine perception. Our researchers will also be available to talk about and demo several recent efforts, including on-device ML applications with MediaPipe, the Auto Arborist Dataset for urban forest monitoring, and much more.
You can also learn more about our research being presented at CVPR 2022 in the list below (Google affiliations in bold).
Organizing Committee
Tutorials Chairs
Include: Boqing Gong
Website Chairs
Include: AJ Piergiovanni
Area Chairs
Include: Alireza Fathi, Cordelia Schmid, Deqing Sun, Jonathan Barron, Michael Ryoo, Supasorn Suwajanakorn, Susanna Ricco
Diversity, Equity, and Inclusion Chairs
Include: Noah Snavely
Panel Discussion: Embodied Computer Vision
Panelists include: Michael Ryoo
Publications
Learning to Prompt for Continual Learning (see blog post)
Zifeng Wang*, Zizhao Zhang, Chen-Yu Lee, Han Zhang, Ruoxi Sun, Xiaoqi Ren, Guolong Su, Vincent Perot, Jennifer Dy, Tomas Pfister
GCR: Gradient Coreset Based Replay Buffer Selection for Continual Learning
Rishabh Tiwari, Krishnateja Killamsetty, Rishabh Iyer, Pradeep Shenoy
Zero-Shot Text-Guided Object Generation with Dream Fields
Ajay Jain, Ben Mildenhall, Jonathan T. Barron, Pieter Abbeel, Ben Poole
Towards End-to-End Unified Scene Text Detection and Layout Analysis
Shangbang Long, Siyang Qin, Dmitry Panteleev, Alessandro Bissacco, Yasuhisa Fujii, Michalis Raptis
FLOAT: Factorized Learning of Object Attributes for Improved Multi-object Multi-part Scene Parsing
Rishubh Singh, Pranav Gupta, Pradeep Shenoy, Ravikiran Sarvadevabhatla
LOLNerf: Learn from One Look
Daniel Rebain, Mark Matthews, Kwang Moo Yi, Dmitry Lagun, Andrea Tagliasacchi
Photorealistic Monocular 3D Reconstruction of Humans Wearing Clothing
Thiemo Alldieck, Mihai Zanfir, Cristian Sminchisescu
Learning Local Displacements for Point Cloud Completion
Yida Wang, David Joseph Tan, Nassir Navab, Federico Tombari
Density-Preserving Deep Point Cloud Compression
Yun He, Xinlin Ren, Danhang Tang, Yinda Zhang, Xiangyang Xue, Yanwei Fu
CMT-DeepLab: Clustering Mask Transformers for Panoptic Segmentation
Qihang Yu*, Huiyu Wang, Dahun Kim, Siyuan Qiao, Maxwell Collins, Yukun Zhu, Hartwig Adam, Alan Yuille, Liang-Chieh Chen
Deformable Sprites for Unsupervised Video Decomposition
Vickie Ye, Zhengqi Li, Richard Tucker, Angjoo Kanazawa, Noah Snavely
Learning with Neighbor Consistency for Noisy Labels
Ahmet Iscen, Jack Valmadre, Anurag Arnab, Cordelia Schmid
Multiview Transformers for Video Recognition
Shen Yan, Xuehan Xiong, Anurag Arnab, Zhichao Lu, Mi Zhang, Chen Sun, Cordelia Schmid
Kubric: A Scalable Dataset Generator
Klaus Greff, Francois Belletti, Lucas Beyer, Carl Doersch, Yilun Du, Daniel Duckworth, David J. Fleet, Dan Gnanapragasam, Florian Golemo, Charles Herrmann, Thomas Kipf, Abhijit Kundu, Dmitry Lagun, Issam Laradji, Hsueh-Ti (Derek) Liu, Henning Meyer, Yishu Miao, Derek Nowrouzezahrai, Cengiz Oztireli, Etienne Pot, Noha Radwan*, Daniel Rebain, Sara Sabour, Mehdi S. M. Sajjadi, Matan Sela, Vincent Sitzmann, Austin Stone, Deqing Sun, Suhani Vora, Ziyu Wang, Tianhao Wu, Kwang Moo Yi, Fangcheng Zhong, Andrea Tagliasacchi
3D Moments from Near-Duplicate Photos
Qianqian Wang, Zhengqi Li, David Salesin, Noah Snavely, Brian Curless, Janne Kontkanen
Mip-NeRF 360: Unbounded Anti-Aliased Neural Radiance Fields
Jonathan T. Barron, Ben Mildenhall, Dor Verbin, Pratul P. Srinivasan, Peter Hedman
RegNeRF: Regularizing Neural Radiance Fields for View Synthesis from Sparse Inputs
Michael Niemeyer*, Jonathan T. Barron, Ben Mildenhall, Mehdi S. M. Sajjadi, Andreas Geiger, Noha Radwan*
Ref-NeRF: Structured View-Dependent Appearance for Neural Radiance Fields
Dor Verbin, Peter Hedman, Ben Mildenhall, Todd Zickler, Jonathan T. Barron, Pratul P. Srinivasan
IRON: Inverse Rendering by Optimizing Neural SDFs and Materials from Photometric Images
Kai Zhang, Fujun Luan, Zhengqi Li, Noah Snavely
MAXIM: Multi-Axis MLP for Image Processing
Zhengzhong Tu*, Hossein Talebi, Han Zhang, Feng Yang, Peyman Milanfar, Alan Bovik, Yinxiao Li
Restormer: Efficient Transformer for High-Resolution Image Restoration
Syed Waqas Zamir, Aditya Arora, Salman Khan, Munawar Hayat, Fahad Shahbaz Khan, Ming-Hsuan Yang
Burst Image Restoration and Enhancement
Akshay Dudhane, Syed Waqas Zamir, Salman Khan, Fahad Shahbaz Khan, Ming-Hsuan Yang
Neural RGB-D Surface Reconstruction
Dejan Azinović, Ricardo Martin-Brualla, Dan B Goldman, Matthias Nießner, Justus Thies
Scene Representation Transformer: Geometry-Free Novel View Synthesis Through Set-Latent Scene Representations
Mehdi S. M. Sajjadi, Henning Meyer, Etienne Pot, Urs Bergmann, Klaus Greff, Noha Radwan*, Suhani Vora, Mario Lučić, Daniel Duckworth, Alexey Dosovitskiy*, Jakob Uszkoreit*, Thomas Funkhouser, Andrea Tagliasacchi*
ZebraPose: Coarse to Fine Surface Encoding for 6DoF Object Pose Estimation
Yongzhi Su, Mahdi Saleh, Torben Fetzer, Jason Rambach, Nassir Navab, Benjamin Busam, Didier Stricker, Federico Tombari
MetaPose: Fast 3D Pose from Multiple Views without 3D Supervision
Ben Usman, Andrea Tagliasacchi, Kate Saenko, Avneesh Sud
GPV-Pose: Category-Level Object Pose Estimation via Geometry-Guided Point-wise Voting
Yan Di, Ruida Zhang, Zhiqiang Lou, Fabian Manhardt, Xiangyang Ji, Nassir Navab, Federico Tombari
Rethinking Deep Face Restoration
Yang Zhao*, Yu-Chuan Su, Chun-Te Chu, Yandong Li, Marius Renn, Yukun Zhu, Changyou Chen, Xuhui Jia
Transferability Metrics for Selecting Source Model Ensembles
Andrea Agostinelli, Jasper Uijlings, Thomas Mensink, Vittorio Ferrari
Robust Fine-Tuning of Zero-Shot Models
Mitchell Wortsman, Gabriel Ilharco, Jong Wook Kim, Mike Li, Simon Kornblith, Rebecca Roelofs, Raphael Gontijo Lopes, Hannaneh Hajishirzi, Ali Farhadi, Hongseok Namkoong, Ludwig Schmidt
Block-NeRF: Scalable Large Scene Neural View Synthesis
Matthew Tancik, Vincent Casser, Xinchen Yan, Sabeek Pradhan, Ben Mildenhall, Pratul P. Srinivasan, Jonathan T. Barron, Henrik Kretzschmar
Light Field Neural Rendering
Mohammad Suhail*, Carlos Esteves, Leonid Sigal, Ameesh Makadia
Transferability Estimation Using Bhattacharyya Class Separability
Michal Pándy, Andrea Agostinelli, Jasper Uijlings, Vittorio Ferrari, Thomas Mensink
Matching Feature Sets for Few-Shot Image Classification
Arman Afrasiyabi, Hugo Larochelle, Jean-François Lalonde, Christian Gagné
Which Model to Transfer? Finding the Needle in the Growing Haystack
Cedric Renggli, André Susano Pinto, Luka Rimanic, Joan Puigcerver, Carlos Riquelme, Ce Zhang, Mario Lučić
Auditing Privacy Defenses in Federated Learning via Generative Gradient Leakage
Zhuohang Li, Jiaxin Zhang, Luyang Liu, Jian Liu
Estimating Example Difficulty Using Variance of Gradients
Chirag Agarwal, Daniel D’souza, Sara Hooker
More Than Words: In-the-Wild Visually-Driven Prosody for Text-to-Speech (see blog post)
Michael Hassid, Michelle Tadmor Ramanovich, Brendan Shillingford, Miaosen Wang, Ye Jia, Tal Remez
Robust Outlier Detection by De-Biasing VAE Likelihoods
Kushal Chauhan, Barath Mohan U, Pradeep Shenoy, Manish Gupta, Devarajan Sridharan
Deep 3D-to-2D Watermarking: Embedding Messages in 3D Meshes and Extracting Them from 2D Renderings
Innfarn Yoo, Huiwen Chang, Xiyang Luo, Ondrej Stava, Ce Liu*, Peyman Milanfar, Feng Yang
Knowledge Distillation: A Good Teacher Is Patient and Consistent
Lucas Beyer, Xiaohua Zhai, Amélie Royer*, Larisa Markeeva*, Rohan Anil, Alexander Kolesnikov
Urban Radiance Fields
Konstantinos Rematas, Andrew Liu, Pratul P. Srinivasan, Jonathan T. Barron, Andrea Tagliasacchi, Thomas Funkhouser, Vittorio Ferrari
Manifold Learning Benefits GANs
Yao Ni, Piotr Koniusz, Richard Hartley, Richard Nock
MaskGIT: Masked Generative Image Transformer
Huiwen Chang, Han Zhang, Lu Jiang, Ce Liu*, William T. Freeman
InOut: Diverse Image Outpainting via GAN Inversion
Yen-Chi Cheng, Chieh Hubert Lin, Hsin-Ying Lee, Jian Ren, Sergey Tulyakov, Ming-Hsuan Yang
Scaling Vision Transformers (see blog post)
Xiaohua Zhai, Alexander Kolesnikov, Neil Houlsby, Lucas Beyer
Fine-Tuning Image Transformers Using Learnable Memory
Mark Sandler, Andrey Zhmoginov, Max Vladymyrov, Andrew Jackson
PokeBNN: A Binary Pursuit of Lightweight Accuracy
Yichi Zhang*, Zhiru Zhang, Lukasz Lew
Bending Graphs: Hierarchical Shape Matching Using Gated Optimal Transport
Mahdi Saleh, Shun-Cheng Wu, Luca Cosmo, Nassir Navab, Benjamin Busam, Federico Tombari
Uncertainty-Aware Deep Multi-View Photometric Stereo
Berk Kaya, Suryansh Kumar, Carlos Oliveira, Vittorio Ferrari, Luc Van Gool
Depth-Supervised NeRF: Fewer Views and Faster Training for Free
Kangle Deng, Andrew Liu, Jun-Yan Zhu, Deva Ramanan
Dense Depth Priors for Neural Radiance Fields from Sparse Input Views
Barbara Roessle, Jonathan T. Barron, Ben Mildenhall, Pratul P. Srinivasan, Matthias Nießner
Trajectory Optimization for Physics-Based Reconstruction of 3D Human Pose from Monocular Video
Erik Gärtner, Mykhaylo Andriluka, Hongyi Xu, Cristian Sminchisescu
Differentiable Dynamics for Articulated 3D Human Motion Reconstruction
Erik Gärtner, Mykhaylo Andriluka, Erwin Coumans, Cristian Sminchisescu
Panoptic Neural Fields: A Semantic Object-Aware Neural Scene Representation
Abhijit Kundu, Kyle Genova, Xiaoqi Yin, Alireza Fathi, Caroline Pantofaru, Leonidas J. Guibas, Andrea Tagliasacchi, Frank Dellaert, Thomas Funkhouser
Pyramid Adversarial Training Improves ViT Performance
Charles Herrmann, Kyle Sargent, Lu Jiang, Ramin Zabih, Huiwen Chang, Ce Liu*, Dilip Krishnan, Deqing Sun
Proper Reuse of Image Classification Features Improves Object Detection
Cristina Vasconcelos, Vighnesh Birodkar, Vincent Dumoulin
SOMSI: Spherical Novel View Synthesis with Soft Occlusion Multi-Sphere Images
Tewodros Habtegebrial, Christiano Gava, Marcel Rogge, Didier Stricker, Varun Jampani
TubeFormer-DeepLab: Video Mask Transformer
Dahun Kim, Jun Xie, Huiyu Wang, Siyuan Qiao, Qihang Yu, Hong-Seok Kim, Hartwig Adam, In So Kweon, Liang-Chieh Chen
Contextualized Spatio-Temporal Contrastive Learning with Self-Supervision
Liangzhe Yuan, Rui Qian*, Yin Cui, Boqing Gong, Florian Schroff, Ming-Hsuan Yang, Hartwig Adam, Ting Liu
When Does Contrastive Visual Representation Learning Work?
Elijah Cole, Xuan Yang, Kimberly Wilber, Oisin Mac Aodha, Serge Belongie
Less Is More: Generating Grounded Navigation Instructions from Landmarks
Su Wang, Ceslee Montgomery, Jordi Orbay, Vighnesh Birodkar, Aleksandra Faust, Izzeddin Gur, Natasha Jaques, Austin Waters, Jason Baldridge, Peter Anderson
Forecasting Characteristic 3D Poses of Human Actions
Christian Diller, Thomas Funkhouser, Angela Dai
BEHAVE: Dataset and Method for Tracking Human Object Interactions
Bharat Lal Bhatnagar, Xianghui Xie, Ilya A. Petrov, Cristian Sminchisescu, Christian Theobalt, Gerard Pons-Moll
Motion-from-Blur: 3D Shape and Motion Estimation of Motion-Blurred Objects in Videos
Denys Rozumnyi, Martin R. Oswald, Vittorio Ferrari, Marc Pollefeys
End-to-End Generative Pretraining for Multimodal Video Captioning (see blog post)
Paul Hongsuck Seo, Arsha Nagrani, Anurag Arnab, Cordelia Schmid
Uncertainty-Aware Adaptation for Self-Supervised 3D Human Pose Estimation
Jogendra Nath Kundu, Siddharth Seth, Pradyumna YM, Varun Jampani, Anirban Chakraborty, R. Venkatesh Babu
Learning ABCs: Approximate Bijective Correspondence for Isolating Factors of Variation with Weak Supervision
Kieran A. Murphy, Varun Jampani, Srikumar Ramalingam, Ameesh Makadia
HumanNeRF: Free-Viewpoint Rendering of Moving People from Monocular Video
Chung-Yi Weng, Brian Curless, Pratul P. Srinivasan, Jonathan T. Barron, Ira Kemelmacher-Shlizerman
Deblurring via Stochastic Refinement
Jay Whang*, Mauricio Delbracio, Hossein Talebi, Chitwan Saharia, Alexandros G. Dimakis, Peyman Milanfar
NeRF in the Dark: High Dynamic Range View Synthesis from Noisy Raw Images
Ben Mildenhall, Peter Hedman, Ricardo Martin-Brualla, Pratul P. Srinivasan, Jonathan T. Barron
CoNeRF: Controllable Neural Radiance Fields
Kacper Kania, Kwang Moo Yi, Marek Kowalski, Tomasz Trzciński, Andrea Tagliasacchi
A Conservative Approach for Unbiased Learning on Unknown Biases
Myeongho Jeon, Daekyung Kim, Woochul Lee, Myungjoo Kang, Joonseok Lee
DeepFusion: Lidar-Camera Deep Fusion for Multi-Modal 3D Object Detection (see blog post)
Yingwei Li*, Adams Wei Yu, Tianjian Meng, Ben Caine, Jiquan Ngiam, Daiyi Peng, Junyang Shen, Yifeng Lu, Denny Zhou, Quoc V. Le, Alan Yuille, Mingxing Tan
Video Frame Interpolation Transformer
Zhihao Shi, Xiangyu Xu, Xiaohong Liu, Jun Chen, Ming-Hsuan Yang
Global Matching with Overlapping Attention for Optical Flow Estimation
Shiyu Zhao, Long Zhao, Zhixing Zhang, Enyu Zhou, Dimitris Metaxas
LiT: Zero-Shot Transfer with Locked-image Text Tuning (see blog post)
Xiaohua Zhai, Xiao Wang, Basil Mustafa, Andreas Steiner, Daniel Keysers, Alexander Kolesnikov, Lucas Beyer
Are Multimodal Transformers Robust to Missing Modality?
Mengmeng Ma, Jian Ren, Long Zhao, Davide Testuggine, Xi Peng
3D-VField: Adversarial Augmentation of Point Clouds for Domain Generalization in 3D Object Detection
Alexander Lehner, Stefano Gasperini, Alvaro Marcos-Ramiro, Michael Schmidt, Mohammad-Ali Nikouei Mahani, Nassir Navab, Benjamin Busam, Federico Tombari
SHIFT: A Synthetic Driving Dataset for Continuous Multi-Task Domain Adaptation
Tao Sun, Mattia Segu, Janis Postels, Yuxuan Wang, Luc Van Gool, Bernt Schiele, Federico Tombari, Fisher Yu
H4D: Human 4D Modeling by Learning Neural Compositional Representation
Boyan Jiang, Yinda Zhang, Xingkui Wei, Xiangyang Xue, Yanwei Fu
Gravitationally Lensed Black Hole Emission Tomography
Aviad Levis, Pratul P. Srinivasan, Andrew A. Chael, Ren Ng, Katherine L. Bouman
Deep Saliency Prior for Reducing Visual Distraction
Kfir Aberman, Junfeng He, Yossi Gandelsman, Inbar Mosseri, David E. Jacobs, Kai Kohlhoff, Yael Pritch, Michael Rubinstein
The Auto Arborist Dataset: A Large-Scale Benchmark for Multiview Urban Forest Monitoring Under Domain Shift
Sara Beery, Guanhang Wu, Trevor Edwards, Filip Pavetic, Bo Majewski, Shreyasee Mukherjee, Stanley Chan, John Morgan, Vivek Rathod, Jonathan Huang
Workshops
Ethical Considerations in Creative Applications of Computer Vision
Chairs and Advisors: Negar Rostamzadeh, Fernando Diaz, Emily Denton, Mark Diaz, Jason Baldridge
Dynamic Neural Networks Meet Computer Vision Organizers
Invited Speaker: Barret Zoph
Precognition: Seeing Through the Future
Organizer: Utsav Prabhu
Invited Speaker: Sella Nevo
Computer Vision in the Built Environment for the Design, Construction, and Operation of Buildings
Invited Speakers: Thomas Funkhouser, Federico Tombari
Neural Architecture Search: Lightweight NAS Challenge
Invited Speaker: Barret Zoph
Transformers in Vision
Organizer: Lucas Beyer
Invited Speakers and Panelists: Alexander Kolesnikov, Mathilde Caron, Arsha Nagrani, Lucas Beyer
Challenge on Learned Image Compression
Organizers: George Toderici, Johannes Balle, Eirikur Agustsson, Nick Johnston, Fabian Mentzer, Luca Versari
Invited Speaker: Debargha Mukherjee
Embodied AI
Organizers: Anthony Francis, Sören Pirk, Alex Ku, Fei Xia, Peter Anderson
Scientific Advisory Board Members: Alexander Toshev, Jie Tan
Invited Speaker: Carolina Parada
Sight and Sound
Organizers: Arsha Nagrani, William Freeman
New Trends in Image Restoration and Enhancement
Organizers: Ming-Hsuan Yang, Vivek Kwatra, George Toderici
EarthVision: Large Scale Computer Vision for Remote Sensing Imagery
Invited Speaker: John Quinn
LatinX in Computer Vision Research
Organizer: Ruben Villegas
Fine-Grained Visual Categorization
Organizer: Kimberly Wilber
The Art of Robustness: Devil and Angel in Adversarial Machine Learning
Organizer: Florian Tramèr
Invited Speaker: Nicholas Carlini
AI for Content Creation
Organizers: Deqing Sun, Huiwen Chang, Lu Jiang
Invited Speaker: Chitwan Saharia
LOng-form VidEo Understanding
Invited Speaker: Cordelia Schmid
Visual Perception and Learning in an Open World
Invited Speaker: Rahul Sukthankar
Media Forensics
Organizer : Christoph Bregler
Technical Committee Members: Shruti Agarwal, Scott McCloskey, Peng Zhou
Vision Datasets Understanding
Organizer: José Lezama
Embedded Vision
Invited Speaker: Matthias Grundmann
Federated Learning for Computer Vision
Invited Speaker: Zheng Xu
Large Scale Holistic Video Understanding
Organizer: David Ross
Invited Speaker: Anurag Arnab
Learning With Limited Labelled Data for Image and Video Understanding
Invited Speaker: Hugo Larochelle
Bridging the Gap Between Computational Photography and Visual Recognition
Invited Speaker: Xiaohua Zhai
Explainable Artificial Intelligence for Computer Vision
Invited Speaker: Been Kim
Robustness in Sequential Data
Organizers: Sayna Ebrahimi, Kevin Murphy
Invited Speakers: Sayna Ebrahimi, Balaji Lakshminarayanan
Sketch-Oriented Deep Learning
Organizer: David Ha
Invited Speaker: Jonas Jongejan
Multimodal Learning and Applications
Invited Speaker: Cordelia Schmid
Computational Cameras and Displays
Organizer: Tali Dekel
Invited Speaker: Peyman Millanfar
Artificial Social Intelligence
Invited Speaker: Natasha Jaques
VizWiz Grand Challenge: Algorithms to Assist People Who Are Blind
Invited Speaker and Panelist: Andrew Howard
Image Matching: Local Features & Beyond
Organizer: Eduard Trulls
Multi-Agent Behavior: Representation, Modeling, Measurement, and Applications
Organizer: Ting Liu
Efficient Deep Learning for Computer Vision
Organizers: Pete Warden, Andrew Howard, Grace Chu, Jaeyoun Kim
Gaze Estimation and Prediction in the Wild
Organizer: Thabo Beeler
Tutorials
Denoising Diffusion-Based Generative Modeling: Foundations and Applications
Invited Speaker: Ruiqi Gao
Algorithmic Fairness: Why It’s Hard and Why It’s Interesting
Invited Speaker: Sanmi Koyejo
Beyond Convolutional Neural Networks
Invited Speakers: Neil Houlsby, Alexander Kolesnikov, Xiaohua Zhai
Joint Ego4D and Egocentric Perception, Interaction & Computing
Invited Speaker: Vittorio Ferrari
Deep AUC Maximization
Invited Speakers: Tianbao Yang
Vision-Based Robot Learning
Organizers: Michael S. Ryoo, Andy Zeng, Pete Florence
Graph Machine Learning for Visual Computing
Organizers: Federico Tombari
Invited Speakers: Federico Tombari, Fabian Manhardt
*Work done while at Google. ↩
Olga Moskvyak’s journey into the world of science
How she moved across the world to discover a passion for (and a career in) machine learning.Read More
AI in the Big Easy: NVIDIA Research Lets Content Creators Improvise With 3D Objects
Jazz is all about improvisation — and NVIDIA is paying tribute to the genre with AI research that could one day enable graphics creators to improvise with 3D objects created in the time it takes to hold a jam session.
The method, NVIDIA 3D MoMa, could empower architects, designers, concept artists and game developers to quickly import an object into a graphics engine to start working with it, modifying scale, changing the material or experimenting with different lighting effects.
NVIDIA Research showcased this technology in a video celebrating jazz and its birthplace, New Orleans, where the paper behind 3D MoMa will be presented this week at the Conference on Computer Vision and Pattern Recognition.
Extracting 3D Objects From 2D Images
Inverse rendering, a technique to reconstruct a series of still photos into a 3D model of an object or scene, “has long been a holy grail unifying computer vision and computer graphics,” said David Luebke, vice president of graphics research at NVIDIA.
“By formulating every piece of the inverse rendering problem as a GPU-accelerated differentiable component, the NVIDIA 3D MoMa rendering pipeline uses the machinery of modern AI and the raw computational horsepower of NVIDIA GPUs to quickly produce 3D objects that creators can import, edit and extend without limitation in existing tools,” he said.
To be most useful for an artist or engineer, a 3D object should be in a form that can be dropped into widely used tools such as game engines, 3D modelers and film renderers. That form is a triangle mesh with textured materials, the common language used by such 3D tools.
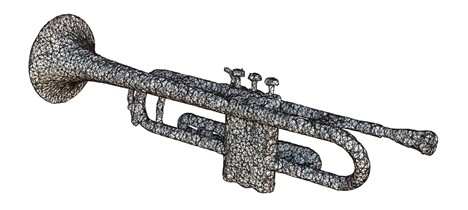
Game studios and other creators would traditionally create 3D objects like these with complex photogrammetry techniques that require significant time and manual effort. Recent work in neural radiance fields can rapidly generate a 3D representation of an object or scene, but not in a triangle mesh format that can be easily edited.
NVIDIA 3D MoMa generates triangle mesh models within an hour on a single NVIDIA Tensor Core GPU. The pipeline’s output is directly compatible with the 3D graphics engines and modeling tools that creators already use.
The pipeline’s reconstruction includes three features: a 3D mesh model, materials and lighting. The mesh is like a papier-mâché model of a 3D shape built from triangles. With it, developers can modify an object to fit their creative vision. Materials are 2D textures overlaid on the 3D meshes like a skin. And NVIDIA 3D MoMa’s estimate of how the scene is lit allows creators to later modify the lighting on the objects.
Tuning Instruments for Virtual Jazz Band
To showcase the capabilities of NVIDIA 3D MoMa, NVIDIA’s research and creative teams started by collecting around 100 images each of five jazz band instruments — a trumpet, trombone, saxophone, drum set and clarinet — from different angles.
NVIDIA 3D MoMa reconstructed these 2D images into 3D representations of each instrument, represented as meshes. The NVIDIA team then took the instruments out of their original scenes and imported them into the NVIDIA Omniverse 3D simulation platform to edit.
In any traditional graphics engine, creators can easily swap out the material of a shape generated by NVIDIA 3D MoMa, as if dressing the mesh in different outfits. The team did this with the trumpet model, for example, instantly converting its original plastic to gold, marble, wood or cork.
Creators can then place the newly edited objects into any virtual scene. The NVIDIA team dropped the instruments into a Cornell box, a classic graphics test for rendering quality. They demonstrated that the virtual instruments react to light just as they would in the physical world, with the shiny brass instruments reflecting brightly, and the matte drum skins absorbing light.
These new objects, generated through inverse rendering, can be used as building blocks for a complex animated scene — showcased in the video’s finale as a virtual jazz band.
The paper behind NVIDIA 3D MoMa will be presented in a session at CVPR on June 22 at 1:30 p.m. Central time. It’s one of 38 papers with NVIDIA authors at the conference. Learn more about NVIDIA Research at CVPR.
The post AI in the Big Easy: NVIDIA Research Lets Content Creators Improvise With 3D Objects appeared first on NVIDIA Blog.
NVIDIA Joins Forum to Help Lay the Foundation of the Metaverse
The metaverse is the next big step in the evolution of the internet — the 3D web — which presents a major opportunity for every industry from entertainment to automotive to manufacturing, robotics and beyond.
That’s why NVIDIA is joining our partners in the Metaverse Standards Forum, an open venue for all interested parties to discuss and debate how best to build the foundations of the metaverse.
From a 2D to a 3D Internet
The early internet of the ’70s and ’80s was accessed purely through text-based interfaces, UNIX shells and consoles. The ’90s introduced the World Wide Web, which made the internet accessible to millions by providing a more natural and intuitive interface with images and text combined into 2D worlds in the form of web pages.
The metaverse that is coming into existence is a 3D spatial overlay of the internet. It continues the trend of making the internet more accessible and more natural for humans by making the interface to the internet indistinguishable from our interface to the real world.
The 3D computer graphics and simulation technologies developed over the past three decades in CAD/CAM, visual effects and video games, combined with the computing power now available, have converged to a point where we can now start building such an interface.
A Place for Both Work and Play
For most people, the term metaverse primarily evokes thoughts of gaming or socializing. They’ll definitely be big, important use cases of the metaverse, but just like with the internet, it won’t be limited to them.
We use the internet for far more than play. Companies and industries run on the internet; it’s part of their essential infrastructure. We believe the same will be true for the emerging metaverse.
For example, retailers are opening virtual shops to sell real and virtual goods. Researchers are using digital twins to design and simulate fusion power plants.
BMW Group is developing a digital twin of an entire factory to more rapidly design and operate efficient and safe factories. NVIDIA is building an AI supercomputer to power a digital twin of the Earth to help researchers study and solve climate change.
A Lesson From the Web
The key to the success of the web from the very start in 1993 was the introduction of a standard and open way of describing a web page — HyperText Markup Language, or HTML. Without HTML’s adoption, we would’ve had disconnected islands on the web, each only linking within themselves.
Fortunately, the creators of the early web and internet understood that open standards — particularly for data formats — were accelerators of growth and a network effect.
The metaverse needs an equivalent to HTML to describe interlinked 3D worlds in glorious detail. Moving between 3D worlds using various tools, viewers and browsers must be seamless and consistent.
The solution is Pixar’s Universal Scene Description (USD) — an open and extensible format, library and composition engine.
USD is one of many of the building blocks we’ll need to build the metaverse. Another is glTF, a 3D transmission format developed within Khronos Group. We see USD and glTF as compatible technologies and hope to see them coevolve as such.
A Constellation of Standards
Neil Trevett, vice president of developer ecosystems at NVIDIA and the president of The Khronos Group, the forum’s host, says the metaverse will require a constellation of standards.
The forum won’t set them, but it’ll be a place where designers and users can learn about and try ones they want to use and identify any that are missing or need to be expanded.
We’re thrilled to see the formation of the Metaverse Standards Forum — a free and open venue where people from every domain can gather to contribute to the exciting new era of the internet: the metaverse!
The post NVIDIA Joins Forum to Help Lay the Foundation of the Metaverse appeared first on NVIDIA Blog.
3D Artist Jae Solina Goes Cyberpunk This Week ‘In the NVIDIA Studio’
Editor’s note: This post is part of our weekly In the NVIDIA Studio series, which celebrates featured artists, offers creative tips and tricks, and demonstrates how NVIDIA Studio technology accelerates creative workflows.
3D artist Jae Solina, who goes by the stage name JSFILMZ, steps In the NVIDIA Studio this week to share his unique 3D creative workflow in the making of Cyberpunk Short Film — a story shrouded in mystery with a tense exchange between two secretive contacts.
As an avid movie buff, JSFILMZ takes inspiration from innovative movie directors Christopher Nolan, David Fincher and Georce Lucas. He admires their abilities to combine technical skill with storytelling heightened by exciting plot twists.
The Cyberpunk Short Film setting displays stunning realism with ray-traced lighting, shadows and reflections — complemented by rich, vibrant colors.
Astonishingly, JSFILMZ created the film in just one day with the NVIDIA Omniverse platform for 3D design collaboration and world simulation, using the Omniverse Machinima app and the Reallusion iClone Connector. He alternated between systems that use an NVIDIA RTX A6000 GPU and a GeForce RTX 3070 Laptop GPU.
The #MadeinMachinima contest ends soon. Omniverse users can build and animate cinematic short stories with Omniverse Machinima for a chance to win RTX-accelerated NVIDIA Studio laptops. Entries are being accepted until Monday, June 27.
An Omniverse Odyssey With Machinima
JSFILMZ’s creative journey starts with scene building in Omniverse Machinima, plugging and moving background objects to create the futuristic cyberpunk diner. His RTX GPUs power Omniverse’s built-in RTX renderer to achieve fast, interactive movement within the viewport while preserving photorealistic detail. The reduction of distracting denoising allows JSFILMZ to focus on creating without having to wait for his scenes to render.
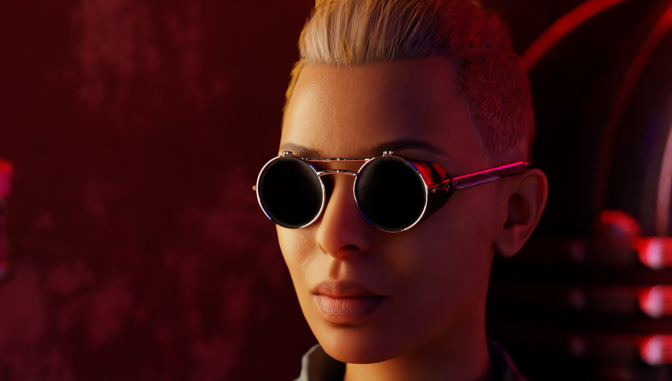
Pulling assets from the NVIDIA MDL material library, JSFILMZ achieved peak realism with every surface, material and texture.
The artist then populated the scene with human character models downloaded from the Reallusion content store.
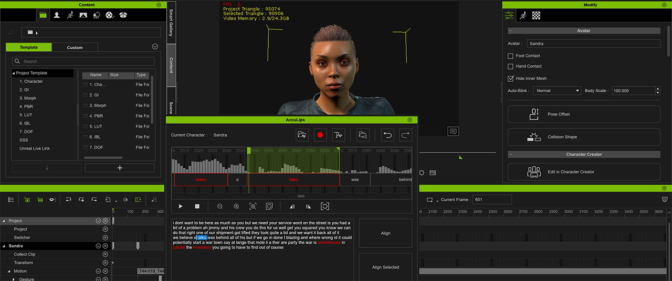
Vocal animation was generated in the Reallusion iClone Connector using the AccuLips feature. It simulates human speech behavior with each mouth shape, naturally taking on the qualities of those that precede or follow them. JSFILMZ simply uploads voiceover files from his actors, and the animations are automatically generated.
To capture animations while sitting, JSFILMZ turned to an Xsens Awinda starter body-motion-capture suit, acting out movements for both characters. Using the Xsens software, he processed, cleaned up and exported the visual effects data.
JSFILMZ integrated unique walking animations for each character by searching and selecting the perfect animation sequences in the Reallusion actorcore store. He returned to the iClone Connector to import and apply separate motion captures to the characters, completing animations for the scene.
The last 3D step was to adjust lighting. For tips on how to light in Omniverse, check out JSFILMZ’s live-streamed tutorial, which offers Omniverse know-how and his lighting technique.
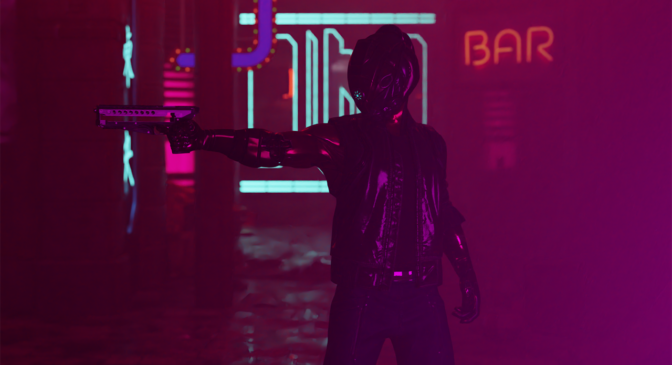
According to JSFILMZ, adding and manipulating lights revealed another advantage of using Machinima: the ability to conveniently switch between real-time ray-traced mode for more fluid movement in the viewport and the interactive path-traced mode for the most accurate, detailed view.
He then exported final renders with ray tracing using the Omniverse RTX Renderer, which is powered by NVIDIA RTX or GeForce RTX GPUs.
Working with multiple 3D applications connected by Omniverse saved JSFILMZ countless hours of rendering, downloading files, converting file types, reuploading and more. “It’s so crazy that I can do all this, all at home,” he said.
Completing Cyberpunk Short Film required editing and color correction in DaVinci Resolve.
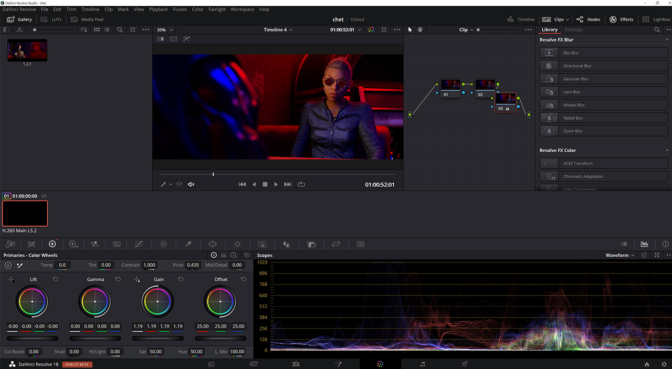
Color grading, video editing and color scope features deployed by JSFILMZ are all accelerated with his GPU, allowing for quick edits. And the NVIDIA hardware encoder and decoder makes the GPU-accelerated export very fast.
And with that, Cyberpunk Short Film was ready for viewing.
3D artists can benefit from JSFILMZ’s NVIDIA Omniverse tutorial YouTube playlist. It’s an extensive overview of the Omniverse platform for creators, covering the basics from installation and set up to in-app features such as lighting, rendering and animating.
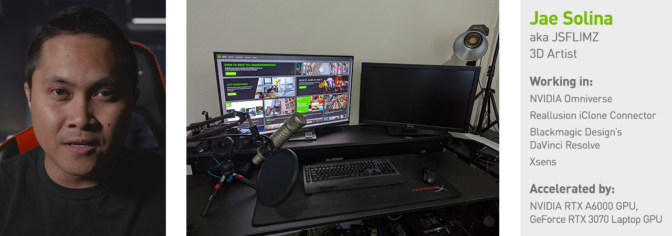
JSFILMZ teaches 3D creative workflows specializing in NVIDIA Omniverse and Unreal Engine 5 on his YouTube channel and via Udemy courses.
Learn more about NVIDIA Omniverse, including tips, tricks and more on the Omniverse YouTube channel. For additional support, explore the Omniverse forums or join the Discord server to chat with the community. Check out the Omniverse Twitter, Instagram and Medium page to stay up to date.
Follow NVIDIA Studio on Instagram, Twitter and Facebook. Access tutorials on the Studio YouTube channel and get updates directly in your inbox by subscribing to the NVIDIA Studio newsletter.
The post 3D Artist Jae Solina Goes Cyberpunk This Week ‘In the NVIDIA Studio’ appeared first on NVIDIA Blog.
NVIDIA Accelerates Open Data Center Innovation
NVIDIA today became a founding member of the Linux Foundation’s Open Programmable Infrastructure (OPI) project, while making its NVIDIA DOCA networking software APIs widely available to foster innovation in the data center.
Businesses are embracing open data centers, which require applications and services that are easily integrated with other solutions for simplified, lower-cost and sustainable management. Moving to open NVIDIA DOCA will help develop and nurture broad and vibrant DPU ecosystems and power unprecedented data center transformation.
The OPI project aims to create a community-driven, standards-based, open ecosystem for accelerating networking and other data center infrastructure tasks using DPUs.
DOCA includes drivers, libraries, services, documentation, sample applications and management tools to speed up and simplify the development and performance of applications. It allows for flexibility and portability for BlueField applications written using accelerated drivers or low-level libraries, such as DPDK, SPDK, Open vSwitch or Open SSL. We plan to continue this support. As part of OPI, developers will be able to create a common programming layer to support many of these open drivers and libraries with DPU acceleration.
DOCA library APIs are already publicly available and documented for developers. Open licensing of these APIs will ensure that applications developed using DOCA will support BlueField DPUs as well as those from other providers.
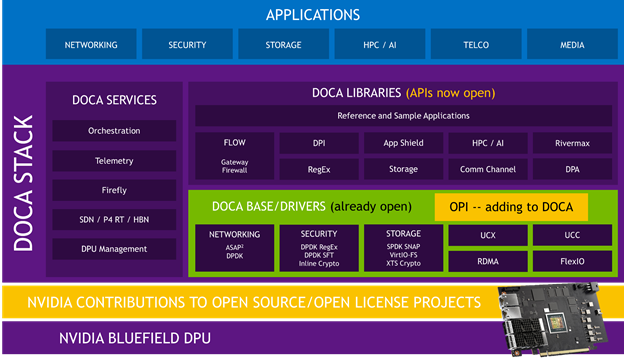
Expanding Use of DPUs
AI, containers and composable infrastructure are increasingly important for enterprise and cloud data centers. This is driving the use of DPUs in servers to support software-defined, hardware-accelerated networking, east-west traffic and zero-trust security.
Only the widespread deployment of DPUs such as NVIDIA BlueField can support the ability to offload, accelerate and isolate data center workloads, including networking, storage, security and DevOps management.
NVIDIA’s history of open innovation over the decades includes engaging with leading consortiums, participating in standards committees and contributing to a range of open source software and communities.
We contribute frequently to open source and open-license projects and software such as the Linux kernel, DPDK, SPDK, NVMe over Fabrics, FreeBSD, Apache Spark, Free Range Routing, SONiC, Open Compute Project and other areas covering networking, virtualization, containers, AI, data science and data encryption.
NVIDIA is often among the top three code contributors to many releases of Linux and DPDK. And we’ve historically included an open source version of our networking drivers in the Linux kernel.
With OPI, customers, ISVs, infrastructure appliance vendors and systems integrators will be able to create applications for BlueField DPUs using DOCA to gain the best possible performance and easiest developer experience for accelerated data center infrastructure.
The post NVIDIA Accelerates Open Data Center Innovation appeared first on NVIDIA Blog.
Build an appointment scheduler interface integrated with Meta using Amazon Lex and Amazon Connect
This blog post is co-written with Nick Vargas and Anna Schreiber from Accenture.
Scheduling customer appointments is often a manual and labor-intensive process. You can utilize advances in self-service technology to automate appointment scheduling.
In this blog post, we show you how to build a self-service appointment scheduling solution built with Amazon Lex and Amazon Connect. This solution allows users to create appointments via Meta Messenger, and receive appointment confirmations through an SMS mobile message. It also provides a web-based dashboard so you can provide call to users with single-click button at the scheduled time.
Amazon Lex integrates with Meta messenger and can be used to enable chat conversations. Lex is a fully-managed artificial intelligence (AI) service with Natural language understanding (NLU) to design, build, test, and deploy conversational interfaces in applications.
Solution overview
The architecture diagram below shows a high-level overview of the interaction between different AWS components and services. The solution consists of these primary components: customer interaction using Meta messenger, appointment scheduling via SMS enabled by Lex and a customer outbound dialer from Connect. This outbound dialer makes it easy to create an outbound call to the customer from a simple UI interface.
This post uses the following sample bot conversation:
User: I would like to book an appointment.
Agent: What appointment can I get you? You can say Billing, General or Offers.
User: Billing
Agent: What’s your first name?
User: Sameer
Agent: What is your phone number with country code?
User: +10001234567
Agent: When should I schedule your Billing appointment?
User: Next week Tuesday
Agent: At what time should I schedule the Billing appointment?
User: 9:00 am
Agent: Sameer, 09:00 is available, should I go ahead and book your appointment?
User: Yes
Agent: Thanks Sameer, your appointment is confirmed for 09:00, and we have texted the details to your phone number.
For the scheduler and customer notification component, an AWS Lambda handler is used to process the scheduling request. The appointment information is then saved to a Amazon DynamoDB database. When the information is saved successfully, a notification is sent to the customer confirming the appointment details via SMS using Amazon Pinpoint.
A React.js application is created to display the saved customer appointments from the database in a calendar view format. This makes it easy for employees to identify the customers who need to be called. A call button from the calendar entry is clicked to initiate the call. This will immediately place an outbound call request to connect the customer with the employee using Amazon Connect.
Prerequisites
For this project, you should have the following prerequisites:
- Downloaded the code files from the GitHub repository.
The repository contains:- The React app files, located under the UI
- The Amazon Connect Contact Flows, located under backend/connect/contact_flows There are four contact flows for this demo with files names
AgentWhisper
,CustomerWaiting
,InboundCall
andOutboundCall
. - A zip file for an Amazon Lex Bot, located in backend/lex directory with file name AppointmentSchedulerBot.zip.
- npm installed on your local machine. Refer how to install node.js and npm on your machine,
The deployment of this solution is automated where possible using CloudFormation, however, some configurations and steps in the deployment are manual.
Deploy the solution
To set up the required infrastructure for the appointment scheduler demo app in your AWS account, complete the following steps:
- Sign in to the AWS Management Console.
- Choose Launch Stack:
- On the Create Stack page, under Specify template, choose Upload a template file.
- Choose the
AppointmentsSchedulerCFTemplate
file that you downloaded from GitHub. - Choose Next.
- For Stack name, enter a unique name for the stack, such as
AppointmentSchedulerDemo
.
- Choose Next, and then choose Next on the Configure stack options page.
- On the Review page, select I acknowledge that AWS CloudFormation might create IAM resources and choose Create.
The stack generates the following resources:
-
- The DynamoDB table
AppointmentSchedulerTable
- The Amazon Pinpoint app
AppointmentSchedulerPinpointApp
- Two AWS Identity and Access Management (IAM) policies:
AppointmentSchedulerPinpointPolicy
AppointmentSchedulerDynamoApiPolicy
- Two IAM roles:
AppointmentsLambdaRole
OutboundContactLambdaRole
- Two Lambda functions:
AppointmentScheduler
AppointmentSchedulerOutboundContact
- The Amazon API Gateway instance Appointments
- Amazon CloudFront distribution
- The Amazon Simple Storage Service (Amazon S3) bucket
appointment-scheduler-website
- The DynamoDB table
Configure the Amazon Pinpoint app
To configure the Amazon Pinpoint app, complete the following steps:
- Go to the Pinpoint console.
- Navigate to the AppointmentSchedulerPinpointApp deployed in above.
- On the left menu under Settings click SMS and Voice.
- Under Number settings click Request Phone Number.
- Select your country of origin, choose Toll-free, and click Next, then Request.
The Amazon Lex bot for this post has one intent, MakeAppointment
, which asks the user the series of questions in the preceding example to elicit the appointment type, date, time, name, and phone number of the customer.
AppointmentTypeValue
is the only custom slot type for this bot and takes one of three values: Billing, General, or Offers. The Name, Phone, Date, and Time slots each use the built-in slot type provided by Amazon Lex.
Deploy the Amazon Lex bot
To deploy the bot, first import the Amazon Lex bot (AppointmentSchedulerLex.zip
) into your account.
- Sign in to the Amazon Lex V2 console.
- If this is your first time using Amazon Lex, you will be shown the Welcome page, choose Create Bot.
- When presented with the Create your bot page, scroll down to the bottom of the page, and select Cancel. If this is not your first-time using Amazon Lex, skip this step.
- Choose Actions, then Import.
- Enter AppointmentSchedulerBot for the bot’s name then choose the .zip archive to import.
- Under IAM permissions, choose Create a role with basic Amazon Lex permissions.
- Under COPPA, choose No.
- Click Import.
- Open the bot by clicking on the bot’s name.
- Under Deployment on the left menu, click Aliases, select TestBotAlias and click English (US) under Languages. Choose the
AppointmentScheduler
Lambda function and click Save.
- Under Bot Versions on the left menu, select Intents and at the bottom right-hand side of the page, click Build.
- [Optional] Once the build has completed, click Test to test the bot using the window that appears on the right (click on the microphone icon to speak to your bot or type in the text box).
Set up an Amazon Connect Instance
To set up your Amazon Connect instance and contact flows, you complete the following steps:
- Set up an Amazon Connect instance.
- Go to the Amazon Connect console.
- If this is the first time you have been to the Amazon Connect console, you will see the Welcome page, choose Get Started.
- If this is not the first time you are using Amazon Connect, click Add an instance.
- For Identity management, select Store users in Amazon Connect.
- For Access URL, type a unique name for your instance, for example,
AppointmentSchedulerDemo
, then choose Next. - On the Add administrator page, add a new administrator account for Amazon Connect. Use this account to log in to your instance later using the unique access URL. Click Next step.
- On the next two pages – Telephony Options and Data storage – accept the default settings and choose Next step.
- On the Review and Create page, choose Create instance.
- Add the Amazon Lex bots to your newly created Amazon Connect instance.
- Log in to the instance and claim a phone number
- Click on the Login URL for your Connect Instance.
- Enter the Administrator credentials you entered upon creation of the instance. This will open the Connect Console.
- From the Dashboard, under Explore your channels of communication select View phone numbers on the right.
- Click Claim a number.
- Choose a Country and leave the default type of DID (Direct Inward Dialing), choose a Phone Number from the dropdown list, and click Next.
- Click Save.
- Add the
OutboundQueue
- From the navigation menu on the left, choose Queues from the Routing menu.
- Click Add New Queue.
- Name the Queue
OutboundQueue
, use the dropdown to set the Hours of operation to Basic Hours and use the dropdown for Outbound caller ID number to select the phone number you claimed earlier.
- Click Add new queue.
- From the navigation menu on the left, choose Routing Profiles from the Users menu.
- Click Basic Routing Profile. Under Routing profile queues, add OutboundQueue and click Save.
- Add the phone number to
BasicQueue
- From the navigation menu on the left, choose Queues from the Routing menu.
- Click on
BasicQueue
. - In the Outbound caller ID number field, add the phone number that you claimed earlier.
- Click Save on the top right corner.
- Import the
InboundCall
contact flow - Then, associate this flow with the phone number.
- Import the
AgentWhisper
,CustomerWaiting
, andOutboundCall
contact flows- From the left navigation menu, choose Contact Flows under Routing.
- Click Create Agent Whisper flow.
- On the right-hand side of the page, click on the down arrow and click Import flow (beta).
- Find the AgentWhisper file and choose Import.
- Click Publish.
- Navigate back to the Contact Flows list and click the down arrow next to Create contact flow.
- Click Create Customer Queue Flow.
- On the right-hand side of the page, click on the down arrow and click Import flow (beta).
- Find the
CustomerWaiting
file and choose Import. - Click Publish.
- Navigate back to the Contact Flows list and click the down arrow next to Create contact flow.
- Choose Create contact flow.
- On the right-hand side of the page, click on the down arrow and click Import flow (beta).
- Find the
OutboundCall
file from the GitHub repository you downloaded earlier and choose Import. - Click Publish.
Edit Lambda Functions:
- Go to the Lambda console.
- Click on the
AppointmentScheduler
function. - Click on Configuration and Environment Variables from the left menu.
- Click Edit. Replace the Value with your Pinpoint Project ID and Toll-free number. Click Save.
- Return to the Lambda console and click on the
AppointmentSchedulerOutboundContact
function. - Repeat step 3 and 4, replacing the values for
CONTACT_FLOW
,INSTANCE_ID
andQUEUE_ID
with the correct values. Click Save once done.- To find the contact flow ID, navigate to the
OutboundCall
Contact Flow in the Amazon Connect Console and click on the arrow next to Show additional flow information. The contact flow ID is the last value after contact-flow/.
- To find the instance ID, navigate to the Amazon Connect Console and click on your instance Alias. The instance ID is the last value in the Instance ARN after instance/.
- To find the queue ID, navigate to the
OutboundQueue
in the Amazon Connect Console and click on the arrow next to Show additional queue information. The contact flow ID is the last value after queue/.
- To find the contact flow ID, navigate to the
The Lex Bots and Amazon Connect Instance are now ready to go. Next, we will deploy the UI.
Edit API Gateway route:
- Go to the API Gateway console
- Click the instance named Appointments
- Under the resources section, click the POST method belonging to the /outcall resource.
- Click Integration Request.
- Then click the edit icon next to the right of the Lambda Function field. Then click the checkmark icon that have appeared to the right of the text field.
- Click OK to add a permission to the Lambda function.
Deploy the UI:
- Configure the UI before deployment
- In your preferred code editor, open the ui folder from the downloaded code files.
- Replace <your-api-ID> and <region> with your API ID (accessible under the ID column of the API Gateway Console) and the region of your deployed resources in the following lines: 103, 168, 310, 397, 438, 453.
- Replace <your-instance-name> with your Amazon Connect instance name on line 172 and 402.
- [Optional] add an app logo in the index.js file, line 331:
In the index.html file, line 5:
- In a terminal, navigate to the ui folder of the downloaded project.
- Run npm install. This will take a few minutes to complete.
- Run npm run-script build. This will generate a build folder in the ui directory.
- Add the code files to the S3 bucket:
- Go to the S3 Console.
- Search for the bucket deployed with the CloudFormation Stack, appointment-scheduler-website-<random_id>.
- Drag and drop the contents of the build folder in the ui directory created in the last step into the bucket.
- Click Upload.
You should now be able to access the application from the CloudFront Distribution.
- Add the CloudFront Distribution as an approved origin.
-
- Go to the Amazon Connect console.
- Select the Instance Alias of the instance to which to add the bot.
- Choose Approved origins.
- Click + Add origin and enter the URL of your CloudFront Distribution.
- Click Add.
-
- Now navigate to your CloudFront Distribution URL plus index.html. (e.g.,
https:// <DistributionDomainName>.cloudfront.net/index.html
)
Clean up
One finished with this solution, make sure to clean up your AWS environment as to not incur unwanted charges.
- Go to the S3 console, empty your bucket created by the CloudFormation template (appointment-scheduler-website).
- Go to the CloudFormation console, delete your stack. Ensure that all resources associated with this stack were deleted successfully.
- Go to the Amazon Connect console, delete your instance.
- Go to the Amazon Lex console, delete the bot you created.
Conclusion
For this blog, Accenture and AWS collaborated to develop a machine learning solution that highlights the use of AWS services to build an automated appointment scheduler. This solution demonstrates how easy it is to build an appointment scheduling solution in AWS. Amazon Lex’s ability to support third-party messaging services such as Meta messenger extends the potential reach of the solution across multiple channels. Customer notification via SMS is implemented with minimal effort using Amazon Pinpoint. With Amazon Connect, an outbound dialer is seamlessly integrated with the calendar view web application enabling employees to immediately connect to customers with a simple click-to-call button.
You can accelerate innovation with the Accenture AWS Business Group (AABG). You can learn from the resources, technical expertise, and industry knowledge of two leading innovators, helping you accelerate the pace of innovation to deliver disruptive products and services. The AABG helps customers ideate and innovate cloud solutions for customers through rapid prototype development. Connect with our team a accentureaws@amazon.com to learn and accelerate how to use machine learning in your products and services.
About the Authors
Sameer Goel is a Sr. Solutions Architect in the Netherlands, who drives customer success by building prototypes on cutting-edge initiatives. Prior to joining AWS, Sameer graduated with a master’s degree from Boston, with a concentration in data science. He enjoys building and experimenting with AI/ML projects on Raspberry Pi.
Nick Vargas is a Manager and Technology Architect at Accenture. He leads the project delivery for a rapid prototyping team within the Accenture AWS Business Group (AABG). He enjoys his morning walks with his dog Bingo, traveling, going to the beach, and hiking.
Anna Schreiber is part of a prototyping team within Accenture’s AWS Business Group (AABG). As a Senior AWS Developer, she has worked on several high-profile proof of concepts that help bring the client’s vision to life. When not working, she enjoys cooking, crafting, and playing fetch with her corgi Gimli.