Despite significant effort, current AI systems pale in their understanding of intuitive physics, in comparison to even very young children. In the present work, we address this AI problem, specifically by drawing on the field of developmental psychology.Read More
Why do Policy Gradient Methods work so well in Cooperative MARL? Evidence from Policy Representation
In cooperative multi-agent reinforcement learning (MARL), due to its on-policy nature, policy gradient (PG) methods are typically believed to be less sample efficient than value decomposition (VD) methods, which are off-policy. However, some recent empirical studies demonstrate that with proper input representation and hyper-parameter tuning, multi-agent PG can achieve surprisingly strong performance compared to off-policy VD methods.
Why could PG methods work so well? In this post, we will present concrete analysis to show that in certain scenarios, e.g., environments with a highly multi-modal reward landscape, VD can be problematic and lead to undesired outcomes. By contrast, PG methods with individual policies can converge to an optimal policy in these cases. In addition, PG methods with auto-regressive (AR) policies can learn multi-modal policies.
Figure 1: different policy representation for the 4-player permutation game.
Why do Policy Gradient Methods work so well in Cooperative MARL? Evidence from Policy Representation
In cooperative multi-agent reinforcement learning (MARL), due to its on-policy nature, policy gradient (PG) methods are typically believed to be less sample efficient than value decomposition (VD) methods, which are off-policy. However, some recent empirical studies demonstrate that with proper input representation and hyper-parameter tuning, multi-agent PG can achieve surprisingly strong performance compared to off-policy VD methods.
Why could PG methods work so well? In this post, we will present concrete analysis to show that in certain scenarios, e.g., environments with a highly multi-modal reward landscape, VD can be problematic and lead to undesired outcomes. By contrast, PG methods with individual policies can converge to an optimal policy in these cases. In addition, PG methods with auto-regressive (AR) policies can learn multi-modal policies.
Figure 1: different policy representation for the 4-player permutation game.
Deep Hierarchical Planning from Pixels
Research into how artificial agents can make decisions has evolved rapidly through advances in deep reinforcement learning. Compared to generative ML models like GPT-3 and Imagen, artificial agents can directly influence their environment through actions, such as moving a robot arm based on camera inputs or clicking a button in a web browser. While artificial agents have the potential to be increasingly helpful to people, current methods are held back by the need to receive detailed feedback in the form of frequently provided rewards to learn successful strategies. For example, despite large computational budgets, even powerful programs such as AlphaGo are limited to a few hundred moves until receiving their next reward.
In contrast, complex tasks like making a meal require decision making at all levels, from planning the menu, navigating to the store to pick up groceries, and following the recipe in the kitchen to properly executing the fine motor skills needed at each step along the way based on high-dimensional sensory inputs. Hierarchical reinforcement learning (HRL) promises to automatically break down such complex tasks into manageable subgoals, enabling artificial agents to solve tasks more autonomously from fewer rewards, also known as sparse rewards. However, research progress on HRL has proven to be challenging; current methods rely on manually specified goal spaces or subtasks, and no general solution exists.
To spur progress on this research challenge and in collaboration with the University of California, Berkeley, we present the Director agent, which learns practical, general, and interpretable hierarchical behaviors from raw pixels. Director trains a manager policy to propose subgoals within the latent space of a learned world model and trains a worker policy to achieve these goals. Despite operating on latent representations, we can decode Director’s internal subgoals into images to inspect and interpret its decisions. We evaluate Director across several benchmarks, showing that it learns diverse hierarchical strategies and enables solving tasks with very sparse rewards where previous approaches fail, such as exploring 3D mazes with quadruped robots directly from first-person pixel inputs.
How Director Works
Director learns a world model from pixels that enables efficient planning in a latent space. The world model maps images to model states and then predicts future model states given potential actions. From predicted trajectories of model states, Director optimizes two policies: The manager chooses a new goal every fixed number of steps, and the worker learns to achieve the goals through low-level actions. However, choosing goals directly in the high-dimensional continuous representation space of the world model would be a challenging control problem for the manager. Instead, we learn a goal autoencoder to compress the model states into smaller discrete codes. The manager then selects discrete codes and the goal autoencoder turns them into model states before passing them as goals to the worker.
All components of Director are optimized concurrently, so the manager learns to select goals that are achievable by the worker. The manager learns to select goals to maximize both the task reward and an exploration bonus, leading the agent to explore and steer towards remote parts of the environment. We found that preferring model states where the goal autoencoder incurs high prediction error is a simple and effective exploration bonus. Unlike prior methods, such as Feudal Networks, our worker receives no task reward and learns purely from maximizing the feature space similarity between the current model state and the goal. This means the worker has no knowledge of the task and instead concentrates all its capacity on achieving goals.
Benchmark Results
Whereas prior work in HRL often resorted to custom evaluation protocols — such as assuming diverse practice goals, access to the agents’ global position on a 2D map, or ground-truth distance rewards — Director operates in the end-to-end RL setting. To test the ability to explore and solve long-horizon tasks, we propose the challenging Egocentric Ant Maze benchmark. This challenging suite of tasks requires finding and reaching goals in 3D mazes by controlling the joints of a quadruped robot, given only proprioceptive and first-person camera inputs. The sparse reward is given when the robot reaches the goal, so the agents have to autonomously explore in the absence of task rewards throughout most of their learning.
![]() |
The Egocentric Ant Maze benchmark measures the ability of agents to explore in a temporally-abstract manner to find the sparse reward at the end of the maze. |
We evaluate Director against two state-of-the-art algorithms that are also based on world models: Plan2Explore, which maximizes both task reward and an exploration bonus based on ensemble disagreement, and Dreamer, which simply maximizes the task reward. Both baselines learn non-hierarchical policies from imagined trajectories of the world model. We find that Plan2Explore results in noisy movements that flip the robot onto its back, preventing it from reaching the goal. Dreamer reaches the goal in the smallest maze but fails to explore the larger mazes. In these larger mazes, Director is the only method to find and reliably reach the goal.
To study the ability of agents to discover very sparse rewards in isolation and separately from the challenge of representation learning of 3D environments, we propose the Visual Pin Pad suite. In these tasks, the agent controls a black square, moving it around to step on differently colored pads. At the bottom of the screen, the history of previously activated pads is shown, removing the need for long-term memory. The task is to discover the correct sequence for activating all the pads, at which point the agent receives the sparse reward. Again, Director outperforms previous methods by a large margin.
![]() |
The Visual Pin Pad benchmark allows researchers to evaluate agents under very sparse rewards and without confounding challenges such as perceiving 3D scenes or long-term memory. |
In addition to solving tasks with sparse rewards, we study Director’s performance on a wide range of tasks common in the literature that typically require no long-term exploration. Our experiment includes 12 tasks that cover Atari games, Control Suite tasks, DMLab maze environments, and the research platform Crafter. We find that Director succeeds across all these tasks with the same hyperparameters, demonstrating the robustness of the hierarchy learning process. Additionally, providing the task reward to the worker enables Director to learn precise movements for the task, fully matching or exceeding the performance of the state-of-the-art Dreamer algorithm.
![]() |
Director solves a wide range of standard tasks with dense rewards with the same hyperparameters, demonstrating the robustness of the hierarchy learning process. |
Goal Visualizations
While Director uses latent model states as goals, the learned world model allows us to decode these goals into images for human interpretation. We visualize the internal goals of Director for multiple environments to gain insights into its decision making and find that Director learns diverse strategies for breaking down long-horizon tasks. For example, on the Walker and Humanoid tasks, the manager requests a forward leaning pose and shifting floor patterns, with the worker filling in the details of how the legs need to move. In the Egocentric Ant Maze, the manager steers the ant robot by requesting a sequence of different wall colors. In the 2D research platform Crafter, the manager requests resource collection and tools via the inventory display at the bottom of the screen, and in DMLab mazes, the manager encourages the worker via the teleport animation that occurs right after collecting the desired object.
![]() |
![]() |
Left: In Egocentric Ant Maze XL, the manager directs the worker through the maze by targeting walls of different colors. Right: In Visual Pin Pad Six, the manager specifies subgoals via the history display at the bottom and by highlighting different pads. |
![]() |
![]() |
Left: In Walker, the manager requests a forward leaning pose with both feet off the ground and a shifting floor pattern, with the worker filling in the details of leg movement. Right: In the challenging Humanoid task, Director learns to stand up and walk reliably from pixels and without early episode terminations. |
![]() |
![]() |
Left: In Crafter, the manager requests resource collection via the inventory display at the bottom of the screen. Right: In DMLab Goals Small, the manager requests the teleport animation that occurs when receiving a reward as a way to communicate the task to the worker. |
Future Directions
We see Director as a step forward in HRL research and are preparing its code to be released in the future. Director is a practical, interpretable, and generally applicable algorithm that provides an effective starting point for the future development of hierarchical artificial agents by the research community, such as allowing goals to only correspond to subsets of the full representation vectors, dynamically learning the duration of the goals, and building hierarchical agents with three or more levels of temporal abstraction. We are optimistic that future algorithmic advances in HRL will unlock new levels of performance and autonomy of intelligent agents.
NAACL: Industry track offers reality checks, new directions
Industry track chair and Amazon principal research scientist Rashmi Gangadharaiah on trends in industry papers and the challenges of building practical dialogue systems.Read More
No Fueling Around: Designers Collaborate in Extended Reality on Porsche Electric Race Car
A one-of-a-kind electric race car revved to life before it was manufactured — or even prototyped — thanks to GPU-powered extended reality technology.
At the Automotive Innovation Forum in May, NVIDIA worked with Autodesk VRED to showcase a photorealistic Porsche electric sports car in augmented reality, with multiple attendees collaborating in the same immersive environment.
The demo delivered a life-size digital twin of the Porsche Mission R in AR and VR, which are collectively known as extended reality, or XR. Using NVIDIA CloudXR, Varjo XR-3 headsets and Lenovo Android tablets, audiences saw the virtual Porsche with photorealistic lighting and shadows.
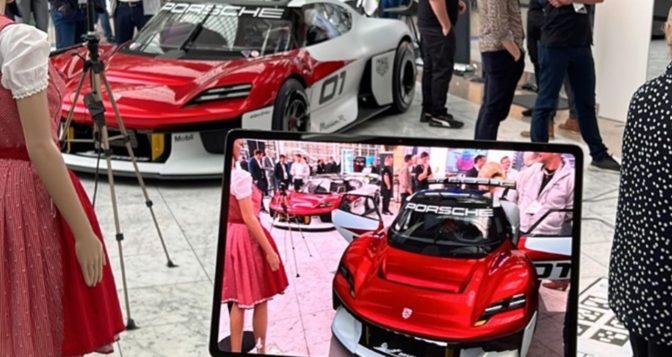
Audiences could view the virtual race car side by side with a physical car on site. With this direct comparison, they witnessed the photorealistic nature of the AR model — from the color of the metals, to the surface of the tires, to the environmental lighting.
The stunning demo, which was shown through an Autodesk VRED collaborative session, ran on NVIDIA RTX-based virtual workstations.
There were two ways to view the demo. First, NVIDIA CloudXR streamed the experience to the tablets from a virtualized NVIDIA Project Aurora server, which was powered by NVIDIA A40 GPUs on a Lenovo ThinkStation SR670 Server. Attendees could also use Varjo headsets, which were locally tethered to NVIDIA RTX A6000 GPUs running on a Lenovo ThinkStation P620 workstation.
Powerful XR Technologies Behind the Streams
Up to five users at a time entered the scene, with two users wearing headsets to see the Porsche car in mixed reality, and three users on tablets to view the car in AR. Users were represented as avatars in the session.
With NVIDIA CloudXR, the forum attendees remotely streamed the photorealistic Porsche model. Built on NVIDIA RTX technology, CloudXR extends NVIDIA RTX Virtual Workstation software, which enables users to stream fully accelerated immersive graphics from a virtualized environment.
This demo used a virtualized Lenovo ThinkStation SR670 server to power NVIDIA’s Project Aurora — a software and hardware platform for XR streaming at the edge. Project Aurora delivers the horsepower of NVIDIA RTX A40 GPUs, so users could experience the rich, real-time graphics of the Porsche model from a machine room over a private 5G network.
Through server-based streaming with Project Aurora, multiple users from different locations were brought together to experience the demo in a single immersive environment. With the help of U.K.-based integrator The Grid Factory, Project Aurora is now available to be deployed in any enterprise.
Learn more about advanced XR streaming with NVIDIA CloudXR.
The post No Fueling Around: Designers Collaborate in Extended Reality on Porsche Electric Race Car appeared first on NVIDIA Blog.
Improving “entity linking” between texts and knowledge bases
New model sets new standard in accuracy while enabling 60-fold speedups.Read More
Onboard PaddleOCR with Amazon SageMaker Projects for MLOps to perform optical character recognition on identity documents
Optical character recognition (OCR) is the task of converting printed or handwritten text into machine-encoded text. OCR has been widely used in various scenarios, such as document electronization and identity authentication. Because OCR can greatly reduce the manual effort to register key information and serve as an entry step for understanding large volumes of documents, an accurate OCR system plays a crucial role in the era of digital transformation.
The open-source community and researchers are concentrating on how to improve OCR accuracy, ease of use, integration with pre-trained models, extension, and flexibility. Among many proposed frameworks, PaddleOCR has gained increasing attention recently. The proposed framework concentrates on obtaining high accuracy while balancing computational efficiency. In addition, the pre-trained models for Chinese and English make it popular in the Chinese language-based market. See the PaddleOCR GitHub repo for more details.
At AWS, we have also proposed integrated AI services that are ready to use with no machine learning (ML) expertise. To extract text and structured data such as tables and forms from documents, you can use Amazon Textract. It uses ML techniques to read and process any type of document, accurately extracting text, handwriting, tables, and other data with no manual effort.
For the data scientists who want the flexibility to use an open-source framework to develop your own OCR model, we also offer the fully managed ML service Amazon SageMaker. SageMaker enables you to implement MLOps best practices throughout the ML lifecycle, and provides templates and toolsets to reduce the undifferentiated heavy lifting to put ML projects in production.
In this post, we concentrate on developing customized models within the PaddleOCR framework on SageMaker. We walk through the ML development lifecycle to illustrate how SageMaker can help you build and train a model, and eventually deploy the model as a web service. Although we illustrate this solution with PaddleOCR, the general guidance is true for arbitrary frameworks to be used on SageMaker. To accompany this post, we also provide sample code in the GitHub repository.
PaddleOCR framework
As a widely adopted OCR framework, PaddleOCR contains rich text detection, text recognition, and end-to-end algorithms. It chooses Differentiable Binarization (DB) and Convolutional Recurrent Neural Network (CRNN) as the basic detection and recognition models, and proposes a series of models, named PP-OCR, for industrial applications after a series of optimization strategies.
The PP-OCR model is aimed at general scenarios and forms a model library of different languages. It consists of three parts: text detection, box detection and rectification, and text recognition, illustrated in the following figure on the PaddleOCR official GitHub repository. You can also refer to the research paper PP-OCR: A Practical Ultra Lightweight OCR System for more information.
To be more specific, PaddleOCR consists of three consecutive tasks:
- Text detection – The purpose of text detection is to locate the text area in the image. Such tasks can be based on a simple segmentation network.
- Box detection and rectification – Each text box needs to be transformed into a horizontal rectangle box for subsequent text recognition. To do this, PaddleOCR proposes to train a text direction classifier (image classification task) to determine the text direction.
- Text recognition – After the text box is detected, the text recognizer model performs inference on each text box and outputs the results according to text box location. PaddleOCR adopts the widely used method CRNN.
PaddleOCR provides high-quality pre-trained models that are comparable to commercial effects. You can either use the pre-trained model for a detection model, direction classifier, or recognition model, or you can fine tune and retrain each individual model to serve your use case. To increase the efficiency and effectiveness of detecting Traditional Chinese and English, we illustrate how to fine-tune the text recognition model. The pre-trained model we choose is ch_ppocr_mobile_v2.0_rec_train, which is a lightweight model, supporting Chinese, English, and number recognition. The following is an example inference result using a Hong Kong identity card.
In the following sections, we walk through how to fine-tune the pre-trained model using SageMaker.
MLOps best practices with SageMaker
SageMaker is a fully managed ML service. With SageMaker, data scientists and developers can quickly and easily build and train ML models, and then directly deploy them into a production-ready managed environment.
Many data scientists use SageMaker for accelerating the ML lifecycle. In this section, we illustrate how SageMaker can help you from experimentation to productionalizing ML. Following the standard steps of an ML project, from the experimental phrase (code development and experiments), to the operational phrase (automatization of the model build workflow and deployment pipelines), SageMaker can bring efficiency in the following steps:
- Explore the data and build the ML code with Amazon SageMaker Studio notebooks.
- Train and tune the model with a SageMaker training job.
- Deploy the model with an SageMaker endpoint for model serving.
- Orchestrate the workflow with Amazon SageMaker Pipelines.
The following diagram illustrates this architecture and workflow.
It’s important to note that you can use SageMaker in a modular way. For example, you can build your code with a local integrated development environment (IDE) and train and deploy your model on SageMaker, or you can develop and train your model in your own cluster compute sources, and use a SageMaker pipeline for workflow orchestration and deploy on a SageMaker endpoint. This means that SageMaker provides an open platform to adapt for your own requirements.
See the code in our GitHub repository and README to understand the code structure.
Provision a SageMaker project
You can use Amazon SageMaker Projects to start your journey. With a SageMaker project, you can manage the versions for your Git repositories so you can collaborate across teams more efficiently, ensure code consistency, and enable continuous integration and continuous delivery (CI/CD). Although notebooks are helpful for model building and experimentation, when you have a team of data scientists and ML engineers working on an ML problem, you need a more scalable way to maintain code consistency and have stricter version control.
SageMaker projects create a preconfigured MLOps template, which includes the essential components for simplifying the PaddleOCR integration:
- A code repository to build custom container images for processing, training, and inference, integrated with CI/CD tools. This allows us to configure our custom Docker image and push to Amazon Elastic Container Registry (Amazon ECR) to be ready to use.
- A SageMaker pipeline that defines steps for data preparation, training, model evaluation, and model registration. This prepares us to be MLOps ready when the ML project goes to production.
- Other useful resources, such as a Git repository for code version control, model group that contains model versions, code change trigger for the model build pipeline, and event-based trigger for the model deployment pipeline.
You can use SageMaker seed code to create standard SageMaker projects, or a specific template that your organization created for team members. In this post, we use the standard MLOps template for image building, model building, and model deployment. For more information about creating a project in Studio, refer to Create an MLOps Project using Amazon SageMaker Studio.
Explore data and build ML code with SageMaker Studio Notebooks
SageMaker Studio notebooks are collaborative notebooks that you can launch quickly because you don’t need to set up compute instances and file storage beforehand. Many data scientists prefer to use this web-based IDE for developing the ML code, quickly debugging the library API, and getting things running with a small sample of data to validate the training script.
In Studio notebooks, you can use a pre-built environment for common frameworks such as TensorFlow, PyTorch, Pandas, and Scikit-Learn. You can install the dependencies to the pre-built kernel, or build up your own persistent kernel image. For more information, refer to Install External Libraries and Kernels in Amazon SageMaker Studio. Studio notebooks also provide a Python environment to trigger SageMaker training jobs, deployment, or other AWS services. In the following sections, we illustrate how to use Studio notebooks as an environment to trigger training and deployment jobs.
SageMaker provides a powerful IDE; it’s an open ML platform where data scientists have the flexibility to use their preferred development environment. For data scientists who prefer a local IDE such as PyCharm or Visual Studio Code, you can use the local Python environment to develop your ML code, and use SageMaker for training in a managed scalable environment. For more information, see Run your TensorFlow job on Amazon SageMaker with a PyCharm IDE. After you have a solid model, you can adopt the MLOps best practices with SageMaker.
Currently, SageMaker also provides SageMaker notebook instances as our legacy solution for the Jupyter Notebook environment. You have the flexibility to run the Docker build command and use SageMaker local mode to train on your notebook instance. We also provide sample code for PaddleOCR in our code repository: ./train_and_deploy/notebook.ipynb.
Build a custom image with a SageMaker project template
SageMaker makes extensive use of Docker containers for build and runtime tasks. You can run your own container with SageMaker easily. See more technical details at Use Your Own Training Algorithms.
However, as a data scientist, building a container might not be straightforward. SageMaker projects provide a simple way for you to manage custom dependencies through an image building CI/CD pipeline. When you use a SageMaker project, you can make updates to the training image with your custom container Dockerfile. For step-by-step instructions, refer to Create Amazon SageMaker projects with image building CI/CD pipelines. With the structure provided in the template, you can modify the provided code in this repository to build a PaddleOCR training container.
For this post, we showcase the simplicity of building a custom image for processing, training, and inference. The GitHub repo contains three folders:
- Preprocessing – image-build-process/
- Training – image-build-train/
- Inference – image-build-inference/
These projects follow a similar structure. Take the training container image as an example; the image-build-train/
repository contains the following files:
- The codebuild-buildspec.yml file, which is used to configure AWS CodeBuild so that the image can be built and pushed to Amazon ECR.
- The Dockerfile used for the Docker build, which contains all dependencies and the training code.
- The train.py entry point for training script, with all hyperparameters (such as learning rate and batch size) that can be configured as an argument. These arguments are specified when you start the training job.
- The dependencies.
When you push the code into the corresponding repository, it triggers AWS CodePipeline to build a training container for you. The custom container image is stored in an Amazon ECR repository, as illustrated in the previous figure. A similar procedure is adopted for generating the inference image.
Train the model with the SageMaker training SDK
After your algorithm code is validated and packaged into a container, you can use a SageMaker training job to provision a managed environment to train the model. This environment is ephemeral, meaning that you can have separate, secure compute resources (such as GPU) or a Multi-GPU distributed environment to run your code. When the training is complete, SageMaker saves the resulting model artifacts to an Amazon Simple Storage Service (Amazon S3) location that you specify. All the log data and metadata persist on the AWS Management Console, Studio, and Amazon CloudWatch.
The training job includes several important pieces of information:
- The URL of the S3 bucket where you stored the training data
- The URL of the S3 bucket where you want to store the output of the job
- The managed compute resources that you want SageMaker to use for model training
- The Amazon ECR path where the training container is stored
For more information about training jobs, see Train Models. The example code for the training job is available at experiments-train-notebook.ipynb.
SageMaker makes the hyperparameters in a CreateTrainingJob
request available in the Docker container in the /opt/ml/input/config/hyperparameters.json
file.
We use the custom training container as the entry point and specify a GPU environment for the infrastructure. All relevant hyperparameters are detailed as parameters, which allows us to track each individual job configuration, and compare them with the experiment tracking.
Because the data science process is very research-oriented, it’s common that multiple experiments are running in parallel. This requires an approach that keeps track of all the different experiments, different algorithms, and potentially different datasets and hyperparameters attempted. Amazon SageMaker Experiments lets you organize, track, compare, and evaluate your ML experiments. We demonstrate this as well in experiments-train-notebook.ipynb. For more details, refer to Manage Machine Learning with Amazon SageMaker Experiments.
Deploy the model for model serving
As for deployment, especially for real-time model serving, many data scientists might find it hard to do without help from operation teams. SageMaker makes it simple to deploy your trained model into production with the SageMaker Python SDK. You can deploy your model to SageMaker hosting services and get an endpoint to use for real-time inference.
In many organizations, data scientists might not be responsible for maintaining the endpoint infrastructure. However, testing your model as an endpoint and guaranteeing the correct prediction behaviors is indeed the responsibility of data scientists. Therefore, SageMaker simplified the tasks for deploying by adding a set of tools and SDK for this.
For the use case in the post, we want to have real-time, interactive, low-latency capabilities. Real-time inference is ideal for this inference workload. However, there are many options adapting to each specific requirement. For more information, refer to Deploy Models for Inference.
To deploy the custom image, data scientists can use the SageMaker SDK, illustrated at
experiments-deploy-notebook.ipynb.
In the create_model
request, the container definition includes the ModelDataUrl
parameter, which identifies the Amazon S3 location where model artifacts are stored. SageMaker uses this information to determine from where to copy the model artifacts. It copies the artifacts to the /opt/ml/model
directory for use by your inference code. The serve
and predictor.py
is the entry point for serving, with the model artifact that is loaded when you start the deployment. For more information, see Use Your Own Inference Code with Hosting Services.
Orchestrate your workflow with SageMaker Pipelines
The last step is to wrap your code as end-to-end ML workflows, and to apply MLOps best practices. In SageMaker, the model building workload, a directed acyclic graph (DAG), is managed by SageMaker Pipelines. Pipelines is a fully managed service supporting orchestration and data lineage tracking. In addition, because Pipelines is integrated with the SageMaker Python SDK, you can create your pipelines programmatically using a high-level Python interface that we used previously during the training step.
We provide an example of pipeline code to illustrate the implementation at pipeline.py.
The pipeline includes a preprocessing step for dataset generation, training step, condition step, and model registration step. At the end of each pipeline run, data scientists may want to register their model for version controls and deploy the best performing one. The SageMaker model registry provides a central place to manage model versions, catalog models, and trigger automated model deployment with approval status of a specific model. For more details, refer to Register and Deploy Models with Model Registry.
In an ML system, automated workflow orchestration helps prevent model performance degradation, in other words model drift. Early and proactive detection of data deviations enables you to take corrective actions, such as retraining models. You can trigger the SageMaker pipeline to retrain a new version of the model after deviations have been detected. The trigger of a pipeline can be also determined by Amazon SageMaker Model Monitor, which continuously monitors the quality of models in production. With the data capture capability to record information, Model Monitor supports data and model quality monitoring, bias, and feature attribution drift monitoring. For more details, see Monitor models for data and model quality, bias, and explainability.
Conclusion
In this post, we illustrated how to run the framework PaddleOCR on SageMaker for OCR tasks. To help data scientists easily onboard SageMaker, we walked through the ML development lifecycle, from building algorithms, to training, to hosting the model as a web service for real-time inference. You can use the template code we provided to migrate an arbitrary framework onto the SageMaker platform. Try it out for your ML project and let us know your success stories.
About the Authors
Junyi(Jackie) LIU is an Senior Applied Scientist at AWS. She has many years of working experience in the field of machine learning. She has rich practical experience in the development and implementation of solutions in the construction of machine learning models in supply chain prediction algorithms, advertising recommendation systems, OCR and NLP area.
Yanwei Cui, PhD, is a Machine Learning Specialist Solutions Architect at AWS. He started machine learning research at IRISA (Research Institute of Computer Science and Random Systems), and has several years of experience building artificial intelligence powered industrial applications in computer vision, natural language processing and online user behavior prediction. At AWS, he shares the domain expertise and helps customers to unlock business potentials, and to drive actionable outcomes with machine learning at scale. Outside of work, he enjoys reading and traveling.
Yi-An CHEN is a Software Developer at Amazon Lab 126. She has more than 10 years experience in developing machine learning driven products across diverse disciplines, including personalization, natural language processing and computer vision. Outside of work, she likes to do long running and biking.
Meta Research PhD Fellowship Spotlight: Solving dynamic problems to reduce algorithm bias
As a continuation of our Fellowship spotlight series, we’re highlighting a 2021 Meta PhD Fellow, Andrés Cristi, PhD candidate at University of Chile.Read More
Drive efficiencies with CI/CD best practices on Amazon Lex
Let’s say you have identified a use case in your organization that you would like to handle via a chatbot. You familiarized yourself with Amazon Lex, built a prototype, and did a few trial interactions with the bot. You liked the overall experience and now want to deploy the bot in your production environment, but aren’t sure about best practices for Amazon Lex. In this post, we review the best practices for developing and deploying Amazon Lex bots, enabling you to streamline the end-to-end bot lifecycle and optimize your operations.
We have covered the planning, design, and configuration phases in previous blog posts. We suggest reviewing these posts to help you build engaging conversations with your bot before you proceed. After you’ve initially configured the bot, you should test it internally and iterate on the bot definition. You’re now ready to deploy it in your production environment (such as a call center), where the bot will process live conversations. Once in production, you should monitor it continuously to make sure it’s meeting your desired business goals. This cycle repeats as you add new use cases and enhancements.
Let’s review the best practices for development, testing, deployment, and monitoring bots.
Development
Consider the following best practices when developing your bot:
- Manage bot schema via code – The Amazon Lex console provides an easy-to-use interface as you design and configure the bot, but relies on manual actions to replicate the setup. We recommend converting the bot schema into code after finishing the design to simplify this step. You can use APIs or AWS CloudFormation (see Creating Amazon Lex V2 resources with AWS CloudFormation) to manage the bot programmatically.
- Checkpoint bot schema with bot versioning – Checkpointing is a common approach often used to revert an application to a last-known stable state. Amazon Lex offers this functionality via bot versioning. We recommend using a new version at each milestone in your development process. This allows you to make incremental changes to your bot definition, with an easy way to revert them in case they don’t work as expected.
- Identify data handling requirements and configure appropriate controls – Amazon Lex follows the AWS shared responsibility model, which includes guidelines for data protection to comply with industry regulations and with your company’s own data privacy standards. Additionally, Amazon Lex adheres to compliance programs such as SOC, PCI, and FedRAMP. Amazon Lex provides the ability to obfuscate slots that are considered sensitive. You should identify your data privacy requirements and configure the appropriate controls in your bot.
Testing
After you have a bot definition, you should test the bot to ensure that it works as intended and is configured correctly. For example, it should have permissions to trigger other services, such as AWS Lambda functions. In addition, you should also test the bot to confirm it’s able to interpret different types of user requests. Consider the following best practices for testing:
- Identify test data – You should gather relevant test data to test bot performance. The test data should include a comprehensive representation of expected user conversations with the bot, especially for IVR use cases where the bot will need to understand voice inputs. The test data should cover different speaking styles and accents. Such test data can provide experience validation for your target customer base.
- Identify user experience metrics – Defining the conversational experience can be hard. You have to anticipate and plan for all the different ways users might engage with the bot. How do you guide the caller without sounding too prescriptive? How do you recover if the caller provides incorrect or incomplete information? To manage the dialog through many different scenarios, you should set a clear goal that covers different speaking styles, acoustic conditions, and modality, and identify objective metrics that you can track. For example, an objective indicator would be “90% of conversations should have less than two re-prompts played to the user,” versus a subjective indicator such as “the majority of conversations should not ask users to repeat their input.”
- Evaluate user experience along the way – In some cases, seemingly small changes can have a big impact on the user experience. For example, consider a situation where you inadvertently introduce a typo in the regular expression used for an account ID slot type, which leads to bot re-prompting the user to provide input again. You should evaluate the user experience, and invest in an automated testing to generate key metrics. You can refer to Evaluating an automatic speech recognition service and Testing accuracy and regression with Amazon Connect and Amazon Lex for examples of how to test and generate key metrics.
Deployment
Once you’re satisfied with the bot’s performance, you’ll want to deploy the bot to start serving your production traffic. As you iterate the bot over the course of its lifecycle, you repeat the deployments, making it a continuous process, so it’s critical to have a streamlined, automated deployment to reduce the chance of errors. Consider the following best practices for deployment:
- Use a multi-account environment – You should follow the AWS recommended multi-account environment setup in your organization and use separate AWS accounts for your development stage and production stage. If you have a multi-Region presence, then you should also use a separate AWS account per Region for production. Using separate AWS accounts per stage offers you security, access, and billing boundaries for your AWS resources.
- Automate promoting a bot from development through to production – When replicating the bot setup in your development stage to your production stage, you should use automated solutions and minimize manual touch points. You should use CloudFormation templates to create your bots. Alternatively, you can use Amazon Lex export and import APIs to provide an automated means to copy a bot schema across accounts.
- Roll out changes in a phased manner – You should deploy changes to your production environment in a phased manner, so that changes are released to a subset of your production traffic before being released to all users. Such an approach gives you the chance to limit the blast radius in case there are any issues with the change. One way you can achieve this is by having a two-phased deployment approach: you create two aliases for a bot (for example, prod-05 and prod-95). You first associate the new bot version with one alias (prod-05 in this example). After you validate the key metrics meet the success criteria, you associate the second alias (prod-95) with the new bot version.
Note that you need to control the distribution of traffic on the client application used to integrate with Amazon Lex bots. For example, if you’re using Amazon Connect to integrate with your bots, you can use a Distribute by percentage contact block in conjunction with two or more Get customer input blocks.
It’s important to note that Amazon Lex provides a test alias out of the box. The test alias is meant to be used for ad hoc manual testing via the Amazon Lex console only, and is not meant to handle production-scale loads. We recommend using a dedicated alias for your production traffic.
Monitoring
Monitoring is important for maintaining reliability, availability, and an effective end-user experience. You should analyze your bot’s metrics and use the learnings as a feedback mechanism to improve the bot schema as well your development, testing, and deployment practices. Amazon Lex supports multiple mechanisms to monitor bots. Consider the following best practices for monitoring your Lex bots:
- Monitor constantly and iterate – Amazon Lex integrates with Amazon CloudWatch to provide near-real-time metrics that can provide you with key insights into your users’ interactions with the bot. These insights can help you gain perspective on the end-user experience. To learn more about the different types of metrics that Amazon Lex emits, see Monitoring Amazon Lex V2 with Amazon CloudWatch. We recommend setting up thresholds to trigger alarms. Similarly, Amazon Lex gives you visibility into the raw input utterances from your users’ interactions with the bot. You should use utterance statistics or conversation logs to gain insights to identify communication patterns and make appropriate changes to your bot as necessary. To learn how to create a personalized analytics dashboard for your bots, refer to Monitor operational metrics for your Amazon Lex chatbot.
The best practices discussed in this post focus primarily on Amazon Lex-specific use cases. In addition to these, you should review and adhere to best practices when managing your cloud infrastructure in AWS. Make sure that your cloud infrastructure is secure and only accessible by authorized users. You should also review and adopt the appropriate AWS security best practices within your organization. Lastly, you should proactively review the AWS quotas for individual AWS services (including Amazon Lex quotas) and request appropriate changes if necessary.
Conclusion
You can use Amazon Lex to enable sophisticated natural language conversations and drive customer service efficiencies. In this post, we reviewed the best practices for the development, testing, deployment, and monitoring phases of a bot lifecycle. With these guidelines, you can improve the end-user experience and achieve better customer engagement. Start building your Amazon Lex conversational experience today!
About the Author
Swapandeep Singh is an engineer with the Amazon Lex team. He works on making interactions with bots smoother and more human-like. Outside of work, he likes to travel and learn about different cultures.