Imagine a surgeon taking video calls with patients across the globe without the need of a human translator. What if a fledgling startup could easily expand their product across borders and into new geographical markets by offering fluid, accurate, multilingual customer support and sales, all without the need of a live human translator? What happens to your business when you’re no longer bound by language?
It’s common today to have virtual meetings with international teams and customers that speak many different languages. Whether they’re internal or external meetings, meaning often gets lost in complex discussions and you may encounter language barriers that prevent you from being as effective as you could be.
In this post, you will learn how to use three fully managed AWS services (Amazon Transcribe, Amazon Translate, and Amazon Polly) to produce a near-real-time speech-to-speech translator solution that can quickly translate a source speaker’s live voice input into a spoken, accurate, translated target language, all with zero machine learning (ML) experience.
Overview of solution
Our translator consists of three fully managed AWS ML services working together in a single Python script by using the AWS SDK for Python (Boto3) for our text translation and text-to-speech portions, and an asynchronous streaming SDK for audio input transcription.
Amazon Transcribe: Streaming speech to text
The first service you use in our stack is Amazon Transcribe, a fully managed speech-to-text service that takes input speech and transcribes it to text. Amazon Transcribe has flexible ingestion methods, batch or streaming, because it accepts either stored audio files or streaming audio data. In this post, you use the asynchronous Amazon Transcribe streaming SDK for Python, which uses the HTTP/2 streaming protocol to stream live audio and receive live transcriptions.
When we first built this prototype, Amazon Transcribe streaming ingestion didn’t support automatic language detection, but this is no longer the case as of November 2021. Both batch and streaming ingestion now support automatic language detection for all supported languages. In this post, we show how a parameter-based solution though a seamless multi-language parameterless design is possible through the use of streaming automatic language detection. After our transcribed speech segment is returned as text, you send a request to Amazon Translate to translate and return the results in our Amazon Transcribe EventHandler
method.
Amazon Translate: State-of-the-art, fully managed translation API
Next in our stack is Amazon Translate, a neural machine translation service that delivers fast, high-quality, affordable, and customizable language translation. As of June of 2022, Amazon Translate supports translation across 75 languages, with new language pairs and improvements being made constantly. Amazon Translate uses deep learning models hosted on a highly scalable and resilient AWS Cloud architecture to quickly deliver accurate translations either in real time or batched, depending on your use case. Using Amazon Translate is straightforward and requires no management of underlying architecture or ML skills. Amazon Translate has several features, like creating and using a custom terminology to handle mapping between industry-specific terms. For more information on Amazon Translate service limits, refer to Guidelines and limits. After the application receives the translated text in our target language, it sends the translated text to Amazon Polly for immediate translated audio playback.
Amazon Polly: Fully managed text-to-speech API
Finally, you send the translated text to Amazon Polly, a fully managed text-to-speech service that can either send back lifelike audio clip responses for immediate streaming playback or batched and saved in Amazon Simple Storage Service (Amazon S3) for later use. You can control various aspects of speech such as pronunciation, volume, pitch, speech rate, and more using standardized Speech Synthesis Markup Language (SSML).
You can synthesize speech for certain Amazon Polly Neural voices using the Newscaster style to make them sound like a TV or radio newscaster. You can also detect when specific words or sentences in the text are being spoken based on the metadata included in the audio stream. This allows the developer to synchronize graphical highlighting and animations, such as the lip movements of an avatar, with the synthesized speech.
You can modify the pronunciation of particular words, such as company names, acronyms, foreign words, or neologisms, for example “P!nk,” “ROTFL,” or “C’est la vie” (when spoken in a non-French voice), using custom lexicons.
Architecture overview
The following diagram illustrates our solution architecture.
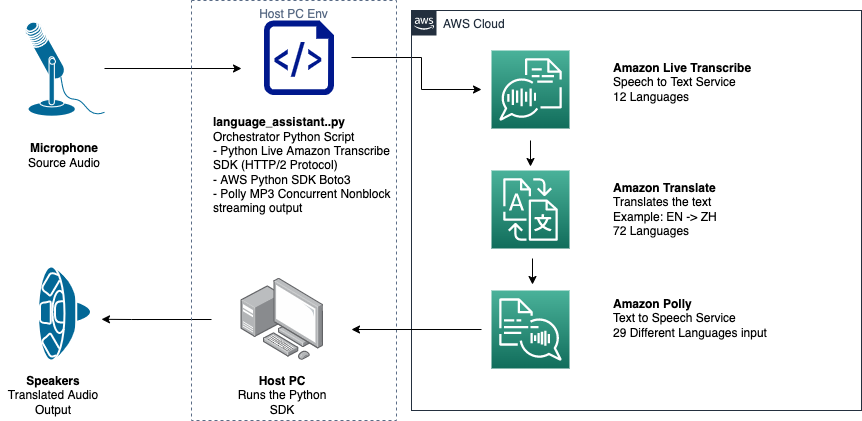
This diagram shows the data flow from the client device to Amazon Transcribe, Amazon Translate, and Amazon Polly
The workflow is as follows:
- Audio is ingested by the Python SDK.
- Amazon Polly converts the speech to text, in 39 possible languages.
- Amazon Translate converts the languages.
- Amazon Live Transcribe converts text to speech.
- Audio is outputted to speakers.
Prerequisites
You need a host machine set up with a microphone, speakers, and reliable internet connection. A modern laptop should work fine for this because no additional hardware is needed. Next, you need to set up the machine with some software tools.
You must have Python 3.7+ installed to use the asynchronous Amazon Transcribe streaming SDK and for a Python module called pyaudio
, which you use to control the machine’s microphone and speakers. This module depends on a C library called portaudio.h
. If you encounter issues with pyaudio
errors, we suggest checking your OS to see if you have the portaudio.h
library installed.
For authorization and authentication of service calls, you create an AWS Identity and Access Management (IAM) service role with permissions to call the necessary AWS services. By configuring the AWS Command Line Interface (AWS CLI) with this IAM service role, you can run our script on your machine without having to pass in keys or passwords, because the AWS libraries are written to use the configured AWS CLI user’s credentials. This is a convenient method for rapid prototyping and ensures our services are being called by an authorized identity. As always, follow the principle of least privilege when assigning IAM policies when creating an IAM user or role.
To summarize, you need the following prerequisites:
- A PC, Mac, or Linux machine with microphone, speakers, and internet connection
- The
portaudio.h
C library for your OS (brew, apt get, wget), which is needed for pyaudio to work - AWS CLI 2.0 with properly authorized IAM user configured by running aws configure in the AWS CLI
- Python 3.7+
- The asynchronous Amazon Transcribe Python SDK
- The following Python libraries:
boto3
amazon-transcribe
pyaudio
asyncio
concurrent
Implement the solution
You will be relying heavily on the asynchronous Amazon Transcribe streaming SDK for Python as a starting point, and are going to build on top of that specific SDK. After you have experimented with the streaming SDK for Python, you add streaming microphone input by using pyaudio
, a commonly used Python open-source library used for manipulating audio data. Then you add Boto3 calls to Amazon Translate and Amazon Polly for our translation and text-to-speech functionality. Finally, you stream out translated speech through the computer’s speakers again with pyaudio
. The Python module concurrent
gives you the ability to run blocking code in its own asynchronous thread to play back your returned Amazon Polly speech in a seamless, non-blocking way.
Let’s import all our necessary modules, transcribe streaming classes, and instantiate some globals:
First, you use pyaudio
to obtain the input device’s sampling rate, device index, and channel count:
If this isn’t working, you can also loop through and print your devices as shown in the following code, and then use the device index to retrieve the device information with pyaudio
:
You use channel_count
, sample_rate
, and dev_index
as parameters in a mic stream. In that stream’s callback function, you use an asyncio
nonblocking thread-safe callback to put the input bytes of the mic stream into an asyncio
input queue. Take note of the loop and input_queue objects created with asyncio
and how they’re used in the following code:
Now when the generator function mic_stream()
is called, it continually yields input bytes as long as there is microphone input data in the input queue.
Now that you know how to get input bytes from the microphone, let’s look at how to write Amazon Polly output audio bytes to a speaker output stream:
Now let’s expand on what you built in the post Asynchronous Amazon Transcribe Streaming SDK for Python. In the following code, you create an executor object using the ThreadPoolExecutor
subclass with three workers with concurrent. You then add an Amazon Translate call on the finalized returned transcript in the EventHandler and pass that translated text, the executor object, and our aws_polly_tts()
function into an asyncio
loop with loop.run_in_executor()
, which runs our Amazon Polly function (with translated input text) asynchronously at the start of next iteration of the asyncio
loop.
Finally, we have the loop_me()
function. In it, you define write_chunks()
, which takes an Amazon Transcribe stream as an argument and asynchronously writes chunks of streaming mic input to it. You then use MyEventHandler()
with the output transcription stream as its argument and create a handler object. Then you use await with asyncio.gather()
and pass in the write_chunks() and handler with the handle_events() method to handle the eventual futures of these coroutines. Lastly, you gather all event loops and loop the loop_me()
function with run_until_complete()
. See the following code:
When the preceding code is run together without errors, you can speak into the microphone and quickly hear your voice translated to Mandarin Chinese. The automatic language detection feature for Amazon Transcribe and Amazon Translate translates any supported input language into the target language. You can speak for quite some time and because of the non-blocking nature of the function calls, all your speech input is translated and spoken, making this an excellent tool for translating live speeches.
Conclusion
Although this post demonstrated how these three fully managed AWS APIs can function seamlessly together, we encourage you to think about how you could use these services in other ways to deliver multilingual support for services or media like multilingual closed captioning for a fraction of the current cost. Medicine, business, and even diplomatic relations could all benefit from an ever-improving, low-cost, low-maintenance translation service.
For more information about the proof of concept code base for this use case check out our Github.
About the Authors
Michael Tran is a Solutions Architect with Envision Engineering team at Amazon Web Services. He provides technical guidance and helps customers accelerate their ability to innovate through showing the art of the possible on AWS. He has built multiple prototypes around AI/ML, and IoT for our customers. You can contact me @Mike_Trann on Twitter.
Cameron Wilkes is a Prototyping Architect on the AWS Industry Accelerator team. While on the team he delivered several ML based prototypes to customers to demonstrate the “Art of the Possible” of ML on AWS. He enjoys music production, off-roading and design.