In November 2021, in collaboration with RStudio PBC, we announced the general availability of RStudio on Amazon SageMaker, the industry’s first fully managed RStudio Workbench IDE in the cloud. You can now bring your current RStudio license to easily migrate your self-managed RStudio environments to Amazon SageMaker in just a few simple steps.
RStudio is one of the most popular IDEs among R developers for machine learning (ML) and data science projects. RStudio provides open-source tools for R and enterprise-ready professional software for data science teams to develop and share their work in the organization. Bringing RStudio on SageMaker not only gives you access to the AWS infrastructure in a fully managed way, but it also gives you native access to SageMaker.
In this post, we explore how you can use SageMaker features via RStudio on SageMaker to build a SageMaker pipeline that builds, processes, trains and registers your R models. We also explore using SageMaker for our model deployment, all using R.
Solution overview
The following diagram shows the architecture used in our solution. All code used in this example can be found in the GitHub repository.
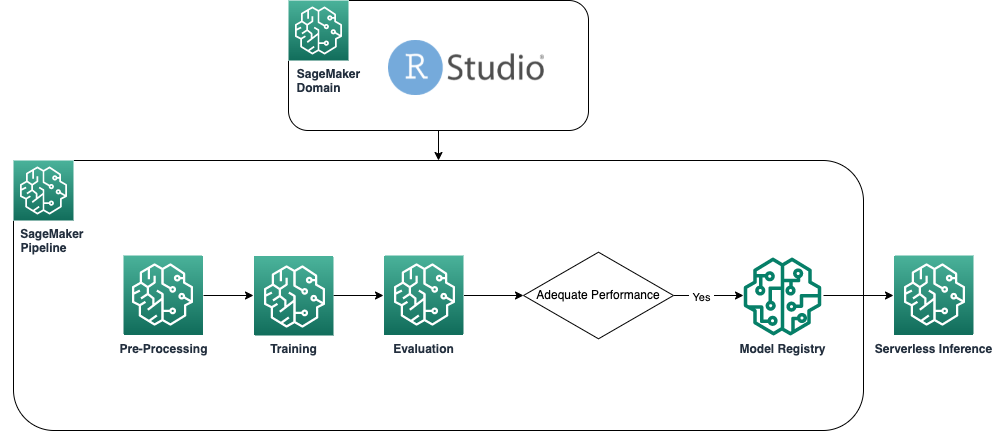
Prerequisites
To follow this post, access to RStudio on SageMaker is required. If you’re new to using RStudio on SageMaker, review Get started with RStudio on Amazon SageMaker.
We also need to build custom Docker containers. We use AWS CodeBuild to build these containers, so you need a few extra AWS Identity and Access Management (IAM) permissions that you might not have by default. Before you proceed, make sure that the IAM role that you’re using has a trust policy with CodeBuild:
{
"Version": "2012-10-17",
"Statement": [
{
"Effect": "Allow",
"Principal": {
"Service": [
"codebuild.amazonaws.com"
]
},
"Action": "sts:AssumeRole"
}
]
}
The following permissions are also required in the IAM role to run a build in CodeBuild and push the image to Amazon Elastic Container Registry (Amazon ECR):
{
"Version": "2012-10-17",
"Statement": [
{
"Effect": "Allow",
"Action": [
"codebuild:DeleteProject",
"codebuild:CreateProject",
"codebuild:BatchGetBuilds",
"codebuild:StartBuild"
],
"Resource": "arn:aws:codebuild:*:*:project/sagemaker-studio*"
},
{
"Effect": "Allow",
"Action": "logs:CreateLogStream",
"Resource": "arn:aws:logs:*:*:log-group:/aws/codebuild/sagemaker-studio*"
},
{
"Effect": "Allow",
"Action": [
"logs:GetLogEvents",
"logs:PutLogEvents"
],
"Resource": "arn:aws:logs:*:*:log-group:/aws/codebuild/sagemaker-studio*:log-stream:*"
},
{
"Effect": "Allow",
"Action": "logs:CreateLogGroup",
"Resource": "*"
},
{
"Effect": "Allow",
"Action": [
"ecr:CreateRepository",
"ecr:BatchGetImage",
"ecr:CompleteLayerUpload",
"ecr:DescribeImages",
"ecr:DescribeRepositories",
"ecr:UploadLayerPart",
"ecr:ListImages",
"ecr:InitiateLayerUpload",
"ecr:BatchCheckLayerAvailability",
"ecr:PutImage"
],
"Resource": "arn:aws:ecr:*:*:repository/sagemaker-studio*"
},
{
"Sid": "ReadAccessToPrebuiltAwsImages",
"Effect": "Allow",
"Action": [
"ecr:BatchGetImage",
"ecr:GetDownloadUrlForLayer"
],
"Resource": [
"arn:aws:ecr:*:763104351884:repository/*",
"arn:aws:ecr:*:217643126080:repository/*",
"arn:aws:ecr:*:727897471807:repository/*",
"arn:aws:ecr:*:626614931356:repository/*",
"arn:aws:ecr:*:683313688378:repository/*",
"arn:aws:ecr:*:520713654638:repository/*",
"arn:aws:ecr:*:462105765813:repository/*"
]
},
{
"Sid": "EcrAuthorizationTokenRetrieval",
"Effect": "Allow",
"Action": [
"ecr:GetAuthorizationToken"
],
"Resource": [
"*"
]
},
{
"Effect": "Allow",
"Action": [
"s3:GetObject",
"s3:DeleteObject",
"s3:PutObject"
],
"Resource": "arn:aws:s3:::sagemaker-*/*"
},
{
"Effect": "Allow",
"Action": [
"s3:CreateBucket"
],
"Resource": "arn:aws:s3:::sagemaker*"
},
{
"Effect": "Allow",
"Action": [
"iam:GetRole",
"iam:ListRoles"
],
"Resource": "*"
},
{
"Effect": "Allow",
"Action": "iam:PassRole",
"Resource": "arn:aws:iam::*:role/*",
"Condition": {
"StringLikeIfExists": {
"iam:PassedToService": "codebuild.amazonaws.com"
}
}
}
]
}
Create baseline R containers
To use our R scripts for processing and training on SageMaker processing and training jobs, we need to create our own Docker containers containing the necessary runtime and packages. The ability to use your own container, which is part of the SageMaker offering, gives great flexibility to developers and data scientists to use the tools and frameworks of their choice, with virtually no limitations.
We create two R-enabled Docker containers: one for processing jobs and one for training and deployment of our models. Processing data typically requires different packages and libraries than modeling, so it makes sense here to separate the two stages and use different containers.
For more details about using containers with SageMaker, refer to Using Docker containers with SageMaker.
The container used for processing is defined as follows:
FROM public.ecr.aws/docker/library/r-base:4.1.2
# Install tidyverse
RUN apt update && apt-get install -y --no-install-recommends
r-cran-tidyverse
RUN R -e "install.packages(c('rjson'))"
ENTRYPOINT ["Rscript"]
For this post, we use a simple and relatively lightweight container. Depending on your or your organization’s needs, you may want to pre-install several more R packages.
The container used for training and deployment is defined as follows:
FROM public.ecr.aws/docker/library/r-base:4.1.2
RUN apt-get -y update && apt-get install -y --no-install-recommends
wget
apt-transport-https
ca-certificates
libcurl4-openssl-dev
libsodium-dev
RUN apt-get update && apt-get install -y python3-dev python3-pip
RUN pip3 install boto3
RUN R -e "install.packages(c('readr','plumber', 'reticulate'),dependencies=TRUE, repos='http://cran.rstudio.com/')"
ENV PATH="/opt/ml/code:${PATH}"
WORKDIR /opt/ml/code
COPY ./docker/run.sh /opt/ml/code/run.sh
COPY ./docker/entrypoint.R /opt/ml/entrypoint.R
RUN /bin/bash -c 'chmod +x /opt/ml/code/run.sh'
ENTRYPOINT ["/bin/bash", "run.sh"]
The RStudio kernel runs on a Docker container, so you won’t be able to build and deploy the containers using Docker commands directly on your Studio session. Instead, you can use the very useful library sagemaker-studio-image-build, which essentially outsources the task of building containers to CodeBuild.
With the following commands, we create two Amazon ECR registries: sagemaker-r-processing
and sagemaker-r-train-n-deploy
, and build the respective containers that we use later:
if (!py_module_available("sagemaker-studio-image-build")){py_install("sagemaker-studio-image-build", pip=TRUE)}
system("cd pipeline-example ; sm-docker build . —file ./docker/Dockerfile-train-n-deploy —repository sagemaker-r-train-and-deploy:1.0")
system("cd pipeline-example ; sm-docker build . —file ./docker/Dockerfile-processing —repository sagemaker-r-processing:1.0")
Create the pipeline
Now that the containers are built and ready, we can create the SageMaker pipeline that orchestrates the model building workflow. The full code of this is under the file pipeline.R
in the repository. The easiest way to create a SageMaker pipeline is by using the SageMaker SDK, which is a Python library that we can access using the library reticulate. This gives us access to all functionalities of SageMaker without leaving the R language environment.
The pipeline we build has the following components:
-
Preprocessing step – This is a SageMaker processing job (utilizing the
sagemaker-r-processing
container) responsible for preprocessing the data and splitting the data into train and test datasets.
-
Training step – This is a SageMaker training job (utilizing the
sagemaker-r-train-n-deploy
container) responsible for training the model. In this example, we train a simple linear model.
-
Evaluation step – This is a SageMaker processing job (utilizing the
sagemaker-r-processing
container) responsible for performing evaluation of the model. Specifically in this example, we’re interested in the RMSE (root mean square error) on the test dataset, which we want to use in the next step as well as to associate with the model itself.
-
Conditional step – This is a conditional step, native to SageMaker pipelines, that allows us to branch the pipeline logic based on some parameter. In this case, the pipeline branches based on the value of RMSE that is calculated in the previous step.
-
Register model step – If the preceding conditional step is
True
, and the performance of the model is acceptable, then the model is registered in the model registry. For more information, refer to Register and Deploy Models with Model Registry.
First call the upsert function to create (or update) the pipeline and then call the start function to actually start running the pipeline:
source("pipeline-example/pipeline.R")
my_pipeline <- get_pipeline(input_data_uri=s3_raw_data)
upserted <- my_pipeline$upsert(role_arn=role_arn)
started <- my_pipeline$start()
Inspect the pipeline and model registry
One of the great things about using RStudio on SageMaker is that by being on the SageMaker platform, you can use the right tool for the right job and swiftly switch between them based on what you need to do.
As soon as we start the pipeline run, we can switch to Amazon SageMaker Studio, which allows us to visualize the pipeline and monitor current and previous runs of it.
To view details about the pipeline we just created and ran, navigate to the Studio IDE interface, choose SageMaker resources, choose Pipelines on the drop-down menu, and choose the pipeline (in this case, AbalonePipelineUsingR
).
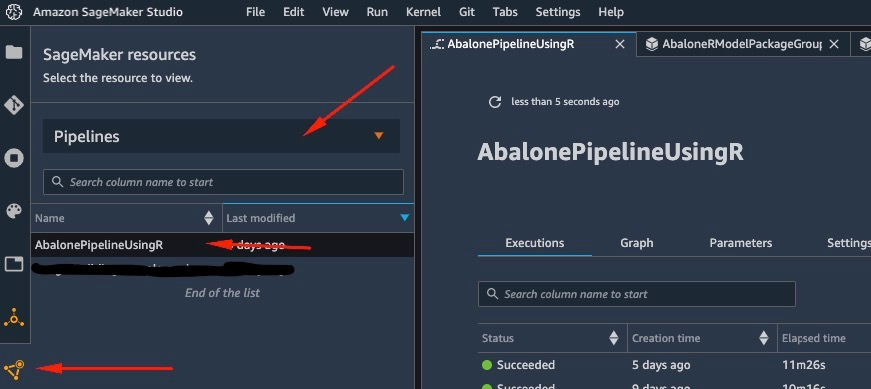
This reveals details of the pipeline, including all current and previous runs. Choose the latest one to bring up a visual representation of the pipeline, as per the following screenshot.
The DAG of the pipeline is created automatically by the service based on the data dependencies between steps, as well as based on custom added dependencies (not added any in this example).
When the run is complete, if successful, you should see all the steps turn green.
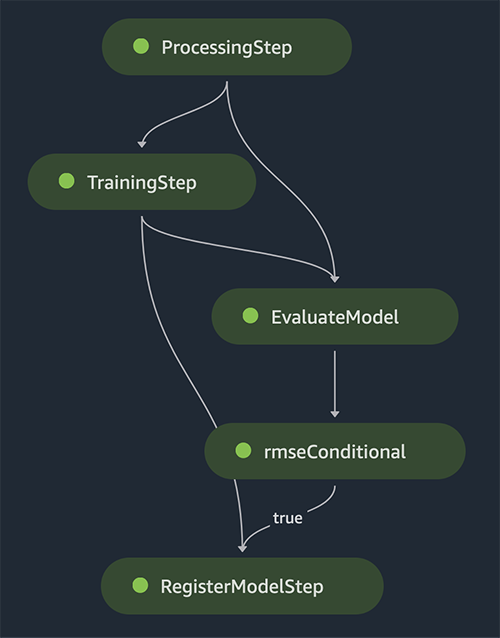
Choosing any of the individual steps brings up details about the specific step, including inputs, outputs, logs, and initial configuration settings. This allows you to drill down in the pipeline and investigate any failed steps.
Similarly, when the pipeline has finished running, a model is saved in the model registry. To access it, in the SageMaker resources pane, choose Model registry on the drop-down and choose your model. This reveals the list of registered models, as shown in the following screenshot. Choose one to open the details page for that particular model version.
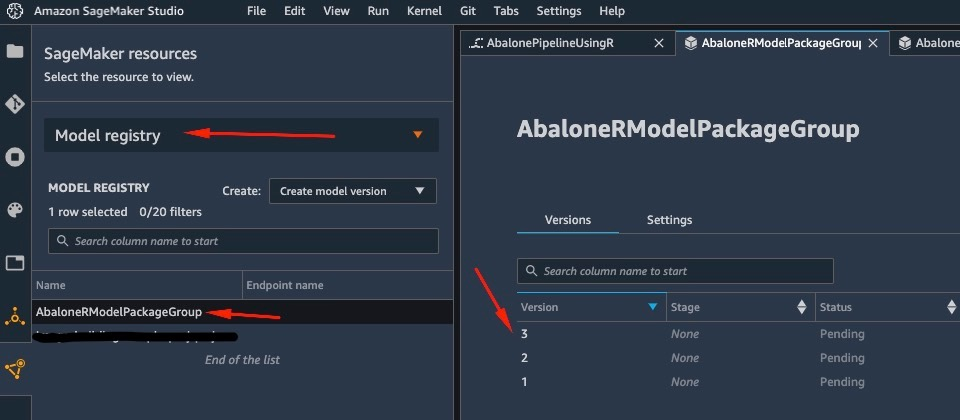
After you open a version of the model, choose Update Status and Approve to approve the model.
At this point, based on your use case, you can set up this approval to trigger further actions, including the deployment of the model as per your needs.
Serverless deployment of the model
After you’ve trained and registered a model on SageMaker, deploying the model on SageMaker is straightforward.
There are several options of how you can deploy a model, such as batch inference, real-time endpoints, or asynchronous endpoints. Each method comes with several required configurations, including choosing the instance type you want as well as the scaling mechanism.
For this example, we use the recently announced feature of SageMaker, Serverless Inference (in preview mode as of the time of writing), to deploy our R model on a serverless endpoint. For this type of endpoint, we only define the amount of RAM that we want to be allocated to the model for inference, as well as the maximum number of allowed concurrent invocations of the model. SageMaker takes care of hosting the model and auto scaling as needed. You’re only charged for the exact number of seconds and data used by the model, with no cost for idle time.
You can deploy the model to a serverless endpoint with the following code:
model_package_arn <- 'ENTER_MODEL_PACKAGE_ARN_HERE'
model <- sagemaker$ModelPackage(
role=role_arn,
model_package_arn=model_package_arn,
sagemaker_session=session)
serverless_config <- sagemaker$serverless$ServerlessInferenceConfig(
memory_size_in_mb=1024L,
max_concurrency=5L)
model$deploy(serverless_inference_config=serverless_config,
endpoint_name="serverless-r-abalone-endpoint")
If you see the error ClientError: An error occurred (ValidationException) when calling the CreateModel operation: Invalid approval status "PendingManualApproval"
the model you want to deploy hasn’t been approved. Follow the steps from the previous section to approve your model.
Invoke the endpoint by sending a request to the HTTP endpoint we deployed, or instead use the SageMaker SDK. In the following code, we invoke the endpoint on some test data:
library(jsonlite)
x = list(features=format_csv(abalone_t[1:3,1:11]))
x = toJSON(x)
# test the endpoint
predictor <- sagemaker$predictor$Predictor(endpoint_name="serverless-r-abalone-endpoint", sagemaker_session=session)
predictor$predict(x)
The endpoint we invoked was a serverless endpoint, and as such we’re charged for the exact duration and data used. You might notice that the first time you invoke the endpoint it takes about a second to respond. This is due to the cold start time of the serverless endpoint. If you make another invocation soon after, the model returns the prediction in real time because it’s already warm.
When you finish experimenting with the endpoint, you can delete it with the following command:
predictor$delete_endpoint(delete_endpoint_config=TRUE)
Conclusion
In this post, we walked through the process of creating a SageMaker pipeline using R in our RStudio environment and showcased how to deploy our R model on a serverless endpoint on SageMaker using the SageMaker model registry.
With the combination of RStudio and SageMaker, you can now create and orchestrate complete end-to-end ML workflows on AWS using our preferred language of choice, R.
To dive deeper into this solution, I encourage you to review the source code of this solution, as well as other examples, on GitHub.
About the Author
Georgios Schinas is a Specialist Solutions Architect for AI/ML in the EMEA region. He is based in London and works closely with customers in UK and Ireland. Georgios helps customers design and deploy machine learning applications in production on AWS with a particular interest in MLOps practices and enabling customers to perform machine learning at scale. In his spare time, he enjoys traveling, cooking and spending time with friends and family.
Read More