3D Parametric Room Representation with RoomPlan
Apple Machine Learning Research
NVIDIA and VMware CEOs Discuss New Era of Enterprise Computing
Reinventing enterprise computing for the modern era, VMware CEO Raghu Raghuram Tuesday announced the availability of the VMware vSphere 8 enterprise workload platform running on NVIDIA DPUs, or data processing units, an initiative formerly known as Project Monterey.
Placing the announcement in context, Raghuram and NVIDIA founder and CEO Jensen Huang discussed how running VMware vSphere 8 for BlueField is a huge moment for enterprise computing and how this reinvents the data center altogether.
“Today, we’ve got customers that are deploying NVIDIA AI in the enterprise, in the data center,” Raghuram said. “Together, we are changing the world for enterprise computing.”
Both agreed AI plays a central role for every company and discussed the growing importance of multi-tenant data centers, hybrid-cloud development, and accelerated infrastructure deployment in a 20-minute conversation at the VMware Explore 2022 conference.
To address this, the companies announced a partnership two years ago to deliver an end-to-end enterprise platform for AI as well as a new architecture for data center, cloud and edge that uses NVIDIA DPUs to support existing and next-generation applications.
The stakes for partners and customers are high: AI has become “mission critical” for every enterprise, Raghuram explained. Yet studies show half of AI projects fail to make it to production, with infrastructure complexity a leading cause, he added.
For example, VMware and NVIDIA are working with healthcare providers to accelerate medical image processing using AI to offer a better quality of service to their patients.
Together with Carilion Clinic, NVIDIA will discuss at VMware Explore how the largest healthcare organization in Virginia is future-proofing their hospitals.
AI for Every Enterprise
New AI-enabled applications include recommender systems, speech and vision analytics, and natural language processing.
This will run on the NVIDIA and VMware AI-Ready Enterprise Platform, which is accelerated by NVIDIA GPUs and DPUs, and optimized and delivered across the breadth of VMware products, Huang said.
Many industries are now embracing VMware and NVIDIA’s joint solutions, Huang said.
In telecom, for example, NTT Communications is deploying multi-tenant services based on the platform. (Watch the breakout session at VMware Explore on “NTTcom Enabling AI Applications for Enterprises in the Cloud.”)
VMware vSphere 8 With NVIDIA DPUs
VMware vSphere 8 with NVIDIA DPUs will be vital to bringing cloud and multi-tenant cloud, hybrid cloud and zero-trust security to enterprises.
Modern organizations continue to generate and process large amounts of data, Raghuram said.
New waves of workloads are starting to emerge that are highly distributed across the data center, network edge and multi-cloud, he added.
Raghuram said that customers need better performance and security in this new era.
DPUs will play a crucial role in the new infrastructure architecture to accelerate performance, free up CPU cycles and provide better security.
“We have rearchitected vSphere to run on DPUs,” Raghuram said. This offloads software-defined infrastructure tasks like network and storage processing, he added.
“And now you get accelerated I/O, and you can have agility for developers, because all of that storage and network processing is now running in the DPU,” he said.
NVIDIA BlueField DPUs
Huang explained that cloud computing and AI are driving a reinvention of data center architecture, and that data centers are the new unit of compute.
The DPU is a new type of processor to reduce the processing burden on CPUs and provide a zero-trust security model, Huang explained.
The NVIDIA BlueField DPU, an accelerated computing platform, is designed for all enterprise workloads and optimized for NVIDIA AI, Huang explained. The BlueField DPU offloads, accelerates and isolates the software-defined infrastructure of the data center — networking, security, storage and virtualization.
“The return on investment — the benefits that DPU-enabled vSphere 8 with NVIDIA BlueField deliver — will be so fast because it frees up so many resources for computing that the payback is going to be instantaneous,” Huang said. “It’s going to be a really fantastic return.”
Security Isolation
This approach is ideal for today’s security challenges. The traditional approach, Raghuram explained, is based on firewalls that focus on the network perimeter.
With the vSphere platform, VMware NSX and advancements in silicon, “we can now bring intrinsic security to life,” Raghuram said.
This new approach, with the NSX distributed firewall running on BlueField DPUs, enables every node to be more secure at virtually every touch point, Huang explained, and the zero-trust security model is finally realized.
“And this is where BlueField and vSphere 8, with NSX running on BlueField, is such an incredible revolution,” Huang said. “We’re essentially going to have a firewall in every single computer.”
vSphere on DPU
Enterprises can get started now. Raghuram announced the first release of vSphere on DPU is available with the vSphere 8 release, with ESXi and NSX support on BlueField DPU.
It lets users improve infrastructure performance by offloading and accelerating functions on the DPU, providing more host resources to business applications, Raghuram said.
Certain latency- and bandwidth-sensitive workloads that previously used virtualization “pass-thru” can now run fully virtualized with similar performance in this new architecture, without losing key vSphere capabilities like vMotion and DRS, Raghuram said.
Infrastructure admins can rely on vSphere to also manage the DPU lifecycle, thereby reducing operational overhead, Raghuram added. And enterprises can boost infrastructure security by isolating infrastructure domains on a DPU.
“The beauty of what the vSphere engineers have done is they have not changed the management model,” Raghuram said. “And so, it can fit seamlessly into the data center architecture of today, while enabling the future to come about.”
Showcasing the vSphere on BlueField DPU solution, Dell and NVIDIA are announcing an enterprise AI platform that uses VMware. It includes AI acceleration by NVIDIA GPUs, infrastructure acceleration by BlueField DPUs, advanced AI frameworks from NVIDIA, and management by vSphere.
All of this will be available soon running on Dell servers and Dell VxRail hyper-converged infrastructure. It allows customers to use familiar VMware vSphere tools to deploy and manage AI infrastructure.
NVIDIA LaunchPad
VMware vSphere users are able to freely experience these workloads today on NVIDIA LaunchPad, Huang said, adding that “it’s available worldwide.”
With LaunchPad, a free program that gives users access to hands-on AI labs, there’s no need to procure and stand up infrastructure to offload, accelerate and isolate vSphere on a DPU before experiencing the lab, Huang explained.
“I can’t wait to try it out myself,” Raghuram said, adding that “this is just the start.”
Sign up now to try the VMware vSphere platform running on NVIDIA BlueField DPUs.
Watch the fireside chat with NVIDIA CEO Jensen Huang and VMware CEO Raghu Raghuram, below.
The post NVIDIA and VMware CEOs Discuss New Era of Enterprise Computing appeared first on NVIDIA Blog.
Janus framework lifts continual learning to the next level
By managing and automating many of the steps involved in continual learning, Janus is helping Amazon’s latest robots adapt to a changing environment.Read More
Create a batch recommendation pipeline using Amazon Personalize with no code
With personalized content more likely to drive customer engagement, businesses continuously seek to provide tailored content based on their customer’s profile and behavior. Recommendation systems in particular seek to predict the preference an end-user would give to an item. Some common use cases include product recommendations on online retail stores, personalizing newsletters, generating music playlist recommendations, or even discovering similar content on online media services.
However, it can be challenging to create an effective recommendation system due to complexities in model training, algorithm selection, and platform management. Amazon Personalize enables developers to improve customer engagement through personalized product and content recommendations with no machine learning (ML) expertise required. Developers can start to engage customers right away by using captured user behavior data. Behind the scenes, Amazon Personalize examines this data, identifies what is meaningful, selects the right algorithms, trains and optimizes a personalization model that is customized for your data, and provides recommendations via an API endpoint.
Although providing recommendations in real time can help boost engagement and satisfaction, sometimes this might not actually be required, and performing this in batch on a scheduled basis can simply be a more cost-effective and manageable option.
This post shows you how to use AWS services to not only create recommendations but also operationalize a batch recommendation pipeline. We walk through the end-to-end solution without a single line of code. We discuss two topics in detail:
- Preparing your data using AWS Glue
- Orchestrating the Amazon Personalize batch inference jobs using AWS Step Functions
Solution overview
In this solution, we use the MovieLens dataset. This dataset includes 86,000 ratings of movies from 2,113 users. We attempt to use this data to generate recommendations for each of these users.
Data preparation is very important to ensure we get customer behavior data into a format that is ready for Amazon Personalize. The architecture described in this post uses AWS Glue, a serverless data integration service, to perform the transformation of raw data into a format that is ready for Amazon Personalize to consume. The solution uses Amazon Personalize to create batch recommendations for all users by using a batch inference. We then use a Step Functions workflow so that the automated workflow can be run by calling Amazon Personalize APIs in a repeatable manner.
The following diagram demonstrates this solution.
We will build this solution with the following steps:
- Build a data transformation job to transform our raw data using AWS Glue.
- Build an Amazon Personalize solution with the transformed dataset.
- Build a Step Functions workflow to orchestrate the generation of batch inferences.
Prerequisites
You need the following for this walkthrough:
- An AWS account
- Permissions to create new AWS Identity and Access Management (IAM) roles
Build a data transformation job to transform raw data with AWS Glue
With Amazon Personalize, input data needs to have a specific schema and file format. Data from interactions between users and items must be in CSV format with specific columns, whereas the list of users for which you want to generate recommendations for must be in JSON format. In this section, we use AWS Glue Studio to transform raw input data into the required structures and format for Amazon Personalize.
AWS Glue Studio provides a graphical interface that is designed for easy creation and running of extract, transform, and load (ETL) jobs. You can visually create data transformation workloads through simple drag-and-drop operations.
We first prepare our source data in Amazon Simple Storage Service (Amazon S3), then we transform the data without code.
- On the Amazon S3 console, create an S3 bucket with three folders: raw, transformed, and curated.
- Download the MovieLens dataset and upload the uncompressed file named user_ratingmovies-timestamp.dat to your bucket under the raw folder.
- On the AWS Glue Studio console, choose Jobs in the navigation pane.
- Select Visual with a source and target, then choose Create.
- Choose the first node called Data source – S3 bucket. This is where we specify our input data.
- On the Data source properties tab, select S3 location and browse to your uploaded file.
- For Data format, choose CSV, and for Delimiter, choose Tab.
- We can choose the Output schema tab to verify that the schema has inferred the columns correctly.
- If the schema doesn’t match your expectations, choose Edit to edit the schema.
Next, we transform this data to follow the schema requirements for Amazon Personalize.
- Choose the Transform – Apply Mapping node and, on the Transform tab, update the target key and data types.
Amazon Personalize, at minimum, expects the following structure for the interactions dataset:
In this example, we exclude the poorly rated movies in the dataset.
- To do so, remove the last node called S3 bucket and add a filter node on the Transform tab.
- Choose Add condition and filter out data where rating < 3.5.
We now write the output back to Amazon S3.
- Expand the Target menu and choose Amazon S3.
- For S3 Target Location, choose the folder named
transformed
. - Choose CSV as the format and suffix the Target Location with
interactions/
.
Next, we output a list of users that we want to get recommendations for.
- Choose the ApplyMapping node again, and then expand the Transform menu and choose ApplyMapping.
- Drop all fields except for
user_id
and rename that field touserId
. Amazon Personalize expects that field to be named userId. - Expand the Target menu again and choose Amazon S3.
- This time, choose JSON as the format, and then choose the transformed S3 folder and suffix it with
batch_users_input/
.
This produces a JSON list of users as input for Amazon Personalize. We should now have a diagram that looks like the following.
We are now ready to run our transform job.
- On the IAM console, create a role called glue-service-role and attach the following managed policies:
AWSGlueServiceRole
AmazonS3FullAccess
For more information on how to create IAM service roles, refer to the Creating a role to delegate permissions to an AWS service.
- Navigate back to your AWS Glue Studio job, and choose the Job details tab.
- Set the job name as
batch-personalize-input-transform-job
. - Choose the newly created IAM role.
- Keep the default values for everything else.
- Choose Save.
- When you’re ready, choose Run and monitor the job in the Runs tab.
- When the job is complete, navigate to the Amazon S3 console to validate that your output file has been successfully created.
We have now shaped our data into the format and structure that Amazon Personalize requires. The transformed dataset should have the following fields and format:
-
Interactions dataset – CSV format with fields
USER_ID
,ITEM_ID
,TIMESTAMP
-
User input dataset – JSON format with element
userId
Build an Amazon Personalize solution with the transformed dataset
With our interactions dataset and user input data in the right format, we can now create our Amazon Personalize solution. In this section, we create our dataset group, import our data, and then create a batch inference job. A dataset group organizes resources into containers for Amazon Personalize components.
- On the Amazon Personalize console, choose Create dataset group.
- For Domain, select Custom.
- Choose Create dataset group and continue.
Next, create the interactions dataset.
We now import the interactions data that we had created earlier.
- Navigate to the S3 bucket in which we created our interactions CSV dataset.
- On the Permissions tab, add the following bucket access policy so that Amazon Personalize has access. Update the policy to include your bucket name.
Navigate back to Amazon Personalize and choose Create your dataset import job. Our interactions dataset should now be importing into Amazon Personalize. Wait for the import job to complete with a status of Active before continuing to the next step. This should take approximately 8 minutes.
- On the Amazon Personalize console, choose Overview in the navigation pane and choose Create solution.
- Enter a solution name.
- For Solution type, choose Item recommendation.
- For Recipe, choose the
aws-user-personalization
recipe. - Choose Create and train solution.
The solution now trains against the interactions dataset that was imported with the user personalization recipe. Monitor the status of this process under Solution versions. Wait for it to complete before proceeding. This should take approximately 20 minutes.
We now create our batch inference job, which generates recommendations for each of the users present in the JSON input.
- In the navigation pane, under Custom resources, choose Batch inference jobs.
- Enter a job name, and for Solution, choose the solution created earlier.
- Choose Create batch inference job.
- For Input data configuration, enter the S3 path of where the
batch_users_input
file is located.
This is the JSON file that contains userId
.
- For Output data configuration path, choose the curated path in S3.
- Choose Create batch inference job.
This process takes approximately 30 minutes. When the job is finished, recommendations for each of the users specified in the user input file are saved in the S3 output location.
We have successfully generated a set of recommendations for all of our users. However, we have only implemented the solution using the console so far. To make sure that this batch inferencing runs regularly with the latest set of data, we need to build an orchestration workflow. In the next section, we show you how to create an orchestration workflow using Step Functions.
Build a Step Functions workflow to orchestrate the batch inference workflow
To orchestrate your pipeline, complete the following steps:
- On the Step Functions console, choose Create State Machine.
- Select Design your workflow visually, then choose Next.
- Drag the
CreateDatasetImportJob
node from the left (you can search for this node in the search box) onto the canvas. - Choose the node, and you should see the configuration API parameters on the right. Record the ARN.
- Enter your own values in the API Parameters text box.
This calls the CreateDatasetImportJob API with the parameter values that you specify.
- Drag the
CreateSolutionVersion
node onto the canvas. - Update the API parameters with the ARN of the solution that you noted down.
This creates a new solution version with the newly imported data by calling the CreateSolutionVersion API.
- Drag the
CreateBatchInferenceJob
node onto the canvas and similarly update the API parameters with the relevant values.
Make sure that you use the $.SolutionVersionArn
syntax to retrieve the solution version ARN parameter from the previous step. These API parameters are passed to the CreateBatchInferenceJob API.
We need to build a wait logic in the Step Functions workflow to make sure the recommendation batch inference job finishes before the workflow completes.
- Find and drag in a Wait node.
- In the configuration for Wait, enter 300 seconds.
This is an arbitrary value; you should alter this wait time according to your specific use case.
- Choose the
CreateBatchInferenceJob
node again and navigate to the Error handling tab. - For Catch errors, enter
Personalize.ResourceInUseException
. - For Fallback state, choose Wait.
This step enables us to periodically check the status of the job and it only exits the loop when the job is complete.
- For ResultPath, enter
$.errorMessage
.
This effectively means that when the “resource in use” exception is received, the job waits for x seconds before trying again with the same inputs.
- Choose Save, and then choose Start the execution.
We have successfully orchestrated our batch recommendation pipeline for Amazon Personalize. As an optional step, you can use Amazon EventBridge to schedule a trigger of this workflow on a regular basis. For more details, refer to EventBridge (CloudWatch Events) for Step Functions execution status changes.
Clean up
To avoid incurring future charges, delete the resources that you created for this walkthrough.
Conclusion
In this post, we demonstrated how to create a batch recommendation pipeline by using a combination of AWS Glue, Amazon Personalize, and Step Functions, without needing a single line of code or ML experience. We used AWS Glue to prep our data into the format that Amazon Personalize requires. Then we used Amazon Personalize to import the data, create a solution with a user personalization recipe, and create a batch inferencing job that generates a default of 25 recommendations for each user, based on past interactions. We then orchestrated these steps using Step Functions so that we can run these jobs automatically.
For steps to consider next, user segmentation is one of the newer recipes in Amazon Personalize, which you might want to explore to create user segments for each row of the input data. For more details, refer to Getting batch recommendations and user segments.
About the author
Maxine Wee is an AWS Data Lab Solutions Architect. Maxine works with customers on their use cases, designs solutions to solve their business problems, and guides them through building scalable prototypes. Prior to her journey with AWS, Maxine helped customers implement BI, data warehousing, and data lake projects in Australia.
AI that can learn the patterns of human language
Human languages are notoriously complex, and linguists have long thought it would be impossible to teach a machine how to analyze speech sounds and word structures in the way human investigators do.
But researchers at MIT, Cornell University, and McGill University have taken a step in this direction. They have demonstrated an artificial intelligence system that can learn the rules and patterns of human languages on its own.
When given words and examples of how those words change to express different grammatical functions (like tense, case, or gender) in one language, this machine-learning model comes up with rules that explain why the forms of those words change. For instance, it might learn that the letter “a” must be added to end of a word to make the masculine form feminine in Serbo-Croatian.
This model can also automatically learn higher-level language patterns that can apply to many languages, enabling it to achieve better results.
The researchers trained and tested the model using problems from linguistics textbooks that featured 58 different languages. Each problem had a set of words and corresponding word-form changes. The model was able to come up with a correct set of rules to describe those word-form changes for 60 percent of the problems.
This system could be used to study language hypotheses and investigate subtle similarities in the way diverse languages transform words. It is especially unique because the system discovers models that can be readily understood by humans, and it acquires these models from small amounts of data, such as a few dozen words. And instead of using one massive dataset for a single task, the system utilizes many small datasets, which is closer to how scientists propose hypotheses — they look at multiple related datasets and come up with models to explain phenomena across those datasets.
“One of the motivations of this work was our desire to study systems that learn models of datasets that is represented in a way that humans can understand. Instead of learning weights, can the model learn expressions or rules? And we wanted to see if we could build this system so it would learn on a whole battery of interrelated datasets, to make the system learn a little bit about how to better model each one,” says Kevin Ellis ’14, PhD ’20, an assistant professor of computer science at Cornell University and lead author of the paper.
Joining Ellis on the paper are MIT faculty members Adam Albright, a professor of linguistics; Armando Solar-Lezama, a professor and associate director of the Computer Science and Artificial Intelligence Laboratory (CSAIL); and Joshua B. Tenenbaum, the Paul E. Newton Career Development Professor of Cognitive Science and Computation in the Department of Brain and Cognitive Sciences and a member of CSAIL; as well as senior author
Timothy J. O’Donnell, assistant professor in the Department of Linguistics at McGill University, and Canada CIFAR AI Chair at the Mila – Quebec Artificial Intelligence Institute.
The research is published today in Nature Communications.
Looking at language
In their quest to develop an AI system that could automatically learn a model from multiple related datasets, the researchers chose to explore the interaction of phonology (the study of sound patterns) and morphology (the study of word structure).
Data from linguistics textbooks offered an ideal testbed because many languages share core features, and textbook problems showcase specific linguistic phenomena. Textbook problems can also be solved by college students in a fairly straightforward way, but those students typically have prior knowledge about phonology from past lessons they use to reason about new problems.
Ellis, who earned his PhD at MIT and was jointly advised by Tenenbaum and Solar-Lezama, first learned about morphology and phonology in an MIT class co-taught by O’Donnell, who was a postdoc at the time, and Albright.
“Linguists have thought that in order to really understand the rules of a human language, to empathize with what it is that makes the system tick, you have to be human. We wanted to see if we can emulate the kinds of knowledge and reasoning that humans (linguists) bring to the task,” says Albright.
To build a model that could learn a set of rules for assembling words, which is called a grammar, the researchers used a machine-learning technique known as Bayesian Program Learning. With this technique, the model solves a problem by writing a computer program.
In this case, the program is the grammar the model thinks is the most likely explanation of the words and meanings in a linguistics problem. They built the model using Sketch, a popular program synthesizer which was developed at MIT by Solar-Lezama.
But Sketch can take a lot of time to reason about the most likely program. To get around this, the researchers had the model work one piece at a time, writing a small program to explain some data, then writing a larger program that modifies that small program to cover more data, and so on.
They also designed the model so it learns what “good” programs tend to look like. For instance, it might learn some general rules on simple Russian problems that it would apply to a more complex problem in Polish because the languages are similar. This makes it easier for the model to solve the Polish problem.
Tackling textbook problems
When they tested the model using 70 textbook problems, it was able to find a grammar that matched the entire set of words in the problem in 60 percent of cases, and correctly matched most of the word-form changes in 79 percent of problems.
The researchers also tried pre-programming the model with some knowledge it “should” have learned if it was taking a linguistics course, and showed that it could solve all problems better.
“One challenge of this work was figuring out whether what the model was doing was reasonable. This isn’t a situation where there is one number that is the single right answer. There is a range of possible solutions which you might accept as right, close to right, etc.,” Albright says.
The model often came up with unexpected solutions. In one instance, it discovered the expected answer to a Polish language problem, but also another correct answer that exploited a mistake in the textbook. This shows that the model could “debug” linguistics analyses, Ellis says.
The researchers also conducted tests that showed the model was able to learn some general templates of phonological rules that could be applied across all problems.
“One of the things that was most surprising is that we could learn across languages, but it didn’t seem to make a huge difference,” says Ellis. “That suggests two things. Maybe we need better methods for learning across problems. And maybe, if we can’t come up with those methods, this work can help us probe different ideas we have about what knowledge to share across problems.”
In the future, the researchers want to use their model to find unexpected solutions to problems in other domains. They could also apply the technique to more situations where higher-level knowledge can be applied across interrelated datasets. For instance, perhaps they could develop a system to infer differential equations from datasets on the motion of different objects, says Ellis.
“This work shows that we have some methods which can, to some extent, learn inductive biases. But I don’t think we’ve quite figured out, even for these textbook problems, the inductive bias that lets a linguist accept the plausible grammars and reject the ridiculous ones,” he adds.
“This work opens up many exciting venues for future research. I am particularly intrigued by the possibility that the approach explored by Ellis and colleagues (Bayesian Program Learning, BPL) might speak to how infants acquire language,” says T. Florian Jaeger, a professor of brain and cognitive sciences and computer science at the University of Rochester, who was not an author of this paper. “Future work might ask, for example, under what additional induction biases (assumptions about universal grammar) the BPL approach can successfully achieve human-like learning behavior on the type of data infants observe during language acquisition. I think it would be fascinating to see whether inductive biases that are even more abstract than those considered by Ellis and his team — such as biases originating in the limits of human information processing (e.g., memory constraints on dependency length or capacity limits in the amount of information that can be processed per time) — would be sufficient to induce some of the patterns observed in human languages.”
This work was funded, in part, by the Air Force Office of Scientific Research, the Center for Brains, Minds, and Machines, the MIT-IBM Watson AI Lab, the Natural Science and Engineering Research Council of Canada, the Fonds de Recherche du Québec – Société et Culture, the Canada CIFAR AI Chairs Program, the National Science Foundation (NSF), and an NSF graduate fellowship.
Meet the Omnivore: Artist Fires Up NVIDIA Omniverse to Glaze Animated Ceramics
Editor’s note: This post is a part of our Meet the Omnivore series, which features individual creators and developers who use NVIDIA Omniverse to accelerate their 3D workflows and create virtual worlds.
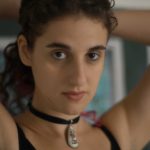
Vanessa Rosa’s art transcends time: it merges traditional and contemporary techniques, gives new life to ancient tales and imagines possible futures.
The U.S.-based 3D artist got her start creating street art in Rio de Janeiro, where she grew up. She’s since undertaken artistic tasks like painting murals for Le Centre in Cotonou, Benin, and publishing children’s books.
Now, her focus is on using NVIDIA Omniverse — a platform for connecting and building custom 3D pipelines — to create what she calls Little Martians, a sci-fi universe in which ceramic humanoids discuss theories related to the past, present and future of humanity.
To kick-start the project, Rosa created the most primitive artwork that she could think of: mask-like ceramics, created with local clay and baked with traditional in-ground kilns.
Then, in a sharply modern twist, she 3D scanned them with applications like Polycam and Regard3D. And to animate them, she recorded herself narrating stories with the motion-capture app Face Cap — as well as generated AI voices from text and used the Omniverse Audio2Face app to create facial animations.
An Accessible, Streamlined Platform for 3D Animation
Prior to the Little Martians project, Rosa seldom relied on technology for her artwork. Only recently did she switch from her laptop to a desktop computer powered by an NVIDIA RTX 5000 GPU, which significantly cut her animation render times.
Omniverse quickly became the springboard for Rosa’s digital workflow.
“I’m new to 3D animation, so NVIDIA applications made it much easier for me to get started rather than having to learn how to rig and animate characters solely in software,” she said. “The power of Omniverse is that it makes 3D simulations accessible to a much larger audience of creators, rather than just 3D professionals.”
After generating animations and voice-overs with Omniverse, she employed an add-on for Blender called Faceit that accepts .json files from Audio2Face.
“This has greatly improved my workflow, as I can continue to develop my projects on Blender after generating animations with Omniverse,” she said.
At the core of Omniverse is Universal Scene Description — an open-source, extensible 3D framework and common language for creating virtual worlds. With USD, creators like Rosa can work with multiple applications and extensions all on a centralized platform, further streamlining workflows.
Considering herself a beginner in 3D animation, Rosa feels she’s “only scratched the surface of what’s possible with Omniverse.” In the future, she plans to use the platform to create more interactive media.
“I love that with this technology, pieces can exist in the physical world, but gain new life in the digital world,” she said. “I’d like to use it to create avatars out of my ceramics, so that a person could interact with it and talk to it using an interface.”
With Little Martians, Rosa hopes to inspire her audience to think about the long processes of history — and empower artists that use traditional techniques to explore the possibilities of design and simulation technology like Omniverse.
“I am always exploring new techniques and sharing my process,” she said. “I believe my work can help other people who love the traditional fine arts to adapt to the digital world.”
Join In on the Creation
Creators and developers across the world can download NVIDIA Omniverse for free, and enterprise teams can use the platform for their 3D projects.
Learn more about NVIDIA’s latest AI breakthroughs powering graphics and virtual worlds at GTC, running online Sept. 19-22. Attend the top sessions for 3D creators and developers to learn more about how Omniverse can accelerate workflows, and join the NVIDIA Omniverse User Group to connect with other artists. Register free now.
Check out artwork from other “Omnivores” and submit projects in the gallery. Connect your workflows to Omniverse with software from Adobe, Autodesk, Epic Games, Maxon, Reallusion and more.
Follow NVIDIA Omniverse on Instagram, Twitter, YouTube and Medium for additional resources and inspiration. Check out the Omniverse forums, and join our Discord server and Twitch channel to chat with the community.
The post Meet the Omnivore: Artist Fires Up NVIDIA Omniverse to Glaze Animated Ceramics appeared first on NVIDIA Blog.
Use Amazon SageMaker pipeline sharing to view or manage pipelines across AWS accounts
On August 9, 2022, we announced the general availability of cross-account sharing of Amazon SageMaker Pipelines entities. You can now use cross-account support for Amazon SageMaker Pipelines to share pipeline entities across AWS accounts and access shared pipelines directly through Amazon SageMaker API calls.
Customers are increasingly adopting multi-account architectures for deploying and managing machine learning (ML) workflows with SageMaker Pipelines. This involves building workflows in development or experimentation (dev) accounts, deploying and testing them in a testing or pre-production (test) account, and finally promoting them to production (prod) accounts to integrate with other business processes. You can benefit from cross-account sharing of SageMaker pipelines in the following use cases:
- When data scientists build ML workflows in a dev account, those workflows are then deployed by an ML engineer as a SageMaker pipeline into a dedicated test account. To further monitor those workflows, data scientists now require cross-account read-only permission to the deployed pipeline in the test account.
- ML engineers, ML admins, and compliance teams, who manage deployment and operations of those ML workflows from a shared services account, also require visibility into the deployed pipeline in the test account. They might also require additional permissions for starting, stopping, and retrying those ML workflows.
In this post, we present an example multi-account architecture for developing and deploying ML workflows with SageMaker Pipelines.
Solution overview
A multi-account strategy helps you achieve data, project, and team isolation while supporting software development lifecycle steps. Cross-account pipeline sharing supports a multi-account strategy, removing the overhead of logging in and out of multiple accounts and improving ML testing and deployment workflows by sharing resources directly across multiple accounts.
In this example, we have a data science team that uses a dedicated dev account for the initial development of the SageMaker pipeline. This pipeline is then handed over to an ML engineer, who creates a continuous integration and continuous delivery (CI/CD) pipeline in their shared services account to deploy this pipeline into a test account. To still be able to monitor and control the deployed pipeline from their respective dev and shared services accounts, resource shares are set up with AWS Resource Access Manager in the test and dev accounts. With this setup, the ML engineer and the data scientist can now monitor and control the pipelines in the dev and test accounts from their respective accounts, as shown in the following figure.
In the workflow, the data scientist and ML engineer perform the following steps:
- The data scientist (DS) builds a model pipeline in the dev account.
- The ML engineer (MLE) productionizes the model pipeline and creates a pipeline, (for this post, we call it
sagemaker-pipeline
). -
sagemaker-pipeline
code is committed to an AWS CodeCommit repository in the shared services account. - The data scientist creates an AWS RAM resource share for
sagemaker-pipeline
and shares it with the shared services account, which accepts the resource share. - From the shared services account, ML engineers are now able to describe, monitor, and administer the pipeline runs in the dev account using SageMaker API calls.
- A CI/CD pipeline triggered in the shared service account builds and deploys the code to the test account using AWS CodePipeline.
- The CI/CD pipeline creates and runs
sagemaker-pipeline
in the test account. - After running
sagemaker-pipeline
in the test account, the CI/CD pipeline creates a resource share forsagemaker-pipeline
in the test account. - A resource share from the test
sagemaker-pipeline
with read-only permissions is created with the dev account, which accepts the resource share. - The data scientist is now able to describe and monitor the test pipeline run status using SageMaker API calls from the dev account.
- A resource share from the test
sagemaker-pipeline
with extended permissions is created with the shared services account, which accepts the resource share. - The ML engineer is now able to describe, monitor, and administer the test pipeline run using SageMaker API calls from the shared services account.
In the following sections, we go into more detail and provide a demonstration on how to set up cross-account sharing for SageMaker pipelines.
How to create and share SageMaker pipelines across accounts
In this section, we walk through the necessary steps to create and share pipelines across accounts using AWS RAM and the SageMaker API.
Set up the environment
First, we need to set up a multi-account environment to demonstrate cross-account sharing of SageMaker pipelines:
- Set up two AWS accounts (dev and test). You can set this up as member accounts of an organization or as independent accounts.
- If you’re setting up your accounts as member of an organization, you can enable resource sharing with your organization. With this setting, when you share resources in your organization, AWS RAM doesn’t send invitations to principals. Principals in your organization gain access to shared resources without exchanging invitations.
- In the test account, launch Amazon SageMaker Studio and run the notebook train-register-deploy-pipeline-model. This creates an example pipeline in your test account. To simplify the demonstration, we use SageMaker Studio in the test account to launch the the pipeline. For real life projects, you should use Studio only in the dev account and launch SageMaker Pipeline in the test account using your CI/CD tooling.
Follow the instructions in the next section to share this pipeline with the dev account.
Set up a pipeline resource share
To share your pipeline with the dev account, complete the following steps:
- On the AWS RAM console, choose Create resource share.
- For Select resource type, choose SageMaker Pipelines.
- Select the pipeline you created in the previous step.
- Choose Next.
- For Permissions, choose your associated permissions.
- Choose Next.
Next, you decide how you want to grant access to principals.
- If you need to share the pipeline only within your organization accounts, select Allow Sharing only within your organization; otherwise select Allow sharing with anyone.
- For Principals, choose your principal type (you can use an AWS account, organization, or organizational unit, based on your sharing requirement). For this post, we share with anyone at the AWS account level.
- Select your principal ID.
- Choose Next.
- On the Review and create page, verify your information is correct and choose Create resource share.
- Navigate to your destination account (for this post, your dev account).
- On the AWS RAM console, under Shared with me in the navigation pane, choose Resource shares.
- Choose your resource share and choose Accept resource share.
Resource sharing permissions
When creating your resource share, you can choose from one of two supported permission policies to associate with the SageMaker pipeline resource type. Both policies grant access to any selected pipeline and all of its runs.
The AWSRAMDefaultPermissionSageMakerPipeline
policy allows the following read-only actions:
The AWSRAMPermissionSageMakerPipelineAllowExecution
policy includes all of the read-only permissions from the default policy, and also allows shared accounts to start, stop, and retry pipeline runs.
The extended pipeline run permission policy allows the following actions:
Access shared pipeline entities through direct API calls
In this section, we walk through how you can use various SageMaker Pipeline API calls to gain visibility into pipelines running in remote accounts that have been shared with you. For testing the APIs against the pipeline running in the test account from the dev account, log in to the dev account and use AWS CloudShell.
For the cross-account SageMaker Pipeline API calls, you always need to use your pipeline ARN as the pipeline identification. That also includes the commands requiring the pipeline name, where you need to use your pipeline ARN as the pipeline name.
To get your pipeline ARN, in your test account, navigate to your pipeline details in Studio via SageMaker Resources.
Choose Pipelines on your resources list.
Choose your pipeline and go to your pipeline Settings tab. You can find the pipeline ARN with your Metadata information. For this example, your ARN is defined as "arn:aws:sagemaker:us-east-1:<account-id>:pipeline/serial-inference-pipeline"
.
ListPipelineExecutions
This API call lists the runs of your pipeline. Run the following command, replacing $SHARED_PIPELINE_ARN
with your pipeline ARN from CloudShell or using the AWS Command Line Interface (AWS CLI) configured with the appropriated AWS Identity and Access Management (IAM) role:
The response lists all the runs of your pipeline with their PipelineExecutionArn
, StartTime
, PipelineExecutionStatus
, and PipelineExecutionDisplayName
:
DescribePipeline
This API call describes the detail of your pipeline. Run the following command, replacing $SHARED_PIPELINE_ARN
with your pipeline ARN:
The response provides the metadata of your pipeline, as well as information about creation and modifications of it:
DescribePipelineExecution
This API call describes the detail of your pipeline run. Run the following command, replacing $SHARED_PIPELINE_ARN
with your pipeline ARN:
The response provides details on your pipeline run, including the PipelineExecutionStatus
, ExperimentName
, and TrialName
:
StartPipelineExecution
This API call starts a pipeline run. Run the following command, replacing $SHARED_PIPELINE_ARN
with your pipeline ARN and $CLIENT_REQUEST_TOKEN
with a unique, case-sensitive identifier that you generate for this run. The identifier should have between 32–128 characters. For instance, you can generate a string using the AWS CLI kms generate-random command.
As a response, this API call returns the PipelineExecutionArn
of the started run:
StopPipelineExecution
This API call stops a pipeline run. Run the following command, replacing $PIPELINE_EXECUTION_ARN
with the pipeline run ARN of your running pipeline and $CLIENT_REQUEST_TOKEN
with an unique, case-sensitive identifier that you generate for this run. The identifier should have between 32–128 characters. For instance, you can generate a string using the AWS CLI kms generate-random command.
As a response, this API call returns the PipelineExecutionArn
of the stopped pipeline:
Conclusion
Cross-account sharing of SageMaker pipelines allows you to securely share pipeline entities across AWS accounts and access shared pipelines through direct API calls, without having to log in and out of multiple accounts.
In this post, we dove into the functionality to show how you can share pipelines across accounts and access them via SageMaker API calls.
As a next step, you can use this feature for your next ML project.
Resources
To get started with SageMaker Pipelines and sharing pipelines across accounts, refer to the following resources:
- Amazon SageMaker Model Building Pipelines
- Cross-Account Support for SageMaker Pipelines
- How resource sharing works
About the authors
Ram Vittal is an ML Specialist Solutions Architect at AWS. He has over 20 years of experience architecting and building distributed, hybrid, and cloud applications. He is passionate about building secure and scalable AI/ML and big data solutions to help enterprise customers with their cloud adoption and optimization journey to improve their business outcomes. In his spare time, he enjoys tennis, photography, and action movies.
Maira Ladeira Tanke is an ML Specialist Solutions Architect at AWS. With a background in data science, she has 9 years of experience architecting and building ML applications with customers across industries. As a technical lead, she helps customers accelerate their achievement of business value through emerging technologies and innovative solutions. In her free time, Maira enjoys traveling and spending time with her family someplace warm.
Gabriel Zylka is a Professional Services Consultant at AWS. He works closely with customers to accelerate their cloud adoption journey. Specialized in the MLOps domain, he focuses on productionizing machine learning workloads by automating end-to-end machine learning lifecycles and helping achieve desired business outcomes. In his spare time, he enjoys traveling and hiking in the Bavarian Alps.
Explore Amazon SageMaker Data Wrangler capabilities with sample datasets
Data preparation is the process of collecting, cleaning, and transforming raw data to make it suitable for insight extraction through machine learning (ML) and analytics. Data preparation is crucial for ML and analytics pipelines. Your model and insights will only be as reliable as the data you use for training them. Flawed data will produce poor results regardless of the sophistication of your algorithms and analytical tools.
Amazon SageMaker Data Wrangler is a service to help data scientists and data engineers simplify and accelerate tabular and time series data preparation and feature engineering through a visual interface. You can import data from multiple data sources, such as Amazon Simple Storage Service (Amazon S3), Amazon Athena, Amazon Redshift, Snowflake, and DataBricks, and process your data with over 300 built-in data transformations and a library of code snippets, so you can quickly normalize, transform, and combine features without writing any code. You can also bring your custom transformations in PySpark, SQL, or Pandas.
Previously, customers wanting to explore Data Wrangler needed to bring their own datasets; we’ve changed that. Starting today, you can begin experimenting with Data Wrangler’s features even faster by using a sample dataset and following suggested actions to easily navigate the product for the first time. In this post, we walk you through this process.
Solution overview
Data Wrangler offers a pre-loaded version of the well-known Titanic dataset, which is widely used to teach and experiment with ML. Data Wrangler’s suggested actions help first-time customers discover features such as Data Wrangler’s Data Quality and Insights Report, a feature that verifies data quality and helps detect abnormalities in your data.
In this post, we create a sample flow with the pre-loaded sample Titanic dataset to show how you can start experimenting with Data Wrangler’s features faster. We then use the processed Titanic dataset to create a classification model to tell us whether a passenger will survive or not, using the training functionality, which allows you to launch an Amazon SageMaker Autopilot experiment within any of the steps in a Data Wrangler flow. Along the way, we can explore Data Wrangler features through the product suggestions that surface in Data Wrangler. These suggestions can help you accelerate your learning curve with Data Wrangler by recommending actions and next steps.
Prerequisites
In order to get all the features described in this post, you need to be running the latest kernel version of Data Wrangler. For any new flow created, the kernel will always be the latest one; nevertheless, for existing flows, you need to update the Data Wrangler application first.
Import the Titanic dataset
The Titanic dataset is a public dataset widely used to teach and experiment with ML. You can use it to create an ML model that predicts which passengers will survive the Titanic shipwreck. Data Wrangler now incorporates this dataset as a sample dataset that you can use to get started with Data Wrangler more quickly. In this post, we perform some data transformations using this dataset.
Let’s create a new Data Wrangler flow and call it Titanic. Data Wrangler shows you two options: you can either import your own dataset or you can use the sample dataset (the Titanic dataset).
You’re presented with a loading bar that indicates the progress of the dataset being imported into Data Wrangler. Click through the carousel to learn more about how Data Wrangler helps you import, prepare, and process datasets for ML. Wait until the bar is fully loaded; this indicates that your dataset is imported and ready for use.
The Titanic dataset is now loaded into our flow. For a description of the dataset, refer to Titanic – Machine Learning from Disaster.
Explore Data Wrangler features
As a first-time Data Wrangler user, you now see suggested actions to help you navigate the product and discover interesting features. Let’s follow the suggested advice.
- Choose the plus sign to get a list of options to modify the dataset.
- Choose Get data insights.
This opens the Analysis tab on the data, in which you can create a Data Quality and Insights Report. When you create this report, Data Wrangler gives you the option to select a target column. A target column is a column that you’re trying to predict. When you choose a target column, Data Wrangler automatically creates a target column analysis. It also ranks the features in the order of their predictive power. When you select a target column, you must specify whether you’re trying to solve a regression or a classification problem. - Choose the column survived as the target column because that’s the value we want to predict.
- For Problem type¸ select Classification¸ because we want to know whether a passenger belongs to the survived or not survived classes.
- Choose Create.
This creates an analysis on your dataset that contains relevant points like a summary of the dataset, duplicate rows, anomalous samples, feature details, and more. To learn more about the Data Quality and Insights Report, refer to Accelerate data preparation with data quality and insights in Amazon SageMaker Data Wrangler and Get Insights On Data and Data Quality.
Let’s get a quick look at the dataset itself. - Choose the Data tab to visualize the data as a table.
Let’s now generate some example data visualizations.
- Choose the Analysis tab to start visualizing your data. You can generate three histograms: the first two visualize the number of people that survived based on the sex and class columns, as shown in the following screenshots.
The third visualizes the ages of the people that boarded the Titanic.
Let’s perform some transformations on the data,
- First, drop the columns ticket, cabin, and name.
- Next, perform one-hot encoding on the categorical columns embarked and sex, and home.dest.
- Finally, fill in missing values for the columns boat and body with a 0 value.
Your dataset now looks something like the following screenshot. - Now split the dataset into three sets: a training set with 70% of the data, a validation set with 20% of the data, and a test set with 10% of the data.
The splits done here use the stratified split approach using the survived variable and are just for the sake of the demonstration.
Now let’s configure the destination of our data.
- Choose the plus sign on each Dataset node, choose Add destination, and choose S3 to add an Amazon S3 destination for the transformed datasets.
- In the Add a destination pane, you can configure the Amazon S3 details to store your processed datasets.
Our Titanic flow should now look like the following screenshot.
You can now transform all the data using SageMaker processing jobs.
- Choose Create job.
- Keep the default values and choose Next.
- Choose Run.
A new SageMaker processing job is now created. You can see the job’s details and track its progress on the SageMaker console under Processing jobs.
When the processing job is complete, you can navigate to any of the S3 locations specified for storing the datasets and query the data just to confirm that the processing was successful. You can now use this data to feed your ML projects.
Launch an Autopilot experiment to create a classifier
You can now launch Autopilot experiments directly from Data Wrangler and use the data at any of the steps in the flow to automatically train a model on the data.
- Choose the Dataset node called Titanic_dataset (train) and navigate to the Train tab.
Before training, you need to first export your data to Amazon S3. - Follow the instructions to export your data to an S3 location of your choice.
You can specify to export the data in CSV or Parquet format for increased efficiency. Additionally, you can specify an AWS Key Management Service (AWS KMS) key to encrypt your data.
On the next page, you configure your Autopilot experiment. - Unless your data is split into several parts, leave the default value under Connect your data.
- For this demonstration, leave the default values for Experiment name and Output data location.
- Under Advanced settings, expand Machine learning problem type.
- Choose Binary classification as the problem type and Accuracy as the objective metric.You specify these two values manually even though Autopilot is capable of inferring them from the data.
- Leave the rest of the fields with the default values and choose Create Experiment.
Wait for a couple of minutes until the Autopilot experiment is complete, and you will see a leaderboard like the following with each of the models obtained by Autopilot.
You can now choose to deploy any of the models in the leaderboard for inference.
Clean up
When you’re not using Data Wrangler, it’s important to shut down the instance on which it runs to avoid incurring additional fees.
To avoid losing work, save your data flow before shutting Data Wrangler down.
- To save your data flow in Amazon SageMaker Studio, choose File, then choose Save Data Wrangler Flow.
Data Wrangler automatically saves your data flow every 60 seconds. - To shut down the Data Wrangler instance, in Studio, choose Running Instances and Kernels.
- Under RUNNING APPS, choose the shutdown icon next to the sagemaker-data-wrangler-1.0 app.
- Choose Shut down all to confirm.
Data Wrangler runs on an ml.m5.4xlarge instance. This instance disappears from RUNNING INSTANCES when you shut down the Data Wrangler app.
After you shut down the Data Wrangler app, it has to restart the next time you open a Data Wrangler flow file. This can take a few minutes.
Conclusion
In this post, we demonstrated how you can use the new sample dataset on Data Wrangler to explore Data Wrangler’s features without needing to bring your own data. We also presented two additional features: the loading page to let you visually track the progress of the data being imported into Data Wrangler, and product suggestions that provide useful tips to get started with Data Wrangler. We went further to show how you can create SageMaker processing jobs and launch Autopilot experiments directly from the Data Wrangler user interface.
To learn more about using data flows with Data Wrangler, refer to Create and Use a Data Wrangler Flow and Amazon SageMaker Pricing. To get started with Data Wrangler, see Prepare ML Data with Amazon SageMaker Data Wrangler. To learn more about Autopilot and AutoML on SageMaker, visit Automate model development with Amazon SageMaker Autopilot.
About the authors
David Laredo is a Prototyping Architect at AWS Envision Engineering in LATAM, where he has helped develop multiple machine learning prototypes. Previously, he worked as a Machine Learning Engineer and has been doing machine learning for over 5 years. His areas of interest are NLP, time series, and end-to-end ML.
Parth Patel is a Solutions Architect at AWS in the San Francisco Bay Area. Parth guides customers to accelerate their journey to the cloud and helps them adopt the AWS Cloud successfully. He focuses on ML and application modernization.
Reverse engineering the NTK: towards first-principles architecture design
Deep neural networks have enabled technological wonders ranging from voice recognition to machine transition to protein engineering, but their design and application is nonetheless notoriously unprincipled.
The development of tools and methods to guide this process is one of the grand challenges of deep learning theory.
In Reverse Engineering the Neural Tangent Kernel, we propose a paradigm for bringing some principle to the art of architecture design using recent theoretical breakthroughs: first design a good kernel function – often a much easier task – and then “reverse-engineer” a net-kernel equivalence to translate the chosen kernel into a neural network.
Our main theoretical result enables the design of activation functions from first principles, and we use it to create one activation function that mimics deep (textrm{ReLU}) network performance with just one hidden layer and another that soundly outperforms deep (textrm{ReLU}) networks on a synthetic task.
<!– –>
Kernels back to networks. Foundational works derived formulae that map from wide neural networks to their corresponding kernels. We obtain an inverse mapping, permitting us to start from a desired kernel and turn it back into a network architecture.
<!– –>