Voice trigger detection is an important task, which enables activating a voice assistant when a target user speaks a keyword phrase. A detector is typically trained on speech data independent of speaker information and used for the voice trigger detection task. However, such a speaker independent voice trigger detector typically suffers from performance degradation on speech from underrepresented groups, such as accented speakers. In this work, we propose a novel voice trigger detector that can use a small number of utterances from a target speaker to improve detection accuracy. Our proposed…Apple Machine Learning Research
Combining Compressions for Multiplicative Size Scaling on Natural Language Tasks
Quantization, knowledge distillation, and magnitude pruning are among the most popular methods for neural network compression in NLP. Independently, these methods reduce model size and can accelerate inference, but their relative benefit and combinatorial inter- actions have not been rigorously studied. For each of the eight possible subsets of these techniques, we compare accuracy vs. model size tradeoffs across six BERT architecture sizes and eight GLUE tasks. We find that quantization and distillation consistently provide greater benefit than pruning. Surprisingly, except for the pair of…Apple Machine Learning Research
Generative Multiplane Images: Making a 2D GAN 3D-Aware
What is really needed to make an existing 2D GAN 3D-aware? To answer this question, we modify a classical GAN, i.e., StyleGANv2, as little as possible. We find that only two modifications are absolutely necessary: 1) a multiplane image style generator branch which produces a set of alpha maps conditioned on their depth; 2) a pose-conditioned discriminator. We refer to the generated output as a ‘generative multiplane image’ (GMPI) and emphasize that its renderings are not only high-quality but also guaranteed to be view-consistent, which makes GMPIs different from many prior works. Importantly…Apple Machine Learning Research
Texturify: Generating Textures on 3D Shape Surfaces
Texture cues on 3D objects are key to compelling visual representations, with the possibility to create high visual fidelity with inherent spatial consistency across different views. Since the availability of textured 3D shapes remains very limited, learning a 3D-supervised data-driven method that predicts a texture based on the 3D input is very challenging. We thus propose Texturify, a GAN-based method that leverages a 3D shape dataset of an object class and learns to reproduce the distribution of appearances observed in real images by generating high-quality textures. In particular, our…Apple Machine Learning Research
Artificial intelligence model can detect Parkinson’s from breathing patterns
Parkinson’s disease is notoriously difficult to diagnose as it relies primarily on the appearance of motor symptoms such as tremors, stiffness, and slowness, but these symptoms often appear several years after the disease onset. Now, Dina Katabi, the Thuan (1990) and Nicole Pham Professor in the Department of Electrical Engineering and Computer Science (EECS) at MIT and principal investigator at MIT Jameel Clinic, and her team have developed an artificial intelligence model that can detect Parkinson’s just from reading a person’s breathing patterns.
The tool in question is a neural network, a series of connected algorithms that mimic the way a human brain works, capable of assessing whether someone has Parkinson’s from their nocturnal breathing — i.e., breathing patterns that occur while sleeping. The neural network, which was trained by MIT PhD student Yuzhe Yang and postdoc Yuan Yuan, is also able to discern the severity of someone’s Parkinson’s disease and track the progression of their disease over time.
Yang and Yuan are co-first authors on a new paper describing the work, published today in Nature Medicine. Katabi, who is also an affiliate of the MIT Computer Science and Artificial Intelligence Laboratory and director of the Center for Wireless Networks and Mobile Computing, is the senior author. They are joined by 12 colleagues from Rutgers University, the University of Rochester Medical Center, the Mayo Clinic, Massachusetts General Hospital, and the Boston University College of Health and Rehabilition.
Over the years, researchers have investigated the potential of detecting Parkinson’s using cerebrospinal fluid and neuroimaging, but such methods are invasive, costly, and require access to specialized medical centers, making them unsuitable for frequent testing that could otherwise provide early diagnosis or continuous tracking of disease progression.
The MIT researchers demonstrated that the artificial intelligence assessment of Parkinson’s can be done every night at home while the person is asleep and without touching their body. To do so, the team developed a device with the appearance of a home Wi-Fi router, but instead of providing internet access, the device emits radio signals, analyzes their reflections off the surrounding environment, and extracts the subject’s breathing patterns without any bodily contact. The breathing signal is then fed to the neural network to assess Parkinson’s in a passive manner, and there is zero effort needed from the patient and caregiver.
“A relationship between Parkinson’s and breathing was noted as early as 1817, in the work of Dr. James Parkinson. This motivated us to consider the potential of detecting the disease from one’s breathing without looking at movements,” Katabi says. “Some medical studies have shown that respiratory symptoms manifest years before motor symptoms, meaning that breathing attributes could be promising for risk assessment prior to Parkinson’s diagnosis.”
The fastest-growing neurological disease in the world, Parkinson’s is the second-most common neurological disorder, after Alzheimer’s disease. In the United States alone, it afflicts over 1 million people and has an annual economic burden of $51.9 billion. The research team’s device was tested on 7,687 individuals, including 757 Parkinson’s patients.
Katabi notes that the study has important implications for Parkinson’s drug development and clinical care. “In terms of drug development, the results can enable clinical trials with a significantly shorter duration and fewer participants, ultimately accelerating the development of new therapies. In terms of clinical care, the approach can help in the assessment of Parkinson’s patients in traditionally underserved communities, including those who live in rural areas and those with difficulty leaving home due to limited mobility or cognitive impairment,” she says.
“We’ve had no therapeutic breakthroughs this century, suggesting that our current approaches to evaluating new treatments is suboptimal,” says Ray Dorsey, a professor of neurology at the University of Rochester and Parkinson’s specialist who co-authored the paper. Dorsey adds that the study is likely one of the largest sleep studies ever conducted on Parkinson’s. “We have very limited information about manifestations of the disease in their natural environment and [Katabi’s] device allows you to get objective, real-world assessments of how people are doing at home. The analogy I like to draw [of current Parkinson’s assessments] is a street lamp at night, and what we see from the street lamp is a very small segment … [Katabi’s] entirely contactless sensor helps us illuminate the darkness.”
This research was performed in collaboration with the University of Rochester, Mayo Clinic, and Massachusetts General Hospital, and is sponsored by the National Institutes of Health, with partial support by the National Science Foundation and the Michael J. Fox Foundation.
An AI-Enabled Drone Could Soon Become Every Rhino Poacher’s… Horn Enemy
Want inspiration? Try being charged by a two-ton African black rhino.
Early in her career, wildlife biologist Zoe Jewell and her team came across a mother rhino and her calf and carefully moved closer to get a better look.
The protective mother rhino charged, chasing Jewell across the dusty savannah. Eventually, Jewell got a flimsy thorn bush between herself and the rhino. Her heart was racing.
“I thought to myself, ‘There has to be a better way,’” she said.
In the latest example of how researchers like Jewell are using the latest technologies to track animals less invasively, a team of researchers has proposed harnessing high-flying AI-equipped drones powered by the NVIDIA Jetson edge AI platform to track the endangered black rhino through the wilds of Namibia.
In a paper published this month in the journal PeerJ, the researchers show the potential of drone-based AI to identify animals in even the remotest areas and provide real-time updates on their status from the air.
For more, read the full paper at https://peerj.com/articles/13779/.
While drones — and technology of just about every kind — have been harnessed to track African wildlife, the proposal promises to help gamekeepers move faster to protect rhinos and other megafauna from poachers.
“We have to be able to stay one step ahead,” said Jewell, co-founder of WildTrack, a global network of biologists and conservationists dedicated to non-invasive wildlife monitoring techniques.
Jewell, president and co-founder of WildTrack, has a B.Sc. in Zoology/Physiology, an M.Sc in Medical Parasitology from the London School of Tropical Medicine and Hygiene and a veterinary medical degree from Cambridge University. She has long sought to find less invasive ways to track, and protect, endangered species, such as the African black rhino.
In addition to Jewell, the paper’s authors include conservation biology and data science specialists at UC Berkeley, the University of Göttingen in Germany, Namibia’s Kuzikus Wildlife Reserve and Duke University.
The stakes are high.
African megafauna have become icons, even as global biodiversity declines.
“Only 5,500 black rhinos stand between this magnificent species, which preceded humans on earth by millions of years, and extinction,” Jewell says.
That’s made them bigger targets for poachers, who sell rhino horns and elephant tusks for huge sums, the paper’s authors report. Rhino horns, for example, reportedly go for as much as $65,000 per kilogram.
To disrupt poaching, wildlife managers must deploy effective protection measures.
This, in turn, depends on getting reliable data fast.
The challenge: many current monitoring technologies are invasive, expensive or impractical.
Satellite monitoring is a potential tool for the biggest animals — such as elephants. But detecting smaller species requires higher resolution imaging.
And the traditional practice of capturing rhinos, attaching a radio collar to the animals and then releasing them can be stressful for humans and rhinos.
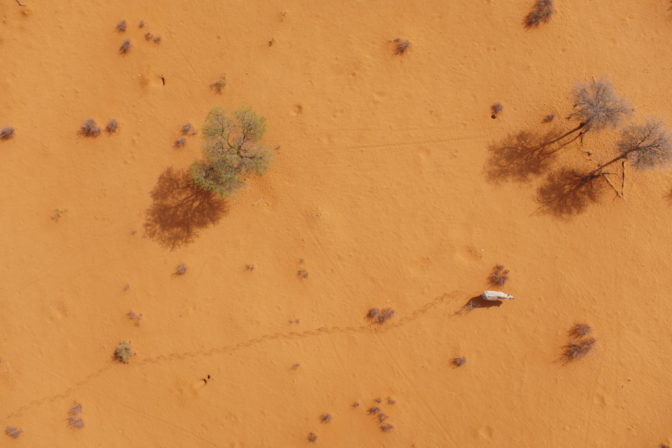
It’s even been found to depress the fertility of captured rhinos.
High-flying drones are already being used to study wildlife unobtrusively.
But rhinos most often live in areas with poor wireless networks, so drones can’t stream images back in real-time.
As a result, images have to be downloaded when drones return to researchers, who then have to comb through images looking to identify the beasts.
Identifying rhinos instantly onboard a drone and alerting authorities before it lands would ensure a speedy response to poachers.
“You can get a notification out and deploy units to where those animals are straight away,” Jewell said. “You could even protect these animals at night using heat signatures.”
To do this, the paper’s authors propose using an NVIDIA Jetson Xavier NX module onboard a Parrot Anafi drone.
The drone can connect to the relatively poor-quality wireless networks available in areas where rhinos live and deliver notifications whenever the target species are spotted.
To build the drone’s AI, the researchers used a YOLOv5l6 object-detection architecture. They trained it to identify a bounding box for one of five objects of interest in a video frame.
Most of the images used for training were gathered in Namibia’s Kuzikus Wildlife Reserve, an area of roughly 100 square kilometers on the edge of the Kalahari desert.
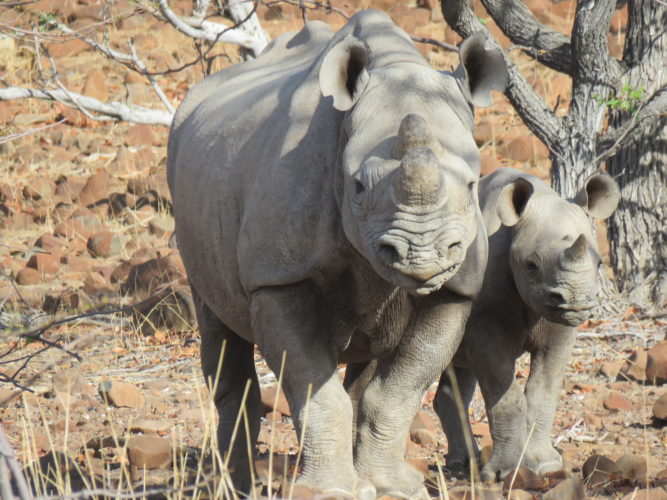
With tourists gone, Jewell reports that her colleagues in Namibia had plenty of time to gather training images for the AI.
The researchers used several technologies to optimize performance and overcome the challenge of small animals in the data.
These techniques included images of other species in the AI’s training data, emulating field conditions with many animals.
They used data augmentation techniques, such as generative adversarial networks, to train the AI on synthetic data, the paper’s authors wrote.
And they also trained the model on a dataset with many kinds of terrain and images taken from different angles and lighting conditions.
Looking at footage of rhinos gathered in the wild, the AI correctly identified black rhinos — the study’s primary target — 81 percent of the time and giraffes 83 percent of the time, they reported.
The next step: putting this system to work in the wild, where wildlife conversationalists are already deploying everything from cameras to radio collars to track rhinos.
Many of the techniques combine the latest technology with ancient practices.
Jewell and WildTrack co-founder Sky Alibhai have already created a system, FIT, that uses sophisticated new techniques to analyze animal tracks (see image of a rhino track, left). The software, initially developed using morphometrics — or the quantitative analysis of an animal’s form — on JMP statistical analysis software, now uses the latest AI techniques.
Jewell says that modern science and the ancient art of tracking are much more alike than you might think.
“’When you follow a footprint, you’re really recreating the origins of science that shaped humanity,” Jewell said. “You’re deciding who made that footprint, and you’re following a trail to see if you’re correct.”
Jewell and her colleagues are now working to take their work another step forward, to use drones to identify rhino trails in the environment.
“Without even seeing them on the ground we’ll be able to create a map of where they’re going and interacting with each other to help us understand how to best protect them,” Jewell says.
All Images courtesy of WildTrack
The post An AI-Enabled Drone Could Soon Become Every Rhino Poacher’s… Horn Enemy appeared first on NVIDIA Blog.
Announcing the winners of the 2022 Network for AI request for proposals
In April, Meta launched the Network for AI request for proposals (RFP). Today, we’re announcing the winners of this award.Read More
Ozge Sahin on the art and science of studying consumer behavior
The Johns Hopkins business school professor and Amazon Scholar focuses on enhancing customer experiences.Read More
Best practices for TensorFlow 1.x acceleration training on Amazon SageMaker
Today, a lot of customers are using TensorFlow to train deep learning models for their clickthrough rate in advertising and personalization recommendations in ecommerce. As the behavior of their clients change, they can accumulate large amounts of new data every day. Model iteration is one of a data scientist’s daily jobs, but they face the problem of taking too long to train on large datasets.
Amazon SageMaker is a fully managed machine learning (ML) platform that could help data scientists focus on models instead of infrastructure, with native support for bring-your-own-algorithms and frameworks such as TensorFlow and PyTorch. SageMaker offers flexible distributed training options that adjust to your specific workflows. Because many data scientists may lack experience in the acceleration training process, in this post we show you the factors that matter for fast deep learning model training and the best practices of acceleration training for TensorFlow 1.x on SageMaker. We also have a sample code of DeepFM distributed training on SageMaker on the GitHub repo.
There are many factors you should consider to maximize CPU/GPU utilization when you run your TensorFlow script on SageMaker, such as infrastructure, type of accelerator, distributed training method, data loading method, mixed precision training, and more.
We discuss best practices in the following areas:
- Accelerate training on a single instance
- Accelerate training on multiple instances
- Data pipelines
- Automatic mixed precision training
Accelerate training on a single instance
When running your TensorFlow script on a single instance, you could choose a computer optimized series such as the Amazon Elastic Compute Cloud (Amazon EC2) C5 series, or an accelerated computing series with multiple GPU in a single instance such as p3.8xlarge, p3.16xlarge, p3dn.24xlarge, and p4d.24xlarge.
In this section, we discuss strategies for multiple CPUs on a single instance, and distributed training with multiple GPUs on a single instance.
Multiple CPUs on a single instance
In this section, we discuss manually setting operators’ parallelism on CPU devices, the tower method, TensorFlow MirroredStrategy, and Horovod.
Manually setting operators’ parallelism on CPU devices
TensorFlow automatically selects the appropriate number of threads to parallelize the operation calculation in the training process. However, you could set the intra_op
threads pool and inter_op
parallelism settings provided by TensorFlow and use environment variables of MKL-DNN to set binding for the OS thread. See the following code:
The environment variable KMP_AFFINITY
of MKL-DNN is set to granularity=fine,compact,1,0
by default. After setting both intra and inter of TensorFlow to the maximum number of vCPUs of the current instance, the upper limit of CPU usage is almost the same as the number of physical cores of the training instance.
If you set os.environ["KMP_AFFINITY"]= "verbose,disabled"
, the OS thread isn’t bound to the hardware hyper thread, and CPU usage could exceed the number of physical cores.
Regarding the settings of TensorFlow intra parallelism, TensorFlow inter parallelism, and the number of MKL-DNN threads, different combinations of these three parameters result in different training speeds. Therefore, you need to test each case to find the best combination. A common situation is to set the three parameters (intra_op_parallelism_threads
and inter_op_parallelism_threads
for TensorFlow, os.environ['OMP_NUM_THREADS']
for MKL-DNN) to half the number of vCPUs (physical core) or the total number of vCPUs.
Tower method
To replicate a model over GPUs, each GPU gets its own instance of the forward pass. The instance of the forward pass is called a tower. The tower method is almost always used for GPU devices. To compare training speed with other methods, here we also use the tower method for our CPU device.
If you don’t set the CPU device manually, TensorFlow don’t use the tower method to average the gradients, so you don’t need to scale the batch size in such cases.
- Set the CPU device manually:
- Use
replicate_model_fn
to wrapmodel_fn
:
- Use
TowerOptimizer
to wrapoptimizer
:
- Wrap your
model_fn
:
- Scale batch size to (NUM_CPU – 1).
Let’s look at the difference of CPU utilization with tower mode enabled. The following figure shows ml.c5.18xlarge instance’s CPU utilization with the following configuration:
No Tower + LibSVM data + pipe mode + MKL-DNN disable binding + TensorFlow intra/inter op parallelism setting to max number of instance’s vCPUs
The following figure shows the ml.c5.18xlarge instance’s CPU utilization with the following configuration:
Tower with set CPU device + LibSVM data + pipe mode + MKL-DNN disable binding + TensorFlow intra/inter op parallelism setting to max number of instance’s vCPUs
The CPU usage is higher when using the tower method, and it exceeds the number of physical cores.
TensorFlow MirroredStrategy
TensorFlow MirroredStrategy means synchronous training across multiple replicas on one machine. This strategy is typically used for training on one machine with multiple GPUs. To compare training speed with another method, we use MirroredStrategy for our CPU device.
When using TensorFlow MirroredStrategy, if you don’t set the CPU device, TensorFlow just uses one CPU as single worker, which is a waste of resources. We recommend manually setting the CPU device, because it will do a reduce operation on /CPU:0
, so the /CPU:0
device isn’t used as a replica here. See the following code:
You need to scale batch size when using MirroredStrategy; for example, scale the batch size to a multiple of the number of GPU devices.
For the sub-strategy when you set CPU device, if you don’t set the cross_device_ops
parameter in tf.distribute.MirroredStrategy()
, TensorFlow uses the ReductionToOneDevice
sub-strategy by default. However, if you set HierarchicalCopyAllReduce
as the sub-strategy, TensorFlow just does the reduce work on /CPU:0
. When you use the TensorFlow dataset API and distribute strategy together, the dataset object should be returned instead of features and labels in function input_fn
.
Usually, TensorFlow MirroredStrategy is slower than the tower method on CPU training, so we don’t recommend using MirroredStrategy on a multi-CPU single host.
Horovod
Horovod is a distributed deep learning training framework for TensorFlow, Keras, PyTorch, and Apache MXNet. The goal of Horovod is to make distributed deep learning fast and easy to use.
There is a parameter of distribution
in the SageMaker Python SDK Estimator API, which you could use to state the Horovod distributed training. SageMaker provisions the infrastructure and runs your script with MPI. See the following code:
When choosing a GPU instance such as ml.p3.8xlarge, you need to pin each GPU for every worker:
To speed up model convergence, scale the learning rate by the number of workers according to the Horovod official documentation. However, in real-world projects, you should scale the learning rate to some extent, but not by the number of workers, which results in bad model performance. For example, if the original learning rate is 0.001, we scale the learning rate to 0.0015, even if number of workers is four or more.
Generally, only the primary (Horovod rank 0) saves the checkpoint and model as well as the evaluation operation. You don’t need to scale the batch size when using Horovod. SageMaker offers Pipe mode to stream data from Amazon Simple Storage Service (Amazon S3) into training instances. When you enable Pipe mode, be aware that different workers on the same host need to use different channels to avoid errors. This is because the first worker process reads the FIFO/channel data, and other worker processes on the same instance will hang because they can’t read data from the same FIFO/channel, so Horovod doesn’t work properly. To avoid this issue, set the channels according to the number of workers per instance. At least make sure that different workers on the same host consume different channels; the same channel can be consumed by workers on a different host.
When using Horovod, you may encounter the following error:
The possible cause for this issue is that a certain rank (such as rank 0) works slower or does more jobs than other ranks, and this causes other ranks to wait for a long time. Although rank 0 sometimes has to do more work than other ranks, it should be noted that rank 0 shouldn’t do much for a long time. For example, for the model evaluation on the validation set and saving checkpoints during training, if it’s inevitable that these operations will take a long time, which could cause errors, one workaround is to let all workers do the same work as rank 0 (checkpoints saving, evaluation, and so on).
Data sharding is one of the most important things to consider when using distributed training. You can use TensorFlow dataset.shard()
in your script. SageMaker also offers a dataset shard feature in the inputs channel by setting distribution=S3shardbykey
in the dataset channel. See the following code:
The following figure shows the result when using Horovod (ml.c5.18xlarge, Horovod + LibSVM + default intra op and inter op setting), which you can compare to the tower method.
Distributed training with multiple GPUs on a single instance
It’s normal to start distributed training with multiple GPUs on a single instance because data scientists only need to manage one instance and take advantage of the high-speed interlink between GPUs. SageMaker training jobs support multiple instance types that have multiple GPUs on a single instance, such as ml.p3.8xlarge, ml.p3.16xlarge, ml.p3dn.24xlarge, and ml.p4d.24xlarge. The method is the same as multiple CPUs in a single instance, but with a few changes in the script.
Tower method
The tower method here is almost the same as in multi-CPU training. You need to scale the batch size according to the number of GPUs in use.
TensorFlow MirroredStrategy
The default sub-strategy of MirroredStrategy
is NcclAllReduce
. You need to scale the batch size according to the number of GPUs in use. See the following code:
Accelerate training on multiple instances
Scaling out is always an option to improve training speed. More and more data scientists choose this as a default option in regards to distributed training. In this section, we discuss strategies for distributed training with multiple hosts.
Multiple CPUs with multiple instances
There are four main methods for using multiple CPUs with multiple instances when enabling distributed training:
-
- Parameter server without manually setting operators’ parallelism on CPU devices
- Parameter server with manually setting operators’ parallelism on CPU devices
- Parameter server with tower (setting CPU devices manually, and set
allow_soft_placement=True in tf.ConfigProto
) - Horovod
When using a parameter server in the tf.estimator
API, the path of checkpoint must be a sharable path such as Amazon S3 or the local path of Amazon Elastic File Service (Amazon EFS) mapping to the container. For a parameter server in tf.keras
, the checkpoint path can be set to the local path. For Horovod, the checkpoint path can be set to a local path of the training instance.
When using a parameter server and the tf.estimator
API with the checkpoint path to Amazon S3, if the model is quite large, you might encounter an error of the primary is stuck at saving checkpoint to S3. You can use SageMaker built-in container TensorFlow 1.15 or TensorFlow 1.15.2 or use Amazon EFS as the checkpoint path of the share.
When using a parameter server for multiple hosts, the parameter load on each parameter server process may be unbalanced (especially when there are relatively large embedding table variables), which could cause errors. You could check the file size of each the shard’s checkpoint in Amazon S3 to determine whether the parameters on the parameter server are balanced, because each parameter server corresponds to a shard of the checkpoint file. To avoid such issues, you can use the partitioner function to try to make the parameters of each parameter server evenly distributed:
Single GPU with multiple instances
SageMaker training jobs support instances that only have one GPU, like the ml.p3.xlarge, ml.g4dn, and ml.g5 series. There are two main methods used in this scenario: parameter servers and Horovod.
The built-in parameter server distributed training method of SageMaker is to start a parameter server process and a worker process for each training instance (each parameter server is only responsible for part of the model parameters), so the default is multi-machine single-GPU training. The SageMaker built-in parameter server distributed training is an asynchronous gradient update method. To reduce the impact of asynchronous updates on training convergence, it’s recommended to reduce the learning rate. If you want to use all the GPUs on the instance, you need to use a combination of parameter servers and the tower method.
For Horovod, just set processes_per_host=1
in the distribution parameter of the SageMaker Python Estimator API.
Multiple GPUs with multiple instances
For parameter servers and the tower method, the code changes are basically the same as the tower method for a single instance with multiple GPUs, and there is no need to manually set the GPU devices.
For Horovod, set processes_per_host in the distribution parameter to the number of GPUs of each training instance. If you use Pipe mode, the number of workers per instance needs to match the number of channels.
Data pipelines
In addition to the infrastructure we have discussed, there is another important thing to consider: the data pipeline. A data pipeline refers to how you load data and transform data before it feeds into neural networks. CPU is used to prepare data, whereas GPU is used to calculate the data from CPU. Because GPU is an expensive resource, more GPU idle time is inefficient; a good data pipeline in your training job could improve GPU and CPU utilization.
When you’re trying to optimize your TensorFlow data input pipeline, consider the API order used in TensorFlow datasets, the training data size (a lot of small files or several large files), batch size, and so on.
Let’s look at the interaction between GPU and CPU during training. The following figures compare interactions with and without a pipeline.
A better pipeline could reduce GPU idle time. Consider the following tips:
- Use simple function logic in extracting features and labels
- Prefetch samples to memory
- Reduce unnecessary disk I/O and networking I/O
- Cache the processed features and labels in memory
- Reduce the number of replication times between CPU and GPU
- Have different workers deal with different parts of the training dataset
- Reduce the times of calling the TensorFlow dataset API
TensorFlow provides a transform API related to dataset formats, and the order of the transformation API in TensorFlow affects training speed a lot. The best order of calling the TensorFlow dataset API needs to be tested. The following are some basic principles:
- Use a vectorized map. This means call the TensorFlow dataset batch API first, then the dataset map API. The custom parsing function provided in the map function, such as
decode_tfrecord
in the sample code, parses a mini batch of data. On the contrary, map first and then batch is a scalar map, and the custom parser function processes just one sample. - Use the TensorFlow dataset cache API to cache features and labels. Put the TensorFlow dataset cache API before the TensorFlow dataset repeat API, otherwise RAM utilization increases linearly epoch by epoch. If the dataset is as large as RAM, don’t use the TensorFlow dataset cache API. If you need to use the TensorFlow dataset cache API and shuffle API, consider use the following order: create TensorFlow dataset object -> cache API -> shuffle API -> batch API -> map API -> repeat API -> prefetch API.
- Use the
tfrecord
dataset format more than LibSVM format. - File mode or Pipe mode depends on your dataset format and amount of files. The
tfrecorddataset
API can setnum_parallel_reads
to read multiple files in parallel and setbuffer_size
to optimize data’s reading, whereas thepipemodedataset
API doesn’t have such settings. Pipe mode is more suitable for situations where a single file is large and the total number of files is small. We recommend using a SageMaker processing job to do the preprocessing work, such as joining multiple files to a bigger file according to labels, using a sampling method to make the dataset more balanced, and shuffling the balanced dataset.
See the following code sample:
For training on CPU instances, setting parallelism of intra op
, inter op
, and the environment variable of MKL-DNN is a good starting point.
Automatic mixed precision training
The last thing we discuss is automatic mixed precision training, which can accelerate speed and result in model performance. As of this writing, Nvidia V100 GPU (P3 instance) and A100 (P4dn instance) support Tensor core. You can enable mixed precision training in TensorFlow when using those types of instances. Starting from version 1.14, TensorFlow has supported automatic mixed precision training. You can use the following statement to wrap your original optimizer:
If the model is small and utilization of GPU is low, there’s no advantage of automatic mixed precision training. If the model is large, automatic mixed precision training can accelerate training speed.
Conclusion
When you start your deep learning model training in SageMaker, consider the following tips to achieve a faster training speed:
- Try the multi-CPU, single-instance method or single-GPU, single-instance method first. If CPU/GPU utilization is very high (for example more than 90%), move to the next step.
- Try more CPUs in single host or more GPUs in single host. If utilization is near the maximum utilization of CPUs or GPUs, move to the next step.
- Try multiple CPUs or multiple GPUs with multiple hosts.
- You need to modify codes when using parameter servers or Horovod. The code modification isn’t the same for the TensorFlow session-based API,
tf.estimator
API, andtf.keras
API. A parameter server or Horovod may show different training speeds in different training cases and tasks, so try both methods if you have the time and budget to determine the best one.
Keep in mind the following advice:
- Check utilization before scaling, optimize your data pipeline, and make CPU and GPU overlap in the timeline.
- First scale up, then scale out.
- If you can’t increate your GPU utilization after all the methods, try CPU. There are many cases (especially for the clickthrough rate ranking model) where the total training time of CPU instance training is shorter and more cost-effective than GPU instance training.
We also have a code sample in the GitHub repo, where we show two samples of DeepFM distributed training on SageMaker. One is a TensorFlow parameter server on CPU instances, the other one is Horovod on GPU instances.
About the Authors
Yuhui Liang is a Sr. Machine Learning Solutions Architect. He’s focused on the promotion and application of machine learning, and deeply involved in many customers’ machine learning projects. He has a rich experience in deep learning distributed training, recommendation systems, and computational advertising.
Shishuai Wang is a Sr. Machine Learning Solutions Architect. He works with AWS customers to help them adopt machine learning on a large scale. He enjoys watching movies and traveling around the world.
Content moderation using machine learning: a dual approach
Posted by Jen Person, Developer Advocate
Being kind: a perennial problem
I’ve often wondered why anonymity drives people to say things that they’d never dare say in person, and it’s unfortunate that comment sections for videos and articles are so often toxic! If you’re interested in content moderation, you can use machine learning to help detect toxic posts which you consider for removal.
ML for web developers
Machine learning is a powerful tool for all sorts of natural language-processing tasks, including translation, sentiment analysis, and predictive text. But perhaps it feels outside the scope of your work. After all, when you’re building a website in JavaScript, you don’t have time to collect and validate data, train a model using Python, and then implement some backend in Python on which to run said model. Not that there’s anything wrong with Python–it’s just that, if you’re a web developer, it’s probably not your language of choice.
Fortunately, TensorFlow.js allows you to run your machine learning model on your website in everybody’s favorite language: JavaScript. Furthermore, TensorFlow.js offers several pre-trained models for common use cases on the web. You can add the power of ML to your website in just a few lines of code! There is even a pre-trained model to help you moderate written content, which is what we’re looking at today.
The text toxicity classifier ML model
There is an existing pretrained model that works well for content moderation: the TensorFlow.js text toxicity classifier model. With this model, you can evaluate text on different labels of unwanted content, including identity attacks, insults, and obscenity. You can try out the demo to see the classifier in action. I admit that I had a bit of fun testing out what sort of content would be flagged as harmful. For example:
I recommend stopping here and playing around with the text toxicity classifier demo. It’s a good idea to see what categories of text the model checks for and determine which ones you would want to filter from your own website. Besides, if you want to know what categories the above quote got flagged for, you’ll have to go to the demo to read the headings.
Once you’ve hurled sufficient insults at the text toxicity classifier model, come back to this blog post to find out how to use it in your own code.
A dual approach
This started as a single tutorial with client and server-side code, but it got a bit lengthy so I decided to split it up. Separating the tutorials also makes it easier to target the part that interests you if you just want to implement one part. In this post, I cover the implementation steps for client-side moderation with TensorFlow.js using a basic website. In part 2, I show how to implement the same model server-side using Cloud Functions for Firebase.
Client-side moderation
Moderating content client-side provides a quicker feedback loop for your users, allowing you to stop harmful discourse before it starts. It can also potentially save on backend costs since inappropriate comments don’t have to be written to the database, evaluated, and then subsequently removed.
Starter code
I used the Firebase text moderation example as the foundation of my demo website. It looks like this:
Keep in mind TensorFlow.js doesn’t require Firebase. You can use whatever hosting, database, and backend solutions that work best for your app’s needs. I just tend to use Firebase because I’m pretty familiar with it already. And quite frankly, TensorFlow.js and Firebase work well together! The website in the Firebase demo showcases content moderation through a basic guestbook using a server-side content moderation system implemented through a Realtime Database-triggered Cloud Function. Don’t worry if this sounds like a lot of jargon. I’ll walk you through the specifics of what you need to know to use the TensorFlow.js model in your own code. That being said, if you want to build this specific example I made, it’s helpful to take a look at the Firebase example on GitHub.
If you’re building the example with me, clone the Cloud Functions samples repo. Then change to the directory of the text moderation app.
cd text–moderation |
This project requires you to have the Firebase CLI installed. If you don’t have it, you can install it using the following npm command:
npm install –g firebase–tools |
Once installed, use the following command to log in:
firebase login |
Run this command to connect the app to your Firebase project:
firebase use —add |
From here, you can select your project in the list, connect Firebase to an existing Google Cloud project, or create a new Firebase project. Once the project is configured, use the following command to deploy Realtime Database security rules and Firebase Hosting:
firebase deploy —only database,hosting |
There is no need to deploy Cloud Functions at this time since we will be changing the sample code entirely.
Note that the Firebase text moderation sample as written uses the Blaze (pay as you go) plan for Firebase. If you choose to follow this demo including the server-side component, your project might need to be upgraded from Spark to Blaze. If you have a billing account set on your project through Google Cloud, you are already upgraded and good to go! Most importantly, if you’re not ready to upgrade your project, then do not deploy the Cloud Functions portion of the sample. You can still use the client-side moderation without Cloud Functions.
To implement client-side moderation in the sample, I added some code to the index.html
and main.js
files in the Firebase text moderation example. There are three main steps to implement when using a TensorFlow.js model: installing the required components, loading the model, and then running the prediction. Let’s add the code for each of these steps.
Install the scripts
Add the required TensorFlow.js dependencies. I added the dependencies as script tags in the HTML, but you can use Node.js if you use a bundler/transpiler for your web app.
<!– index.html –> <!– scripts for TensorFlow.js –> <script src=“https://cdn.jsdelivr.net/npm/@tensorflow/tfjs/dist/tf.min.js”> </script> <script src=“https://cdn.jsdelivr.net/npm/@tensorflow-models/toxicity”></script> |
Load the model
Add the following code to load the text toxicity model in the Guestbook()
function. The Guestbook()
function is part of the original Firebase sample. It initializes the Guestbook
components and is called on page load.
// main.js // Initializes the Guestbook. function Guestbook() {
// The minimum prediction confidence. const threshold = 0.9; // Load the model. Users optionally pass in a threshold and an array of // labels to include. toxicity.load(threshold).then(model => { toxicity_model = model; }); //… |
The threshold
of the model is the minimum prediction confidence you want to use to set the model’s predictions to true
or false
–that is, how confident the model is that the text does or does not contain the given type of toxic content. The scale for the threshold is 0-1.0. In this case, I set the threshold to .9, which means the model will predict true
or false
if it is 90% confident in its findings. It is up to you to decide what threshold works for your use case. You may even want to try out the text toxicity classifier demo with some phrases that could come up on your website to determine how the model handles them.
toxicity.load
loads the model, passing the threshold. Once loaded, it sets toxicity_model
to the model
value.
Run the prediction
Add a checkContent
function that runs the model predictions on messages upon clicking “Add message”:
// main.js Guestbook.checkContent = function(message) { if (!toxicity_model) { console.log(‘no model found’); return false; }
const messages = [message];
return toxicity_model.classify(messages).then(predictions => {
for (let item of predictions) { for (let i in item.results) { console.log(item.results[i].match) if (item.results[i].match === true) { console.log(‘toxicity found’); return true; } } } console.log(‘no toxicity found’); return false; }); } |
This function does the following:
- Verifies that the model load has completed. If
toxicity_model
has a value, then theload()
function has finished loading the model. - Puts the message into an array called
messages
, as an array is the object type that theclassify
function accepts. - Calls
classify
on themessages
array. - Iterates through the prediction results.
predictions
is an array of objects each representing a different language label. You may want to know about only specific labels rather than iterating through them all. For example, if your use case is a website for hosting the transcripts of rap battles, you probably don’t want to detect and remove insults. - Checks if the content is a match for that label. if the
match
value istrue
, then the model has detected the given type of unwanted language. If the unwanted language is detected, the function returns true. There’s no need to keep checking the rest of the results, since the content has already been deemed inappropriate. - If the function iterates through all the results and no label match is set to
true
, then the function returnsfalse
– meaning no undesirable language was found. The match label can also benull
. In that case, its value isn’ttrue
, so it’s considered acceptable language. I will talk more about thenull
option in a future post.
Add a call to the checkContent
in the saveMessage
function:
// main.js // Saves a new message on the Firebase DB. Guestbook.prototype.saveMessage = function(e) { e.preventDefault(); if (!this.messageInput.value || !this.nameInput.value) { return; }
Guestbook.checkContent(this.messageInput.value).then((toxic) => { if (toxic === true) { // display a message to the user to be kind Guestbook.displaySnackbar(); // clear the message field Guestbook.resetMaterialTextfield(this.messageInput); return; } //… |
After a couple quick checks for input values, the contents of the message box is passed to the checkContent
function.
If the content passes this check, the message is written to the Realtime Database. If not, a snack bar displays reminding the message author to be kind. The snack bar isn’t anything special, so I’m not going to include the code here. You can see it in the full example code, or implement a snack bar of your own.
Try it out
If you’ve been following along in your own code, run this terminal command in your project folder to deploy the website:
firebase deploy –only hosting |
Using client-side moderation like this could catch most issues before they occur. But a clever user might open developer tools and try to find a way to write obscenities directly to the database, circumventing the content check. That’s where server-side moderation comes in.
If you enjoyed this article and would like to learn more about TensorFlow.js, here are some things you can do:
- Check out the TensorFlow.js edX course by Jason Mayes. If you are even remotely interested in using TensorFlow.js, I cannot recommend this enough. It might look like a lot at first, but the course is broken up into easy-to-follow manageable pieces.
- View all the TensorFlow.js pretrained models in the TFJS repository on GitHub.
- Play around with TensorFlow.js projects on Glitch.
- To see an example of ML image moderation on the web, try out Gant Laborde’s NSFW TFJS image checker.