In this post, we demonstrate how to use Amazon Polly—a leading cloud service that converts text into lifelike speech—to read the content of a webpage and highlight the content as it’s being read. Adding audio playback to a webpage improves the accessibility and visitor experience of the page. Audio-enhanced content is more impactful and memorable, draws more traffic to the page, and taps into the spending power of visitors. It also improves the brand of the company or organization that publishes the page. Text-to-speech technology makes these business benefits attainable. We accelerate that journey by demonstrating how to achieve this goal using Amazon Polly.
This capability improves accessibility for visitors with disabilities, and could be adopted as part of your organization’s accessibility strategy. Just as importantly, it enhances the page experience for visitors without disabilities. Both groups have significant spending power and spend more freely from pages that use audio enhancement to grab their attention.
Overview of solution
PollyReadsThePage
(PRTP)—as we refer to the solution—allows a webpage publisher to drop an audio control onto their webpage. When the visitor chooses Play on the control, the control reads the page and highlights the content. PRTP uses the general capability of Amazon Polly to synthesize speech from text. It invokes Amazon Polly to generate two artifacts for each page:
- The audio content in a format playable by the browser: MP3
- A speech marks file that indicates for each sentence of text:
- The time during playback that the sentence is read
- The location on the page the sentence appears
When the visitor chooses Play, the browser plays the MP3 file. As the audio is read, the browser checks the time, finds in the marks file which sentence to read at that time, locates it on the page, and highlights it.
PRTP allows the visitor to read in different voices and languages. Each voice requires its own pair of files. PRTP uses neural voices. For a list of supported neural voices and languages, see Neural Voices. For a full list of standard and neural voices in Amazon Polly, see Voices in Amazon Polly.
We consider two types of webpages: static and dynamic pages. In a static page, the content is contained within the page and changes only when a new version of the page is published. The company might update the page daily or weekly as part of its web build process. For this type of page, it’s possible to pre-generate the audio files at build time and place them on the web server for playback. As the following figure shows, the script PRTP Pre-Gen
invokes Amazon Polly to generate the audio. It takes as input the HTML page itself and, optionally, a configuration file that specifies which text from the page to extract (Text Extract Config
). If the extract config is omitted, the pre-gen script makes a sensible choice of text to extract from the body of the page. Amazon Polly outputs the files in an Amazon Simple Storage Service (Amazon S3) bucket; the script copies them to your web server. When the visitor plays the audio, the browser downloads the MP3 directly from the web server. For highlights, a drop-in library, PRTP.js
, uses the marks file to highlight the text being read.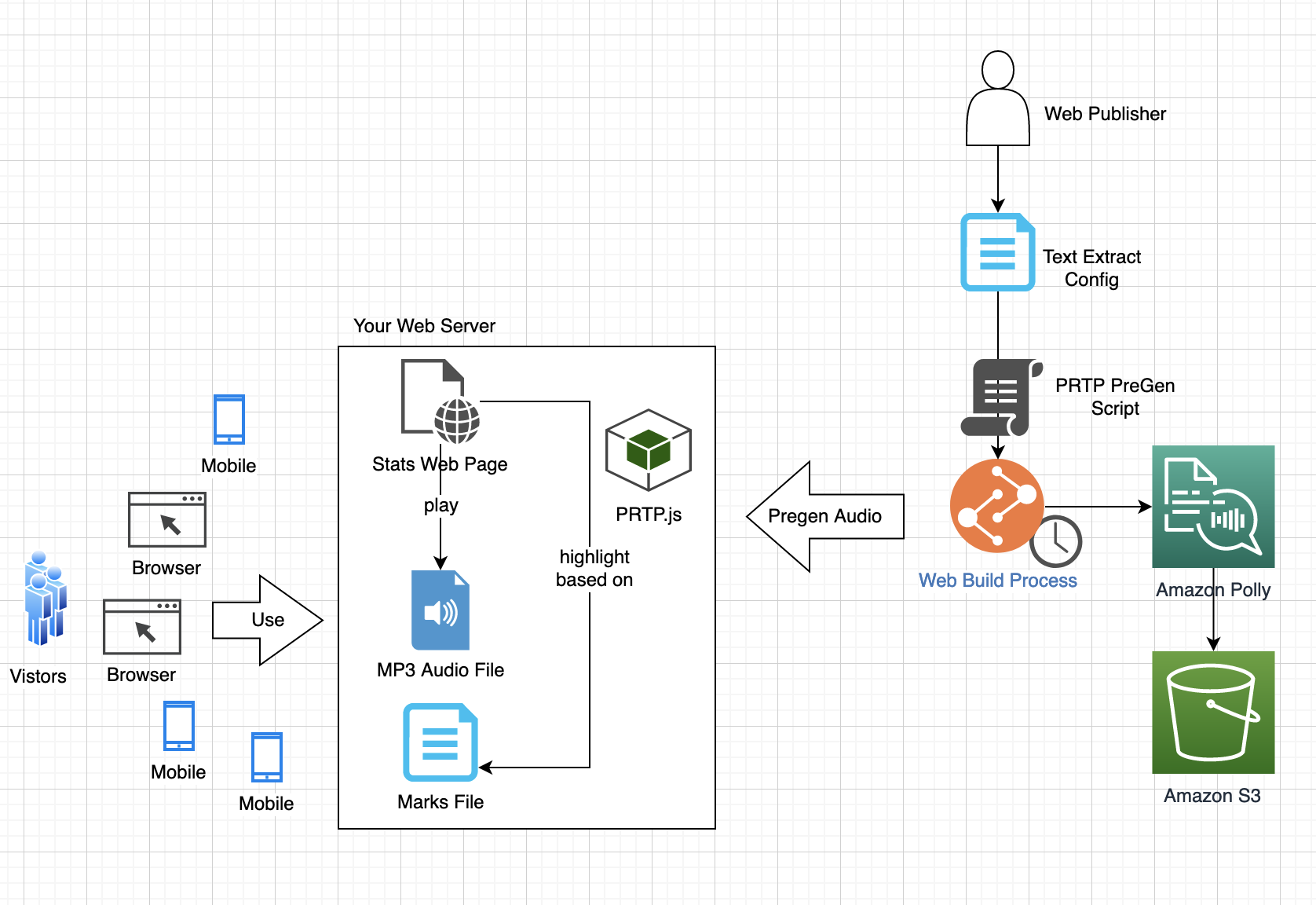
The content of a dynamic page changes in response to the visitor interaction, so audio can’t be pre-generated but must be synthesized dynamically. As the following figure shows, when the visitor plays the audio, the page uses PRTP.js
to generate the audio in Amazon Polly, and it highlights the synthesized audio using the same approach as with static pages. To access AWS services from the browser, the visitor requires an AWS identity. We show how to use an Amazon Cognito identity pool to allow the visitor just enough access to Amazon Polly and the S3 bucket to render the audio.
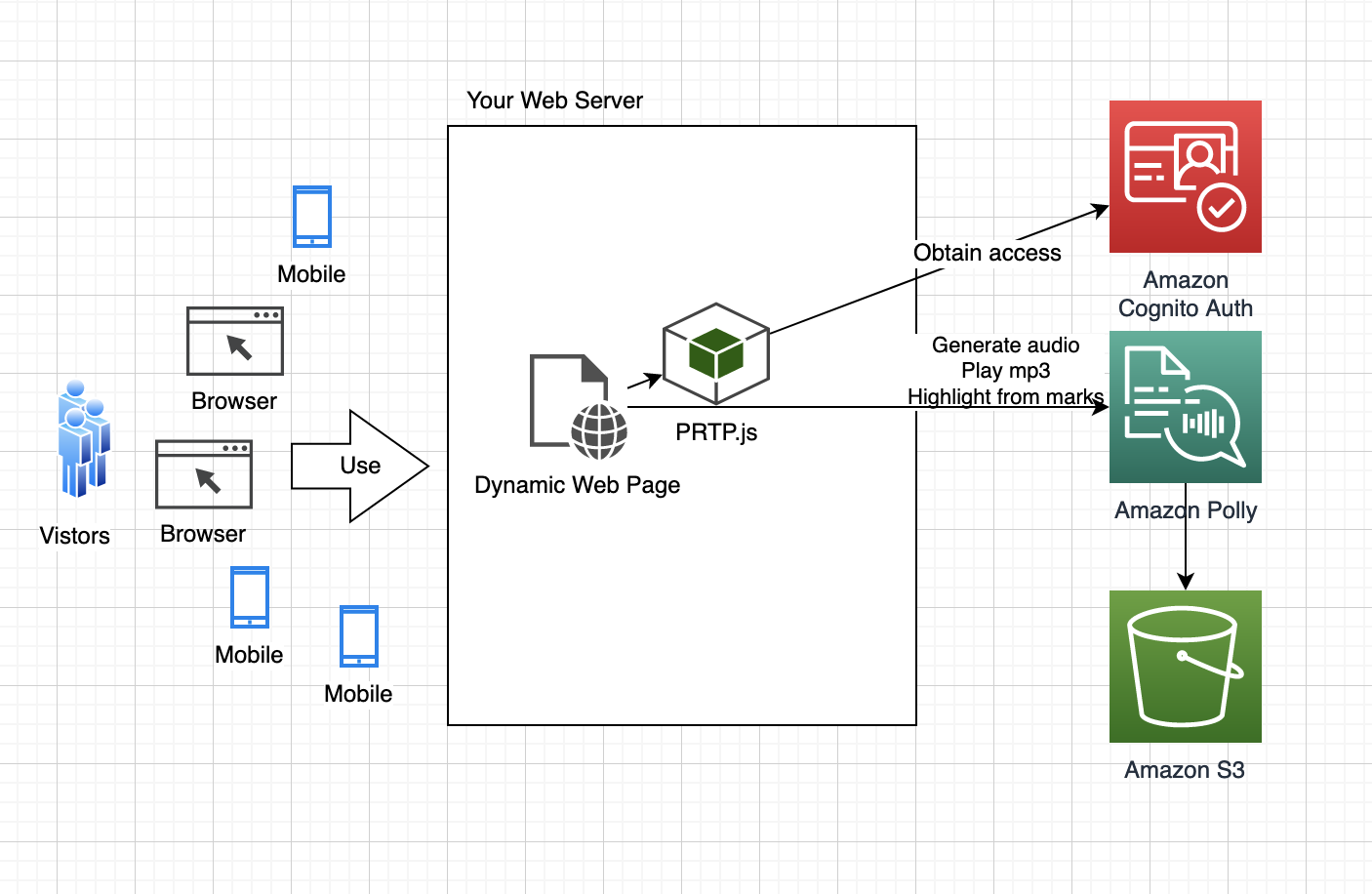
Generating both Mp3 audio and speech marks requires the Polly service to synthesize the same input twice. Refer to the Amazon Polly Pricing Page to understand cost implications. Pre-generation saves costs because synthesis is performed at build time rather than on-demand for each visitor interaction.
The code accompanying this post is available as an open-source repository on GitHub.
To explore the solution, we follow these steps:
- Set up the resources, including the pre-gen build server, S3 bucket, web server, and Amazon Cognito identity.
- Run the static pre-gen build and test static pages.
- Test dynamic pages.
Prerequisites
To run this example, you need an AWS account with permission to use Amazon Polly, Amazon S3, Amazon Cognito, and (for demo purposes) AWS Cloud9.
Provision resources
We share an AWS CloudFormation template to create in your account a self-contained demo environment to help you follow along with the post. If you prefer to set up PRTP in your own environment, refer to instructions in README.md.
To provision the demo environment using CloudFormation, first download a copy of the CloudFormation template. Then complete the following steps:
- On the AWS CloudFormation console, choose Create stack.
- Choose With new resources (standard).
- Select Upload a template file.
- Choose Choose file to upload the local copy of the template that you downloaded. The name of the file is
prtp.yml
.
- Choose Next.
- Enter a stack name of your choosing. Later you enter this again as a replacement for <StackName>.
- You may keep default values in the Parameters section.
- Choose Next.
- Continue through the remaining sections.
- Read and select the check boxes in the Capabilities section.
- Choose Create stack.
- When the stack is complete, find the value for
BucketName
in the stack outputs.
We encourage you to review the stack with your security team prior to using it a production environment.
Set up the web server and pre-gen server in an AWS Cloud9 IDE
Next, on the AWS Cloud9 console, locate the environment PRTPDemoCloud9
created by the CloudFormation stack. Choose Open IDE to open the AWS Cloud9 environment. Open a terminal window and run the following commands, which clones the PRTP code, sets up pre-gen dependencies, and starts a web server to test with:
#Obtain PRTP code
cd /home/ec2-user/environment
git clone https://github.com/aws-samples/amazon-polly-reads-the-page.git
# Navigate to that code
cd amazon-polly-reads-the-page/setup
# Install Saxon and html5 Python lib. For pre-gen.
sh ./setup.sh <StackName>
# Run Python simple HTTP server
cd ..
./runwebserver.sh <IngressCIDR>
For <StackName>, use the name you gave the CloudFormation stack. For <IngressCIDR>, specify a range of IP addresses allowed to access the web server. To restrict access to the browser on your local machine, find your IP address using https://whatismyipaddress.com/ and append /32
to specify the range. For example, if your IP is 10.2.3.4, use 10.2.3.4/32
. The server listens on port 8080. The public IP address on which the server listens is given in the output. For example:
Test static pages
In your browser, navigate to PRTPStaticDefault.html
. (If you’re using the demo, the URL is http://<cloud9host>:8080/web/PRTPStaticDefault.html
, where <cloud9host> is the public IP address that you discovered in setting up the IDE.) Choose Play on the audio control at the top. Listen to the audio and watch the highlights. Explore the control by changing speeds, changing voices, pausing, fast-forwarding, and rewinding. The following screenshot shows the page; the text “Skips hidden paragraph” is highlighted because it is currently being read.
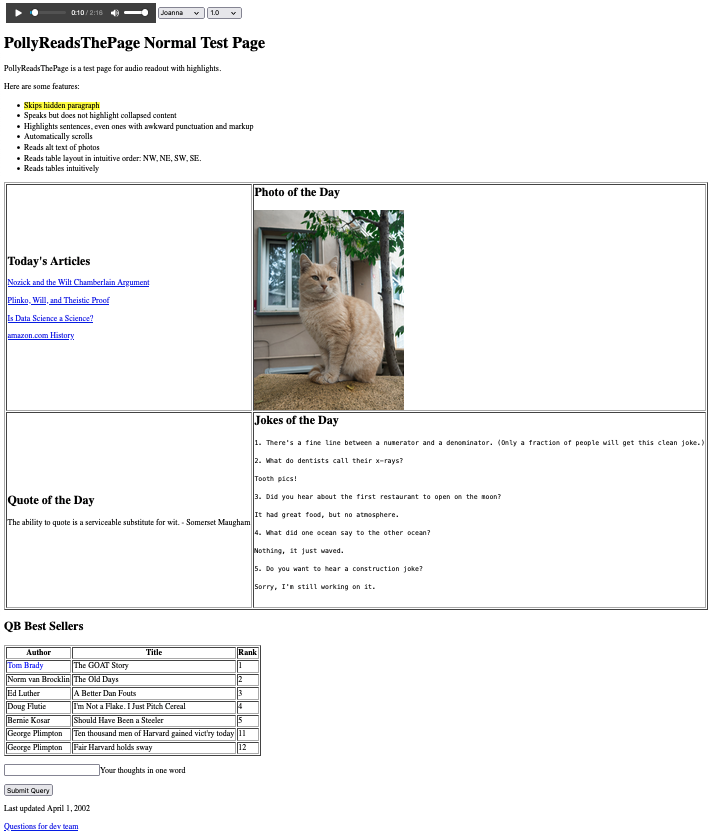
Try the same for PRTPStaticConfig.html
and PRTPStaticCustom.html
. The results are similar. For example, all three read the alt text for the photo of the cat (“Random picture of a cat”). All three read NE, NW, SE, and SW as full words (“northeast,” “northwest,” “southeast,” “southwest”), taking advantage of Amazon Polly lexicons.
Notice the main differences in audio:
-
PRTPStaticDefault.html
reads all the text in the body of the page, including the wrapup portion at the bottom with “Your thoughts in one word,” “Submit Query,” “Last updated April 1, 2020,” and “Questions for the dev team.” PRTPStaticConfig.html
and PRTPStaticCustom.html
don’t read these because they explicitly exclude the wrapup from speech synthesis.
-
PRTPStaticCustom.html
reads the QB Best Sellers table differently from the others. It reads the first three rows only, and reads the row number for each row. It repeats the columns for each row. PRTPStaticCustom.html
uses a custom transformation to tailor the readout of the table. The other pages use default table rendering.
-
PRTPStaticCustom.html
reads “Tom Brady” at a louder volume than the rest of the text. It uses the speech synthesis markup language (SSML) prosody
tag to tailor the reading of Tom Brady. The other pages don’t tailor in this way.
-
PRTPStaticCustom.html
, thanks to a custom transformation, reads the main tiles in NW, SW, NE, SE order; that is, it reads “Today’s Articles,” “Quote of the Day,” “Photo of the Day,” “Jokes of the Day.” The other pages read in the order the tiles appear in the natural NW, NE, SW, SE order they appear in the HTML: “Today’s Articles,” “Photo of the Day,” “Quote of the Day,” “Jokes of the Day.”
Let’s dig deeper into how the audio is generated, and how the page highlights the text.
Static pre-generator
Our GitHub repo includes pre-generated audio files for the PRPTStatic
pages, but if you want to generate them yourself, from the bash shell in the AWS Cloud9 IDE, run the following commands:
# navigate to examples
cd /home/ec2-user/environment/amazon-polly-reads-the-page-blog/pregen/examples
# Set env var for my S3 bucket. Example, I called mine prtp-output
S3_BUCKET=prtp-output # Use output BucketName from CloudFormation
#Add lexicon for pronuniciation of NE NW SE NW
#Script invokes aws polly put-lexicon
./addlexicon.sh.
#Gen each variant
./gen_default.sh
./gen_config.sh
./gen_custom.sh
Now let’s look at how those scripts work.
Default case
We begin with gen_default.sh
:
cd ..
python FixHTML.py ../web/PRTPStaticDefault.html
example/tmp_wff.html
./gen_ssml.sh example/tmp_wff.html generic.xslt example/tmp.ssml
./run_polly.sh example/tmp.ssml en-US Joanna
../web/polly/PRTPStaticDefault compass
./run_polly.sh example/tmp.ssml en-US Matthew
../web/polly/PRTPStaticDefault compass
The script begins by running the Python program FixHTML.py
to make the source HTML file PRTPStaticDefault.html
well-formed. It writes the well-formed version of the file to example/tmp_wff.html
. This step is crucial for two reasons:
- Most source HTML is not well formed. This step repairs the source HTML to be well formed. For example, many HTML pages don’t close
P
elements. This step closes them.
- We keep track of where in the HTML page we find text. We need to track locations using the same document object model (DOM) structure that the browser uses. For example, the browser automatically adds a
TBODY
to a TABLE
. The Python program follows the same well-formed repairs as the browser.
gen_ssml.sh
takes the well-formed HTML as input, applies an XML stylesheet transformation (XSLT) transformation to it, and outputs an SSML file. (SSML is the language in Amazon Polly to control how audio is rendered from text.) In the current example, the input is example/tmp_wff.html
. The output is example/tmp.ssml
. The transform’s job is to decide what text to extract from the HTML and feed to Amazon Polly. generic.xslt
is a sensible default XSLT transform for most webpages. In the following example code snippet, it excludes the audio control, the HTML header, as well as HTML elements like script
and form
. It also excludes elements with the hidden attribute. It includes elements that typically contain text, such as P
, H1
, and SPAN
. For these, it renders both a mark, including the full XPath expression of the element, and the value of the element.
<!-- skip the header -->
<xsl:template match="html/head">
</xsl:template>
<!-- skip the audio itself -->
<xsl:template match="html/body/table[@id='prtp-audio']">
</xsl:template>
<!-- For the body, work through it by applying its templates. This is the default. -->
<xsl:template match="html/body">
<speak>
<xsl:apply-templates />
</speak>
</xsl:template>
<!-- skip these -->
<xsl:template match="audio|option|script|form|input|*[@hidden='']">
</xsl:template>
<!-- include these -->
<xsl:template match="p|h1|h2|h3|h4|li|pre|span|a|th/text()|td/text()">
<xsl:for-each select=".">
<p>
<mark>
<xsl:attribute name="name">
<xsl:value-of select="prtp:getMark(.)"/>
</xsl:attribute>
</mark>
<xsl:value-of select="normalize-space(.)"/>
</p>
</xsl:for-each>
</xsl:template>
The following is a snippet of the SSML that is rendered. This is fed as input to Amazon Polly. Notice, for example, that the text “Skips hidden paragraph” is to be read in the audio, and we associate it with a mark, which tells us that this text occurs in the location on the page given by the XPath expression /html/body[1]/div[2]/ul[1]/li[1]
.
<speak>
<p><mark name="/html/body[1]/div[1]/h1[1]"/>PollyReadsThePage Normal Test Page</p>
<p><mark name="/html/body[1]/div[2]/p[1]"/>PollyReadsThePage is a test page for audio readout with highlights.</p>
<p><mark name="/html/body[1]/div[2]/p[2]"/>Here are some features:</p>
<p><mark name="/html/body[1]/div[2]/ul[1]/li[1]"/>Skips hidden paragraph</p>
<p><mark name="/html/body[1]/div[2]/ul[1]/li[2]"/>Speaks but does not highlight collapsed content</p>
…
</speak>
To generate audio in Amazon Polly, we call the script run_polly.sh
. It runs the AWS Command Line Interface (AWS CLI) command aws polly start-speech-synthesis-task
twice: once to generate MP3 audio, and again to generate the marks file. Because the generation is asynchronous, the script polls until it finds the output in the specified S3 bucket. When it finds the output, it downloads to the build server and copies the files to the web/polly
folder. The following is a listing of the web folders:
- PRTPStaticDefault.html
- PRTPStaticConfig.html
- PRTPStaticCustom.html
- PRTP.js
- polly/PRTPStaticDefault/Joanna.mp3, Joanna.marks, Matthew.mp3, Matthew.marks
- polly/PRTPStaticConfig/Joanna.mp3, Joanna.marks, Matthew.mp3, Matthew.marks
- polly/PRTPStaticCustom/Joanna.mp3, Joanna.marks, Matthew.mp3, Matthew.marks
Each page has its own set of voice-specific MP3 and marks files. These files are the pre-generated files. The page doesn’t need to invoke Amazon Polly at runtime; the files are part of the web build.
Config-driven case
Next, consider gen_config.sh
:
cd ..
python FixHTML.py ../web/PRTPStaticConfig.html
example/tmp_wff.html
python ModGenericXSLT.py example/transform_config.json
example/tmp.xslt
./gen_ssml.sh example/tmp_wff.html example/tmp.xslt
example/tmp.ssml
./run_polly.sh example/tmp.ssml en-US Joanna
../web/polly/PRTPStaticConfig compass
./run_polly.sh example/tmp.ssml en-US Matthew
../web/polly/PRTPStaticConfig compass
The script is similar to the script in the default case, but the bolded lines indicate the main difference. Our approach is config-driven. We tailor the content to be extracted from the page by specifying what to extract through configuration, not code. In particular, we use the JSON file transform_config.json
, which specifies that the content to be included are the elements with IDs title
, main
, maintable
, and qbtable
. The element with ID wrapup
should be excluded. See the following code:
{
"inclusions": [
{"id" : "title"} ,
{"id": "main"},
{"id": "maintable"},
{"id": "qbtable" }
],
"exclusions": [
{"id": "wrapup"}
]
}
We run the Python program ModGenericXSLT.py
to modify generic.xslt
, used in the default case, to use the inclusions and exclusions that we specify in transform_config.json
. The program writes the results to a temp file (example/tmp.xslt
), which it passes to gen_ssml.sh
as its XSLT transform.
This is a low-code option. The web publisher doesn’t need to know how to write XSLT. But they do need to understand the structure of the HTML page and the IDs used in its main organizing elements.
Customization case
Finally, consider gen_custom.sh
:
cd ..
python FixHTML.py ../web/PRTPStaticCustom.html
example/tmp_wff.html
./gen_ssml.sh example/tmp_wff.html example/custom.xslt
example/tmp.ssml
./run_polly.sh example/tmp.ssml en-US Joanna
../web/polly/PRTPStaticCustom compass
./run_polly.sh example/tmp.ssml en-US Matthew
../web/polly/PRTPStaticCustom compass
This script is nearly identical to the default script, except it uses its own XSLT—example/custom.xslt
—rather than the generic XSLT. The following is a snippet of the XSLT:
<!-- Use NW, SW, NE, SE order for main tiles! -->
<xsl:template match="*[@id='maintable']">
<mark>
<xsl:attribute name="name">
<xsl:value-of select="stats:getMark(.)"/>
</xsl:attribute>
</mark>
<xsl:variable name="tiles" select="./tbody"/>
<xsl:variable name="tiles-nw" select="$tiles/tr[1]/td[1]"/>
<xsl:variable name="tiles-ne" select="$tiles/tr[1]/td[2]"/>
<xsl:variable name="tiles-sw" select="$tiles/tr[2]/td[1]"/>
<xsl:variable name="tiles-se" select="$tiles/tr[2]/td[2]"/>
<xsl:variable name="tiles-seq" select="($tiles-nw, $tiles-sw, $tiles-ne, $tiles-se)"/>
<xsl:for-each select="$tiles-seq">
<xsl:apply-templates />
</xsl:for-each>
</xsl:template>
<!-- Say Tom Brady load! -->
<xsl:template match="span[@style = 'color:blue']" >
<p>
<mark>
<xsl:attribute name="name">
<xsl:value-of select="prtp:getMark(.)"/>
</xsl:attribute>
</mark>
<prosody volume="x-loud">Tom Brady</prosody>
</p>
</xsl:template>
If you want to study the code in detail, refer to the scripts and programs in the GitHub repo.
Browser setup and highlights
The static pages include an HTML5 audio control, which takes as its audio source the MP3 file generated by Amazon Polly and residing on the web server:
<audio id="audio" controls>
<source src="polly/PRTPStaticDefault/en/Joanna.mp3" type="audio/mpeg">
</audio>
At load time, the page also loads the Amazon Polly-generated marks file. This occurs in the PRTP.js
file, which the HTML page includes. The following is a snippet of the marks file for PRTPStaticDefault
:
{“time”:11747,“type”:“sentence”,“start”:289,“end”:356,“value”:“PollyReadsThePage is a test page for audio readout with highlights.“}
{“time”:15784,“type”:“ssml”,“start”:363,“end”:403,“value”:“/html/body[1]/div[2]/p[2]“}
{“time”:16427,“type”:“sentence”,“start”:403,“end”:426,“value”:“Here are some features:“}
{“time”:17677,“type”:“ssml”,“start”:433,“end”:480,“value”:“/html/body[1]/div[2]/ul[1]/li[1]“}
{“time”:18344,“type”:“sentence”,“start”:480,“end”:502,“value”:“Skips hidden paragraph”}
{“time”:19894,“type”:“ssml”,“start”:509,“end”:556,“value”:“/html/body[1]/div[2]/ul[1]/li[2]“}
{“time”:20537,“type”:“sentence”,“start”:556,“end”:603,“value”:“Speaks but does not highlight collapsed content”}
During audio playback, there is an audio timer event handler in PRTP.js
that checks the audio’s current time, finds the text to highlight, finds its location on the page, and highlights it. The text to be highlighted is an entry of type sentence
in the marks file. The location is the XPath expression in the name attribute of the entry of type SSML that precedes the sentence. For example, if the time is 18400, according to the marks file, the sentence to be highlighted is “Skips hidden paragraph,” which starts at 18334. The location is the SSML entry at time 17667: /html/body[1]/div[2]/ul[1]/li[1]
.
Test dynamic pages
The page PRTPDynamic.html
demonstrates dynamic audio readback using default, configuration-driven, and custom audio extraction approaches.
Default case
In your browser, navigate to PRTPDynamic.html
. The page has one query parameter, dynOption
, which accepts values default
, config
, and custom
. It defaults to default
, so you may omit it in this case. The page has two sections with dynamic content:
-
Latest Articles – Changes frequently throughout the day
-
Greek Philosophers Search By Date – Allows the visitor to search for Greek philosophers by date and shows the results in a table
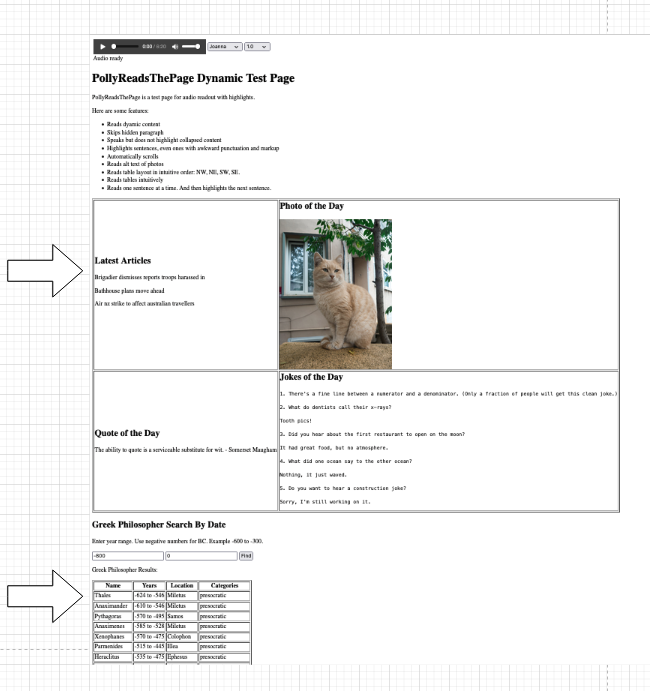
Create some content in the Greek Philosopher section by entering a date range of -800 to 0, as shown in the example. Then choose Find.
Now play the audio by choosing Play in the audio control.
Behind the scenes, the page runs the following code to render and play the audio:
buildSSMLFromDefault();
chooseRenderAudio();
setVoice();
First it calls the function buildSSMLFromDefault
in PRTP.js
to extract most of the text from the HTML page body. That function walks the DOM tree, looking for text in common elements such as p
, h1
, pre
, span
, and td
. It ignores text in elements that usually don’t contain text to be read aloud, such as audio
, option
, and script
. It builds SSML markup to be input to Amazon Polly. The following is a snippet showing extraction of the first row from the philosopher
table:
<speak>
...
<p><mark name="/HTML[1]/BODY[1]/DIV[3]/DIV[1]/DIV[1]/TABLE[1]/TBODY[1]/TR[2]/TD[1]"/>Thales</p>
<p><mark name="/HTML[1]/BODY[1]/DIV[3]/DIV[1]/DIV[1]/TABLE[1]/TBODY[1]/TR[2]/TD[2]"/>-624 to -546</p>
<p><mark name="/HTML[1]/BODY[1]/DIV[3]/DIV[1]/DIV[1]/TABLE[1]/TBODY[1]/TR[2]/TD[3]"/>Miletus</p>
<p><mark name="/HTML[1]/BODY[1]/DIV[3]/DIV[1]/DIV[1]/TABLE[1]/TBODY[1]/TR[2]/TD[4]"/>presocratic</p>
...
</speak>
The chooseRenderAudio
function in PRTP.js
begins by initializing the AWS SDK for Amazon Cognito, Amazon S3, and Amazon Polly. This initialization occurs only once. If chooseRenderAudio
is invoked again because the content of the page has changed, the initialization is skipped. See the following code:
AWS.config.region = env.REGION
AWS.config.credentials = new AWS.CognitoIdentityCredentials({
IdentityPoolId: env.IDP});
audioTracker.sdk.connection = {
polly: new AWS.Polly({apiVersion: '2016-06-10'}),
s3: new AWS.S3()
};
It generates MP3 audio from Amazon Polly. The generation is synchronous for small SSML inputs and asynchronous (with output sent to the S3 bucket) for large SSML inputs (greater than 6,000 characters). In the synchronous case, we ask Amazon Polly to provide the MP3 file using a presigned URL. When the synthesized output is ready, we set the src
attribute of the audio control to that URL and load the control. We then request the marks file and load it the same way as in the static case. See the following code:
// create signed URL
const signer = new AWS.Polly.Presigner(pollyAudioInput, audioTracker.sdk.connection.polly);
// call Polly to get MP3 into signed URL
signer.getSynthesizeSpeechUrl(pollyAudioInput, function(error, url) {
// Audio control uses signed URL
audioTracker.audioControl.src =
audioTracker.sdk.audio[audioTracker.voice];
audioTracker.audioControl.load();
// call Polly to get marks
audioTracker.sdk.connection.polly.synthesizeSpeech(
pollyMarksInput, function(markError, markData) {
const marksStr = new
TextDecoder().decode(markData.AudioStream);
// load marks into page the same as with static
doLoadMarks(marksStr);
});
});
Config-driven case
In your browser, navigate to PRTPDynamic.html?dynOption=config
. Play the audio. The audio playback is similar to the default case, but there are minor differences. In particular, some content is skipped.
Behind the scenes, when using the config
option, the page extracts content differently than in the default case. In the default case, the page uses buildSSMLFromDefault
. In the config-driven case, the page specifies the sections it wants to include and exclude:
const ssml = buildSSMLFromConfig({
"inclusions": [
{"id": "title"},
{"id": "main"},
{"id": "maintable"},
{"id": "phil-result"},
{"id": "qbtable"},
],
"exclusions": [
{"id": "wrapup"}
]
});
The buildSSMLFromConfig
function, defined in PRTP.js
, walks the DOM tree in each of the sections whose ID is provided under inclusions
. It extracts content from each and combines them together, in the order specified, to form an SSML document. It excludes the sections specified under exclusions
. It extracts content from each section in the same way buildSSMLFromDefault
extracts content from the page body.
Customization case
In your browser, navigate to PRTPDynamic.html?dynOption=custom
. Play the audio. There are three noticeable differences. Let’s note these and consider the custom code that runs behind the scenes:
- It reads the main tiles in NW, SW, NE, SE order. The custom code gets each of these cell blocks from
maintable
and adds them to the SSML in NW, SW, NE, SE order:
const nw = getElementByXpath("//*[@id='maintable']//tr[1]/td[1]");
const sw = getElementByXpath("//*[@id='maintable']//tr[2]/td[1]");
const ne = getElementByXpath("//*[@id='maintable']//tr[1]/td[2]");
const se = getElementByXpath("//*[@id='maintable']//tr[2]/td[2]");
[nw, sw, ne, se].forEach(dir => buildSSMLSection(dir, []));
- “Tom Brady” is spoken loudly. The custom code puts “Tom Brady” text inside an SSML
prosody
tag:
if (cellText == "Tom Brady") {
addSSMLMark(getXpathOfNode( node.childNodes[tdi]));
startSSMLParagraph();
startSSMLTag("prosody", {"volume": "x-loud"});
addSSMLText(cellText);
endSSMLTag();
endSSMLParagraph();
}
- It reads only the first three rows of the quarterback table. It reads the column headers for each row. Check the code in the GitHub repo to discover how this is implemented.
Clean up
To avoid incurring future charges, delete the CloudFormation stack.
Conclusion
In this post, we demonstrated a technical solution to a high-value business problem: how to use Amazon Polly to read the content of a webpage and highlight the content as it’s being read. We showed this using both static and dynamic pages. To extract content from the page, we used DOM traversal and XSLT. To facilitate highlighting, we used the speech marks capability in Amazon Polly.
Learn more about Amazon Polly by visiting its service page.
Feel free to ask questions in the comments.
About the authors
Mike Havey is a Solutions Architect for AWS with over 25 years of experience building enterprise applications. Mike is the author of two books and numerous articles. Visit his Amazon author page to read more.
Vineet Kachhawaha is a Solutions Architect at AWS with expertise in machine learning. He is responsible for helping customers architect scalable, secure, and cost-effective workloads on AWS.
Read More