Our Operating Principles have come to define both our commitment to prioritising widespread benefit, as well as the areas of research and applications we refuse to pursue. These principles have been at the heart of our decision making since DeepMind was founded, and continue to be refined as the AI landscape changes and grows. They are designed for our role as a research-driven science company and consistent with Google’s AI principles.Read More
How our principles helped define AlphaFold’s release
Our Operating Principles have come to define both our commitment to prioritising widespread benefit, as well as the areas of research and applications we refuse to pursue. These principles have been at the heart of our decision making since DeepMind was founded, and continue to be refined as the AI landscape changes and grows. They are designed for our role as a research-driven science company and consistent with Google’s AI principles.Read More
LOLNeRF: Learn from One Look
An important aspect of human vision is our ability to comprehend 3D shape from the 2D images we observe. Achieving this kind of understanding with computer vision systems has been a fundamental challenge in the field. Many successful approaches rely on multi-view data, where two or more images of the same scene are available from different perspectives, which makes it much easier to infer the 3D shape of objects in the images.
There are, however, many situations where it would be useful to know 3D structure from a single image, but this problem is generally difficult or impossible to solve. For example, it isn’t necessarily possible to tell the difference between an image of an actual beach and an image of a flat poster of the same beach. However it is possible to estimate 3D structure based on what kind of 3D objects occur commonly and what similar structures look like from different perspectives.
In “LOLNeRF: Learn from One Look”, presented at CVPR 2022, we propose a framework that learns to model 3D structure and appearance from collections of single-view images. LOLNeRF learns the typical 3D structure of a class of objects, such as cars, human faces or cats, but only from single views of any one object, never the same object twice. We build our approach by combining Generative Latent Optimization (GLO) and neural radiance fields (NeRF) to achieve state-of-the-art results for novel view synthesis and competitive results for depth estimation.
Combining GLO and NeRF
GLO is a general method that learns to reconstruct a dataset (such as a set of 2D images) by co-learning a neural network (decoder) and table of codes (latents) that is also an input to the decoder. Each of these latent codes re-creates a single element (such as an image) from the dataset. Because the latent codes have fewer dimensions than the data elements themselves, the network is forced to generalize, learning common structure in the data (such as the general shape of dog snouts).
NeRF is a technique that is very good at reconstructing a static 3D object from 2D images. It represents an object with a neural network that outputs color and density for each point in 3D space. Color and density values are accumulated along rays, one ray for each pixel in a 2D image. These are then combined using standard computer graphics volume rendering to compute a final pixel color. Importantly, all these operations are differentiable, allowing for end-to-end supervision. By enforcing that each rendered pixel (of the 3D representation) matches the color of ground truth (2D) pixels, the neural network creates a 3D representation that can be rendered from any viewpoint.
We combine NeRF with GLO by assigning each object a latent code and concatenating it with standard NeRF inputs, giving it the ability to reconstruct multiple objects. Following GLO, we co-optimize these latent codes along with network weights during training to reconstruct the input images. Unlike standard NeRF, which requires multiple views of the same object, we supervise our method with only single views of any one object (but multiple examples of that type of object). Because NeRF is inherently 3D, we can then render the object from arbitrary viewpoints. Combining NeRF with GLO gives it the ability to learn common 3D structure across instances from only single views while still retaining the ability to recreate specific instances of the dataset.
Camera Estimation
In order for NeRF to work, it needs to know the exact camera location, relative to the object, for each image. Unless this was measured when the image was taken, it is generally unknown. Instead, we use the MediaPipe Face Mesh to extract five landmark locations from the images. Each of these 2D predictions correspond to a semantically consistent point on the object (e.g., the tip of the nose or corners of the eyes). We can then derive a set of canonical 3D locations for the semantic points, along with estimates of the camera poses for each image, such that the projection of the canonical points into the images is as consistent as possible with the 2D landmarks.
![]() |
Example MediaPipe landmarks and segmentation masks (images from CelebA). |
Hard Surface and Mask Losses
Standard NeRF is effective for accurately reproducing the images, but in our single-view case, it tends to produce images that look blurry when viewed off-axis. To address this, we introduce a novel hard surface loss, which encourages the density to adopt sharp transitions from exterior to interior regions, reducing blurring. This essentially tells the network to create “solid” surfaces, and not semi-transparent ones like clouds.
We also obtained better results by splitting the network into separate foreground and background networks. We supervised this separation with a mask from the MediaPipe Selfie Segmenter and a loss to encourage network specialization. This allows the foreground network to specialize only on the object of interest, and not get “distracted” by the background, increasing its quality.
Results
We surprisingly found that fitting only five key points gave accurate enough camera estimates to train a model for cats, dogs, or human faces. This means that given only a single view of your beloved cats Schnitzel, Widget and friends, you can create a new image from any other angle.
![]() |
Top: example cat images from AFHQ. Bottom: A synthesis of novel 3D views created by LOLNeRF. |
Conclusion
We’ve developed a technique that is effective at discovering 3D structure from single 2D images. We see great potential in LOLNeRF for a variety of applications and are currently investigating potential use-cases.
![]() |
Interpolation of feline identities from linear interpolation of learned latent codes for different examples in AFHQ. |
Code Release
We acknowledge the potential for misuse and importance of acting responsibly. To that end, we will only release the code for reproducibility purposes, but will not release any trained generative models.
Acknowledgements
We would like to thank Andrea Tagliasacchi, Kwang Moo Yi, Viral Carpenter, David Fleet, Danica Matthews, Florian Schroff, Hartwig Adam and Dmitry Lagun for continuous help in building this technology.
Get up to Speed: Five Reasons Not to Miss NVIDIA CEO Jensen Huang’s GTC Keynote Sept. 20
Think fast. Enterprise AI, new gaming technology, the metaverse and the 3D internet, and advanced AI technologies tailored to just about every industry are all coming your way.
NVIDIA founder and CEO Jensen Huang’s keynote at NVIDIA GTC on Tuesday, Sept. 20, is the best way to get ahead of all these trends.
NVIDIA’s virtual technology conference, which takes place Sept. 19-22, sits at the intersections of business and technology, science and the arts in a way no other event can.
This GTC will focus on neural graphics — which bring together AI and visual computing to create stunning new possibilities — the metaverse, an update on large language models, and the changes coming to every industry with the latest generation of recommender systems.
The free online gathering features speakers from every corner of industry, academia and research.
Speakers include Johnson & Johnson CTO Rowena Yao; Boeing Vice President Linda Hapgood; Polestar COO Dennis Nobelius; Deutsche Bank CTO Bernd Leukert; UN Assistant Secretary-General Ahunna Eziakonwa; UC San Diego distinguished professor Henrik Christensen, and hundreds more.
For those who want to get hands on, GTC features developer sessions for newbies and veteran developers.
Two-hour training labs are included for those who sign up for a free conference pass. Those who want to dig deeper can sign up for one of 21 full-day virtual hands-on workshops at a special price of $149, and for group purchases of more than five seats, we are offering a special of $99 per seat.
Finally, GTC offers networking opportunities that bring together people working on the most challenging problems of our time from all over the planet.
Register free and start loading up your calendar with content today.
The post Get up to Speed: Five Reasons Not to Miss NVIDIA CEO Jensen Huang’s GTC Keynote Sept. 20 appeared first on NVIDIA Blog.
Get better insight from reviews using Amazon Comprehend
“85% of buyers trust online reviews as much as a personal recommendation” – Gartner
Consumers are increasingly engaging with businesses through digital surfaces and multiple touchpoints. Statistics show that the majority of shoppers use reviews to determine what products to buy and which services to use. As per Spiegel Research Centre, the purchase likelihood for a product with five reviews is 270% greater than the purchase likelihood of a product with no reviews. Reviews have the power to influence consumer decisions and strengthen brand value.
In this post, we use Amazon Comprehend to extract meaningful information from product reviews, analyze it to understand how users of different demographics are reacting to products, and discover aggregated information on user affinity towards a product. Amazon Comprehend is a fully managed and continuously trained natural language processing (NLP) service that can extract insight about content of a document or text.
Solution overview
Today, reviews can be provided by customers in various ways, such as star ratings, free text or natural language, or social media shares. Free text or natural language reviews help build trust, as it’s an independent opinion from consumers. It’s often used by product teams to interact with customers through review channels. It’s a proven fact that when customers feel heard, their feeling about the brand improves. Whereas it’s comparatively easier to analyze star ratings or social media shares, natural language or free text reviews pose multiple challenges, like identifying keywords or phrases, topics or concepts, and sentiment or entity-level sentiments. The challenge is mainly due to the variability of length in written text and plausible presence of both signals and noise. Furthermore, the information can either be very clear and explicit (for example, with keywords and key phrases) or unclear and implicit (abstract topics and concepts). Even more challenging is understanding different types of sentiments and relating them to appropriate products and services. Nevertheless, it’s highly critical to understand this information and textual signals in order to provide a frictionless customer experience.
In this post, we use a publicly available NLP – fast.ai dataset to analyze the product reviews provided by customers. We start by using an unsupervised machine learning (ML) technique known as topic modeling. This a popular unsupervised technique that discovers abstract topics that can occur in a text review collection. Topic modeling is a clustering problem that is unsupervised, meaning that the models have no knowledge on possible target variables (such as topics in a review). The topics are represented as clusters. Often, the number of clusters in a corpus of documents is decided with the help of domain experts or by using some standard statistical analysis. The model outputs generally have three components: numbered clusters (topic 0, topic 1, and so on), keywords associated to each cluster, and representative clusters for each document (or review in our case). By its inherent nature, topic models don’t generate human-readable labels for the clusters or topics, which is a common misconception. Something to note about topic modeling in general is that it’s a mixed membership model— every document in the model may have a resemblance to every topic. The topic model learns in an iterative Bayesian process to determine the probability that each document is associated with a given theme or topic. The model output depends on selecting the number of topics optimally. A small number of topics can result in the topics being too broad, and a larger number of topics may result in redundant topics or topics with similarity. There are a number of ways to evaluate topic models:
- Human judgment – Observation-based, interpretation-based
- Quantitative metrics – Perplexity, coherence calculations
- Mixed approach – A combination of judgment-based and quantitative approaches
Perplexity is calculated by splitting a dataset into two parts—a training set and a test set. Likelihood is usually calculated as a logarithm, so this metric is sometimes referred to as the held-out log-likelihood. Perplexity is a predictive metric. It assesses a topic model’s ability to predict a test set after having been trained on a training set. One of the shortcomings of perplexity is that it doesn’t capture context, meaning that it doesn’t capture the relationship between words in a topic or topics in a document. However, the idea of semantic context is important for human understanding. Measures such as the conditional likelihood of the co- occurrence of words in a topic can be helpful. These approaches are collectively referred to as coherence. For this post, we focus on the human judgment (observation-based) approach, namely observing the top n words in a topic.
The solution consists of the following high-level steps:
- Set up an Amazon SageMaker notebook instance.
- Create a notebook.
- Perform exploratory data analysis.
- Run your Amazon Comprehend topic modeling job.
- Generate topics and understand sentiment.
- Use Amazon QuickSight to visualize data and generate reports.
You can use this solution in any AWS Region, but you need to make sure that the Amazon Comprehend APIs and SageMaker are in the same Region. For this post, we use the Region US East (N. Virginia).
Set up your SageMaker notebook instance
You can interact with Amazon Comprehend via the AWS Management Console, AWS Command Line Interface (AWS CLI), or Amazon Comprehend API. For more information, refer to Getting started with Amazon Comprehend. We use a SageMaker notebook and Python (Boto3) code throughout this post to interact with the Amazon Comprehend APIs.
- On the Amazon SageMaker console, under Notebook in the navigation pane, choose
Notebook instances. - Choose Create notebook instance.
- Specify a notebook instance name and set the instance type as ml.r5.2xlarge.
- Leave the rest of the default settings.
- Create an AWS Identity and Access Management (IAM) role with
AmazonSageMakerFullAccess
and access to any necessary Amazon Simple Storage Service (Amazon S3) buckets and Amazon Comprehend APIs. - Choose Create notebook instance.
After a few minutes, your notebook instance is ready. - To access Amazon Comprehend from the notebook instance, you need to attach the
ComprehendFullAccess
policy to your IAM role.
For a security overview of Amazon Comprehend, refer to Security in Amazon Comprehend.
Create a notebook
After you open the notebook instance that you provisioned, on the Jupyter console, choose New and then Python 3 (Data Science). Alternatively, you can access the sample code file in the GitHub repo. You can upload the file to the notebook instance to run it directly or clone it.
The GitHub repo contains three notebooks:
data_processing.ipynb
model_training.ipynb
topic_mapping_sentiment_generation.ipynb
Perform exploratory data analysis
We use the first notebook (data_processing.ipynb
) to explore and process the data. We start by simply loading the data from an S3 bucket into a DataFrame.
# Bucket containing the data
BUCKET = 'clothing-shoe-jewel-tm-blog'
# Item ratings and metadata
S3_DATA_FILE = 'Clothing_Shoes_and_Jewelry.json.gz' # Zip
S3_META_FILE = 'meta_Clothing_Shoes_and_Jewelry.json.gz' # Zip
S3_DATA = 's3://' + BUCKET + '/' + S3_DATA_FILE
S3_META = 's3://' + BUCKET + '/' + S3_META_FILE
# Transformed review, input for Comprehend
LOCAL_TRANSFORMED_REVIEW = os.path.join('data', 'TransformedReviews.txt')
S3_OUT = 's3://' + BUCKET + '/out/' + 'TransformedReviews.txt'
# Final dataframe where topics and sentiments are going to be joined
S3_FEEDBACK_TOPICS = 's3://' + BUCKET + '/out/' + 'FinalDataframe.csv'
def convert_json_to_df(path):
"""Reads a subset of a json file in a given path in chunks, combines, and returns
"""
# Creating chunks from 500k data points each of chunk size 10k
chunks = pd.read_json(path, orient='records',
lines=True,
nrows=500000,
chunksize=10000,
compression='gzip')
# Creating a single dataframe from all the chunks
load_df = pd.DataFrame()
for chunk in chunks:
load_df = pd.concat([load_df, chunk], axis=0)
return load_df
# Review data
original_df = convert_json_to_df(S3_DATA)
# Metadata
original_meta = convert_json_to_df(S3_META)
In the following section, we perform exploratory data analysis (EDA) to understand the data. We start by exploring the shape of the data and metadata. For authenticity, we use verified reviews only.
# Shape of reviews and metadata
print('Shape of review data: ', original_df.shape)
print('Shape of metadata: ', original_meta.shape)
# We are interested in verified reviews only
# Also checking the amount of missing values in the review data
print('Frequency of verified/non verified review data: ', original_df['verified'].value_counts())
print('Frequency of missing values in review data: ', original_df.isna().sum())
We further explore the count of each category, and see if any duplicate data is present.
# Count of each categories for EDA.
print('Frequncy of different item categories in metadata: ', original_meta['category'].value_counts())
# Checking null values for metadata
print('Frequency of missing values in metadata: ', original_meta.isna().sum())
# Checking if there are duplicated data. There are indeed duplicated data in the dataframe.
print('Duplicate items in metadata: ', original_meta[original_meta['asin'].duplicated()])
When we’re satisfied with the results, we move to the next step of preprocessing the data. Amazon Comprehend recommends providing at least 1,000 documents in each topic modeling job, with each document at least three sentences long. Documents must be in UTF-8 formatted text files. In the following step, we make sure that data is in the recommended UTF-8 format and each input is no more than 5,000 bytes in size.
def clean_text(df):
"""Preprocessing review text.
The text becomes Comprehend compatible as a result.
This is the most important preprocessing step.
"""
# Encode and decode reviews
df['reviewText'] = df['reviewText'].str.encode("utf-8", "ignore")
df['reviewText'] = df['reviewText'].str.decode('ascii')
# Replacing characters with whitespace
df['reviewText'] = df['reviewText'].replace(r'r+|n+|t+|u2028',' ', regex=True)
# Replacing punctuations
df['reviewText'] = df['reviewText'].str.replace('[^ws]','', regex=True)
# Lowercasing reviews
df['reviewText'] = df['reviewText'].str.lower()
return df
def prepare_input_data(df):
"""Encoding and getting reviews in byte size.
Review gets encoded to utf-8 format and getting the size of the reviews in bytes.
Comprehend requires each review input to be no more than 5000 Bytes
"""
df['review_size'] = df['reviewText'].apply(lambda x:len(x.encode('utf-8')))
df = df[(df['review_size'] > 0) & (df['review_size'] < 5000)]
df = df.drop(columns=['review_size'])
return df
# Only data points with a verified review will be selected and the review must not be missing
filter = (original_df['verified'] == True) & (~original_df['reviewText'].isna())
filtered_df = original_df[filter]
# Only a subset of fields are selected in this experiment.
filtered_df = filtered_df[['asin', 'reviewText', 'summary', 'unixReviewTime', 'overall', 'reviewerID']]
# Just in case, once again, dropping data points with missing review text
filtered_df = filtered_df.dropna(subset=['reviewText'])
print('Shape of review data: ', filtered_df.shape)
# Dropping duplicate items from metadata
original_meta = original_meta.drop_duplicates(subset=['asin'])
# Only a subset of fields are selected in this experiment.
original_meta = original_meta[['asin', 'category', 'title', 'description', 'brand', 'main_cat']]
# Clean reviews using text cleaning pipeline
df = clean_text(filtered_df)
# Dataframe where Comprehend outputs (topics and sentiments) will be added
df = prepare_input_data(df)
We then save the data to Amazon S3 and also keep a local copy in the notebook instance.
# Saving dataframe on S3 df.to_csv(S3_FEEDBACK_TOPICS, index=False)
# Reviews are transformed per Comprehend guideline- one review per line
# The txt file will be used as input for Comprehend
# We first save the input file locally
with open(LOCAL_TRANSFORMED_REVIEW, "w") as outfile:
outfile.write("n".join(df['reviewText'].tolist()))
# Transferring the transformed review (input to Comprehend) to S3
!aws s3 mv {LOCAL_TRANSFORMED_REVIEW} {S3_OUT}
This completes our data processing phase.
Run an Amazon Comprehend topic modeling job
We then move to the next phase, where we use the preprocessed data to run a topic modeling job using Amazon Comprehend. At this stage, you can either use the second notebook (model_training.ipynb
) or use the Amazon Comprehend console to run the topic modeling job. For instructions on using the console, refer to Running analysis jobs using the console. If you’re using the notebook, you can start by creating an Amazon Comprehend client using Boto3, as shown in the following example.
# Client and session information
session = boto3.Session()
s3 = boto3.resource('s3')
# Account id. Required downstream.
account_id = boto3.client('sts').get_caller_identity().get('Account')
# Initializing Comprehend client
comprehend = boto3.client(service_name='comprehend',
region_name=session.region_name)
You can submit your documents for topic modeling in two ways: one document per file, or one document per line.
We start with 5 topics (k-number), and use one document per line. There is no single best way as a standard practice to select k or the number of topics. You may try out different values of k, and select the one that has the largest likelihood.
# Number of topics set to 5 after having a human-in-the-loop
# This needs to be fully aligned with topicMaps dictionary in the third script
NUMBER_OF_TOPICS = 5
# Input file format of one review per line
input_doc_format = "ONE_DOC_PER_LINE"
# Role arn (Hard coded, masked)
data_access_role_arn = "arn:aws:iam::XXXXXXXXXXXX:role/service-role/AmazonSageMaker-ExecutionRole-XXXXXXXXXXXXXXX"
Our Amazon Comprehend topic modeling job requires you to pass an InputDataConfig
dictionary object with S3, InputFormat
, and DocumentReadAction
as required parameters. Similarly, you need to provide the OutputDataConfig
object with S3 and DataAccessRoleArn
as required parameters. For more information, refer to the Boto3 documentation for start_topics_detection_job
.
# Constants for S3 bucket and input data file
BUCKET = 'clothing-shoe-jewel-tm-blog'
input_s3_url = 's3://' + BUCKET + '/out/' + 'TransformedReviews.txt'
output_s3_url = 's3://' + BUCKET + '/out/' + 'output/'
# Final dataframe where we will join Comprehend outputs later
S3_FEEDBACK_TOPICS = 's3://' + BUCKET + '/out/' + 'FinalDataframe.csv'
# Local copy of Comprehend output
LOCAL_COMPREHEND_OUTPUT_DIR = os.path.join('comprehend_out', '')
LOCAL_COMPREHEND_OUTPUT_FILE = os.path.join(LOCAL_COMPREHEND_OUTPUT_DIR, 'output.tar.gz')
INPUT_CONFIG={
# The S3 URI where Comprehend input is placed.
'S3Uri': input_s3_url,
# Document format
'InputFormat': input_doc_format,
}
OUTPUT_CONFIG={
# The S3 URI where Comprehend output is placed.
'S3Uri': output_s3_url,
}
You can then start an asynchronous topic detection job by passing the number of topics, input configuration object, output configuration object, and an IAM role, as shown in the following example.
# Reading the Comprehend input file just to double check if number of reviews
# and the number of lines in the input file have an exact match.
obj = s3.Object(input_s3_url)
comprehend_input = obj.get()['Body'].read().decode('utf-8')
comprehend_input_lines = len(comprehend_input.split('n'))
# Reviews where Comprehend outputs will be merged
df = pd.read_csv(S3_FEEDBACK_TOPICS)
review_df_length = df.shape[0]
# The two lengths must be equal
assert comprehend_input_lines == review_df_length
# Start Comprehend topic modelling job.
# Specifies the number of topics, input and output config and IAM role ARN
# that grants Amazon Comprehend read access to data.
start_topics_detection_job_result = comprehend.start_topics_detection_job(
NumberOfTopics=NUMBER_OF_TOPICS,
InputDataConfig=INPUT_CONFIG,
OutputDataConfig=OUTPUT_CONFIG,
DataAccessRoleArn=data_access_role_arn)
print('start_topics_detection_job_result: ' + json.dumps(start_topics_detection_job_result))
# Job ID is required downstream for extracting the Comprehend results
job_id = start_topics_detection_job_result["JobId"]
print('job_id: ', job_id)
You can track the current status of the job by calling the DescribeTopicDetectionJob
operation. The status of the job can be one of the following:
- SUBMITTED – The job has been received and is queued for processing
- IN_PROGRESS – Amazon Comprehend is processing the job
- COMPLETED – The job was successfully completed and the output is available
- FAILED – The job didn’t complete
# Topic detection takes a while to complete.
# We can track the current status by calling Use the DescribeTopicDetectionJob operation.
# Keeping track if Comprehend has finished its job
description = comprehend.describe_topics_detection_job(JobId=job_id)
topic_detection_job_status = description['TopicsDetectionJobProperties']["JobStatus"]
print(topic_detection_job_status)
while topic_detection_job_status not in ["COMPLETED", "FAILED"]:
time.sleep(120)
topic_detection_job_status = comprehend.describe_topics_detection_job(JobId=job_id)['TopicsDetectionJobProperties']["JobStatus"]
print(topic_detection_job_status)
topic_detection_job_status = comprehend.describe_topics_detection_job(JobId=job_id)['TopicsDetectionJobProperties']["JobStatus"]
print(topic_detection_job_status)
When the job is successfully complete, it returns a compressed archive containing two files: topic-terms.csv and doc-topics.csv. The first output file, topic-terms.csv
, is a list of topics in the collection. For each topic, the list includes, by default, the top terms by topic according to their weight. The second file, doc-topics.csv
, lists the documents associated with a topic and the proportion of the document that is concerned with the topic. Because we specified ONE_DOC_PER_LINE
earlier in the input_doc_format
variable, the document is identified by the file name and the 0-indexed line number within the file. For more information on topic modeling, refer to Topic modeling.
The outputs of Amazon Comprehend are copied locally for our next steps.
# Bucket prefix where model artifacts are stored
prefix = f'{account_id}-TOPICS-{job_id}'
# Model artifact zipped file
artifact_file = 'output.tar.gz'
# Location on S3 where model artifacts are stored
target = f's3://{BUCKET}/out/output/{prefix}/{artifact_file}'
# Copy Comprehend output from S3 to local notebook instance
! aws s3 cp {target} ./comprehend-out/
# Unzip the Comprehend output file.
# Two files are now saved locally-
# (1) comprehend-out/doc-topics.csv and
# (2) comprehend-out/topic-terms.csv
comprehend_tars = tarfile.open(LOCAL_COMPREHEND_OUTPUT_FILE)
comprehend_tars.extractall(LOCAL_COMPREHEND_OUTPUT_DIR)
comprehend_tars.close()
Because the number of topics is much less than the vocabulary associated with the document collection, the topic space representation can be viewed as a dimensionality reduction process as well. You may use this topic space representation of documents to perform clustering. On the other hand, you can analyze the frequency of words in each cluster to determine topic associated with each cluster. For this post, we don’t perform any other techniques like clustering.
Generate topics and understand sentiment
We use the third notebook (topic_mapping_sentiment_generation.ipynb
) to find how users of different demographics are reacting to products, and also analyze aggregated information on user affinity towards a particular product.
We can combine the outputs from the previous notebook to get topics and associated terms for each topic. However, the topics are numbered and may lack explainability. Therefore, we prefer to use a human-in-the-loop with enough domain knowledge and subject matter expertise to name the topics by looking at their associated terms. This process can be considered as a mapping from topic numbers to topic names. However, it’s noteworthy that the individual list of terms for the topics can be mutually inclusive and therefore may create multiple mappings. The human-in-the-loop should formalize the mappings based on the context of the use case. Otherwise, the downstream performance may be impacted.
We start by declaring the variables. For each review, there can be multiple topics. We count their frequency and select a maximum of three most frequent topics. These topics are reported as the representative topics of a review. First, we define a variable TOP_TOPICS
to hold the maximum number of representative topics. Second, we define and set values to the language_code
variable to support the required language parameter of Amazon Comprehend. Finally, we create topicMaps
, which is a dictionary that maps topic numbers to topic names.
# boto3 session to access service
session = boto3.Session()
comprehend = boto3.client( 'comprehend',
region_name=session.region_name)
# S3 bucket
BUCKET = 'clothing-shoe-jewel-tm-blog'
# Local copy of doc-topic file
DOC_TOPIC_FILE = os.path.join('comprehend-out', 'doc-topics.csv')
# Final dataframe where we will join Comprehend outputs later
S3_FEEDBACK_TOPICS = 's3://' + BUCKET + '/out/' + 'FinalDataframe.csv'
# Final output
S3_FINAL_OUTPUT = 's3://' + BUCKET + '/out/' + 'reviewTopicsSentiments.csv'
# Top 3 topics per product will be aggregated
TOP_TOPICS = 3
# Working on English language only.
language_code = 'en'
# Topic names for 5 topics created by human-in-the-loop or SME feed
topicMaps = {
0: 'Product comfortability',
1: 'Product Quality and Price',
2: 'Product Size',
3: 'Product Color',
4: 'Product Return',
}
Next, we use the topic-terms.csv file generated by Amazon Comprehend to connect the unique terms associated with each topic. Then, by applying the mapping dictionary on this topic-term association, we connect the unique terms to the topic names.
# Loading documents and topics assigned to each of them by Comprehend
docTopics = pd.read_csv(DOC_TOPIC_FILE)
docTopics.head()
# Creating a field with doc number.
# This doc number is the line number of the input file to Comprehend.
docTopics['doc'] = docTopics['docname'].str.split(':').str[1]
docTopics['doc'] = docTopics['doc'].astype(int)
docTopics.head()
# Load topics and associated terms
topicTerms = pd.read_csv(DOC_TOPIC_FILE)
# Consolidate terms for each topic
aggregatedTerms = topicTerms.groupby('topic')['term'].aggregate(lambda term: term.unique().tolist()).reset_index()
# Sneak peek
aggregatedTerms.head(10)
This mapping improves the readability and explainability of the topics generated by Amazon Comprehend, as we can see in the following DataFrame.
Furthermore, we join the topic number, terms, and names to the initial input data, as shown in the following steps.
This returns topic terms and names corresponding to each review. The topic numbers and terms are joined with each review and then further joined back to the original DataFrame we saved in the first notebook.
# Load final dataframe where Comprehend results will be merged to
feedbackTopics = pd.read_csv(S3_FEEDBACK_TOPICS)
# Joining topic numbers to main data
# The index of feedbackTopics is referring to doc field of docTopics dataframe
feedbackTopics = pd.merge(feedbackTopics,
docTopics,
left_index=True,
right_on='doc',
how='left')
# Reviews will now have topic numbers, associated terms and topics names
feedbackTopics = feedbackTopics.merge(aggregatedTerms,
on='topic',
how='left')
feedbackTopics.head()
We generate sentiment for the review text using detect_sentiment
. It inspects text and returns an inference of the prevailing sentiment (POSITIVE, NEUTRAL, MIXED, or NEGATIVE).
def detect_sentiment(text, language_code):
"""Detects sentiment for a given text and language
"""
comprehend_json_out = comprehend.detect_sentiment(Text=text, LanguageCode=language_code)
return comprehend_json_out
# Comprehend output for sentiment in raw json
feedbackTopics['comprehend_sentiment_json_out'] = feedbackTopics['reviewText'].apply(lambda x: detect_sentiment(x, language_code))
# Extracting the exact sentiment from raw Comprehend Json
feedbackTopics['sentiment'] = feedbackTopics['comprehend_sentiment_json_out'].apply(lambda x: x['Sentiment'])
# Sneak peek
feedbackTopics.head(2)
Both topics and sentiments are tightly coupled with reviews. Because we will be aggregating topics and sentiments at product level, we need to create a composite key by combining the topics and sentiments generated by Amazon Comprehend.
# Creating a composite key of topic name and sentiment.
# This is because we are counting frequency of this combination.
feedbackTopics['TopicSentiment'] = feedbackTopics['TopicNames'] + '_' + feedbackTopics['sentiment']
Afterwards, we aggregate at product level and count the composite keys for each product.
This final step helps us better understand the granularity of the reviews per product and categorizing it per topic in an aggregated manner. For instance, we can consider the values shown for topicDF DataFrame. For the first product, of all the reviews for it, overall the customers had a positive experience on product return, size, and comfort. For the second product, the customers had mostly a mixed-to-positive experience on product return and a positive experience on product size.
# Create product id group
asinWiseDF = feedbackTopics.groupby('asin')
# Each product now has a list of topics and sentiment combo (topics can appear multiple times)
topicDF = asinWiseDF['TopicSentiment'].apply(lambda x:list(x)).reset_index()
# Count appreances of topics-sentiment combo for product
topicDF['TopTopics'] = topicDF['TopicSentiment'].apply(Counter)
# Sorting topics-sentiment combo based on their appearance
topicDF['TopTopics'] = topicDF['TopTopics'].apply(lambda x: sorted(x, key=x.get, reverse=True))
# Select Top k topics-sentiment combo for each product/review
topicDF['TopTopics'] = topicDF['TopTopics'].apply(lambda x: x[:TOP_TOPICS])
# Sneak peek
topicDF.head()
Our final DataFrame consists of this topic information and sentiment information joined back to the final DataFrame named feedbackTopics
that we saved on Amazon S3 in our first notebook.
# Adding the topic-sentiment combo back to product metadata
finalDF = S3_FEEDBACK_TOPICS.merge(topicDF, on='asin', how='left')
# Only selecting a subset of fields
finalDF = finalDF[['asin', 'TopTopics', 'category', 'title']]
# Saving the final output locally
finalDF.to_csv(S3_FINAL_OUTPUT, index=False)
Use Amazon QuickSight to visualize the data
You can use QuickSight to visualize the data and generate reports. QuickSight is a business intelligence (BI) service that you can use to consume data from many different sources and build intelligent dashboards. In this example, we generate a QuickSight analysis using the final dataset we produced, as shown in the following example visualizations.
To learn more about Amazon QuickSight, refer to Getting started with Amazon Quicksight.
Cleanup
At the end, we need to shut down the notebook instance we have used in this experiment from AWS Console.
Conclusion
In this post, we demonstrated how to use Amazon Comprehend to analyze product reviews and find the top topics using topic modeling as a technique. Topic modeling enables you to look through multiple topics and organize, understand, and summarize them at scale. You can quickly and easily discover hidden patterns that are present across the data, and then use that insight to make data-driven decisions. You can use topic modeling to solve numerous business problems, such as automatically tagging customer support tickets, routing conversations to the right teams based on topic, detecting the urgency of support tickets, getting better insights from conversations, creating data-driven plans, creating problem-focused content, improving sales strategy, and identifying customer issues and frictions.
These are just a few examples, but you can think of many more business problems that you face in your organization on a daily basis, and how you can use topic modeling with other ML techniques to solve those.
About the Authors
Gurpreet is a Data Scientist with AWS Professional Services based out of Canada. She is passionate about helping customers innovate with Machine Learning and Artificial Intelligence technologies to tap business value and insights from data. In her spare time, she enjoys hiking outdoors and reading books.i
Rushdi Shams is a Data Scientist with AWS Professional Services, Canada. He builds machine learning products for AWS customers. He loves to read and write science fictions.
Wrick Talukdar is a Senior Architect with Amazon Comprehend Service team. He works with AWS customers to help them adopt machine learning on a large scale. Outside of work, he enjoys reading and photography.
Prepare data at scale in Amazon SageMaker Studio using serverless AWS Glue interactive sessions
Amazon SageMaker Studio is the first fully integrated development environment (IDE) for machine learning (ML). It provides a single, web-based visual interface where you can perform all ML development steps, including preparing data and building, training, and deploying models.
AWS Glue is a serverless data integration service that makes it easy to discover, prepare, and combine data for analytics, ML, and application development. AWS Glue enables you to seamlessly collect, transform, cleanse, and prepare data for storage in your data lakes and data pipelines using a variety of capabilities, including built-in transforms.
Data engineers and data scientists can now interactively prepare data at scale using their Studio notebook’s built-in integration with serverless Spark sessions managed by AWS Glue. Starting in seconds and automatically stopping compute when idle, AWS Glue interactive sessions provide an on-demand, highly-scalable, serverless Spark backend to achieve scalable data preparation within Studio. Notable benefits of using AWS Glue interactive sessions on Studio notebooks include:
- No clusters to provision or manage
- No idle clusters to pay for
- No up-front configuration required
- No resource contention for the same development environment
- The exact same serverless Spark runtime and platform as AWS Glue extract, transform, and load (ETL) jobs
In this post, we show you how to prepare data at scale in Studio using serverless AWS Glue interactive sessions.
Solution overview
To implement this solution, you complete the following high-level steps:
- Update your AWS Identity and Access Management (IAM) role permissions.
- Launch an AWS Glue interactive session kernel.
- Configure your interactive session.
- Customize your interactive session and run a scalable data preparation workload.
Update your IAM role permissions
To start, you need to update your Studio user’s IAM execution role with the required permissions. For detailed instructions, refer to Permissions for Glue interactive sessions in SageMaker Studio.
You first add the managed policies to your execution role:
- On the IAM console, choose Roles in the navigation pane.
- Find the Studio execution role that you will use, and choose the role name to go to the role summary page.
- On the Permissions tab, on the Add Permissions menu, choose Attach policies.
- Select the managed policies
AmazonSageMakerFullAccess
andAwsGlueSessionUserRestrictedServiceRole
- Choose Attach policies.
The summary page shows your newly-added managed policies.Now you add a custom policy and attach it to your execution role. - On the Add Permissions menu, choose Create inline policy.
- On the JSON tab, enter the following policy:
- Modify your role’s trust relationship:
Launch an AWS Glue interactive session kernel
If you already have existing users within your Studio domain, you may need to have them shut down and restart their Jupyter Server to pick up the new notebook kernel images.
Upon reloading, you can create a new Studio notebook and select your preferred kernel. The built-in SparkAnalytics 1.0
image should now be available, and you can choose your preferred AWS Glue kernel (Glue Scala Spark or Glue PySpark).
Configure your interactive session
You can easily configure your AWS Glue interactive session with notebook cell magics prior to initialization. Magics are small commands prefixed with % at the start of Jupyter cells that provide shortcuts to control the environment. In AWS Glue interactive sessions, magics are used for all configuration needs, including:
- %region – The AWS Region in which to initialize a session. The default is the Studio Region.
- %iam_role – The IAM role ARN to run your session with. The default is the user’s SageMaker execution role.
- %worker_type – The AWS Glue worker type. The default is standard.
- %number_of_workers – The number of workers that are allocated when a job runs. The default is five.
- %idle_timeout – The number of minutes of inactivity after which a session will time out. The default is 2,880 minutes.
- %additional_python_modules – A comma-separated list of additional Python modules to include in your cluster. This can be from PyPi or Amazon Simple Storage Service (Amazon S3).
- %%configure – A JSON-formatted dictionary consisting of AWS Glue-specific configuration parameters for a session.
For a comprehensive list of configurable magic parameters for this kernel, use the %help
magic within your notebook.
Your AWS Glue interactive session will not start until the first non-magic cell is run.
Customize your interactive session and run a data preparation workload
As an example, the following notebook cells show how you can customize your AWS Glue interactive session and run a scalable data preparation workload. In this example, we perform an ETL task to aggregate air quality data for a given city, grouping by the hour of the day.
We configure our session to save our Spark logs to an S3 bucket for real-time debugging, which we see later in this post. Be sure that the iam_role
that is running your AWS Glue session has write access to the specified S3 bucket.
Next, we load our dataset directly from Amazon S3. Alternatively, you could load data using your AWS Glue Data Catalog.
Finally, we write our transformed dataset to an output bucket location that we defined:
After you’ve completed your work, you can end your AWS Glue interactive session immediately by simply shutting down the Studio notebook kernel, or you could use the %stop_session
magic.
Debugging and Spark UI
In the preceding example, we specified the ”--enable-spark-ui”: “true”
argument along with a "--spark-event-logs-path": location
. This configures our AWS Glue session to record the sessions logs so that we can utilize a Spark UI to monitor and debug our AWS Glue job in real time.
For the process for launching and reading those Spark logs, refer to Launching the Spark history server. In the following screenshot, we’ve launched a local Docker container that has permission to read the S3 bucket the contains our logs. Optionally, you could host an Amazon Elastic Compute Cloud (Amazon EC2) instance to do this, as described in the preceding linked documentation.
Pricing
When you use AWS Glue interactive sessions on Studio notebooks, you’re charged separately for resource usage on AWS Glue and Studio notebooks.
AWS charges for AWS Glue interactive sessions based on how long the session is active and the number of Data Processing Units (DPUs) used. You’re charged an hourly rate for the number of DPUs used to run your workloads, billed in increments of 1 second. AWS Glue interactive sessions assign a default of 5 DPUs and require a minimum of 2 DPUs. There is also a 1-minute minimum billing duration for each interactive session. To see the AWS Glue rates and pricing examples, or to estimate your costs using the AWS Pricing Calculator, see AWS Glue pricing.
Your Studio notebook runs on an EC2 instance and you’re charged for the instance type you choose, based on the duration of use. Studio assigns you a default EC2 instance type of ml-t3-medium when you select the SparkAnalytics
image and associated kernel. You can change the instance type of your Studio notebook to suit your workload. For information about SageMaker Studio pricing, see Amazon SageMaker Pricing.
Conclusion
The native integration of Studio notebooks with AWS Glue interactive sessions facilitates seamless and scalable serverless data preparation for data scientists and data engineers. We encourage you to try out this new functionality in Studio!
See Prepare Data using AWS Glue Interactive Sessions for more information.
About the authors
Sean Morgan is a Senior ML Solutions Architect at AWS. He has experience in the semiconductor and academic research fields, and uses his experience to help customers reach their goals on AWS. In his free time Sean is an activate open source contributor/maintainer and is the special interest group lead for TensorFlow Addons.
Sumedha Swamy is a Principal Product Manager at Amazon Web Services. He leads SageMaker Studio team to build it into the IDE of choice for interactive data science and data engineering workflows. He has spent the past 15 years building customer-obsessed consumer and enterprise products using Machine Learning. In his free time he likes photographing the amazing geology of the American Southwest.
AI on the Stars: Hyperrealistic Avatars Propel Startup to ‘America’s Got Talent’ Finals
More than 6 million pairs of eyes will be on real-time AI avatar technology in this week’s finale of America’s Got Talent — currently the second-most popular primetime TV show in the U.S..
Metaphysic, a member of the NVIDIA Inception global network of technology startups, is one of 11 acts competing for $1 million and a headline slot in AGT’s Las Vegas show in tonight’s final on NBC. It’s the first AI act to reach an AGT finals.
Called “the best act of the series so far” and “one of the most unique things we’ve ever seen on this show” by notoriously tough judge Simon Cowell, the team’s performances involve a demonstration of photorealistic AI avatars, animated in real time by singers on stage.
In Metaphysic’s semifinals act, three singers — Daniel Emmet, Patrick Dailey and John Riesen — lent their voices to AI avatars of Cowell, fellow judge Howie Mandel and host Terry Crews, performing the opera piece “Nessun Dorma.” For the finale, the team plans to “bring back one of the greatest rock and roll icons of all time,” but it’s keeping the audience guessing.
The AGT winner will be announced on Wednesday, Sept. 14.
“Metaphysic’s history-making run on America’s Got Talent has allowed us to showcase the application of AI on one of the most-watched stages in the world,” said the startup’s co-founder and CEO Tom Graham, who appears on the show alongside co-founder Chris Umé.
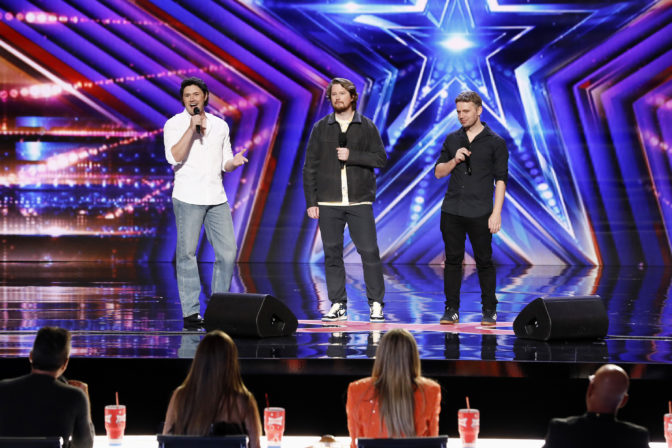
“While overall awareness of synthetic media has grown in recent years, Metaphysic’s AGT performances provide a front-row seat into how this technology could impact the future of everything, from the internet to entertainment to education,” he said.
Capturing Imaginations While Raising AI Awareness
Founded in 2021, London-based Metaphysic is developing AI technologies to help creators build virtual identities and synthetic content that is hyperrealistic, moving beyond the so-called uncanny valley.
The team initially went viral last year for DeepTomCruise, a TikTok channel featuring videos where actor Miles Fisher animated an AI avatar of Tom Cruise. The posts garnered around 100 million views and “provided many people with their first introduction to the incredible capabilities of synthetic media,” Graham said.
By bringing its AI avatars to the AGT stage, the company has been able to reach millions more viewers — with sophisticated camera rigs and performers on stage demonstrating how the technology works live and in real time.
AI, GPU Acceleration Behind the Curtain
Metaphysic’s AI avatar software pipeline includes variants of the popular StyleGAN model developed by NVIDIA Research. The team, which uses the TensorFlow deep learning framework, relies on NVIDIA CUDA software to accelerate its work on NVIDIA GPUs.
“Without NVIDIA hardware and software libraries, we wouldn’t be able to pull off these hyperreal results to the level we have,” said Jo Plaete, director of product innovation at Metaphysic. “The computation provided by our NVIDIA hardware platforms allows us to train larger and more complex models at a speed that allows us to iterate on them quickly, which results in those most perfectly tuned results.”
For both AI model development and inference during live performances, Metaphysic uses NVIDIA DGX systems as well as other workstations and data center configurations with NVIDIA GPUs — including NVIDIA A100 Tensor Core GPUs.
“Excellent hardware support has helped us troubleshoot things really fast when in need,” said Plaete. “And having access to the research and engineering teams helps us get a deeper understanding of the tools and how we can leverage them in our pipelines.”
Following AGT, Metaphysic plans to pursue several collaborations in the entertainment industry. The company has also launched a consumer-facing platform, called Every Anyone, that enables users to create their own hyperrealistic AI avatars.
Discover the latest in AI and metaverse technology by registering free for NVIDIA GTC, running online Sept. 19-22. Metaphysic will be part of the panel “AI for VCs: NVIDIA Inception Global Startup Showcase.”
Header photo by Chris Haston/NBC, courtesy of Metaphysic
The post AI on the Stars: Hyperrealistic Avatars Propel Startup to ‘America’s Got Talent’ Finals appeared first on NVIDIA Blog.
Computing for the health of the planet
The health of the planet is one of the most important challenges facing humankind today. From climate change to unsafe levels of air and water pollution to coastal and agricultural land erosion, a number of serious challenges threaten human and ecosystem health.
Ensuring the health and safety of our planet necessitates approaches that connect scientific, engineering, social, economic, and political aspects. New computational methods can play a critical role by providing data-driven models and solutions for cleaner air, usable water, resilient food, efficient transportation systems, better-preserved biodiversity, and sustainable sources of energy.
The MIT Schwarzman College of Computing is committed to hiring multiple new faculty in computing for climate and the environment, as part of MIT’s plan to recruit 20 climate-focused faculty under its climate action plan. This year the college undertook searches with several departments in the schools of Engineering and Science for shared faculty in computing for health of the planet, one of the six strategic areas of inquiry identified in an MIT-wide planning process to help focus shared hiring efforts. The college also undertook searches for core computing faculty in the Department of Electrical Engineering and Computer Science (EECS).
The searches are part of an ongoing effort by the MIT Schwarzman College of Computing to hire 50 new faculty — 25 shared with other academic departments and 25 in computer science and artificial intelligence and decision-making. The goal is to build capacity at MIT to help more deeply infuse computing and other disciplines in departments.
Four interdisciplinary scholars were hired in these searches. They will join the MIT faculty in the coming year to engage in research and teaching that will advance physical understanding of low-carbon energy solutions, Earth-climate modeling, biodiversity monitoring and conservation, and agricultural management through high-performance computing, transformational numerical methods, and machine-learning techniques.
“By coordinating hiring efforts with multiple departments and schools, we were able to attract a cohort of exceptional scholars in this area to MIT. Each of them is developing and using advanced computational methods and tools to help find solutions for a range of climate and environmental issues,” says Daniel Huttenlocher, dean of the MIT Schwarzman College of Computing and the Henry Warren Ellis Professor of Electrical Engineering and Computer Science. “They will also help strengthen cross-departmental ties in computing across an important, critical area for MIT and the world.”
“These strategic hires in the area of computing for climate and the environment are an incredible opportunity for the college to deepen its academic offerings and create new opportunity for collaboration across MIT,” says Anantha P. Chandrakasan, dean of the MIT School of Engineering and the Vannevar Bush Professor of Electrical Engineering and Computer Science. “The college plays a pivotal role in MIT’s overarching effort to hire climate-focused faculty — introducing the critical role of computing to address the health of the planet through innovative research and curriculum.”
The four new faculty members are:
Sara Beery will join MIT as an assistant professor in the Faculty of Artificial Intelligence and Decision-Making in EECS in September 2023. Beery received her PhD in computing and mathematical sciences at Caltech in 2022, where she was advised by Pietro Perona. Her research focuses on building computer vision methods that enable global-scale environmental and biodiversity monitoring across data modalities, tackling real-world challenges including strong spatiotemporal correlations, imperfect data quality, fine-grained categories, and long-tailed distributions. She partners with nongovernmental organizations and government agencies to deploy her methods in the wild worldwide and works toward increasing the diversity and accessibility of academic research in artificial intelligence through interdisciplinary capacity building and education.
Priya Donti will join MIT as an assistant professor in the faculties of Electrical Engineering and Artificial Intelligence and Decision-Making in EECS in academic year 2023-24. Donti recently finished her PhD in the Computer Science Department and the Department of Engineering and Public Policy at Carnegie Mellon University, co-advised by Zico Kolter and Inês Azevedo. Her work focuses on machine learning for forecasting, optimization, and control in high-renewables power grids. Specifically, her research explores methods to incorporate the physics and hard constraints associated with electric power systems into deep learning models. Donti is also co-founder and chair of Climate Change AI, a nonprofit initiative to catalyze impactful work at the intersection of climate change and machine learning that is currently running through the Cornell Tech Runway Startup Postdoc Program.
Ericmoore Jossou will join MIT as an assistant professor in a shared position between the Department of Nuclear Science and Engineering and the faculty of electrical engineering in EECS in July 2023. He is currently an assistant scientist at the Brookhaven National Laboratory, a U.S. Department of Energy-affiliated lab that conducts research in nuclear and high energy physics, energy science and technology, environmental and bioscience, nanoscience, and national security. His research at MIT will focus on understanding the processing-structure-properties correlation of materials for nuclear energy applications through advanced experiments, multiscale simulations, and data science. Jossou obtained his PhD in mechanical engineering in 2019 from the University of Saskatchewan.
Sherrie Wang will join MIT as an assistant professor in a shared position between the Department of Mechanical Engineering and the Institute for Data, Systems, and Society in academic year 2023-24. Wang is currently a Ciriacy-Wantrup Postdoctoral Fellow at the University of California at Berkeley, hosted by Solomon Hsiang and the Global Policy Lab. She develops machine learning for Earth observation data. Her primary application areas are improving agricultural management and forecasting climate phenomena. She obtained her PhD in computational and mathematical engineering from Stanford University in 2021, where she was advised by David Lobell.
Computing for the health of the planet
The health of the planet is one of the most important challenges facing humankind today. From climate change to unsafe levels of air and water pollution to coastal and agricultural land erosion, a number of serious challenges threaten human and ecosystem health.
Ensuring the health and safety of our planet necessitates approaches that connect scientific, engineering, social, economic, and political aspects. New computational methods can play a critical role by providing data-driven models and solutions for cleaner air, usable water, resilient food, efficient transportation systems, better-preserved biodiversity, and sustainable sources of energy.
The MIT Schwarzman College of Computing is committed to hiring multiple new faculty in computing for climate and the environment, as part of MIT’s plan to recruit 20 climate-focused faculty under its climate action plan. This year the college undertook searches with several departments in the schools of Engineering and Science for shared faculty in computing for health of the planet, one of the six strategic areas of inquiry identified in an MIT-wide planning process to help focus shared hiring efforts. The college also undertook searches for core computing faculty in the Department of Electrical Engineering and Computer Science (EECS).
The searches are part of an ongoing effort by the MIT Schwarzman College of Computing to hire 50 new faculty — 25 shared with other academic departments and 25 in computer science and artificial intelligence and decision-making. The goal is to build capacity at MIT to help more deeply infuse computing and other disciplines in departments.
Four interdisciplinary scholars were hired in these searches. They will join the MIT faculty in the coming year to engage in research and teaching that will advance physical understanding of low-carbon energy solutions, Earth-climate modeling, biodiversity monitoring and conservation, and agricultural management through high-performance computing, transformational numerical methods, and machine-learning techniques.
“By coordinating hiring efforts with multiple departments and schools, we were able to attract a cohort of exceptional scholars in this area to MIT. Each of them is developing and using advanced computational methods and tools to help find solutions for a range of climate and environmental issues,” says Daniel Huttenlocher, dean of the MIT Schwarzman College of Computing and the Henry Warren Ellis Professor of Electrical Engineering and Computer Science. “They will also help strengthen cross-departmental ties in computing across an important, critical area for MIT and the world.”
“These strategic hires in the area of computing for climate and the environment are an incredible opportunity for the college to deepen its academic offerings and create new opportunity for collaboration across MIT,” says Anantha P. Chandrakasan, dean of the MIT School of Engineering and the Vannevar Bush Professor of Electrical Engineering and Computer Science. “The college plays a pivotal role in MIT’s overarching effort to hire climate-focused faculty — introducing the critical role of computing to address the health of the planet through innovative research and curriculum.”
The four new faculty members are:
Sara Beery will join MIT as an assistant professor in the Faculty of Artificial Intelligence and Decision-Making in EECS in September 2023. Beery received her PhD in computing and mathematical sciences at Caltech in 2022, where she was advised by Pietro Perona. Her research focuses on building computer vision methods that enable global-scale environmental and biodiversity monitoring across data modalities, tackling real-world challenges including strong spatiotemporal correlations, imperfect data quality, fine-grained categories, and long-tailed distributions. She partners with nongovernmental organizations and government agencies to deploy her methods in the wild worldwide and works toward increasing the diversity and accessibility of academic research in artificial intelligence through interdisciplinary capacity building and education.
Priya Donti will join MIT as an assistant professor in the faculties of Electrical Engineering and Artificial Intelligence and Decision-Making in EECS in academic year 2023-24. Donti recently finished her PhD in the Computer Science Department and the Department of Engineering and Public Policy at Carnegie Mellon University, co-advised by Zico Kolter and Inês Azevedo. Her work focuses on machine learning for forecasting, optimization, and control in high-renewables power grids. Specifically, her research explores methods to incorporate the physics and hard constraints associated with electric power systems into deep learning models. Donti is also co-founder and chair of Climate Change AI, a nonprofit initiative to catalyze impactful work at the intersection of climate change and machine learning that is currently running through the Cornell Tech Runway Startup Postdoc Program.
Ericmoore Jossou will join MIT as an assistant professor in a shared position between the Department of Nuclear Science and Engineering and the faculty of electrical engineering in EECS in July 2023. He is currently an assistant scientist at the Brookhaven National Laboratory, a U.S. Department of Energy-affiliated lab that conducts research in nuclear and high energy physics, energy science and technology, environmental and bioscience, nanoscience, and national security. His research at MIT will focus on understanding the processing-structure-properties correlation of materials for nuclear energy applications through advanced experiments, multiscale simulations, and data science. Jossou obtained his PhD in mechanical engineering in 2019 from the University of Saskatchewan.
Sherrie Wang will join MIT as an assistant professor in a shared position between the Department of Mechanical Engineering and the Institute for Data, Systems, and Society in academic year 2023-24. Wang is currently a Ciriacy-Wantrup Postdoctoral Fellow at the University of California at Berkeley, hosted by Solomon Hsiang and the Global Policy Lab. She develops machine learning for Earth observation data. Her primary application areas are improving agricultural management and forecasting climate phenomena. She obtained her PhD in computational and mathematical engineering from Stanford University in 2021, where she was advised by David Lobell.
Amazon scientists win best-paper award for ad auction simulator
Paper introduces a unified view of the learning-to-bid problem and presents AuctionGym, a simulation environment that enables reproducible validation of new solutions.Read More