New auto-scheduler speeds optimization process sixfold while improving performance of resulting code up to 70%.Read More
GeForce NOW Supports Over 1,400 Games Streaming Instantly
This GFN Thursday marks a milestone: With the addition of six new titles this week, more than 1,400 games are now available to stream from the GeForce NOW library.
Plus, GeForce NOW members streaming to supported Smart TVs from Samsung and LG can get into their games faster with an improved user interface.
Your Games, Your Way
With more than 1,400 games streaming instantly on GeForce NOW, there’s always something new to play.
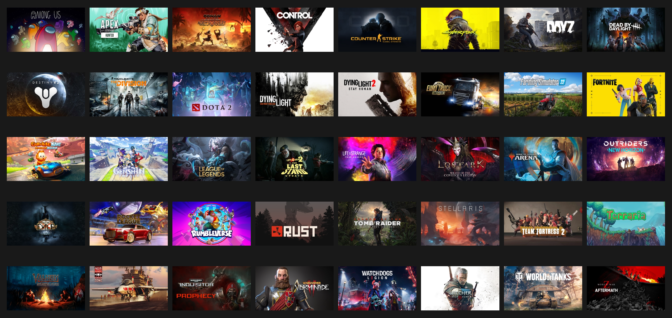
Enjoy stunning stories like Mass Effect Legendary Edition or Life is Strange: True Colors, streaming to PCs and even Macs in 4K resolution with an RTX 3080 membership. Group up with friends in Lost Ark or betray them for fun in Among Us. Squad up for victory in Apex Legends, Rocket League and Counter-Strike: Global Offensive — and don’t worry about lagging behind, thanks to ultra-low latency.
For those craving something spooky, have a drop-dead good time ghost hunting in Phasmophobia or struggle to survive and slay in Dead by Daylight. Games like these sound scary good in 5.1 and 7.1 surround sound for Priority and 3080 members.
Build out your library, starting with over 100 free-to-play titles like League of Legends and Rumbleverse. RTX 3080 and Priority members can also experience real-time ray tracing in games like Dying Light 2, Loopmancer and Cyberpunk 2077, which launched a new 1.6 update this week, bringing even more content to Night City.
Take the action on the go with mobile devices. Fortnite on GeForce NOW with touch controls on mobile is available to all members, streaming through the Safari web browser on iOS and the GeForce NOW Android app. Or tap your way through Teyvet in Genshin Impact, streaming to mobile devices with touch controls.
With new games arriving on the cloud every week, the choices are endless.
Stream on TVs
GeForce NOW members streaming to Samsung and LG TVs can now quickly discover and easily launch top games through an improved UI.
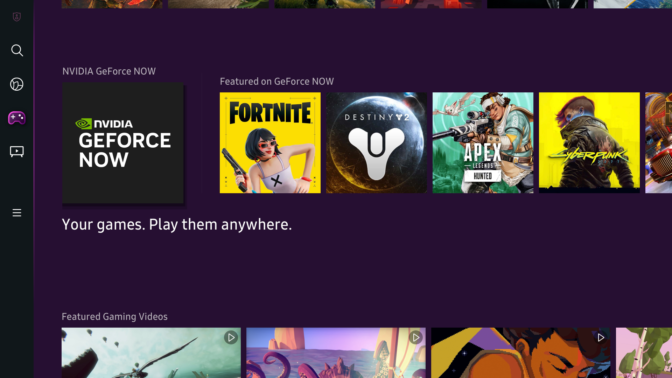
Samsung has integrated a “Featured on GeForce NOW” row in the Samsung Gaming Hub, streaming on select 2022 4K TVs. The list is curated and updated regularly — showcasing new, popular and recently released games. GeForce NOW is also integrated into other rows, like “Popular Games,” which Samsung also curates weekly. Pick out a game from these menus and easily launch the GeForce NOW app.
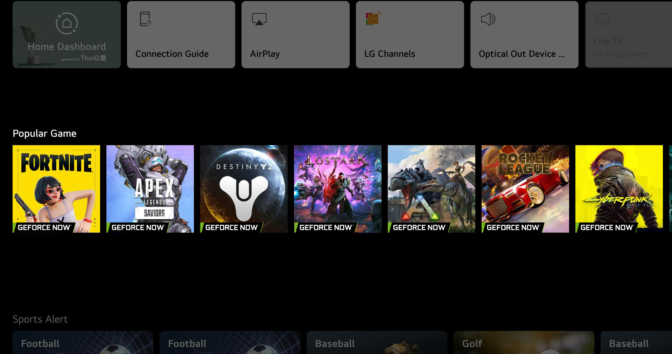
LG updated its UI with a home-screen “Gaming Shelf.” This addition brings GeForce NOW titles right onto the home screen, adding a new layer of game discoverability for members streaming to supported 2022 and 2021 LG TVs. Members with supported TVs can download the GeForce NOW app and check out the new UI today.
Revolutionize the Weekend
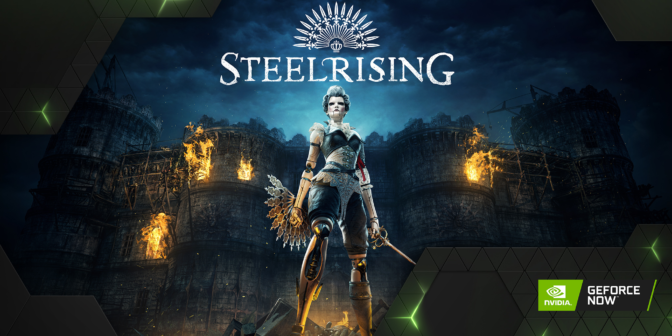
Charge into the weekend with six new titles streaming from the cloud:
- Gloomwood (New release on Steam)
- TRAIL OUT (New release on Steam)
- Shatterline (New release on Steam, Sept 8)
- Steelrising (New release on Steam and Epic Games Store, Sept. 8)
- Broken Pieces (New release on Steam, Sept. 9)
- Realm Royale Reforged (Epic Games Store)
What are you planning to play this weekend? Let us know on Twitter or in the comments below.
The post GeForce NOW Supports Over 1,400 Games Streaming Instantly appeared first on NVIDIA Blog.
AI system makes models like DALL-E 2 more creative
The internet had a collective feel-good moment with the introduction of DALL-E, an artificial intelligence-based image generator inspired by artist Salvador Dali and the lovable robot WALL-E that uses natural language to produce whatever mysterious and beautiful image your heart desires. Seeing typed-out inputs like “smiling gopher holding an ice cream cone” instantly spring to life clearly resonated with the world.
Getting said smiling gopher and attributes to pop up on your screen is not a small task. DALL-E 2 uses something called a diffusion model, where it tries to encode the entire text into one description to generate an image. But once the text has a lot of more details, it’s hard for a single description to capture it all. Moreover, while they’re highly flexible, they sometimes struggle to understand the composition of certain concepts, like confusing the attributes or relations between different objects.
To generate more complex images with better understanding, scientists from MIT’s Computer Science and Artificial Intelligence Laboratory (CSAIL) structured the typical model from a different angle: they added a series of models together, where they all cooperate to generate desired images capturing multiple different aspects as requested by the input text or labels. To create an image with two components, say, described by two sentences of description, each model would tackle a particular component of the image.
The seemingly magical models behind image generation work by suggesting a series of iterative refinement steps to get to the desired image. It starts with a “bad” picture and then gradually refines it until it becomes the selected image. By composing multiple models together, they jointly refine the appearance at each step, so the result is an image that exhibits all the attributes of each model. By having multiple models cooperate, you can get much more creative combinations in the generated images.
Take, for example, a red truck and a green house. The model will confuse the concepts of red truck and green house when these sentences get very complicated. A typical generator like DALL-E 2 might make a green truck and a red house, so it’ll swap these colors around. The team’s approach can handle this type of binding of attributes with objects, and especially when there are multiple sets of things, it can handle each object more accurately.
“The model can effectively model object positions and relational descriptions, which is challenging for existing image-generation models. For example, put an object and a cube in a certain position and a sphere in another. DALL-E 2 is good at generating natural images but has difficulty understanding object relations sometimes,” says MIT CSAIL PhD student and co-lead author Shuang Li, “Beyond art and creativity, perhaps we could use our model for teaching. If you want to tell a child to put a cube on top of a sphere, and if we say this in language, it might be hard for them to understand. But our model can generate the image and show them.”
Making Dali proud
Composable Diffusion — the team’s model — uses diffusion models alongside compositional operators to combine text descriptions without further training. The team’s approach more accurately captures text details than the original diffusion model, which directly encodes the words as a single long sentence. For example, given “a pink sky” AND “a blue mountain in the horizon” AND “cherry blossoms in front of the mountain,” the team’s model was able to produce that image exactly, whereas the original diffusion model made the sky blue and everything in front of the mountains pink.
“The fact that our model is composable means that you can learn different portions of the model, one at a time. You can first learn an object on top of another, then learn an object to the right of another, and then learn something left of another,” says co-lead author and MIT CSAIL PhD student Yilun Du. “Since we can compose these together, you can imagine that our system enables us to incrementally learn language, relations, or knowledge, which we think is a pretty interesting direction for future work.”
While it showed prowess in generating complex, photorealistic images, it still faced challenges since the model was trained on a much smaller dataset than those like DALL-E 2, so there were some objects it simply couldn’t capture.
Now that Composable Diffusion can work on top of generative models, such as DALL-E 2, the scientists want to explore continual learning as a potential next step. Given that more is usually added to object relations, they want to see if diffusion models can start to “learn” without forgetting previously learned knowledge — to a place where the model can produce images with both the previous and new knowledge.
“This research proposes a new method for composing concepts in text-to-image generation not by concatenating them to form a prompt, but rather by computing scores with respect to each concept and composing them using conjunction and negation operators,” says Mark Chen, co-creator of DALL-E 2 and research scientist at OpenAI. “This is a nice idea that leverages the energy-based interpretation of diffusion models so that old ideas around compositionality using energy-based models can be applied. The approach is also able to make use of classifier-free guidance, and it is surprising to see that it outperforms the GLIDE baseline on various compositional benchmarks and can qualitatively produce very different types of image generations.”
“Humans can compose scenes including different elements in a myriad of ways, but this task is challenging for computers,” says Bryan Russel, research scientist at Adobe Systems. “This work proposes an elegant formulation that explicitly composes a set of diffusion models to generate an image given a complex natural language prompt.”
Alongside Li and Du, the paper’s co-lead authors are Nan Liu, a master’s student in computer science at the University of Illinois at Urbana-Champaign, and MIT professors Antonio Torralba and Joshua B. Tenenbaum. They will present the work at the 2022 European Conference on Computer Vision.
The research was supported by Raytheon BBN Technologies Corp., Mitsubishi Electric Research Laboratory, and DEVCOM Army Research Laboratory.
AI system makes models like DALL-E 2 more creative
The internet had a collective feel-good moment with the introduction of DALL-E, an artificial intelligence-based image generator inspired by artist Salvador Dali and the lovable robot WALL-E that uses natural language to produce whatever mysterious and beautiful image your heart desires. Seeing typed-out inputs like “smiling gopher holding an ice cream cone” instantly spring to life clearly resonated with the world.
Getting said smiling gopher and attributes to pop up on your screen is not a small task. DALL-E 2 uses something called a diffusion model, where it tries to encode the entire text into one description to generate an image. But once the text has a lot of more details, it’s hard for a single description to capture it all. Moreover, while they’re highly flexible, they sometimes struggle to understand the composition of certain concepts, like confusing the attributes or relations between different objects.
To generate more complex images with better understanding, scientists from MIT’s Computer Science and Artificial Intelligence Laboratory (CSAIL) structured the typical model from a different angle: they added a series of models together, where they all cooperate to generate desired images capturing multiple different aspects as requested by the input text or labels. To create an image with two components, say, described by two sentences of description, each model would tackle a particular component of the image.
The seemingly magical models behind image generation work by suggesting a series of iterative refinement steps to get to the desired image. It starts with a “bad” picture and then gradually refines it until it becomes the selected image. By composing multiple models together, they jointly refine the appearance at each step, so the result is an image that exhibits all the attributes of each model. By having multiple models cooperate, you can get much more creative combinations in the generated images.
Take, for example, a red truck and a green house. The model will confuse the concepts of red truck and green house when these sentences get very complicated. A typical generator like DALL-E 2 might make a green truck and a red house, so it’ll swap these colors around. The team’s approach can handle this type of binding of attributes with objects, and especially when there are multiple sets of things, it can handle each object more accurately.
“The model can effectively model object positions and relational descriptions, which is challenging for existing image-generation models. For example, put an object and a cube in a certain position and a sphere in another. DALL-E 2 is good at generating natural images but has difficulty understanding object relations sometimes,” says MIT CSAIL PhD student and co-lead author Shuang Li, “Beyond art and creativity, perhaps we could use our model for teaching. If you want to tell a child to put a cube on top of a sphere, and if we say this in language, it might be hard for them to understand. But our model can generate the image and show them.”
Making Dali proud
Composable Diffusion — the team’s model — uses diffusion models alongside compositional operators to combine text descriptions without further training. The team’s approach more accurately captures text details than the original diffusion model, which directly encodes the words as a single long sentence. For example, given “a pink sky” AND “a blue mountain in the horizon” AND “cherry blossoms in front of the mountain,” the team’s model was able to produce that image exactly, whereas the original diffusion model made the sky blue and everything in front of the mountains pink.
“The fact that our model is composable means that you can learn different portions of the model, one at a time. You can first learn an object on top of another, then learn an object to the right of another, and then learn something left of another,” says co-lead author and MIT CSAIL PhD student Yilun Du. “Since we can compose these together, you can imagine that our system enables us to incrementally learn language, relations, or knowledge, which we think is a pretty interesting direction for future work.”
While it showed prowess in generating complex, photorealistic images, it still faced challenges since the model was trained on a much smaller dataset than those like DALL-E 2, so there were some objects it simply couldn’t capture.
Now that Composable Diffusion can work on top of generative models, such as DALL-E 2, the scientists want to explore continual learning as a potential next step. Given that more is usually added to object relations, they want to see if diffusion models can start to “learn” without forgetting previously learned knowledge — to a place where the model can produce images with both the previous and new knowledge.
“This research proposes a new method for composing concepts in text-to-image generation not by concatenating them to form a prompt, but rather by computing scores with respect to each concept and composing them using conjunction and negation operators,” says Mark Chen, co-creator of DALL-E 2 and research scientist at OpenAI. “This is a nice idea that leverages the energy-based interpretation of diffusion models so that old ideas around compositionality using energy-based models can be applied. The approach is also able to make use of classifier-free guidance, and it is surprising to see that it outperforms the GLIDE baseline on various compositional benchmarks and can qualitatively produce very different types of image generations.”
“Humans can compose scenes including different elements in a myriad of ways, but this task is challenging for computers,” says Bryan Russel, research scientist at Adobe Systems. “This work proposes an elegant formulation that explicitly composes a set of diffusion models to generate an image given a complex natural language prompt.”
Alongside Li and Du, the paper’s co-lead authors are Nan Liu, a master’s student in computer science at the University of Illinois at Urbana-Champaign, and MIT professors Antonio Torralba and Joshua B. Tenenbaum. They will present the work at the 2022 European Conference on Computer Vision.
The research was supported by Raytheon BBN Technologies Corp., Mitsubishi Electric Research Laboratory, and DEVCOM Army Research Laboratory.
My journey from DeepMind intern to mentor
Former intern turned intern manager, Richard Everett, describes his journey to DeepMind, sharing tips and advice for aspiring DeepMinders. The 2023 internship applications will open on the 16th September, please visit https://dpmd.ai/internshipsatdeepmind for more information.Read More
My journey from DeepMind intern to mentor
Former intern turned intern manager, Richard Everett, describes his journey to DeepMind, sharing tips and advice for aspiring DeepMinders. The 2023 internship applications will open on the 16th September, please visit https://dpmd.ai/internshipsatdeepmind for more information.Read More
Announcing TensorFlow Official Build Collaborators
Posted by Rostam Dinyari, Nitin Srinivasan, Douglas Yarrington and Rishika Sinha of the TensorFlow team
Starting with TensorFlow 2.10, we are excited to announce our collaboration with Intel, AWS, ARM, and Linaro to develop official TensorFlow builds. This means that when you pip install TensorFlow on Windows Native and Linux Aarch64 hosts, you will receive a build of TensorFlow that has been reviewed and vetted by these platform experts. This happens transparently, and there are no changes to your workflow . We’ve updated the pip install scripts so it’s automatic for you.
Official builds are TensorFlow releases that follow rigorous functional and performance testing standards Google engineers and our collaborators publish with each release, which we align with our published support expectations under the SIG Build forum. Collaborators monitor the builds daily and publish artifacts to the community in coordination with the overall TensorFlow release schedule.
For the majority of use cases, there will be no changes to the behavior of pip install or pip uninstall TensorFlow. However, for Windows Native and Linux Aarch64 based systems an additional pip uninstall step may be needed. You can find details about install, uninstall and other best practices on tensorflow.org/install/pip.
Over time, we expect the number of collaborators to expand but for now we want to share with you the progress we have made together to release increasingly performant and robust builds for these important platforms. You can learn more about each of the collaborations below.
Intel Collaboration
We are pleased to share that Intel has joined the 3P Official Build program to take ownership over Windows Native CPU builds. This will include responsibility for managing both nightly and final production releases. We and Intel do not expect this to disrupt end user experiences; users simply install TensorFlow as usual and the Intel produced Python binary artifacts (wheel files) will be correctly installed.
AWS, ARM and Linaro Collaboration
We are especially pleased to announce the availability of official builds for ARM Aarch64, specifically tuned for AWS Graviton instances. Together, the experts at Linaro have supported Google, AWS and ARM to ensure a highly performant version of TensorFlow is available on the emerging class of Aarch64 devices.
Next steps
Transfer learning for TensorFlow image classification models in Amazon SageMaker
Amazon SageMaker provides a suite of built-in algorithms, pre-trained models, and pre-built solution templates to help data scientists and machine learning (ML) practitioners get started on training and deploying ML models quickly. You can use these algorithms and models for both supervised and unsupervised learning. They can process various types of input data, including tabular, image, and text.
Starting today, SageMaker provides a new built-in algorithm for image classification: Image Classification – TensorFlow. It is a supervised learning algorithm that supports transfer learning for many pre-trained models available in TensorFlow Hub. It takes an image as input and outputs probability for each of the class labels. You can fine-tune these pre-trained models using transfer learning even when a large number of training images aren’t available. It’s available through the SageMaker built-in algorithms as well as through the SageMaker JumpStart UI inside Amazon SageMaker Studio. For more information, refer to its documentation Image Classification – TensorFlow and the example notebook Introduction to SageMaker TensorFlow – Image Classification.
Image classification with TensorFlow in SageMaker provides transfer learning on many pre-trained models available in TensorFlow Hub. According to the number of class labels in the training data, a classification layer is attached to the pre-trained TensorFlow Hub model. The classification layer consists of a dropout layer and a dense layer, which is a fully connected layer with 2-norm regularizer that is initialized with random weights. The model training has hyperparameters for the dropout rate of the dropout layer and the L2 regularization factor for the dense layer. Then either the whole network, including the pre-trained model, or only the top classification layer can be fine-tuned on the new training data. In this transfer learning mode, you can achieve training even with a smaller dataset.
How to use the new TensorFlow image classification algorithm
This section describes how to use the TensorFlow image classification algorithm with the SageMaker Python SDK. For information on how to use it from the Studio UI, see SageMaker JumpStart.
The algorithm supports transfer learning for the pre-trained models listed in TensorFlow Hub Models. Each model is identified by a unique model_id
. The following code shows how to fine-tune MobileNet V2 1.00 224 identified by model_id
tensorflow-ic-imagenet-mobilenet-v2-100-224-classification-4
on a custom training dataset. For each model_id
, in order to launch a SageMaker training job through the Estimator class of the SageMaker Python SDK, you need to fetch the Docker image URI, training script URI, and pre-trained model URI through the utility functions provided in SageMaker. The training script URI contains all the necessary code for data processing, loading the pre-trained model, model training, and saving the trained model for inference. The pre-trained model URI contains the pre-trained model architecture definition and the model parameters. Note that the Docker image URI and the training script URI are the same for all the TensorFlow image classification models. The pre-trained model URI is specific to the particular model. The pre-trained model tarballs have been pre-downloaded from TensorFlow Hub and saved with the appropriate model signature in Amazon Simple Storage Service (Amazon S3) buckets, such that the training job runs in network isolation. See the following code:
With these model-specific training artifacts, you can construct an object of the Estimator class:
Next, for transfer learning on your custom dataset, you might need to change the default values of the training hyperparameters, which are listed in Hyperparameters. You can fetch a Python dictionary of these hyperparameters with their default values by calling hyperparameters.retrieve_default
, update them as needed, and then pass them to the Estimator class. Note that the default values of some of the hyperparameters are different for different models. For large models, the default batch size is smaller and the train_only_top_layer
hyperparameter is set to True
. The hyperparameter Train_only_top_layer
defines which model parameters change during the fine-tuning process. If train_only_top_layer
is True
, parameters of the classification layers change and the rest of the parameters remain constant during the fine-tuning process. On the other hand, if train_only_top_layer
is False
, all parameters of the model are fine-tuned. See the following code:
The following code provides a default training dataset hosted in S3 buckets. We provide the tf_flowers
dataset as a default dataset for fine-tuning the models. The dataset comprises images of five types of flowers. The dataset has been downloaded from TensorFlow under the Apache 2.0 License.
Finally, to launch the SageMaker training job for fine-tuning the model, call .fit
on the object of the Estimator class, while passing the S3 location of the training dataset:
For more information about how to use the new SageMaker TensorFlow image classification algorithm for transfer learning on a custom dataset, deploy the fine-tuned model, run inference on the deployed model, and deploy the pre-trained model as is without first fine-tuning on a custom dataset, see the following example notebook: Introduction to SageMaker TensorFlow – Image Classification.
Input/output interface for the TensorFlow image classification algorithm
You can fine-tune each of the pre-trained models listed in TensorFlow Hub Models to any given dataset comprising images belonging to any number of classes. The objective is to minimize prediction error on the input data. The model returned by fine-tuning can be further deployed for inference. The following are the instructions for how the training data should be formatted for input to the model:
- Input – A directory with as many sub-directories as the number of classes. Each sub-directory should have images belonging to that class in .jpg, .jpeg, or .png format.
- Output – A fine-tuned model that can be deployed for inference or can be further trained using incremental training. A preprocessing and postprocessing signature is added to the fine-tuned model such that it takes raw .jpg image as input and returns class probabilities. A file mapping class indexes to class labels is saved along with the models.
The input directory should look like the following example if the training data contains images from two classes: roses
and dandelion
. The S3 path should look like s3://bucket_name/input_directory/
. Note the trailing /
is required. The names of the folders and roses
, dandelion
, and the .jpg filenames can be anything. The label mapping file that is saved along with the trained model on the S3 bucket maps the folder names roses and dandelion to the indexes in the list of class probabilities the model outputs. The mapping follows alphabetical ordering of the folder names. In the following example, index 0 in the model output list corresponds to dandelion
, and index 1 corresponds to roses
.
Inference with the TensorFlow image classification algorithm
The generated models can be hosted for inference and support encoded .jpg, .jpeg, and .png image formats as the application/x-image
content type. The input image is resized automatically. The output contains the probability values, the class labels for all classes, and the predicted label corresponding to the class index with the highest probability, encoded in JSON format. The TensorFlow image classification model processes a single image per request and outputs only one line in the JSON. The following is an example of a response in JSON:
If accept
is set to application/json
, then the model only outputs probabilities. For more details on training and inference, see the sample notebook Introduction to SageMaker TensorFlow – Image Classification.
Use SageMaker built-in algorithms through the JumpStart UI
You can also use SageMaker TensorFlow image classification and any of the other built-in algorithms with a few clicks via the JumpStart UI. JumpStart is a SageMaker feature that allows you to train and deploy built-in algorithms and pre-trained models from various ML frameworks and model hubs through a graphical interface. It also allows you to deploy fully fledged ML solutions that string together ML models and various other AWS services to solve a targeted use case. Check out Run text classification with Amazon SageMaker JumpStart using TensorFlow Hub and Hugging Face models to find out how to use JumpStart to train an algorithm or pre-trained model in a few clicks.
Conclusion
In this post, we announced the launch of the SageMaker TensorFlow image classification built-in algorithm. We provided example code on how to do transfer learning on a custom dataset using a pre-trained model from TensorFlow Hub using this algorithm. For more information, check out documentation and the example notebook.
About the authors
Dr. Ashish Khetan is a Senior Applied Scientist with Amazon SageMaker built-in algorithms and helps develop machine learning algorithms. He got his PhD from University of Illinois Urbana-Champaign. He is an active researcher in machine learning and statistical inference, and has published many papers in NeurIPS, ICML, ICLR, JMLR, ACL, and EMNLP conferences.
Dr. Vivek Madan is an Applied Scientist with the Amazon SageMaker JumpStart team. He got his PhD from University of Illinois Urbana-Champaign and was a Post Doctoral Researcher at Georgia Tech. He is an active researcher in machine learning and algorithm design, and has published papers in EMNLP, ICLR, COLT, FOCS, and SODA conferences.
João Moura is an AI/ML Specialist Solutions Architect at Amazon Web Services. He is mostly focused on NLP use cases and helping customers optimize deep learning model training and deployment. He is also an active proponent of low-code ML solutions and ML-specialized hardware.
Raju Penmatcha is a Senior AI/ML Specialist Solutions Architect at AWS. He works with education, government, and nonprofit customers on machine learning and artificial intelligence related projects, helping them build solutions using AWS. When not helping customers, he likes traveling to new places.
Improve transcription accuracy of customer-agent calls with custom vocabulary in Amazon Transcribe
Many AWS customers have been successfully using Amazon Transcribe to accurately, efficiently, and automatically convert their customer audio conversations to text, and extract actionable insights from them. These insights can help you continuously enhance the processes and products that directly improve the quality and experience for your customers.
In many countries, such as India, English is not the primary language of communication. Indian customer conversations contain regional languages like Hindi, with English words and phrases spoken randomly throughout the calls. In the source media files, there can be proper nouns, domain-specific acronyms, words, or phrases that the default Amazon Transcribe model isn’t aware of. Transcriptions for such media files can have inaccurate spellings for those words.
In this post, we demonstrate how you can provide more information to Amazon Transcribe with custom vocabularies to update the way Amazon Transcribe handles transcription of your audio files with business-specific terminology. We show the steps to improve the accuracy of transcriptions for Hinglish calls (Indian Hindi calls containing Indian English words and phrases). You can use the same process to transcribe audio calls with any language supported by Amazon Transcribe. After you create custom vocabularies, you can transcribe audio calls with accuracy and at scale by using our post call analytics solution, which we discuss more later in this post.
Solution overview
We use the following Indian Hindi audio call (SampleAudio.wav
) with random English words to demonstrate the process.
We then walk you through the following high-level steps:
- Transcribe the audio file using the default Amazon Transcribe Hindi model.
- Measure model accuracy.
- Train the model with custom vocabulary.
- Measure the accuracy of the trained model.
Prerequisites
Before we get started, we need to confirm that the input audio file meets the transcribe data input requirements.
A monophonic recording, also referred to as mono, contains one audio signal, in which all the audio elements of the agent and the customer are combined into one channel. A stereophonic recording, also referred to as stereo, contains two audio signals to capture the audio elements of the agent and the customer in two separate channels. Each agent-customer recording file contains two audio channels, one for the agent and one for the customer.
Low-fidelity audio recordings, such as telephone recordings, typically use 8,000 Hz sample rates. Amazon Transcribe supports processing mono recorded and also high-fidelity audio files with sample rates between 16,000–48,000 Hz.
For improved transcription results and to clearly distinguish the words spoken by the agent and the customer, we recommend using audio files recorded at 8,000 Hz sample rate and are stereo channel separated.
You can use a tool like ffmpeg to validate your input audio files from the command line:
In the returned response, check the line starting with Stream in the Input section, and confirm that the audio files are 8,000 Hz and stereo channel separated:
When you build a pipeline to process a large number of audio files, you can automate this step to filter files that don’t meet the requirements.
As an additional prerequisite step, create an Amazon Simple Storage Service (Amazon S3) bucket to host the audio files to be transcribed. For instructions, refer to Create your first S3 bucket.Then upload the audio file to the S3 bucket.
Transcribe the audio file with the default model
Now we can start an Amazon Transcribe call analytics job using the audio file we uploaded.In this example, we use the AWS Management Console to transcribe the audio file.You can also use the AWS Command Line Interface (AWS CLI) or AWS SDK.
- On the Amazon Transcribe console, choose Call analytics in the navigation pane.
- Choose Call analytics jobs.
- Choose Create job.
- For Name, enter a name.
- For Language settings, select Specific language.
- For Language, choose Hindi, IN (hi-IN).
- For Model type, select General model.
- For Input file location on S3, browse to the S3 bucket containing the uploaded audio file.
- In the Output data section, leave the defaults.
- In the Access permissions section, select Create an IAM role.
- Create a new AWS Identity and Access Management (IAM) role named HindiTranscription that provides Amazon Transcribe service permissions to read the audio files from the S3 bucket and use the AWS Key Management Service (AWS KMS) key to decrypt.
- In the Configure job section, leave the defaults, including Custom vocabulary deselected.
- Choose Create job to transcribe the audio file.
When the status of the job is Complete, you can review the transcription by choosing the job (SampleAudio).
The customer and the agent sentences are clearly separated out, which helps us identify whether the customer or the agent spoke any specific words or phrases.
Measure model accuracy
Word error rate (WER) is the recommended and most commonly used metric for evaluating the accuracy of Automatic Speech Recognition (ASR) systems. The goal is to reduce the WER as much possible to improve the accuracy of the ASR system.
To calculate WER, complete the following steps. This post uses the open-source asr-evaluation evaluation tool to calculate WER, but other tools such as SCTK or JiWER are also available.
-
Install the
asr-evaluation
tool, which makes the wer script available on your command line.
Use a command line on macOS or Linux platforms to run the wer commands shown later in the post. - Copy the transcript from the Amazon Transcribe job details page to a text file named
hypothesis.txt
.
When you copy the transcription from the console, you’ll notice a new line character between the wordsAgent :, Customer :,
and the Hindi script.
The new line characters have been removed to save space in this post. If you choose to use the text as is from the console, make sure that the reference text file you create also has the new line characters, because the wer tool compares line by line. - Review the entire transcript and identify any words or phrases that need to be corrected:
Customer : हेलो,
Agent : गुड मोर्निग इंडिया ट्रेवल एजेंसी सेम है। लावन्या बात कर रही हूँ किस तरह से मैं आपकी सहायता कर सकती हूँ।
Customer : मैं बहुत दिनों उनसे हैदराबाद ट्रेवल के बारे में सोच रहा था। क्या आप मुझे कुछ अच्छे लोकेशन के बारे में बता सकती हैं?
Agent :हाँ बिल्कुल। हैदराबाद में बहुत सारे प्लेस है। उनमें से चार महीना गोलकुंडा फोर सलार जंग म्यूजियम और बिरला प्लेनेटोरियम मशहूर है।
Customer : हाँ बढिया थैंक यू मैं अगले सैटरडे और संडे को ट्राई करूँगा।
Agent : एक सजेशन वीकेंड में ट्रैफिक ज्यादा रहने के चांसेज है।
Customer : सिरियसली एनी टिप्स चिकन शेर
Agent : आप टेक्सी यूस कर लो ड्रैब और पार्किंग का प्राब्लम नहीं होगा।
Customer : ग्रेट आइडिया थैंक्यू सो मच।The highlighted words are the ones that the default Amazon Transcribe model didn’t render correctly. - Create another text file named
reference.txt
, replacing the highlighted words with the desired words you expect to see in the transcription:
Customer : हेलो,
Agent : गुड मोर्निग सौथ इंडिया ट्रेवल एजेंसी से मैं । लावन्या बात कर रही हूँ किस तरह से मैं आपकी सहायता कर सकती हूँ।
Customer : मैं बहुत दिनोंसे हैदराबाद ट्रेवल के बारे में सोच रहा था। क्या आप मुझे कुछ अच्छे लोकेशन के बारे में बता सकती हैं?
Agent : हाँ बिल्कुल। हैदराबाद में बहुत सारे प्लेस है। उनमें से चार मिनार गोलकोंडा फोर्ट सालार जंग म्यूजियम और बिरला प्लेनेटोरियम मशहूर है।
Customer : हाँ बढिया थैंक यू मैं अगले सैटरडे और संडे को ट्राई करूँगा।
Agent : एक सजेशन वीकेंड में ट्रैफिक ज्यादा रहने के चांसेज है।
Customer : सिरियसली एनी टिप्स यू केन शेर
Agent : आप टेक्सी यूस कर लो ड्रैव और पार्किंग का प्राब्लम नहीं होगा।
Customer : ग्रेट आइडिया थैंक्यू सो मच। - Use the following command to compare the reference and hypothesis text files that you created:
You get the following output:
The wer command compares text from the files reference.txt
and hypothesis.txt
. It reports errors for each sentence and also the total number of errors (WER: 9.848% ( 13 / 132)) in the entire transcript.
From the preceding output, wer reported 13 errors out of 132 words in the transcript. These errors can be of three types:
- Substitution errors – These occur when Amazon Transcribe writes one word in place of another. For example, in our transcript, the word “महीना (Mahina)” was written instead of “मिनार (Minar)” in sentence 4.
- Deletion errors – These occur when Amazon Transcribe misses a word entirely in the transcript.In our transcript, the word “सौथ (South)” was missed in sentence 2.
- Insertion errors – These occur when Amazon Transcribe inserts a word that wasn’t spoken. We don’t see any insertion errors in our transcript.
Observations from the transcript created by the default model
We can make the following observations based on the transcript:
- The total WER is 9.848%, meaning 90.152% of the words are transcribed accurately.
- The default Hindi model transcribed most of the English words accurately. This is because the default model is trained to recognize the most common English words out of the box. The model is also trained to recognize Hinglish language, where English words randomly appear in Hindi conversations. For example:
- गुड मोर्निग – Good morning (sentence 2).
- ट्रेवल एजेंसी – Travel agency (sentence 2).
- ग्रेट आइडिया थैंक्यू सो मच – Great idea thank you so much (sentence 9).
- Sentence 4 has the most errors, which are the names of places in the Indian city Hyderabad:
- हाँ बिल्कुल। हैदराबाद में बहुत सारे प्लेस है। उनमें से चार महीना गोलकुंडा फोर सलार जंग म्यूजियम और बिरला प्लेनेटोरियम मशहूर है।
In the next step, we demonstrate how to correct the highlighted words in the preceding sentence using custom vocabulary in Amazon Transcribe:
- चार महीना (Char Mahina) should be चार मिनार (Char Minar)
- गोलकुंडा फोर (Golcunda Four) should be गोलकोंडा फोर्ट (Golconda Fort)
- सलार जंग (Salar Jung) should be सालार जंग (Saalar Jung)
Train the default model with a custom vocabulary
To create a custom vocabulary, you need to build a text file in a tabular format with the words and phrases to train the default Amazon Transcribe model. Your table must contain all four columns (Phrase
, SoundsLike
, IPA
, and DisplayAs
), but the Phrase
column is the only one that must contain an entry on each row. You can leave the other columns empty. Each column must be separated by a tab character, even if some columns are left empty. For example, if you leave the IPA
and SoundsLike
columns empty for a row, the Phrase
and DisplaysAs
columns in that row must be separated with three tab characters (between Phrase
and IPA
, IPA
and SoundsLike
, and SoundsLike
and DisplaysAs
).
To train the model with a custom vocabulary, complete the following steps:
- Create a file named
HindiCustomVocabulary.txt
with the following content.You can only use characters that are supported for your language. Refer to your language’s character set for details.
The columns contain the following information:
-
Phrase
– Contains the words or phrases that you want to transcribe accurately. The highlighted words or phrases in the transcript created by the default Amazon Transcribe model appear in this column. These words are generally acronyms, proper nouns, or domain-specific words and phrases that the default model isn’t aware of. This is a mandatory field for every row in the custom vocabulary table. In our transcript, to correct “गोलकुंडा फोर (Golcunda Four)” from sentence 4, use “गोलकुंडा-फोर (Golcunda-Four)” in this column. If your entry contains multiple words, separate each word with a hyphen (-); do not use spaces. -
IPA
– Contains the words or phrases representing speech sounds in the written form. The column is optional; you can leave its rows empty. This column is intended for phonetic spellings using only characters in the International Phonetic Alphabet (IPA). Refer to Hindi character set for the allowed IPA characters for the Hindi language. In our example, we’re not using IPA. If you have an entry in this column, yourSoundsLike
column must be empty. -
SoundsLike
– Contains words or phrases broken down into smaller pieces (typically based on syllables or common words) to provide a pronunciation for each piece based on how that piece sounds. This column is optional; you can leave the rows empty. Only add content to this column if your entry includes a non-standard word, such as a brand name, or to correct a word that is being incorrectly transcribed. In our transcript, to correct “सलार जंग (Salar Jung)” from sentence 4, use “सा-लार-जंग (Saa-lar-jung)” in this column. Do not use spaces in this column. If you have an entry in this column, yourIPA
column must be empty. -
DisplaysAs
– Contains words or phrases with the spellings you want to see in the transcription output for the words or phrases in thePhrase
field. This column is optional; you can leave the rows empty. If you don’t specify this field, Amazon Transcribe uses the contents of thePhrase
field in the output file. For example, in our transcript, to correct “गोलकुंडा फोर (Golcunda Four)” from sentence 4, use “गोलकोंडा फोर्ट (Golconda Fort)” in this column.
-
-
Upload the text file (
HindiCustomVocabulary.txt
) to an S3 bucket.Now we create a custom vocabulary in Amazon Transcribe. - On the Amazon Transcribe console, choose Custom vocabulary in the navigation pane.
- For Name, enter a name.
- For Language, choose Hindi, IN (hi-IN).
- For Vocabulary input source, select S3 location.
- For Vocabulary file location on S3, enter the S3 path of the
HindiCustomVocabulary.txt
file. - Choose Create vocabulary.
- Transcribe the
SampleAudio.wav
file with the custom vocabulary, with the following parameters:- For Job name , enter
SampleAudioCustomVocabulary
. - For Language, choose Hindi, IN (hi-IN).
- For Input file location on S3, browse to the location of
SampleAudio.wav
. - For IAM role, select Use an existing IAM role and choose the role you created earlier.
- In the Configure job section, select Custom vocabulary and choose the custom vocabulary
HindiCustomVocabulary
.
- For Job name , enter
- Choose Create job.
Measure model accuracy after using custom vocabulary
Copy the transcript from the Amazon Transcribe job details page to a text file named hypothesis-custom-vocabulary.txt
:
Customer : हेलो,
Agent : गुड मोर्निग इंडिया ट्रेवल एजेंसी सेम है। लावन्या बात कर रही हूँ किस तरह से मैं आपकी सहायता कर सकती हूँ।
Customer : मैं बहुत दिनों उनसे हैदराबाद ट्रेवल के बारे में सोच रहा था। क्या आप मुझे कुछ अच्छे लोकेशन के बारे में बता सकती हैं?
Agent : हाँ बिल्कुल। हैदराबाद में बहुत सारे प्लेस है। उनमें से चार मिनार गोलकोंडा फोर्ट सालार जंग म्यूजियम और बिरला प्लेनेटोरियम मशहूर है।
Customer : हाँ बढिया थैंक यू मैं अगले सैटरडे और संडे को ट्राई करूँगा।
Agent : एक सजेशन वीकेंड में ट्रैफिक ज्यादा रहने के चांसेज है।
Customer : सिरियसली एनी टिप्स चिकन शेर
Agent : आप टेक्सी यूस कर लो ड्रैब और पार्किंग का प्राब्लम नहीं होगा।
Customer : ग्रेट आइडिया थैंक्यू सो मच।
Note that the highlighted words are transcribed as desired.
Run the wer
command again with the new transcript:
You get the following output:
Observations from the transcript created with custom vocabulary
The total WER is 6.061%, meaning 93.939% of the words are transcribed accurately.
Let’s compare the wer output for sentence 4 with and without custom vocabulary. The following is without custom vocabulary:
The following is with custom vocabulary:
There are no errors in sentence 4. The names of the places are transcribed accurately with the help of custom vocabulary, thereby reducing the overall WER from 9.848% to 6.061% for this audio file. This means that the accuracy of transcription improved by nearly 4%.
How custom vocabulary improved the accuracy
We used the following custom vocabulary:
Amazon Transcribe checks if there are any words in the audio file that sound like the words mentioned in the Phrase
column. Then the model uses the entries in the IPA
, SoundsLike
, and DisplaysAs
columns for those specific words to transcribe with the desired spellings.
With this custom vocabulary, when Amazon Transcribe identifies a word that sounds like “गोलकुंडा-फोर (Golcunda-Four),” it transcribes that word as “गोलकोंडा फोर्ट (Golconda Fort).”
Recommendations
The accuracy of transcription also depends on parameters like the speakers’ pronunciation, overlapping speakers, talking speed, and background noise. Therefore, we recommend that you to follow the process with a variety of calls (with different customers, agents, interruptions, and so on) that cover the most commonly used domain-specific words for you to build a comprehensive custom vocabulary.
In this post, we learned the process to improve accuracy of transcribing one audio call using custom vocabulary. To process thousands of your contact center call recordings every day, you can use post call analytics, a fully automated, scalable, and cost-efficient end-to-end solution that takes care of most of the heavy lifting. You simply upload your audio files to an S3 bucket, and within minutes, the solution provides call analytics like sentiment in a web UI. Post call analytics provides actionable insights to spot emerging trends, identify agent coaching opportunities, and assess the general sentiment of calls.Post call analytics is an open-source solution that you can deploy using AWS CloudFormation.
Note that custom vocabularies don’t use the context in which the words were spoken, they only focus on individual words that you provide. To further improve the accuracy, you can use custom language models. Unlike custom vocabularies, which associate pronunciation with spelling, custom language models learn the context associated with a given word. This includes how and when a word is used, and the relationship a word has with other words. To create a custom language model, you can use the transcriptions derived from the process we learned for a variety of calls, and combine them with content from your websites or user manuals that contains domain-specific words and phrases.
To achieve the highest transcription accuracy with batch transcriptions, you can use custom vocabularies in conjunction with your custom language models.
Conclusion
In this post, we provided detailed steps to accurately process Hindi audio files containing English words using call analytics and custom vocabularies in Amazon Transcribe. You can use these same steps to process audio calls with any language supported by Amazon Transcribe.
After you derive the transcriptions with your desired accuracy, you can improve your agent-customer conversations by training your agents. You can also understand your customer sentiments and trends. With the help of speaker diarization, loudness detection, and vocabulary filtering features in the call analytics, you can identify whether it was the agent or customer who raised their tone or spoke any specific words. You can categorize calls based on domain-specific words, capture actionable insights, and run analytics to improve your products. Finally, you can translate your transcripts to English or other supported languages of your choice using Amazon Translate.
About the Authors
Sarat Guttikonda is a Sr. Solutions Architect in AWS World Wide Public Sector. Sarat enjoys helping customers automate, manage, and govern their cloud resources without sacrificing business agility. In his free time, he loves building Legos with his son and playing table tennis.
Lavanya Sood is a Solutions Architect in AWS World Wide Public Sector based out of New Delhi, India. Lavanya enjoys learning new technologies and helping customers in their cloud adoption journey. In her free time, she loves traveling and trying different foods.
Announcing TensorFlow Lite in Google Play Services General Availability
Posted by Bernhard Bauer and Terry Heo, Software Engineers, Google
Today we’re excited to announce that the Google Play services API for TensorFlow Lite is generally available on Android devices. We recommend this distribution as the path to adding custom machine learning to your apps. Last year, we launched a public beta of TensorFlow Lite in Google Play services at Google I/O. Since then, we’ve received lots of feedback and made improvements to the API. Most recently, we added the GPU delegate and Task Library support. Today we’re moving from beta to general availability on billions of Android devices globally.
TensorFlow Lite in Google Play services is already used by Google teams, including ML Kit, serving over a billion monthly active users and running more than 100 billion daily inferences.
TensorFlow Lite is an inference runtime optimized for mobile devices, and now that it’s part of Google Play services, it helps you deliver better ML experiences because it:
- Reduces your app size by up to 5 MB compared to statically bundling TensorFlow Lite with your app
- Uses the same API as available when bundling TF Lite into your app
- Receives regular performance updates in the background so it’s always getting better automatically
Get started by learning how to add TensorFlow Lite in Google Play Services to your Android app.Read More