Fellowships will provide support to pursue research in the fields of artificial intelligence and robotics.Read More
Hello, World: NIO Expands Global Footprint With Intelligent Vehicle Experiences
When it comes to reimagining the next generation of automotive, NIO is thinking outside the car.
This month, the China-based electric vehicle maker introduced its lineup to four new countries in Europe — Denmark, Germany, the Netherlands and Sweden — along with an innovative subscription-based ownership model. The countries join NIO’s customer base in China and Norway.
The models launching in the European market are all built on the NIO Adam supercomputer, which uses four NVIDIA DRIVE Orin systems-on-a-chip to deliver software-defined AI features.
These intelligent capabilities, which will gradually enable automated driving on expressways and urban areas, as well as autonomous parking and battery swap, are just the start of NIO’s fresh take on the vehicle ownership experience.
As the automaker expands its footprint, it is emphasizing membership rather than pure ownership. NIO vehicles are available via flexible subscription models, and customers can access club spaces, called NIO Houses, that offer a wide array of amenities.
International Supermodels
NIO’s lineup sports a premium model for every type of driver.
The flagship ET7 sedan boasts a spacious interior, with more than 620 miles of battery range and an impressive 0-to-60 miles per hour in under four seconds. For the mid-size segment, the ET5 is an EV that’s as agile as it is comfortable, borrowing the same speed and immersive interior as its predecessor in a more compact package.
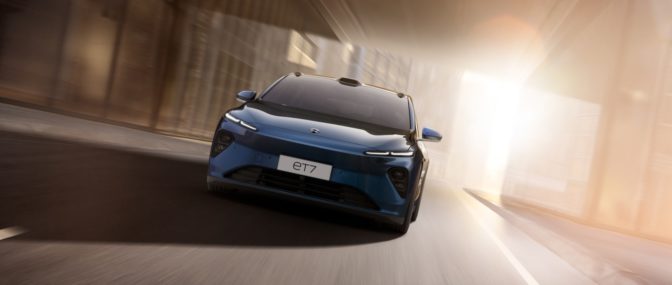
Finally, the ES7 — renamed the EL7 for the European market — is an electric SUV for rugged and urban drivers alike. The intelligent EV sports 10 driving modes, including a camping mode for off-road adventures.
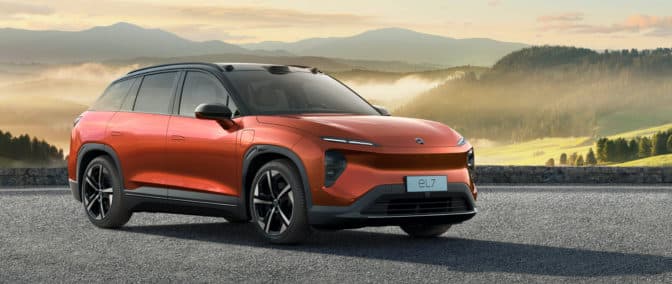
All three models run on the high-performance, centralized Adam supercomputer. With more than 1,000 trillion operations per second of performance provided by four DRIVE Orin SoCs, Adam can power a wide range of intelligent features, with enough headroom to add new capabilities over the air.
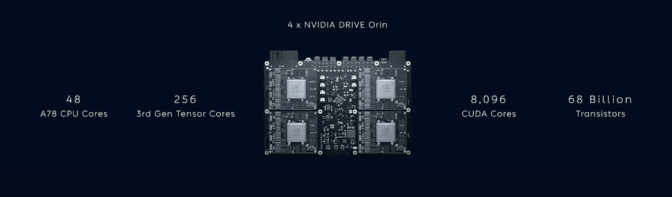
Using multiple SoCs, Adam integrates the redundancy and diversity necessary for safe autonomous operation. The first two SoCs process the 8GB of data produced every second by the vehicle’s sensor set.
The third Orin serves as a backup to ensure the system can operate safely in any situation. And the fourth enables local training, improving the vehicle with fleet learning and personalizing the driving experience based on individual user preferences.
While NIO’s models vary in size and design, they all share the same intelligent DNA, so every customer has access to the cutting edge in AI transportation.
A NIO Way Forward
The NIO experience doesn’t end when the drive is over — it aims to create an entire lifestyle.
Customers in new markets won’t be buying the vehicles, they’ll sign for leases as long as 60 months or as short as one month. These subscriptions include insurance, maintenance, winter tires, a courtesy car, battery swapping and the option to upgrade battery services.
The purpose of the business model is to offer the utmost flexibility, so customers always have access to the best vehicle for their needs, whatever they may be and however often they may change.
Additionally, every customer has access to the NIO House. This community space offers co-working areas, cafes, workout facilities, playrooms for children and more. NIO Houses exist in more than 80 places around the world, with locations planned for Amsterdam, Berlin, Copenhagen, Düsseldorf, Frankfurt, Gothenburg, Hamburg, Rotterdam and Stockholm.
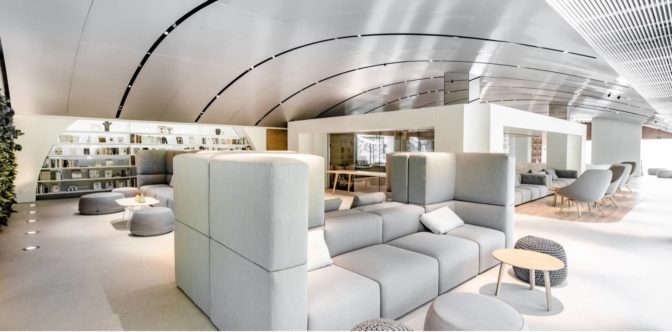
Deliveries to the expanded European markets are scheduled to start with the ET7 sedan on Sunday, Oct. 16, with the EL7 and ET5 set to ship in January and March of 2023, respectively.
The post Hello, World: NIO Expands Global Footprint With Intelligent Vehicle Experiences appeared first on NVIDIA Blog.
SPIN: An Empirical Evaluation on Sharing Parameters of Isotropic Networks
Recent isotropic networks, such as ConvMixer and vision transformers, have found significant success across visual recognition tasks, matching or outperforming non-isotropic convolutional neural networks (CNNs). Isotropic architectures are particularly well-suited to cross-layer weight sharing, an effective neural network compression technique. In this paper, we perform an empirical evaluation on methods for sharing parameters in isotropic networks (SPIN). We present a framework to formalize major weight sharing design decisions and perform a comprehensive empirical evaluation of this design…Apple Machine Learning Research
Crossmodal-3600 — Multilingual Reference Captions for Geographically Diverse Images
Image captioning is the machine learning task of automatically generating a fluent natural language description for a given image. This task is important for improving accessibility for visually impaired users and is a core task in multimodal research encompassing both vision and language modeling.
However, datasets for image captioning are primarily available in English. Beyond that, there are only a few datasets covering a limited number of languages that represent just a small fraction of the world’s population. Further, these datasets feature images that severely under-represent the richness and diversity of cultures from across the globe. These aspects have hindered research on image captioning for a wide variety of languages, and directly hamper the deployment of accessibility solutions for a large potential audience around the world.
Today we present and make publicly available the Crossmodal 3600 (XM3600) image captioning evaluation dataset as a robust benchmark for multilingual image captioning that enables researchers to reliably compare research contributions in this emerging field. XM3600 provides 261,375 human-generated reference captions in 36 languages for a geographically diverse set of 3600 images. We show that the captions are of high quality and the style is consistent across languages.
The Crossmodal 3600 dataset includes reference captions in 36 languages for each of a geographically diverse set of 3600 images. All images used with permission under the CC-BY 2.0 license. |
Overview of the Crossmodal 3600 Dataset
Creating large training and evaluation datasets in multiple languages is a resource-intensive endeavor. Recent work has shown that it is feasible to build multilingual image captioning models trained on machine-translated data with English captions as the starting point. However, some of the most reliable automatic metrics for image captioning are much less effective when applied to evaluation sets with translated image captions, resulting in poorer agreement with human evaluations compared to the English case. As such, trustworthy model evaluation at present can only be based on extensive human evaluation. Unfortunately, such evaluations usually cannot be replicated across different research efforts, and therefore do not offer a fast and reliable mechanism to automatically evaluate multiple model parameters and configurations (e.g., model hill climbing) or to compare multiple lines of research.
XM3600 provides 261,375 human-generated reference captions in 36 languages for a geographically diverse set of 3600 images from the Open Images dataset. We measure the quality of generated captions by comparing them to the manually provided captions using the CIDEr metric, which ranges from 0 (unrelated to the reference captions) to 10 (perfectly matching the reference captions). When comparing pairs of models, we observed strong correlations between the differences in the CIDEr scores of the model outputs, and side-by-side human evaluations comparing the model outputs. , making XM3600 is a reliable tool for high-quality automatic comparisons between image captioning models on a wide variety of languages beyond English.
Language Selection
We chose 30 languages beyond English, roughly based on their percentage of web content. In addition, we chose an additional five languages that include under-resourced languages that have many native speakers or major native languages from continents that would not be covered otherwise. Finally, we also included English as a baseline, thus resulting in a total of 36 languages, as listed in the table below.
Arabic | Bengali* | Chinese | Croatian | Cusco Quechua* |
Czech | |||||
Danish | Dutch | English | Filipino | Finnish | French | |||||
German | Greek | Hebrew | Hindi | Hungarian | Indonesian | |||||
Italian | Japanese | Korean | Maori* | Norwegian | Persian | |||||
Polish | Portuguese | Romanian | Russian | Spanish | Swahili* | |||||
Swedish | Telugu* | Thai | Turkish | Ukrainian | Vietnamese |
List of languages used in XM3600. *Low-resource languages with many native speakers, or major native languages from continents that would not be covered otherwise. |
Image Selection
The images were selected from among those in the Open Images dataset that have location metadata. Since there are many regions where more than one language is spoken, and some areas are not well covered by these images, we designed an algorithm to maximize the correspondence between selected images and the regions where the targeted languages are spoken. The algorithm starts with the selection of images with geo-data corresponding to the languages for which we have the smallest pool (e.g., Persian) and processes them in increasing order of their candidate image pool size. If there aren’t enough images in an area where a language is spoken, then we gradually expand the geographic selection radius to: (i) a country where the language is spoken; (ii) a continent where the language is spoken; and, as last resort, (iii) from anywhere in the world. This strategy succeeded in providing our target number of 100 images from an appropriate region for most of the 36 languages, except for Persian (where 14 continent-level images are used) and Hindi (where all 100 images are at the global level, because the in-region images were assigned to Bengali and Telugu).
English![]() Photo by Chris Sampson |
Swahili![]() Photo by Henrik Palm |
Telugu![]() Photo by rojypala |
||
Cusco Quechua![]() Photo by McKay Savage |
Filipino![]() Photo by Simon Schoeters |
Chinese![]() Photo by Stefan Krasowski |
Sample images showcasing the geographical diversity of the annotated images. Images used under CC BY 2.0 license. |
Caption Generation
In total, all 3600 images (100 images per language) are annotated in all 36 languages, each with an average of two annotations per language, yielding a total of 261,375 captions.
Annotators work in batches of 15 images. The first screen shows all 15 images with their captions in English as generated by a captioning model trained to output a consistent style of the form “<main salient objects> doing <activities> in the <environment>”, often with object attributes, such as a “smiling” person, “red” car, etc. The annotators are asked to rate the caption quality given guidelines for a 4-point scale from “excellent” to “bad”, plus an option for “not_enough_information”. This step forces the annotators to carefully assess caption quality and it primes them to internalize the style of the captions. The following screens show the images again but individually and without the English captions, and the annotators are asked to produce descriptive captions in the target language for each image.
The image batch size of 15 was chosen so that the annotators would internalize the style without remembering the exact captions. Thus, we expect the raters to generate captions based on the image content only and lacking translation artifacts. For example in the example shown below, the Spanish caption mentions “number 42” and the Thai caption mentions “convertibles”, none of which are mentioned in the English captions. The annotators were also provided with a protocol to use when creating the captions, thus achieving style consistency across languages.
![]() Photo by Brian Solis |
English | • A vintage sports car in a showroom with many other vintage sports cars | ||
• The branded classic cars in a row at display | ||||
Spanish | • Automóvil clásico deportivo en exhibición de automóviles de galería — (Classic sports car in gallery car show) | |||
• Coche pequeño de carreras color plateado con el número 42 en una exhibición de coches — (Small silver racing car with the number 42 at a car show) | ||||
Thai | • รถเปิดประทุนหลายสีจอดเรียงกันในที่จัดแสดง — (Multicolored convertibles line up in the exhibit) | |||
• รถแข่งวินเทจจอดเรียงกันหลายคันในงานจัดแสดง — (Several vintage racing cars line up at the show.) |
Sample captions in three different languages (out of 36 — see full list of captions in Appendix A of the Crossmodal-3600 paper), showcasing the creation of annotations that are consistent in style across languages, while being free of direct-translation artifacts (e.g., the Spanish “number 42” or the Thai “convertibles” would not be possible when directly translating from the English versions). Image used under CC BY 2.0 license. |
Caption Quality and Statistics
We ran two to five pilot studies per language to troubleshoot the caption generation process and to ensure high quality captions. We then manually evaluated a random subset of captions. First we randomly selected a sample of 600 images. Then, to measure the quality of captions in a particular language, for each image, we selected for evaluation one of the manually generated captions. We found that:
- For 25 out of 36 languages, the percentage of captions rated as “Good” or “Excellent” is above 90%, and the rest are all above 70%.
- For 26 out of 36 languages, the percentage of captions rated as “Bad” is below 2%, and the rest are all below 5%.
For languages that use spaces to separate words, the number of words per caption can be as low as 5 or 6 for some agglutinative languages like Cusco Quechua and Czech, and as high as 18 for an analytic language like Vietnamese. The number of characters per caption also varies drastically — from mid-20s for Korean to mid-90s for Indonesian — depending on the alphabet and the script of the language.
Empirical Evaluation and Results
We empirically measured the ability of the XM3600 annotations to rank image captioning model variations by training four variations of a multilingual image captioning model and comparing the CIDEr differences of the models’ outputs over the XM3600 dataset for 30+ languages, to side-by-side human evaluations. We observed strong correlations between the CIDEr differences and the human evaluations. These results support the use of the XM3600 references as a means to achieve high-quality automatic comparisons between image captioning models on a wide variety of languages beyond English.
Recent Uses
Recently PaLI used XM3600 to evaluate model performance beyond English for image captioning, image-to-text retrieval and text-to-image retrieval. The key takeaways they found when evaluating on XM3600 were that multilingual captioning greatly benefits from scaling the PaLI models, especially for low-resource languages.
Acknowledgements
We would like to acknowledge the coauthors of this work: Xi Chen and Radu Soricut.
Learn How NVIDIA Advances AI for Enterprises, at Oracle CloudWorld
NVIDIA and Oracle are teaming to make the power of AI accessible to enterprises across industries. These include healthcare, financial services, automotive and a broad range of natural language processing use cases driven by large language models, such as chatbots, personal assistants, document summarization and article completion.
Join NVIDIA and Oracle experts at Oracle CloudWorld, running Oct. 17-20 in Las Vegas, to learn more about technology breakthroughs and steps companies can take to unlock the potential of enterprise data with AI.
Attend the fireside chat featuring NVIDIA founder and CEO Jensen Huang and Oracle CEO Safra Catz taking place on Tuesday, Oct. 18, at 9 a.m. PT to learn how NVIDIA AI is being enabled for enterprises globally on Oracle.
NVIDIA and Oracle bring together all the key ingredients for speeding AI adoption for enterprises: the ability to securely access and manage data within Oracle’s Enterprise Data Management platforms; on-demand access to the massive computational power of NVIDIA-accelerated infrastructure at scale to build and train AI models using this data; and an NVIDIA AI developer performance-optimized stack that simplifies and accelerates building and deploying AI-enabled enterprise products and services at scale.
The NVIDIA AI platform, combined with Oracle Cloud Infrastructure, paves the way to an AI-powered enterprise, regardless of where a business is in its AI adoption journey. The platform offers GPU-accelerated deep learning frameworks, pretrained AI models, enterprise-grade software development kits and application-specific frameworks for various use cases.
Register for Oracle CloudWorld and dive into these sessions and demos to learn more:
- Keynote Fireside Address: Driving Impactful Business Results — featuring Huang and Catz, in conversation with leaders of global brands, discovering how they solve complex problems by working with Oracle. This session takes place on Tuesday, Oct. 18, from 9-10:15 a.m. PT.
- Oracle Making AI Approachable for Everyone — featuring Ian Buck, vice president of hyperscale and high-performance computing at NVIDIA, and Elad Ziklik, vice president of AI and data science services at Oracle. This session takes place on Tuesday, Oct. 18, from 11-11:45 a.m. PT.
- Achieving Database Operational Excellence Using Events and Functions at NVIDIA — featuring Chris May, senior manager at NVIDIA, and Riaz Kapadia, enterprise cloud architect at Oracle. This session takes place on Tuesday, Oct. 18, from 12:15 -1 p.m. PT.
- MLOps at Scale With Kubeflow on Oracle Cloud Infrastructure — featuring Richard Wang, senior cloud and machine learning solutions architect at NVIDIA, and Sesh Dehalisan, distinguished cloud architect at Oracle. This session takes place on Tuesday, Oct. 18, from 12:15-1 p.m. PT.
- Next-Generation AI Empowering Human Expertise — featuring Bryan Catanzaro, vice president of applied deep learning research at NVIDIA; Erich Elsen, co-founder and head of machine learning at Adept AI; and Rich Clayton, vice president of product strategy for analytics at Oracle. This session takes place on Tuesday, Oct. 18, from 12:30-1 p.m. PT.
- NVIDIA’s Migration From On-Premises to MySQL HeatWave — featuring Chris May, senior manager at NVIDIA; Radha Chinnaswamy, consultant at NVIDIA; and Sastry Vedantam, MySQL master principal solution engineer at Oracle. This session takes place on Tuesday, Oct. 18, from 4-4:45 p.m. PT.
- Scale Large Language Models With NeMo Megatron — featuring Richard Wang, senior cloud and machine learning solutions architect at NVIDIA; Anup Ojah, senior manager of cloud engineering at Oracle; and Tanina Cadwell, solutions architect at Vyasa Analytics. This session takes place on Wednesday, Oct. 19, from 11:30 a.m. to 12:15 p.m. PT.
- Serve ML Models at Scale With Triton Inference Server on OCI — featuring Richard Wang, senior cloud and machine learning solutions architect at NVIDIA, and Joanne Lei, master principal cloud architect at Oracle. This session takes place on Wednesday, Oct. 19, from 1:15-2 p.m. PT.
- Accelerating Java on the GPU — featuring Ken Hester, solutions architect director at NVIDIA, and Paul Sandoz, Java architect at Oracle. This session takes place on Thursday, Oct. 20, from 10:15-10:45 a.m. PT.
- NVIDIA AI Software for Business Outcomes: Integrating NVIDIA AI Into Your Applications — featuring Kari Briski, vice president of software product management for AI and high-performance computing software development kits at NVIDIA. This session takes place on demand.
Visit NVIDIA’s Oracle CloudWorld showcase page to discover more about NVIDIA and Oracle’s collaboration and innovations for cloud-based solutions.
The post Learn How NVIDIA Advances AI for Enterprises, at Oracle CloudWorld appeared first on NVIDIA Blog.
Press Art to Continue: New AI Tools Promise Art With the Push of a Button — But Reality Is More Complicated
Alien invasions. Gritty dystopian megacities. Battlefields swarming with superheroes. As one of Hollywood’s top concept artists, Drew Leung can visualize any world you can think of, except one where AI takes his job.
He would know. He’s spent the past few months trying to make it happen, testing every AI tool he could. “If your whole goal is to use AI to replace artists, you’ll find it really disappointing,” Leung said.
Pros and amateurs alike, however, are finding these new tools intriguing. For amateur artists — who may barely know which way to hold a paintbrush — AI gives them almost miraculous capabilities.
Thanks to AI tools such as Midjourney, OpenAI’s Dall·E, DreamStudio, and open-source software such as Stable Diffusion, AI-generated art is everywhere, spilling out across the globe through social media such as Facebook and Twitter, the tight-knit communities on Reddit and Discord, and image-sharing services like Pinterest and Instagram.
The trend has sparked an uproarious discussion in the art community. Some are relying on AI to accelerate their creative process — doing in minutes what used to take a day or more, such as instantly generating mood boards with countless iterations on a theme.
Others, citing issues with how the data used to train these systems is collected and managed, are wary. “I’m frustrated because this could be really exciting if done right,” said illustrator and concept artist Karla Ortiz, who currently refuses to use AI for art altogether.
NVIDIA’s creative team provided a taste of what these tools can do in the hands of a skilled artist during NVIDIA founder and CEO Jensen Huang’s keynote at the most recent NVIDIA GTC technology conference.
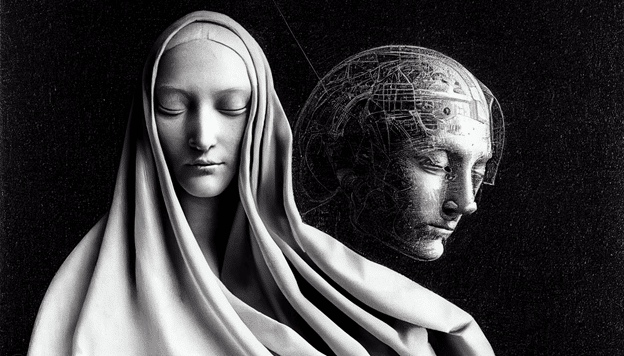
Highlights included a woman representing AI created in the drawing style of Leonardo da Vinci and an image of 19th-century English mathematician Ada Lovelace, considered by many the first computer programmer, holding a modern game controller.
More Mechanical Than Magical
After months of experimentation, Leung — known for his work on more than a score of epic movies including Black Panther and Captain America: Civil War, among other blockbusters — compares AI art tools to a “kaleidoscope” that combines colors and shapes in unexpected ways with a twist of your wrist.
Used that way, some artists say AI is most interesting when an artist pushes it hard enough to break. AI can instantly reveal visual clichés — because it fails when asked to do things it hasn’t seen before, Leung said.
And because AI tools are fed by vast quantities of data, AI can expose biases across collections of millions of images — such as poor representation of people of color — because it struggles to produce images outside a narrow ideal.
New Technologies, OId Conversations
Such promises and pitfalls put AI at the center of conversations about the intersections of technology and technique, automation and innovation, that have been going on long before AI, or even computers, existed.
After Louis-Jacques-Mandé Daguerre invented photography in 1839, painter Charles Baudelaire declared photography “art’s most mortal enemy.”
With the motto, “You push the button, we do the rest,” George Eastman’s affordable handheld cameras made photography accessible to anyone in 1888. It took years for 19th-century promoter and photographer Alfred Stieglitz, who played a key role transforming photography into an accepted art form, to come around.
Remaking More Than Art
Over the next century new technologies, like color photography, offset printmaking and digital art, inspired new movements from expressionism to surrealism, pop art to post-modernism.
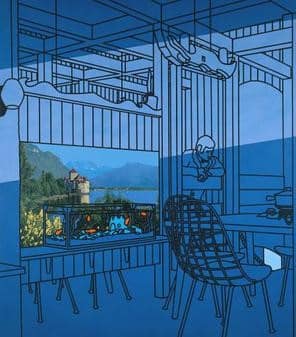
The emergence of AI art continues the cycle. And the technology driving it, called transformers, like the technologies that led to past art movements, is driving changes far outside the art world.
First introduced in 2017, transformers are a type of neural network that learns context and, thus, meaning, from data. They’re now among the most vibrant areas for research in AI.
A single pretrained model can perform amazing feats — including text generation, translation and even software programming — and is the basis of the new generation of AI that can turn text into detailed images.
The diffusion models powering AI image tools, such as Dall·E and Dall·E 2, are transformer-based generative models that refine and rearrange pixels again and again until the image matches a user’s text description.
More’s coming. NVIDIA GPUs — the parallel processing engines that make modern AI possible — are being fine-tuned to support ever more powerful applications of the technology.
Introduced earlier this year, the Hopper FP8 Transformer Engine in NVIDIA’s latest GPUs will soon be embedded across vast server farms, in autonomous vehicles and in powerful desktop GPUs.
Intense Conversations
All these possibilities have sparked intense conversations.
Artist Jason Allen ignited a worldwide controversy by winning a contest at the Colorado State Fair with an AI-generated painting.
Attorney Steven Frank has renewed old conversations in art history by using AI to reassess the authenticity of some of the world’s most mysterious artworks, such as “Salvator Mundi,” left, a painting now attributed to da Vinci.
Philosophers, ethicists and computer scientists such as Ahmed Elgammal at Rutgers University are debating if it’s possible to separate techniques that AI can mimic with the intentions of the human artists who created them.
Ortiz is among a number raising thorny questions about how the data used to train AI is collected and managed. And once an AI is trained on an image, it can’t unlearn what it’s been trained to do, Ortiz says.
Some, such as New York Times writer Kevin Roose, wonder if AI will eventually start taking away jobs from artists.
Others, such as Jason Scott, an artist and archivist at the Internet Archive, dismiss AI art as “no more dangerous than a fill tool.”
Such whirling conversations — about how new techniques and technologies change how art is made, why art is made, what it depicts, and how art, in turn, remakes us — have always been an element of art. Maybe even the most important element.
“Art is a conversation we are all invited to,” American author Rachel Hartman once wrote.
Ortiz says this means we should be thoughtful. “Are these tools assisting the artist, or are they there to be the artist?” she asked.
It’s a question all of us should ponder. Controversially, anthropologist Eric Gans connects the first act of imbuing physical objects with a special significance or meaning — the first art — to the origin of language itself.
In this context, AI will, inevitably, reshape some of humanity’s oldest conversations. Maybe even our very oldest conversation. The stakes could not be higher.
Featured image: Portrait of futuristic Ada Lovelace, playing video games, editorial photography style by NVIDIA’s creative team, using Midjourney.
The post Press Art to Continue: New AI Tools Promise Art With the Push of a Button — But Reality Is More Complicated appeared first on NVIDIA Blog.
Stopping malaria in its tracks
Developing a vaccine that could save hundreds of thousands of livesRead More
GeForce NOW Streams High-Res, 120-FPS PC Gaming to World’s First Cloud Gaming Chromebooks
High-end PC gaming arrives on more devices this GFN Thursday.
GeForce NOW RTX 3080 members can now stream their favorite PC games at up to 1600p and 120 frames per second in a Chrome browser. No downloads, no installs, just victory.
Even better, NVIDIA has worked with Google to support the newest Chromebooks, which are the first laptops custom built for cloud gaming, with gorgeous 1600p resolution 120Hz+ displays. They come with a free three-month GeForce NOW RTX 3080 membership, the highest performance tier.
On top of these new ways to play, this GFN Thursday brings hordes of fun with 11 new titles streaming from the cloud — including the Warhammer 40,000: Darktide closed beta, available Oct. 14-16.
High-Performance PC Gaming, Now on Chromebooks
Google’s newest Chromebooks are the first built for cloud gaming, and include GeForce NOW right out of the box.
These new cloud gaming Chromebooks — the Acer Chromebook 516 GE, the ASUS Chromebook Vibe CX55 Flip and the Lenovo Ideapad Gaming Chromebook — all include high refresh rate, high-resolution displays, gaming keyboards, fast WiFi 6 connectivity and immersive audio. And with the GeForce NOW RTX 3080 membership, gamers can instantly stream 1,400+ PC games from the GeForce NOW library at up to 1600p at 120 FPS.
That means Chromebook gamers can jump right into over 100 free-to-play titles, including major franchises like Fortnite, Genshin Impact and League of Legends. RTX 3080 members can explore the worlds of Cyberpunk 2077, Control and more with RTX ON, only through GeForce NOW. Compete online with ultra-low latency and other features perfect for playing.
The GeForce NOW app comes preinstalled on these cloud gaming Chromebooks, so users can jump straight into the gaming — just tap, search, launch and play. Plus, pin games from GeForce NOW right to the app shelf to get back into them with just a click.
For new and existing members, every cloud gaming Chromebook includes a free three-month RTX 3080 membership through the Chromebook Perks program.
Stop! Warhammer Time
Fatshark leaps thousands of years into the future to bring gamers Warhammer 40,000: Darktide on Tuesday, Nov. 30.
Gamers who’ve preordered on Steam can get an early taste of the game with a closed beta period, running Oct. 14-16.
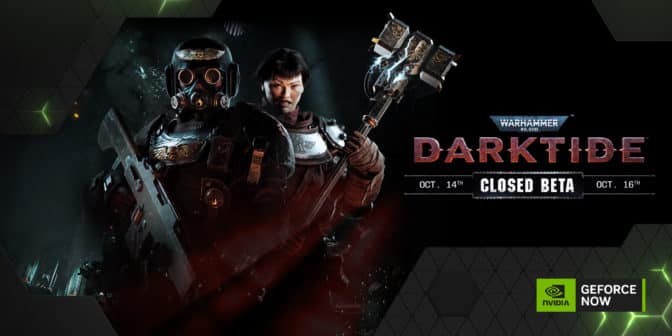
Head to the industrial city of Tertium to combat the forces of Chaos, using Vermintide 2’s lauded melee system and a range of deadly Warhammer 40,000 weapons. Personalize your play style with a character-creation system and delve deep into the city to put a stop to the horrors that lurk.
The fun doesn’t stop there. Members can look for these new titles streaming this week:
- Asterigos: Curse of the Stars (New release on Steam)
- Kamiwaza: Way of the Thief (New release on Steam)
- LEGO Bricktales (New release on Steam and Epic Games)
- Ozymandias: Bronze Age Empire Sim (New release on Steam)
- PC Building Simulator 2 (New release on Epic Games)
- The Last Oricru (New release on Steam, Oct. 13)
- Rabbids: Party of Legends (New release on Ubisoft, Oct. 13)
- The Darkest Tales (New release on Steam,Oct. 13)
- Scorn (New Release on Steam and Epic Games, Oct. 14)
- Warhammer 40,000: Darktide Closed Beta (New release on Steam, available from Oct. 14th, 7 AM PT to Oct. 17th 1 AM PT)
- Dual Universe (Steam)
Finally, we have a question for you – we promise not to tattle. Let us know your answer on Twitter or in the comments below.
Be honest, how many times have you played games on GFN with chrome browser in class or a meeting?
—
NVIDIA GeForce NOW (@NVIDIAGFN) October 12, 2022
The post GeForce NOW Streams High-Res, 120-FPS PC Gaming to World’s First Cloud Gaming Chromebooks appeared first on NVIDIA Blog.
Scaling PyTorch models on Cloud TPUs with FSDP
Introduction
The research community has witnessed a lot of successes with large models across NLP, computer vision, and other domains in recent years. Many of these successes were enabled by Cloud TPUs – which are powerful hardware for distributed training. To support TPUs in PyTorch, the PyTorch/XLA library provides a backend for XLA devices (most notably TPUs) and lays the groundwork for scaling large PyTorch models on TPUs.
However, most existing modeling scaling tools in the PyTorch ecosystem assume GPU (or CPU) devices, often depend on specific features in CUDA, and do not work directly on TPUs. The lack of scaling tools makes it challenging to build large models that cannot fit into the memory of a single TPU chip.
To support model scaling on TPUs, we implemented the widely-adopted Fully Sharded Data Parallel (FSDP) algorithm for XLA devices as part of the PyTorch/XLA 1.12 release. We provide an FSDP interface with a similar high-level design to the CUDA-based PyTorch FSDP class while also handling several restrictions in XLA (see Design Notes below for more details). This FSDP interface allowed us to easily build models with e.g. 10B+ parameters on TPUs and has enabled many research explorations.
Using Fully Sharded Data Parallel (FSDP) in PyTorch/XLA
We provide a wrapper class XlaFullyShardedDataParallel
over a given PyTorch model to shard its parameters across data-parallel workers. An example usage is as follows:
import torch
import torch_xla.core.xla_model as xm
from torch_xla.distributed.fsdp import XlaFullyShardedDataParallel as FSDP
model = FSDP(my_module)
optim = torch.optim.Adam(model.parameters(), lr=0.0001)
output = model(x, y)
loss = output.sum()
loss.backward()
optim.step()
Wrapping an nn.Module
instance with XlaFullyShardedDataParallel
enables the ZeRO-2 algorithm on it, where its gradients and the optimizer states are sharded for the entire training process. During its forward and backward passes, the full parameters of the wrapped module are first reconstructed from their corresponding shards for computation.
Nested FSDP wrapping can be used to further save memory. This allows the model to store only the full parameters of one individual layer at any given time. For nested FSDP, one should first wrap its individual submodules with an inner FSDP before wrapping the base model with an outer FSDP. This allows the model to store only the full parameters of one individual layer at any given time. And having an outer wrapper ensures to handle any leftover parameters, corresponding to the ZeRO-3 algorithm. Nested FSDP wrapping can be applied at any depth of submodules and there can be more than 2 layers of nesting.
Model checkpoint saving and loading for models and optimizers can be done like before by saving and loading their .state_dict()
. Meanwhile, each training process should save its own checkpoint file of the sharded model parameters and optimizer states, and load the checkpoint file for the corresponding rank when resuming (regardless of ZeRO-2 or ZeRO-3, i.e. nested wrapping or not). A command line tool and a Python interface are provided to consolidate the sharded model checkpoint files together into a full/unshareded model checkpoint file.
Gradient checkpointing (also referred to as “activation checkpointing” or “rematerialization”) is another common technique for model scaling and can be used in conjunction with FSDP. We provide checkpoint_module
, a wrapper function over a given nn.Module
instance for gradient checkpointing (based on torch_xla.utils.checkpoint.checkpoint
).
The MNIST and ImageNet examples below provide illustrative usages of (plain or nested) FSDP, saving and consolidation of model checkpoints, as well as gradient checkpointing.
Starting examples of FSDP in PyTorch/XLA
Training MNIST and ImageNet with FSDP
MNIST and ImageNet classification can often be used as starting points to build more complicated deep learning models. We provide the following FSDP examples on these two datasets:
- MNIST: test/test_train_mp_mnist_fsdp_with_ckpt.py (it also illustrates checkpoint saving and consolidation)
- ImageNet: test/test_train_mp_imagenet_fsdp.py
A comparison of them with the vanilla data-parallel examples of MNIST and ImageNet illustrates how to adapt a training script to use FSDP. A major distinction to keep in mind is that when stepping the optimizer on an FSDP-wrapped model, one should directly call optimizer.step()
instead of xm.optimizer_step(optimizer)
. The latter reduces the gradients across ranks, which is not what we need in FSDP, where the gradients are already reduced and sharded (from a reduce-scatter op in its backward pass).
Installation
FSDP is available from the PyTorch/XLA 1.12 and newer nightly releases. Please refer to https://github.com/pytorch/xla#-available-images-and-wheels for a guide on installation as well as Cloud TPU allocation. Then clone PyTorch/XLA repo on a TPU VM as follows
mkdir -p ~/pytorch && cd ~/pytorch
git clone --recursive https://github.com/pytorch/xla.git
cd ~/
Train MNIST on v3-8 TPU
It gets around 98.9 accuracy for 2 epochs:
python3 ~/pytorch/xla/test/test_train_mp_mnist_fsdp_with_ckpt.py
--batch_size 16 --drop_last --num_epochs 2
--use_nested_fsdp
The script above automatically tests consolidation of the sharded model checkpoints at the end. You can also manually consolidate the sharded checkpoint files via
python3 -m torch_xla.distributed.fsdp.consolidate_sharded_ckpts
--ckpt_prefix /tmp/mnist-fsdp/final_ckpt
--ckpt_suffix "_rank-*-of-*.pth"
Train ImageNet with ResNet-50 on v3-8 TPU
It gets around 75.9 accuracy for 100 epochs, same as what one would get without using FSDP; download and preprocess the ImageNet-1k dataset to /datasets/imagenet-1k
:
python3 ~/pytorch/xla/test/test_train_mp_imagenet_fsdp.py
--datadir /datasets/imagenet-1k --drop_last
--model resnet50 --test_set_batch_size 64 --eval_interval 10
--lr 0.4 --batch_size 128 --num_warmup_epochs 5
--lr_scheduler_divide_every_n_epochs 30 --lr_scheduler_divisor 10
--num_epochs 100
--use_nested_fsdp
You can also explore other options in these two examples, such as --use_gradient_checkpointing
to apply gradient checkpointing (i.e. activation checkpointing) on the ResNet blocks, or --compute_dtype bfloat16
to perform forward and backward passes in bfloat16 precision.
Examples on large-scale models
When building large models on TPUs, we often need to be aware of the memory constraints (e.g. 16 GB per core in TPU v3 and 32 GB per chip in TPU v4). For large models that cannot fit into a single TPU memory or the host CPU memory, one should use nested FSDP to implement the ZeRO-3 algorithm interleave submodule construction with inner FSDP wrapping, so that the full model never needs to be stored in memory during construction.
We illustrate these cases in https://github.com/ronghanghu/ptxla_scaling_examples, which provides examples of training a Vision Transformer (ViT) model with 10B+ parameters on a TPU v3 pod (with 128 cores) as well as other cases.
Design Notes
One might wonder why we need to develop a separate FSDP class in PyTorch/XLA instead of directly reusing PyTorch’s FSDP class or extending it to the XLA backend. The main motivation behind a separate FSDP class in PyTorch/XLA is that the native PyTorch’s FSDP class heavily relies on CUDA features that are not supported by XLA devices, while XLA also has several unique characteristics that need special handling. These distinctions require a different implementation of FSDP that would be much easier to build in a separate class.
Changes in API calls
One prominent distinction is that the native PyTorch FSDP is built upon separate CUDA streams for asynchronous execution in eager mode, while PyTorch/XLA runs in lazy mode and also does not support streams. In addition, TPU requires that all devices homogeneously run the same program. As a result, in the PyTorch/XLA FSDP implementation, CUDA calls and per-process heterogeneity need to be replaced by XLA APIs and alternative homogeneous implementations.
Tensor Storage Handling
Another prominent distinction is how to free a tensor’s storage, which is much harder in XLA than in CUDA. To implement ZeRO-3, one needs to free the storage of full parameters after a module’s forward pass, so that the next module can reuse this memory buffer for subsequent computation. PyTorch’s FSPD accomplishes this on CUDA by freeing the actual storage of a parameter p
via p.data.storage().resize_(0)
. However, XLA tensors do not have this .storage()
handle given that the XLA HLO IRs are completely functional and do not provide any ops to deallocate a tensor or resize its storage. Below the PyTorch interface, only the XLA compiler can decide when to free a TPU device memory corresponding to an XLA tensor, and a prerequisite is that the memory can only be released when the tensor object gets deallocated in Python – which cannot happen in FSDP because these parameter tensors are referenced as module attributes and also saved by PyTorch autograd for the backward pass.
Our solution to this issue is to split a tensor’s value properties from its autograd Variable properties, and to free a nn.Parameter
tensor by setting its .data
attribute to a dummy scalar of size 1. This way the actual data tensor for the full parameter gets dereferenced in Python so that XLA can recycle its memory for other computation, while autograd can still trace the base nn.Parameter
as a weak reference to the parameter data. To get this to work, one also needs to handle views over the parameters as views in PyTorch also hold references to its actual data (this required fixing a shape-related issue with views in PyTorch/XLA).
Working with XLA compiler
The solution above should be enough to free full parameters if the XLA compiler faithfully preserves the operations and their execution order in our PyTorch program. But there is another problem – XLA attempts to optimize the program to speed up its execution by applying common subexpression elimination (CSE) to the HLO IRs. In a naive implementation of FSDP, the XLA compiler typically eliminates the 2nd all-gather in the backward pass to reconstruct the full parameters when it sees that it is a repeated computation from the forward pass, and directly holds and reuses the full parameters we want to free up after the forward pass. To guard against this undesired compiler behavior, we introduced the optimization barrier op into PyTorch/XLA and used it to stop eliminating the 2nd all-gather. This optimization barrier is also applied to a similar case of gradient checkpointing to prevent CSE between forward and backward passes that could eliminate the rematerialization.
In the future, if the distinctions between CUDA and XLA become not as prominent as mentioned above, it could be worth considering a merge of the PyTorch/XLA FSDP with the native PyTorch FSDP to have a unified interface.
Acknowledgments
Thanks to Junmin Hao from AWS for reviewing the PyTorch/XLA FSDP pull request. Thanks to Brian Hirsh from the Meta PyTorch team for support on the PyTorch core issues. Thanks to Isaack Karanja, Will Cromar, and Blake Hechtman from Google for support on GCP, XLA, and TPU issues.
Thanks to Piotr Dollar, Wan-Yen Lo, Alex Berg, Ryan Mark, Kaiming He, Xinlei Chen, Saining Xie, Shoubhik Debnath, Min Xu, and Vaibhav Aggarwal from Meta FAIR for various TPU-related discussions.
Large and Fully Charged: Polestar 3 Sets New Standard for Premium Electric SUVs
The age of electric vehicles has arrived and, with it, an entirely new standard for premium SUVs.
Polestar, the performance EV brand spun out from Volvo Cars, launched its third model today in Copenhagen. With the Polestar 3, the automaker has taken SUV design back to the drawing board, building a vehicle as innovative as the technology it features.
The EV premieres a new aerodynamic profile from the brand, in addition to sustainable materials and advanced active and passive safety systems. The Polestar 3 also maintains some attributes of a traditional SUV, including a powerful and wide stance.
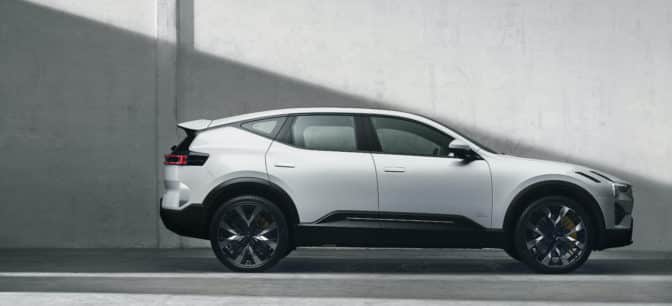
It features a 14.5-inch center display for easily accessible infotainment, in addition to 300 miles of battery range to tackle trips of any distance.
The Polestar 3 is the brand’s first SUV, as well as its first model to run on the high-performance, centralized compute of the NVIDIA DRIVE platform. This software-defined architecture lends the Polestar 3 its cutting-edge personality, making it an SUV that tops the list in every category.
Reigning Supreme
The crown jewel of a software-defined vehicle is its core compute — and the Polestar 3 is built with top-of-the-line hardware and software.
The NVIDIA DRIVE high-performance AI compute platform processes data from the SUV’s multiple sensors and cameras to enable advanced driver-assistance safety (ADAS) features and driver monitoring.
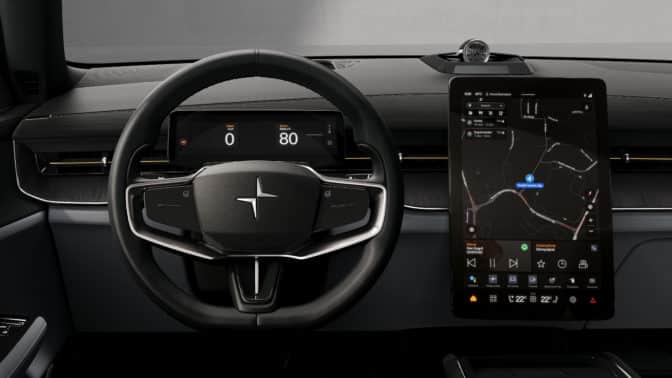
This ADAS system combines technology from Zenseact, Luminar and Smart Eye that integrates seamlessly thanks to the centralized computing power of NVIDIA DRIVE.
By running on a software-defined architecture, these automated driving features will continue to gain new functionality via over-the-air updates and eventually perform autonomous highway driving.
The Polestar 3 customers’ initial purchase won’t remain the same years or even months later — it will be constantly improving and achieving capabilities not yet even dreamed of.
Charging Ahead
The Polestar 3 kicks off a new phase for the automaker, which is accelerating its product and international growth plans.
The SUV will begin deliveries late next year. Starting with the Polestar 3, the automaker expects to launch a new car every year for the next three years and aims to expand its presence to at least 30 global markets by the end of 2023.
The automaker is targeting 10x growth in global sales, to reach 290,000 vehicles sold by the end of 2025 from about 29,000 in 2021.
And with its future-forward SUV, Polestar is adding a dazzling jewel to its already star-studded crown.
The post Large and Fully Charged: Polestar 3 Sets New Standard for Premium Electric SUVs appeared first on NVIDIA Blog.