Announcing the winners of the 2022 AI4AI Research request for proposals
In August, Meta launched the 2022 AI4AI Research request for proposals (RFP). Today, we’re announcing the winners of this award.Read More
Souped-Up Auto Quotes: ProovStation Delivers GPU-Driven AI Appraisals
Vehicle appraisals are getting souped up with a GPU-accelerated AI overhaul.
ProovStation, a four-year-old startup based in Lyon, France, is taking on the ambitious computer-vision quest of automating vehicle inspection and repair estimates, aiming AI-driven super-high-resolution stations at businesses worldwide.
It recently launched three of its state-of-the-art vehicle inspection scanners at French retail giant Carrefour’s Montesson, Vénissieux and Aix-en-Provence locations. The ProovStation drive-thru vehicle scanners are deployed at Carrefour parking lots for drivers to pull in to experience the free service.
The self-serve stations are designed for users to provide vehicle info and ride off with a value report and repair estimate in under two minutes. It also enables drivers to obtain a dealer offer to buy their car as quickly as within just seconds — which holds promise for consumers, as well as used car dealers and auctioneers.
Much is at play across cameras and sensors, high-fidelity graphics, multiple damage detection models, and models and analytics to turn damage detection into repair estimates and purchase offers.
“People often ask me how I’ve gotten so much AI going in this, and I tell them it’s because I work with NVIDIA Inception,” said Gabriel Tissandier, general manager and chief product officer at ProovStation.
Tapping into NVIDIA GPUs and NVIDIA Metropolis software development kits enables ProovStation to scan 5GB of image and sensor data per car and apply multiple vision AI detection models simultaneously, among other tasks.
ProovStation uses the NVIDIA DeepStream SDK to build its sophisticated vision AI pipeline and optimizes AI inference throughput using Triton Inference Server.
The setup enables ProovStation to run inference for the quick vehicle analysis turnarounds on this groundbreaking industrial edge AI application.
Driving Advances: Bernard Groupe Dealerships
ProovStation is deploying its stations at a quick clip. That’s been possible because founder Gabriel Tissandier in the early stages connected with an ideal ally in Cedric Bernard, whose family’s Groupe Bernard car dealerships and services first invested in 2017 to boost its own operations.
Groupe Bernard has collected massive amounts of image data from its own businesses for ProovStation prototypes. Bernard left the family business to join Tissandier as the startup’s co-founder and CEO, and co-founder Anton Komyza joined them, and it’s been a wild ride of launches since.
ProovStation is a member of NVIDIA Inception, a program that accelerates cutting-edge startups with access to hardware and software platforms, technical training, as well as AI ecosystem support.
“People often ask me how I’ve gotten so much AI going in this, and I tell them it’s because I work with NVIDIA Inception,” said Tissandier, general manager and chief product officer at ProovStation.
Launching AI Stations Across Markets
ProovStation has deployed 35 scanning stations into operation so far, and it expects to double that number next year. It has launched its powerful edge AI-driven stations in Europe and the United States.
Early adopters include Groupe Bernard, U.K. vehicle sales site BCA Marketplace, OK Mobility car rentals in Spain and Germany’s Sixt car rentals. It also works with undisclosed U.S. automakers and a major online vehicle seller.
Car rental service Sixt has installed a station at Lyon Saint-Exupery Airport with the aim of making car pickups and returns easier.
“Sixt wants to really change the experience of renting a car,” said Tissandier.
Creating an ‘AI Super Factory’ for Damage Datasets
ProovStation has built up data science expertise and a dedicated team to handle its many specialized datasets for the difficult challenge of damage detection.
“To go from a damage review to a damage estimate can sometimes be really tricky,” said Tissandier.
ProovStation has a team of 10 experts in its AI Super Factory dedicated to labeling data with its own specialized software. They have processed more than 2 million images with labels so far, defining a taxonomy of more than 100 types of damages and more than 100 types of parts.
“We knew we needed this level of accuracy to make it reliable and efficient for businesses. Labeling images is super important, especially for us, so we invented some ways to label specific damages,” he said.
Tissandier said that the data science team members and others are brought up to speed on AI with courses from the NVIDIA Deep Learning Institute.
Delivering Data Collection With NVIDIA Industrial Edge AI
ProovStation scans a vehicle with 10 different cameras in its station and takes 300 images — or 5GB of data — for running on its detection models. NVIDIA GPUs enable ProovStation’s AI inference pipeline in 90 seconds to provide detection, assessment of damages, localization, measurements and estimates. Wheels are scanned with an electromagnetic frequency device from tire company Michelin for wear estimates. All of it runs on the NVIDIA edge AI system.
Using two NVIDIA GPUs in a station allows ProovStation to process all of this in high-resolution image analysis for improved accuracy. That data is also transferred to the cloud so ProovStation’s data science team can use it for further training.
Cameras, lighting and positioning are big issues. Detection models can be thrown off by things like glares on glass-shiney cars. ProovStation uses a defectometry model, which allows it to run detection while projecting lines onto vehicle surfaces, highlighting spots where problems appear in the lines.
It’s a challenging problem to solve that leads to business opportunities.
“All of the automotive industry is inspecting cars to provide services — to sell you new tires, to repair your car or windshield, it always starts with an inspection,” said Tissandier.
The post Souped-Up Auto Quotes: ProovStation Delivers GPU-Driven AI Appraisals appeared first on NVIDIA Blog.
The science of strength: How data analytics is transforming college basketball
In the 1990s, if you suggested that the corner three-pointer was the best shot in basketball, you might have been laughed out of the gym.
The game was still dominated largely by a fleet of seven-foot centers, most of whom couldn’t shoot from more than a few feet out from the basket. Even the game’s best player, Michael Jordan, was a mid-range specialist who averaged under two three-point attempts per game for his career.
Fast forward to today, and the best players average around a dozen long-ball attempts per game — typically favoring shots from the corner.
What’s changed? Analytics.
“When I first started in the profession, 10 to 12 years ago, data analytics was almost nonexistent in training rooms,” says Adam Petway, the director of strength and conditioning for men’s basketball at the University of Louisville. “Today, we have force platform technology, we have velocity-based training, we have GPS tracking during games and in training, all to get a more objective analysis to help our athletes. So it’s grown exponentially.”
Petway, who previously worked on the coaching staffs of the NBA’s Philadelphia 76ers and Washington Wizards, holds a bachelor’s degree in sports science, an MBA with an emphasis in sport management, and a doctorate in sports science. Recently, he extended his education through MIT Professional Education’s Applied Data Science Program (ADSP).
“The impetus behind enrolling in ADSP was primarily a curiosity to learn and a desire to get better,” Petway says. “In my time in pro and college sports, we’ve had whole departments dedicated to data science, so I know it’s a skill set I’ll need in the future.”
Applying new skills
Petway took classes in a live online format. Although he was the only strength and conditioning coach in his cohort — learning alongside lawyers, professors, and business executives — he says that the focus on data gave all of his classmates a common language of sorts.
“In many people’s minds, the worlds of data science and NCAA strength and conditioning training may not cross. We are finding that there are many other professional and industry sectors that can benefit from data science and analytics, which explains why we are seeing an ever-growing range of professionals from around the globe enroll in our Applied Data Science Program,” says Bhaskar Pant, executive director of MIT Professional Education. “It’s exciting to hear how change-makers like Adam are using the knowledge they gained from the program to tackle their most pressing challenges using data science tools.”
“Having access to such high-level practitioners within data science was something that I found very, very helpful,” Petway says. “The chance to interact with my classmates, and the chance to interact in small groups with the professionals and the professors, was unbelievable. When you’re writing code in Python you might mess up a semicolon and a comma, and get 200 characters into the code and realize that it’s not going to work. So the ability to stop and ask questions, and really get into the material with a cohort of peers from different industries, that was really helpful.”
Petway points to his newfound abilities to code in Python, and to run data through artificial intelligence programs that utilize unsupervised learning techniques, as major takeaways from his experience. Sports teams produce a wealth of data, he notes, but coaches need to be able to process that information in ways that lead to actionable insights.
“Now I’m able to create decision trees, do visualization with data, and run a principal component analysis,” Petway says. “So instead of relying on third-party companies to come in and tell me what to do, I can take all of that data and disseminate the results myself, which not only saves me time, but it saves a lot of money.”
In addition to giving him new capabilities in his coaching role, the skills were crucial to the research for a paper that Petway and a team of several other authors published in the International Journal of Strength and Conditioning this year. “The data came from my PhD program around five years ago,” Petway notes. “I had the data already, but I couldn’t properly visualize it and analyze it until I took the MIT Professional Education course.”
“MIT’s motto is ‘mens et manus’ (‘mind and hand’), which translates to experience-based learning. As such, there was great thought put into how the Applied Data Science Program is structured. The expectation is that every participant not only gains foundational skills, but also learns how to apply that knowledge in real-world scenarios. We are thrilled to see learning from our course applied to top-level college basketball,” says Munther Dahleh, director of the Institute for Data, Systems, and Society, the William A. Coolidge Professor of Electrical Engineering and Computer Science at MIT, and one of the instructors of ADSP.
Data’s growing role in sports
Analytics are pushing the field of strength and conditioning far beyond the days when trainers would simply tell players to do a certain number of reps in the weight room, Petway says. Wearable devices help to track how much ground athletes cover during practice, as well as their average speed. Data from a force platform helps Petway to analyze the force with which basketball players jump (and land), and even to determine how much force an athlete is generating from each leg. Using a tool called a linear position transducer, Petway can measure how fast athletes are moving a prescribed load during weight-lifting exercises.
“Instead of telling someone to do 90 percent of their squat max, we’re telling them to squat 200 kilos, and to move it at a rate above one meter per second,” says Petway. “So it’s more power- and velocity-driven than your traditional weight training.”
The goal is to not only improve athlete’s performance, Petway says, but also to create training programs that minimize the chance of injury. Sometimes, that means deviating from well-worn sports cliches about “giving 110 percent” or “leaving it all on the court.”
“There’s a misconception that doing more is always better,” Petway says. “One of my mentors would always say, ‘Sometimes you have to have the courage to do less.’ The most important thing for our athletes is being available for competition. We can use data analytics now to forecast the early onset of fatigue. If we see that their power output in the weight room is decreasing, we may need to intervene with rest before things get worse. It’s about using information to make more objective decisions.”
The ability to create visuals from data, Petway says, has greatly enhanced his ability to communicate with athletes and other coaches about what he’s seeing in the numbers. “It’s a really powerful tool, being able to take a bunch of data points and show that things are trending up or down, along with the intervention we’re going to need to make based on what the data suggests,” he says.
Ultimately, Petway notes, coaches are primarily interested in just one data point: wins and losses. But as more sports professionals see that data science can lead to more wins, he says, analytics will continue to gain a foothold in the industry. “If you can show that preparing a certain way leads to a higher likelihood that the team will win, that really speaks coaches’ language,” he says. “They just want to see results. And if data science can help deliver those results, they’re going to be bought in.”
The science of strength: How data analytics is transforming college basketball
In the 1990s, if you suggested that the corner three-pointer was the best shot in basketball, you might have been laughed out of the gym.
The game was still dominated largely by a fleet of seven-foot centers, most of whom couldn’t shoot from more than a few feet out from the basket. Even the game’s best player, Michael Jordan, was a mid-range specialist who averaged under two three-point attempts per game for his career.
Fast forward to today, and the best players average around a dozen long-ball attempts per game — typically favoring shots from the corner.
What’s changed? Analytics.
“When I first started in the profession, 10 to 12 years ago, data analytics was almost nonexistent in training rooms,” says Adam Petway, the director of strength and conditioning for men’s basketball at the University of Louisville. “Today, we have force platform technology, we have velocity-based training, we have GPS tracking during games and in training, all to get a more objective analysis to help our athletes. So it’s grown exponentially.”
Petway, who previously worked on the coaching staffs of the NBA’s Philadelphia 76ers and Washington Wizards, holds a bachelor’s degree in sports science, an MBA with an emphasis in sport management, and a doctorate in sports science. Recently, he extended his education through MIT Professional Education’s Applied Data Science Program (ADSP).
“The impetus behind enrolling in ADSP was primarily a curiosity to learn and a desire to get better,” Petway says. “In my time in pro and college sports, we’ve had whole departments dedicated to data science, so I know it’s a skill set I’ll need in the future.”
Applying new skills
Petway took classes in a live online format. Although he was the only strength and conditioning coach in his cohort — learning alongside lawyers, professors, and business executives — he says that the focus on data gave all of his classmates a common language of sorts.
“In many people’s minds, the worlds of data science and NCAA strength and conditioning training may not cross. We are finding that there are many other professional and industry sectors that can benefit from data science and analytics, which explains why we are seeing an ever-growing range of professionals from around the globe enroll in our Applied Data Science Program,” says Bhaskar Pant, executive director of MIT Professional Education. “It’s exciting to hear how change-makers like Adam are using the knowledge they gained from the program to tackle their most pressing challenges using data science tools.”
“Having access to such high-level practitioners within data science was something that I found very, very helpful,” Petway says. “The chance to interact with my classmates, and the chance to interact in small groups with the professionals and the professors, was unbelievable. When you’re writing code in Python you might mess up a semicolon and a comma, and get 200 characters into the code and realize that it’s not going to work. So the ability to stop and ask questions, and really get into the material with a cohort of peers from different industries, that was really helpful.”
Petway points to his newfound abilities to code in Python, and to run data through artificial intelligence programs that utilize unsupervised learning techniques, as major takeaways from his experience. Sports teams produce a wealth of data, he notes, but coaches need to be able to process that information in ways that lead to actionable insights.
“Now I’m able to create decision trees, do visualization with data, and run a principal component analysis,” Petway says. “So instead of relying on third-party companies to come in and tell me what to do, I can take all of that data and disseminate the results myself, which not only saves me time, but it saves a lot of money.”
In addition to giving him new capabilities in his coaching role, the skills were crucial to the research for a paper that Petway and a team of several other authors published in the International Journal of Strength and Conditioning this year. “The data came from my PhD program around five years ago,” Petway notes. “I had the data already, but I couldn’t properly visualize it and analyze it until I took the MIT Professional Education course.”
“MIT’s motto is ‘mens et manus’ (‘mind and hand’), which translates to experience-based learning. As such, there was great thought put into how the Applied Data Science Program is structured. The expectation is that every participant not only gains foundational skills, but also learns how to apply that knowledge in real-world scenarios. We are thrilled to see learning from our course applied to top-level college basketball,” says Munther Dahleh, director of the Institute for Data, Systems, and Society, the William A. Coolidge Professor of Electrical Engineering and Computer Science at MIT, and one of the instructors of ADSP.
Data’s growing role in sports
Analytics are pushing the field of strength and conditioning far beyond the days when trainers would simply tell players to do a certain number of reps in the weight room, Petway says. Wearable devices help to track how much ground athletes cover during practice, as well as their average speed. Data from a force platform helps Petway to analyze the force with which basketball players jump (and land), and even to determine how much force an athlete is generating from each leg. Using a tool called a linear position transducer, Petway can measure how fast athletes are moving a prescribed load during weight-lifting exercises.
“Instead of telling someone to do 90 percent of their squat max, we’re telling them to squat 200 kilos, and to move it at a rate above one meter per second,” says Petway. “So it’s more power- and velocity-driven than your traditional weight training.”
The goal is to not only improve athlete’s performance, Petway says, but also to create training programs that minimize the chance of injury. Sometimes, that means deviating from well-worn sports cliches about “giving 110 percent” or “leaving it all on the court.”
“There’s a misconception that doing more is always better,” Petway says. “One of my mentors would always say, ‘Sometimes you have to have the courage to do less.’ The most important thing for our athletes is being available for competition. We can use data analytics now to forecast the early onset of fatigue. If we see that their power output in the weight room is decreasing, we may need to intervene with rest before things get worse. It’s about using information to make more objective decisions.”
The ability to create visuals from data, Petway says, has greatly enhanced his ability to communicate with athletes and other coaches about what he’s seeing in the numbers. “It’s a really powerful tool, being able to take a bunch of data points and show that things are trending up or down, along with the intervention we’re going to need to make based on what the data suggests,” he says.
Ultimately, Petway notes, coaches are primarily interested in just one data point: wins and losses. But as more sports professionals see that data science can lead to more wins, he says, analytics will continue to gain a foothold in the industry. “If you can show that preparing a certain way leads to a higher likelihood that the team will win, that really speaks coaches’ language,” he says. “They just want to see results. And if data science can help deliver those results, they’re going to be bought in.”
Host code-server on Amazon SageMaker
Machine learning (ML) teams need the flexibility to choose their integrated development environment (IDE) when working on a project. It allows you to have a productive developer experience and innovate at speed. You may even use multiple IDEs within a project. Amazon SageMaker lets ML teams choose to work from fully managed, cloud-based environments within Amazon SageMaker Studio, SageMaker Notebook Instances, or from your local machine using local mode.
SageMaker provides a one-click experience to Jupyter and RStudio to build, train, debug, deploy, and monitor ML models. In this post, we will also share a solution for hosting code-server on SageMaker.
With code-server, users can run VS Code on remote machines and access it in a web browser. For ML teams, hosting code-server on SageMaker provides minimal changes to a local development experience, and allows you to code from anywhere, on scalable cloud compute. With VS Code, you can also use built-in Conda environments with AWS-optimized TensorFlow and PyTorch, managed Git repositories, local mode, and other features provided by SageMaker to speed up your delivery. For IT admins, it allows you to standardize and expedite the provisioning of managed, secure IDEs in the cloud, to quickly onboard and enable ML teams in their projects.
Solution overview
In this post, we cover installation for both Studio environments (Option A), and notebook instances (Option B). For each option, we walk through a manual installation process that ML teams can run in their environment, and an automated installation that IT admins can set up for them via the AWS Command Line Interface (AWS CLI).
The following diagram illustrates the architecture overview for hosting code-server on SageMaker.
Our solution speeds up the install and setup of code-server in your environment. It works for both JupyterLab 3 (recommended) and JupyterLab 1 that run within Studio and SageMaker notebook instances. It is made of shell scripts that do the following based on the option.
For Studio (Option A), the shell script does the following:
- Installs code-server on the Jupyter Server for a user profile. For more details, see Dive deep into Amazon SageMaker Studio Notebooks architecture.
- Adds a code-server shortcut on the Studio launcher for fast access to the IDE.
- Creates a dedicated Conda environment for managing dependencies.
- Installs the Python extension on the IDE.
For SageMaker notebook instances (Option B), the shell script does the following:
- Installs code-server.
- Adds a code-server shortcut on the Jupyter notebook file menu and JupyterLab launcher for fast access to the IDE.
- Creates a dedicated Conda environment for managing dependencies.
- Installs the Python and Docker extensions on the IDE.
In the following sections, we walk through the solution install process for Option A and Option B. Make sure you have access to Studio or a notebook instance.
Option A: Host code-server on Studio
To host code-server on Studio, complete the following steps:
- Choose System terminal in your Studio launcher.
- To install the code-server solution, run the following commands in your system terminal:
The commands should take a few seconds to complete.
- Reload the browser page, where you can see a Code Server button in your Studio launcher.
- Choose Code Server to open a new browser tab, allowing you to access code-server from your browser.
The Python extension is already installed, and you can get to work in your ML project.
You can open your project folder in VS Code and select the pre-built Conda environment to run your Python scripts.
Automate the code-server install for users in a Studio domain
As an IT admin, you can automate the installation for Studio users by using a lifecycle configuration. It can be done for all users’ profiles under a Studio domain or for specific ones. See Customize Amazon SageMaker Studio using Lifecycle Configurations for more details.
For this post, we create a lifecycle configuration from the install-codeserver script, and attach it to an existing Studio domain. The install is done for all the user profiles in the domain.
From a terminal configured with the AWS CLI and appropriate permissions, run the following commands:
After Jupyter Server restarts, the Code Server button appears in your Studio launcher.
Option B: Host code-server on a SageMaker notebook instance
To host code-server on a SageMaker notebook instance, complete the following steps:
- Launch a terminal via Jupyter or JupyterLab for your notebook instance.
If you use Jupyter, choose Terminal on the New menu.
- To install the code-server solution, run the following commands in your terminal:
The code-server and extensions installations are persistent on the notebook instance. However, if you stop or restart the instance, you need to run the following command to reconfigure code-server:
sudo ./setup-codeserver.sh
The commands should take a few seconds to run. You can close the terminal tab when you see the following.
- Now reload the Jupyter page and check the New menu again.
The Code Server option should now be available.
You can also launch code-server from JupyterLab using a dedicated launcher button, as shown in the following screenshot.
Choosing Code Server will open a new browser tab, allowing you to access code-server from your browser. The Python and Docker extensions are already installed, and you can get to work in your ML project.
Automate the code-server install on a notebook instance
As an IT admin, you can automate the code-server install with a lifecycle configuration running on instance creation, and automate the setup with one running on instance start.
Here, we create an example notebook instance and lifecycle configuration using the AWS CLI. The on-create
config runs install-codeserver, and on-start
runs setup-codeserver.
From a terminal configured with the AWS CLI and appropriate permissions, run the following commands:
The code-server install is now automated for the notebook instance.
Conclusion
With code-server hosted on SageMaker, ML teams can run VS Code on scalable cloud compute, code from anywhere, and speed up their ML project delivery. For IT admins, it allows them to standardize and expedite the provisioning of managed, secure IDEs in the cloud, to quickly onboard and enable ML teams in their projects.
In this post, we shared a solution you can use to quickly install code-server on both Studio and notebook instances. We shared a manual installation process that ML teams can run on their own, and an automated installation that IT admins can set up for them.
To go further in your learnings, visit AWSome SageMaker on GitHub to find all the relevant and up-to-date resources needed for working with SageMaker.
About the Authors
Giuseppe Angelo Porcelli is a Principal Machine Learning Specialist Solutions Architect for Amazon Web Services. With several years software engineering an ML background, he works with customers of any size to deeply understand their business and technical needs and design AI and Machine Learning solutions that make the best use of the AWS Cloud and the Amazon Machine Learning stack. He has worked on projects in different domains, including MLOps, Computer Vision, NLP, and involving a broad set of AWS services. In his free time, Giuseppe enjoys playing football.
Sofian Hamiti is an AI/ML specialist Solutions Architect at AWS. He helps customers across industries accelerate their AI/ML journey by helping them build and operationalize end-to-end machine learning solutions.
Eric Pena is a Senior Technical Product Manager in the AWS Artificial Intelligence Platforms team, working on Amazon SageMaker Interactive Machine Learning. He currently focuses on IDE integrations on SageMaker Studio . He holds an MBA degree from MIT Sloan and outside of work enjoys playing basketball and football.
Exploring the uncertainty of predictions
Tatevik Sekhposyan, Amazon Scholar and Texas A&M University professor, enjoys the flexibility of economics and how embracing uncertainty can enhance prediction.Read More
Real estate brokerage firm John L. Scott uses Amazon Textract to strike racially restrictive language from property deeds for homeowners
Founded more than 91 years ago in Seattle, John L. Scott Real Estate’s core value is Living Life as a Contribution®. The firm helps homebuyers find and buy the home of their dreams, while also helping sellers move into the next chapter of their home ownership journey. John L. Scott currently operates over 100 offices with more than 3,000 agents throughout Washington, Oregon, Idaho, and California.
When company operating officer Phil McBride joined the company in 2007, one of his initial challenges was to shift the company’s public website from an on-premises environment to a cloud-hosted one. According to McBride, a world of resources opened up to John L. Scott once the company started working with AWS to build an easily controlled, cloud-enabled environment.
Today, McBride is taking on the challenge of uncovering and modifying decades-old discriminatory restrictions in home titles and deeds. What he didn’t expect was enlisting the help of AWS for the undertaking.
In this post, we share how John L. Scott uses Amazon Textract and Amazon Comprehend to identify racially restrictive language from such documents.
A problem rooted in historic discrimination
Racial covenants restrict who can buy, sell, lease, or occupy a property based on race (see the following example document). Although no longer enforceable since the Fair Housing Act of 1968, racial covenants became pervasive across the country during the post-World War II housing boom and are still present in the titles of millions of homes. Racial covenants are direct evidence of the real estate industry’s complicity and complacency when it came to the government’s racist policies of the past, including redlining.
In 2019, McBride spoke in support of Washington state legislation that served as the next step in correcting the historic injustice of racial language in covenants. In 2021, a bill was passed that required real estate agents to provide notice of any unlawful recorded covenant or deed restriction to purchasers at the time of sale. A year after the legislation passed and homeowners were notified, John L. Scott discovered that only five homeowners in the state of Washington acted on updating their own property deeds.
“The challenge lies in the sheer volume of properties in the state of Washington, and the current system to update your deeds,” McBride said. “The process to update still is very complicated, so only the most motivated homeowners would put in the research and legwork to modify their deed. This just wasn’t going to happen at scale.”
Initial efforts to find restrictive language have found university students and community volunteers manually reading documents and recording findings. But in Washington state alone, millions of documents needed to be analyzed. A manual approach wouldn’t scale effectively.
Machine learning overcomes manual and complicated processes
With the support of AWS Global Impact Computing Specialists and Solutions Architects, John L. Scott has built an intelligent document processing solution that helps homeowners easily identify racially restrictive covenants in their property title documents. This intelligent document processing solution uses machine learning to scan titles, deeds, and other property documents, searching the text for racially restrictive language. The Washington State Association of County Auditors is also working with John L. Scott to provide digitized deeds, titles, and CC&Rs from their database, starting with King County, Washington.
Once these racial covenants are identified, John L. Scott team members guide homeowners through the process of modifying the discriminatory restrictions from their home’s title, with the support of online notary services such as Notarize.
With a goal of building a solution that the lean team at John L. Scott could manage, McBride’s team worked with AWS to evaluate different services and stitch them together in a modular, repeatable way that met the team’s vision and principles for speed and scale. To minimize management overhead and maximize scalability, the team worked together to build a serverless architecture for handling document ingestion and restrictive language identification using several key AWS services:
- Amazon Simple Storage Service – Documents are stored in an Amazon S3 data lake for secure and highly available storage.
- AWS Lambda – Documents are processed by Lambda as they arrive in the S3 data lake. Original document images are split into single-page files and analyzed with Amazon Textract (text detection) and Amazon Comprehend (text analysis).
- Amazon Textract – Amazon Textract automatically converts raw images into text blocks, which are scanned using fuzzy string pattern matching for restrictive language. When restrictive language is identified, Lambda functions create new image files that highlight the language using the coordinates supplied by Amazon Textract. Finally, records of the restrictive findings are stored in an Amazon DynamoDB table.
- Amazon Comprehend – Amazon Comprehend analyzes the text output from Amazon Textract and identifies useful data (entities) like dates and locations within the text. This information is key to identifying where and when restrictions were in effect.
The following diagram illustrates the architecture of the serverless ingestion and identification pipeline.
Building from this foundation, the team also incorporates parcel information (via GeoJSON and shapefiles) from county governments to identify affected property owners so they can be notified and begin the process of remediation. A forthcoming public website will also soon allow property owners to input their address to see if their property is affected by restrictive documents.
Setting a new example for the 21st Century
When asked about what’s next, McBride said working with Amazon Textract and Amazon Comprehend has helped his team serve as an example to other counties and real estate firms across the country who want to bring the project into their geographic area.
“Not all areas will have robust programs like we do in Washington state, with University of Washington volunteers indexing deeds and notifying the homeowners,” McBride said. “However, we hope offering this intelligent document processing solution in the public domain will help others drive change in their local communities.”
Learn more
- The Color of Law: A Forgotten History of How Our Government Segregated America by Richard Rothstein (book; audiobook)
- Racial covenants, a relic of the past, are still on the books across the country from NPR
- Racial Restrictive Covenants map from The Seattle Civil Rights & Labor History Project
About the authors
Jeff Stockamp is a Senior Solutions Architect based in Seattle, Washington. Jeff helps guide customers as they build well architected-applications and migrate workloads to AWS. Jeff is a constant builder and spends his spare time building Legos with his son.
Jarman Hauser is a Business Development and Go-to-Market Strategy leader at AWS. He works with customers on leveraging technology in unique ways to solve some of the worlds most challenging social, environmental, and economic challenges globally.
Moussa Koulbou is a Senior Solutions Architecture leader at AWS. He helps customers shape their cloud strategy and accelerate their digital velocity by creating the connection between intent and action. He leads a high-performing Solutions Architects team to deliver enterprise-grade solutions that leverage AWS cutting-edge technology to enable growth and solve the most critical business and social problems.
PyTorch’s Tracing Based Selective Build
Introduction
TL;DR: It can be challenging to run PyTorch on mobile devices, SBCs (Single Board Computers), and IOT devices. When compiled, the PyTorch library is huge and includes dependencies that might not be needed for the on-device use case.
To run a specific set of models on-device, we actually require only a small subset of the features in the PyTorch library. We found that using a PyTorch runtime generated using selective build can achieve up to 90% reduction in binary size (for the CPU and QuantizedCPU backends on an x86-64 build on Linux). In this blog, we share our experience of generating model-specific minimal runtimes using Selective Build and show you how to do the same.
Why is this important for app developers?
Using a PyTorch runtime generated by selective build can reduce the size of AI-powered apps by 30+ MB – a significant reduction for a typical mobile app! Making mobile applications more lightweight has many benefits – they are runnable on a wider variety of devices, consume less cellular data, and can be downloaded and updated faster on user’s devices.
What does the Developer Experience look like?
This method can work seamlessly with any existing PyTorch Mobile deployment workflows. All you need to do is replace the general PyTorch runtime library with a runtime customized for the specific models you wish to use in your application. The general steps in this process are:
- Build the PyTorch Runtime in instrumentation mode (this is called an instrumentation build of PyTorch). This will record the used operators, kernels and features.
- Run your models through this instrumentation build by using the provided model_tracer binary. This will generate a single YAML file that stores all the features used by your model. These features will be preserved in the minimal runtime.
- Build PyTorch using this YAML file as input. This is the selective build technique, and it greatly reduces the size of the final PyTorch binary.
- Use this selectively-built PyTorch library to reduce the size of your mobile application!
Building the PyTorch Runtime in a special “instrumentation” mode ( by passing the TRACING_BASED=1
build option) generates an instrumentation build runtime of PyTorch, along with a model_tracer binary. Running a model with this build allows us to trace the parts of PyTorch used by the model.
Figure 1: Instrumentation build of PyTorch
# Clone the PyTorch repo
git clone https://github.com/pytorch/pytorch.git
cd pytorch
# Build the model_tracer
USE_NUMPY=0 USE_DISTRIBUTED=0 USE_CUDA=0 TRACING_BASED=1
python setup.py develop
Now this instrumentation build is used to run a model inference with representative inputs. The model_tracer binary observes parts of the instrumentation build that were activated during the inference run, and dumps it to a YAML file.
Figure 2: YAML file generated by running model(s) on an instrumentation build
# Generate YAML file
./build/bin/model_tracer
--model_input_path /tmp/path_to_model.ptl
--build_yaml_path /tmp/selected_ops.yaml
Now we build the PyTorch Runtime again, but this time using the YAML file generated by the tracer. The runtime now only includes those parts that are needed for this model. This is called “Selectively built PyTorch runtime” in the diagram below.
# Clean out cached configuration
make clean
# Build PyTorch using Selected Operators (from the YAML file)
# using the host toolchain, and use this generated library
BUILD_PYTORCH_MOBILE_WITH_HOST_TOOLCHAIN=1
USE_LIGHTWEIGHT_DISPATCH=0
BUILD_LITE_INTERPRETER=1
SELECTED_OP_LIST=/tmp/selected_ops.yaml
TRACING_BASED=1
./scripts/build_mobile.sh
Figure 3: Selective Build of PyTorch and model execution on a selectively built PyTorch runtime
Show me the code!
We’ve put together a notebook to illustrate what the process above looks like in code using a simple PyTorch model.
For a more hands-on tutorial to deploy this on Android/iOS this tutorial should be helpful.
Technical FAQs
Why is Tracing needed for a Selective Build of PyTorch?
In PyTorch, CPU kernels can call other operators via the PyTorch Dispatcher. Simply including the set of root operators called directly by the model is not sufficient as there might be many more being called under-the-hood transitively. Running the model on representative inputs and observing the actual list of operators called (aka “tracing”) is the most accurate way of determining what parts of PyTorch are used.
Additionally, factors such as which dtypes a kernel should handle are also runtime features that depend on actual input provided to the model. Hence, the tracing mechanism is extremely suitable for this purpose.
Which features can be selected (in or out) by using Tracing Based Selective Build?
The following features can be selected for the PyTorch runtime during the tracing based selective build process:
- CPU/QuantizedCPU kernels for PyTorch’s ATen Operators: If a PyTorch Operator is not needed by a model targeted at a selectively built runtime, then the registration of that CPU kernel is omitted in the runtime. This is controlled via Torchgen code-gen.
- Primary Operators: This is controlled by a macro named TORCH_SELECTIVE_SCHEMA (via templated selective build) that either selects a primary operator or de-selects it based on information in a generated header file.
- Code that handles specific dtypes in CPU kernels: This is performed by generating exception throws in specific case statements in the switch case generated by the macro AT_PRIVATE_CHECK_SELECTIVE_BUILD.
- Registration of Custom C++ Classes that extend PyTorch: This is controlled by the macro TORCH_SELECTIVE_CLASS, which can be used when registering Custom C++ Classes. The torch::selective_class_<> helper is to be used in conjunction with the macro TORCH_SELECTIVE_CLASS.
What is the structure of the YAML file used during the build?
The YAML file generated after tracing looks like the example below. It encodes all the elements of the “selectable” build feature as specified above.
include_all_non_op_selectives: false
build_features: []
operators:
aten::add.Tensor:
is_used_for_training: false
is_root_operator: true
include_all_overloads: false
aten::len.t:
is_used_for_training: false
is_root_operator: true
include_all_overloads: false
kernel_metadata:
_local_scalar_dense_cpu:
- Float
add_stub:
- Float
copy_:
- Bool
- Byte
mul_cpu:
- Float
custom_classes: []
How exactly is code eliminated from the generated binary?
Depending on the specific scenario, there are 2 main techniques that are used to hint the compiler and linker about unused and unreachable code. This code is then cleaned up by the compiler or linker as unreachable code.
[1] Unreferenced functions removed by the Linker
When a function that isn’t transitively referenced from any visible function is present in the compiled object files that are being linked together, the linker will remove it (if the right build flags are provided). This is leveraged in 2 scenarios by the selective build system.
Kernel Registration in the Dispatcher
If an operator’s kernel isn’t needed, then it isn’t registered with the dispatcher. An unregistered kernel means that the function is unreachable, and it will be removed by the linker.
Templated Selective Build
The general idea here is that a class template specialization is used to select a class that either captures a reference to a function or not (depending on whether it’s used) and the linker can come along and clean out the unreferenced function.
For example, in the code below, there’s no reference to the function “fn2
”, so it will be cleaned up by the linker since it’s not referenced anywhere.
#include <vector>
#include <cstdio>
template <typename T, bool>
struct FunctionSelector {
T fn_;
FunctionSelector(T fn): fn_(fn) {}
T get() { return this->fn_; }
};
// The "false" specialization of this class does NOT retain the argument passed
// to the class constructor, which means that the function pointer passed in
// is considered to be unreferenced in the program (unless it is referenced
// elsewhere).
template <typename T>
struct FunctionSelector<T, false> {
FunctionSelector(T) {}
};
template <typename T>
FunctionSelector<T, true> make_function_selector_true(T fn) {
return FunctionSelector<T, true>(fn);
}
template <typename T>
FunctionSelector<T, false> make_function_selector_false(T fn) {
return FunctionSelector<T, false>(fn);
}
typedef void(*fn_ptr_type)();
std::vector<fn_ptr_type> fns;
template <typename T>
void add_fn(FunctionSelector<T, true> fs) {
fns.push_back(fs.get());
}
template <typename T>
void add_fn(FunctionSelector<T, false>) {
// Do nothing.
}
// fn1 will be kept by the linker since it is added to the vector "fns" at
// runtime.
void fn1() {
printf("fn1n");
}
// fn2 will be removed by the linker since it isn't referenced at all.
void fn2() {
printf("fn2n");
}
int main() {
add_fn(make_function_selector_true(fn1));
add_fn(make_function_selector_false(fn2));
}
[2] Dead Code Eliminated by the Compiler
C++ Compilers can detect dead (unreachable) code by analyzing the code’s control flow statically. For example, if there’s a code-path that comes after an unconditional exception throw, then all the code after it will be marked as dead code and not converted to object code by the compiler. Typically, compilers require the use of the -fdce
flag to eliminate dead code.
In the example below, you can see that the C++ code on the left (in the red boxes) doesn’t have any corresponding generated object code on the right.
Figure 4: Dead Code Elimination by C++ Compilers
This property is leveraged in the bodies of PyTorch kernel implementations that have a lot of repeated code to handle multiple dtypes of a Tensor. A dtype is the underlying data-type that the Tensor stores elements of. This can be one of float, double, int64, bool, int8, etc…
Almost every PyTorch CPU kernel uses a macro of the form AT_DISPATCH_ALL_TYPES* that is used to substitute some code specialized for every dtype that the kernel needs to handle. For example:
AT_DISPATCH_ALL_TYPES_AND_COMPLEX_AND3(
kBool, kHalf, kBFloat16, dtype, "copy_kernel", [&] {
cpu_kernel_vec(
iter,
[=](scalar_t a) -> scalar_t { return a; },
[=](Vectorized<scalar_t> a) -> Vectorized<scalar_t> { return a; });
});
The macro AT_DISPATCH_ALL_TYPES_AND_COMPLEX_AND3
internally has a switch-case statement that looks like the code in Figure-4 above. The tracing process records the dtypes triggered for the kernel tag “copy_kernel
” and the build process processes these tags and inserts throw
statements in every case
statement that is handling the dtype that isn’t required for this kernel tag.
This is how dtype selectivity is implemented in PyTorch’s Tracing Based Selective Build.
Conclusion
Tracing Based Selective Build is a practical and scalable approach to selecting only the used parts of an application to retain code that static analysis can not detect. This code is usually extremely data/input dependent in nature.
This article provides detailed insights into how Tracing Based Selective Build works under the hood, and the technical details related to its implementation. These techniques can also be applied to other applications and situations that can benefit from reduced binary size.
AI Supercomputer to Power $200 Million Oregon State University Innovation Complex
As a civil engineer, Scott Ashford used explosives to make the ground under Japan’s Sendai airport safer in an earthquake. Now, as the dean of the engineering college at Oregon State University, he’s at ground zero of another seismic event.
In its biggest fundraising celebration in nearly a decade, Oregon State announced plans today for a $200 million center where faculty and students can plug into resources that will include one of the world’s fastest university supercomputers.
The 150,000-square-foot center, due to open in 2025, will accelerate work at Oregon State’s top-ranked programs in agriculture, computer sciences, climate science, forestry, oceanography, robotics, water resources, materials sciences and more with the help of AI.
A Beacon in AI, Robotics
In honor of a $50 million gift to the OSU Foundation from NVIDIA’s founder and CEO and his wife — who earned their engineering degrees at OSU and met in one of its labs — it will be named the Jen-Hsun and Lori Huang Collaborative Innovation Complex (CIC).
“The CIC and new supercomputer will help Oregon State be recognized as one of the world’s leading universities for AI, robotics and simulation,” said Ashford, whose engineering college includes more than 10,000 of OSU’s 35,000 students.
“We discovered our love for computer science and engineering at OSU,” said Jen-Hsun and Lori Huang. “We hope this gift will help inspire future generations of students also to fall in love with technology and its capacity to change the world.
“AI is the most transformative technology of our time,” they added. “To harness this force, engineering students need access to a supercomputer, a time machine, to accelerate their research. This new AI supercomputer will enable OSU students and researchers to make very important advances in climate science, oceanography, materials science, robotics and other fields.”
A Hub for Students
With an extended-reality theater, robotics and drone playground and a do-it-yourself maker space, the new complex is expected to attract students from across the university. “It has the potential to transform not only the college of engineering, but the entire university, and have a positive economic and environmental impact on the state and the nation,” Ashford said.
The three-story facility will include a clean room, as well as labs for materials scientists, environmental researchers and more.
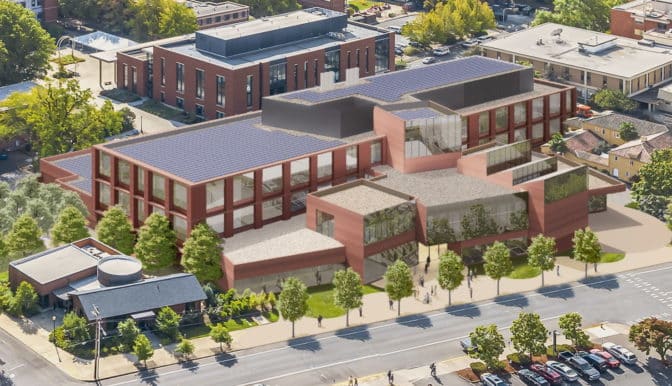
Ashford expects that over the next decade the center will attract top researchers, as well as research projects potentially worth hundreds of millions of dollars.
“Our donors and university leaders are excited about investing in a collaborative, transdisciplinary approach to problem solving and discovery — it will revitalize our engineering triangle and be an amazing place to study and conduct research,” he said.
A Forest of Opportunities
He gave several examples of the center’s potential. Among them:
- Environmental and electronics researchers may collaborate to design and deploy sensors and use AI to analyze their data, finding where in the ocean or forest hard-to-track endangered species are breeding so their habitats can be protected.
- Students can use augmented reality to train in simulated clean rooms on techniques for making leading-edge chips. Federal and Oregon state officials aim to expand workforce development for the U.S. semiconductor industry, Ashford said.
- Robotics researchers could create lifelike simulations of their drones and robots to accelerate training and testing. (Cassie, a biped robot designed at OSU, just made Guinness World Records for the fastest 100-meter dash by a bot.)
- Students at OSU and its sister college in Germany, DHBW-Ravensburg, could use NVIDIA Omniverse — a platform for building and operating metaverse applications and connecting their 3D pipelines — to enhance design of their award-winning, autonomous, electric race cars.
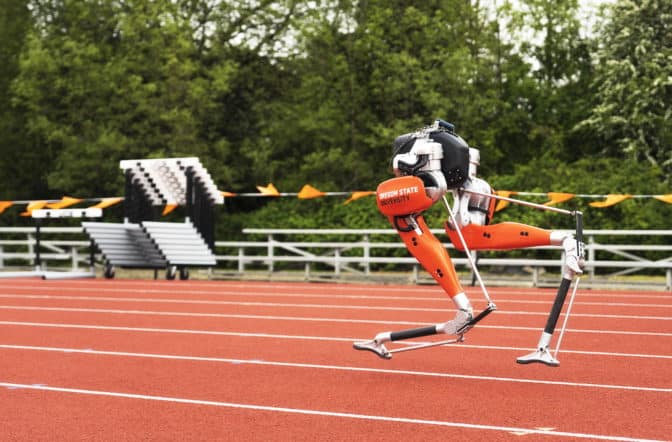
Building AI Models, Digital Twins
Such efforts will be accelerated with NVIDIA AI and Omniverse, software that can expand the building’s physical labs with simulations and digital twins so every student can have a virtual workbench.
OSU will get state-of-the-art NVIDIA DGX SuperPOD and OVX SuperPOD clusters once the complex’s data center is ready. With an eye on energy efficiency, water that cooled computer racks will then help heat more than 500,000 square feet of campus buildings.
The SuperPOD will likely include a mix of about 60 DGX and OVX systems — powered by next-generation CPUs, GPUs and networking — creating a system powerful enough to train the largest AI models and perform complex digital twin simulations. Ashford notes OSU won a project working with the U.S. Department of Energy because its existing computer center has a handful of DGX systems.
Advancing Diversity, Inclusion
At the Oct. 14 OSU Foundation event announcing the naming of the new complex, Oregon State officials thanked donors and kicked off a university-wide fundraising campaign. OSU has requested support from the state of Oregon for construction of the building and seeks additional philanthropic investments to expand its research and support its hiring and diversity goals.
OSU’s president, Jayathi Murthy, said the complex provides an opportunity to advance diversity, equity and inclusion in the university’s STEM education and research. OSU’s engineering college is already among the top-ranked U.S. schools for tenured or tenure-track engineering faculty who are women.
AI Universities Sprout
Oregon State also is among a small but growing set of universities accelerating their journeys in AI and high performance computing.
A recent whitepaper described efforts at University of Florida to spread AI across its curriculum as part of a partnership with NVIDIA that enabled it to install HiPerGator, a DGX SuperPOD based on NVIDIA DGX A100 systems with NVIDIA A100 Tensor Core GPUs.
Following Florida’s example, Southern Methodist University announced last fall its plans to make the Dallas area a hub of AI development around its new DGX SuperPOD.
“We’re seeing a lot of interest in the idea of AI universities from Asia, Europe and across the U.S.,” said Cheryl Martin, who leads NVIDIA’s efforts in higher education research.
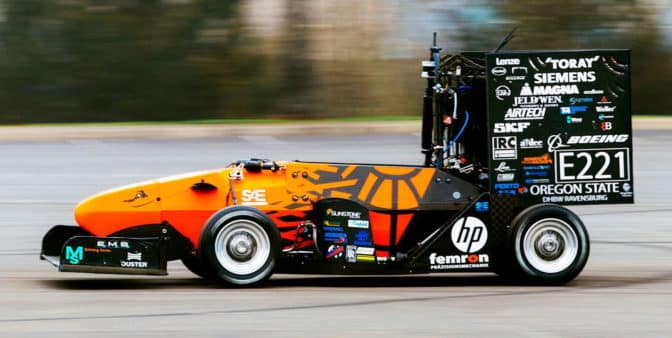
The post AI Supercomputer to Power $200 Million Oregon State University Innovation Complex appeared first on NVIDIA Blog.