Amazon SageMaker is a fully managed service for data science and machine learning (ML) workflows. It helps data scientists and developers prepare, build, train, and deploy high-quality ML models quickly by bringing together a broad set of capabilities purpose-built for ML.
In 2021, AWS announced the integration of NVIDIA Triton Inference Server in SageMaker. You can use NVIDIA Triton Inference Server to serve models for inference in SageMaker. By using an NVIDIA Triton container image, you can easily serve ML models and benefit from the performance optimizations, dynamic batching, and multi-framework support provided by NVIDIA Triton. Triton helps maximize the utilization of GPU and CPU, further lowering the cost of inference.
In some scenarios, users want to deploy multiple models. For example, an application for revising English composition always includes several models, such as BERT for text classification and GECToR to grammar checking. A typical request may flow across multiple models, like data preprocessing, BERT, GECToR, and postprocessing, and they run serially as inference pipelines. If these models are hosted on different instances, the additional network latency between these instances increases the overall latency. For an application with uncertain traffic, deploying multiple models on different instances will inevitably lead to inefficient utilization of resources.
Consider another scenario, in which users develop multiple models with different versions, and each model uses a different training framework. A common practice is to use multiple containers, each of which deploys a model. But this will cause increased workload and costs for development, operation, and maintenance. In this post, we discuss how SageMaker and NVIDIA Triton Inference Server can solve this problem.
Solution overview
Let’s look at how SageMaker inference works. SageMaker invokes the hosting service by running a Docker container. The Docker container launches a RESTful inference server (such as Flask) to serve HTTP requests for inference. The inference server loads the model and listens to port 8080 providing external service. The client application sends a POST request to the SageMaker endpoint, SageMaker passes the request to the container, and returns the inference result from the container to the client.
In our architecture, we use NVIDIA Triton Inference Server, which provides concurrent runs of multiple models from different frameworks, and we use a Flask server to process client-side requests and dispatch these requests to the backend Triton server. While launching a Docker container, the Triton server and Flask server are started automatically. The Triton server loads multiple models and exposes ports 8000, 8001, and 8002 as gRPC, HTTP, and metrics server. The Flask server listens to 8080 ports and parses the original request and payload, and then invokes the local Triton backend via model name and version information. For the client side, it adds the model name and model version in the request in addition to the original payload, so that Flask is able to route the inference request to the correct model on Triton server.
The following diagram illustrates this process.
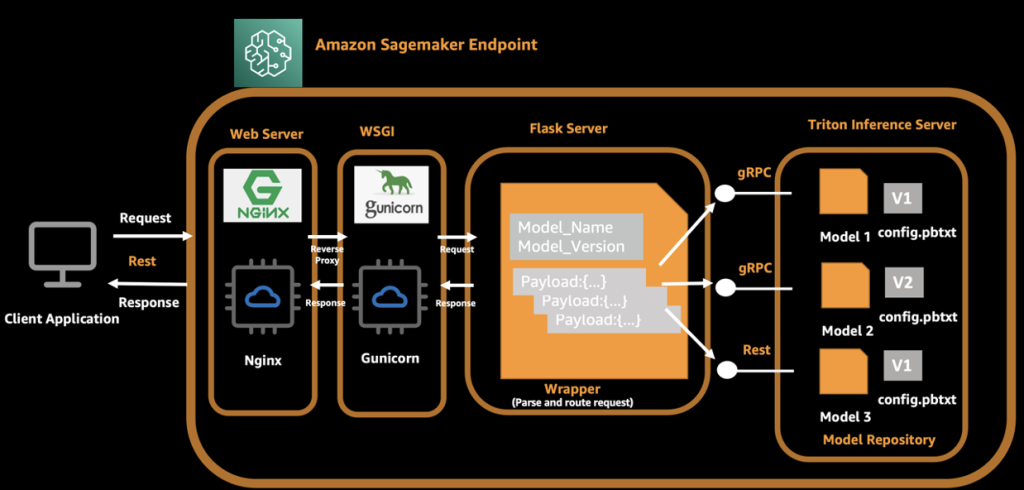
A complete API call from the client is as follows:
- The client assembles the request and initiates the request to a SageMaker endpoint.
- The Flask server receives and parses the request, and gets the model name, version, and payload.
- The Flask server assembles the request again and routes to the corresponding endpoint of the Triton server according to the model name and version.
- The Triton server runs an inference request and sends responses to the Flask server.
- The Flask server receives the response message, assembles the message again, and returns it to the client.
- The client receives and parses the response, and continues to subsequent business procedures.
In the following sections, we introduce the steps needed to prepare a model and build the TensorRT engine, prepare a Docker image, create a SageMaker endpoint, and verify the result.
Prepare models and build the engine
We demonstrate hosting three typical ML models in our solution: image classification (ResNet50), object detection (YOLOv5), and a natural language processing (NLP) model (BERT-base). NVIDIA Triton Inference Server supports multiple formats, including TensorFlow 1. x and 2. x, TensorFlow SavedModel, TensorFlow GraphDef, TensorRT, ONNX, OpenVINO, and PyTorch TorchScript.
The following table summarizes our model details.
Model Name |
Model Size |
Format |
ResNet50 |
52M |
Tensor RT |
YOLOv5 |
38M |
Tensor RT |
BERT-base |
133M |
ONNX RT |
NVIDIA provides detailed documentation describing how to generate the TensorRT engine. To achieve best performance, the TensorRT engine must be built over the device. This means the build time and runtime require the same computer capacity. For example, a TensorRT engine built on a g4dn instance can’t be deployed on a g5 instance.
You can generate your own TensorRT engines according to your needs. For test purposes, we prepared sample codes and deployable models with the TensorRT engine. The source code is also available on GitHub.
Next, we use an Amazon Elastic Compute Cloud (Amazon EC2) G4dn instance to generate the TensorRT engine with the following steps. We use YOLOv5 as an example.
- Launch a G4dn.2xlarge EC2 instance with the Deep Learning AMI (Ubuntu 20.04) in the
us-east-1
Region.
- Open a terminal window and use the
ssh
command to connect to the instance.
- Run the following commands one by one:
nvidia-docker run --gpus all -it --rm -v `pwd`/workspace:/workspace nvcr.io/nvidia/pytorch:22.04-py3
git clone -b v7.0.1 https://github.com/ultralytics/yolov5
pip install seaborn
pip install onnx-simplifier
cd yolov5
wget https://github.com/ultralytics/yolov5/releases/download/v6.2/yolov5s.pt
python export.py --weights yolov5s.pt --include onnx --simplify --imgsz 640 640 --device 0
onnxsim yolov5s.onnx yolov5s-sim.onnx
trtexec --onnx=yolov5s-sim.onnx --saveEngine=model.plan --explicitBatch --workspace=1024*12
- Create a
config.pbtxt
file:
name: "yolov5s"
platform: "tensorrt_plan"
input: [
{
name: "images"
data_type: TYPE_FP32
format: FORMAT_NONE
dims: [1, 3, 640, 640 ]
}
]
output: [
{
name: "output",
data_type: TYPE_FP32
dims: [1,25200,85 ]
}
]
- Create the following file structure and put the generated files in the appropriate location:
mkdir yolov5s
mkdir -p yolov5s/1
cp config.pbtxt yolov5s
cp model.plan yolov5s/1
yolov5s
├── 1
│ └── model.plan
└── config.pbtxt
Test the TensorRT engine
Before we deploy to SageMaker, we start a Triton server to verify these three models are configured correctly. Use the following command to start a Triton server and load the models:
docker run --gpus all --rm -p8000:8000 -p8001:8001 -v<MODEL_ROOT_DIR>/model_repository:/models nvcr.io/nvidia/tritonserver:22.04-py3 tritonserver --model-repository=/models
If you receive the following prompt message, it means the Triton server is started correctly.
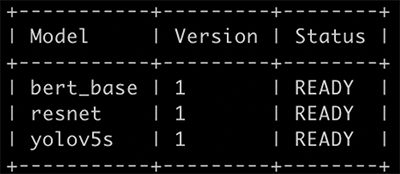
Enter nvidia-smi
in the terminal to see GPU memory usage.
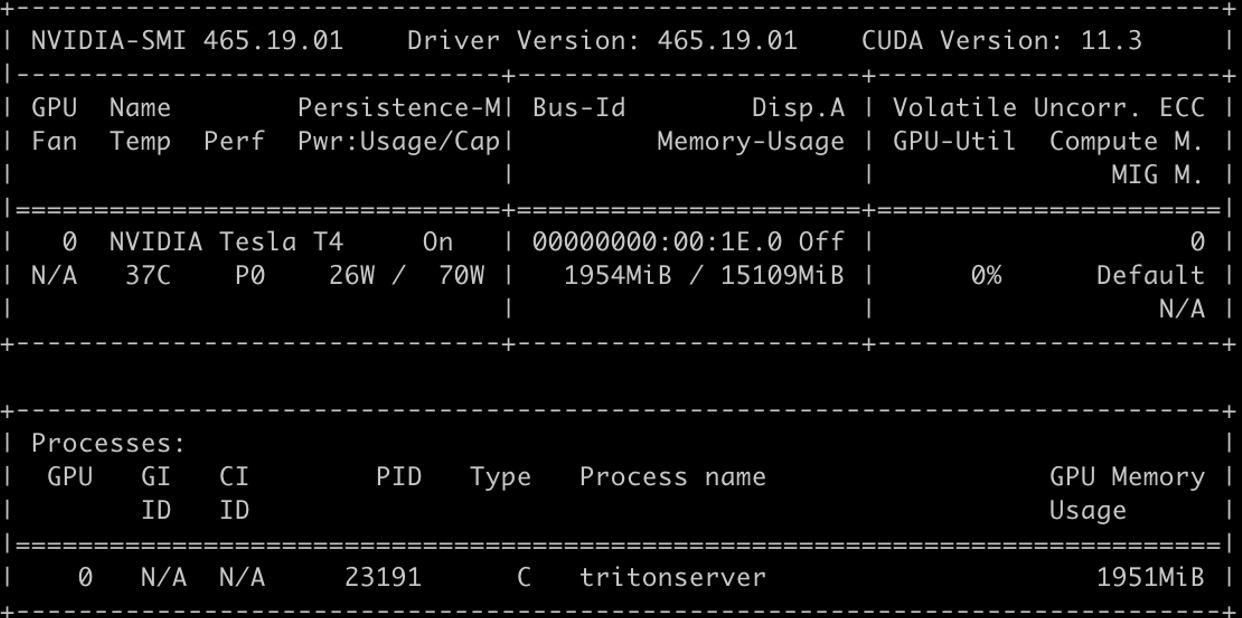
Client implementation for inference
The file structure is as follows:
- serve – The wrapper that starts the inference server. The Python script starts the NGINX, Flask, and Triton server.
- predictor.py – The Flask implementation for
/ping
and /invocations
endpoints, and dispatching requests.
- wsgi.py – The startup shell for the individual server workers.
- base.py – The abstract method definition that each client requires to implement their inference method.
- client folder – One folder per client:
- nginx.conf – The configuration for the NGINX primary server.
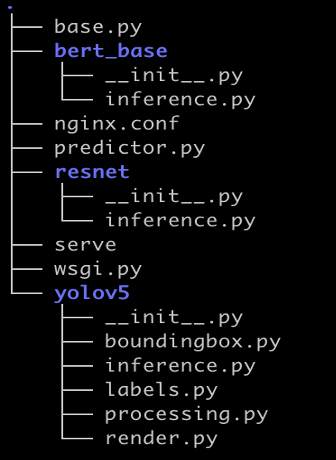
We define an abstract method to implement the inference interface, and each client implements this method:
from abc import ABC, abstractmethod
class Base(ABC):
@abstractmethod
def inference(self,img):
pass
The Triton server exposes an HTTP endpoint on port 8000, a gRPC endpoint on port 8001, and a Prometheus metrics endpoint on port 8002. The following is a sample ResNet client with a gRPC call. You can implement the HTTP interface or gRPC interface according to your use case.
from base import Base
import numpy as np
import tritonclient.grpc as grpcclient
from PIL import Image
import cv2
class Resnet(Base):
def image_transform_onnx(self, image, size: int) -> np.ndarray:
'''Image transform helper for onnx runtime inference.'''
img = cv2.cvtColor(image, cv2.COLOR_BGR2RGB) #OpenCV follows BGR convention and PIL follows RGB
image = Image.fromarray(img)
image = image.resize((size,size))
# now our image is represented by 3 layers - Red, Green, Blue
# each layer has a 224 x 224 values representing
image = np.array(image)
# dummy input for the model at export - torch.randn(1, 3, 224, 224)
image = image.transpose(2,0,1).astype(np.float32)
# our image is currently represented by values ranging between 0-255
# we need to convert these values to 0.0-1.0 - those are the values that are expected by our model
image /= 255
image = image[None, ...]
return image
def inference(self, img):
INPUT_SHAPE = (224, 224)
TRITON_IP = "localhost"
TRITON_PORT = 8001
MODEL_NAME = "resnet"
INPUTS = []
OUTPUTS = []
INPUT_LAYER_NAME = "input"
OUTPUT_LAYER_NAME = "output"
INPUTS.append(grpcclient.InferInput(INPUT_LAYER_NAME, [1, 3, INPUT_SHAPE[0], INPUT_SHAPE[1]], "FP32"))
OUTPUTS.append(grpcclient.InferRequestedOutput(OUTPUT_LAYER_NAME, class_count=3))
TRITON_CLIENT = grpcclient.InferenceServerClient(url=f"{TRITON_IP}:{TRITON_PORT}")
INPUTS[0].set_data_from_numpy(self.image_transform_onnx(img, 224))
results = TRITON_CLIENT.infer(model_name=MODEL_NAME, inputs=INPUTS, outputs=OUTPUTS, headers={})
output = np.squeeze(results.as_numpy(OUTPUT_LAYER_NAME))
#print(output)
lista = [x.decode('utf-8') for x in output.tolist()]
return lista
In this architecture, the NGINX, Flask, and Triton servers should be started at the beginning. Edit the serve file and add a line to start the Triton server.
Build a Docker image and push the image to Amazon ECR
The Docker file code looks as follows:
FROM nvcr.io/nvidia/tritonserver:22.04-py3
# Add arguments to achieve the version, python and url
ARG PYTHON=python3
ARG PYTHON_PIP=python3-pip
ARG PIP=pip3
ENV LANG=C.UTF-8
RUN apt-key adv --keyserver keyserver.ubuntu.com --recv-keys A4B469963BF863CC
&& apt-get update
&& apt-get install -y nginx
&& apt-get install -y libgl1-mesa-glx
&& apt-get clean
&& rm -rf /var/lib/apt/lists/*
RUN ${PIP} install -U --no-cache-dir
tritonclient[all]
torch
torchvision
pillow==9.1.1
scipy==1.8.1
transformers==4.20.1
opencv-python==4.6.0.66
flask
gunicorn
&&
ldconfig &&
apt-get clean &&
apt-get autoremove &&
rm -rf /var/lib/apt/lists/* /tmp/* ~/* &&
mkdir -p /opt/program/models/
COPY sm /opt/program
COPY model /opt/program/models
WORKDIR /opt/program
ENTRYPOINT ["python3", "serve"]
Install and configure the aws-cli
client with the following code:
sudo apt install awscli
sudo apt install git-all
aws configure
# # input AWS Access Key ID, AWS Secret Access Key, Default region name and Default output format
Run the following command to build the Docker image and push the image to Amazon Elastic Container Registry (Amazon ECR). Provide your Region and account ID.
aws ecr get-login-password --region <regionID> | docker login --username AWS --password-stdin <accountID>.dkr.ecr.<regionID>.amazonaws.com
docker build -t inference/mytriton .
docker tag inference/mytriton:latest <accountID>.dkr.ecr. <regionID>.amazonaws.com/inference/mytriton:latest
docker push <accountID>.dkr.ecr.<regionID>.amazonaws.com/inference/mytriton:latest
Create a SageMaker endpoint and test the endpoint
Now it’s time to verify the result. Launch a notebook instance with an ml.c5.xlarge instance from the SageMaker console, and create a notebook with the conda_python3
kernel. The following code snippet shows an example deployment of an inference endpoint. The source code is available in the GitHub repo.
role = get_execution_role()
sess = sage.Session()
account = sess.boto_session.client('sts').get_caller_identity()['Account']
region = sess.boto_session.region_name
image = '{}.dkr.ecr.{}.amazonaws.com/inference/mytriton:latest'.format(account, region)
model = sess.create_model(
name="mytriton", role=role, container_defs=image)
endpoint_cfg=sess.create_endpoint_config(
name="MYTRITONCFG",
model_name="mytriton",
initial_instance_count=1,
instance_type="ml.g4dn.xlarge"
)
endpoint=sess.create_endpoint(
endpoint_name="MyTritonEndpoint", config_name="MYTRITONCFG")
Wait about 3 minutes until the inference server is started to verify the result.
The following code is the ResNet client request:
## resnet client
runtime = boto3.Session().client('runtime.sagemaker')
img = cv2.imread('dog.jpg')
string_img = base64.b64encode(cv2.imencode('.jpg', img)[1]).decode()
payload = json.dumps({"modelname": "resnet","payload": {"img":string_img}})
endpoint="MyTritonEndpoint"
response = runtime.invoke_endpoint(EndpointName=endpoint,ContentType="application/json",Body=payload,Accept='application/json')
out=response['Body'].read()
res=eval(out)
print(res)
We get the following response:
{'modelname': 'resnet', 'result': ['11.250000:250:250:malamute, malemute, Alaskan malamute', '9.914062:249:249:Eskimo dog, husky', '9.906250:248:248:Saint Bernard, St Bernard']}
The following code is the YOLOv5 client request:
# yolov5 client
payload = json.dumps({"modelname": "yolov5","payload": {"img":string_img}})
endpoint="MyTritonEndpoint"
response = runtime.invoke_endpoint(EndpointName=endpoint,ContentType="application/json",Body=payload,Accept='application/json')
out=response['Body'].read()
res=eval(out)
print(str(out))
We get the following response:
b'{"modelname": "yolov5", "result": [[16, 0.9168673157691956, 111.92530059814453, 258.53240966796875, 262.0159606933594, 533.407958984375, 768, 576], [2, 0.6941519379615784, 392.20037841796875, 573.6005249023438, 142.55178833007812, 224.56454467773438, 768, 576], [1, 0.5813695788383484, 131.8942413330078, 473.7420654296875, 179.61459350585938, 427.0913391113281, 768, 576], [7, 0.5316226482391357, 392.82275390625, 572.4647216796875, 144.685546875, 223.052734375, 768, 576]]}'
The following code is the BERT client request:
# bert client
text="The world has [MASK] people."
payload = json.dumps({"modelname": "bert_base","payload": {"text":text}})
endpoint="MyTritonEndpoint"
response = runtime.invoke_endpoint(EndpointName=endpoint,ContentType="application/json",Body=payload,Accept='application/json')
out=response['Body'].read()
res=eval(out)
print(res)
We get the following response:
{'modelname': 'bert_base', 'result': [{'token': 'The world has many people.', 'score': 0.16609132289886475}, {'token': 'The world has no people.', 'score': 0.07334889471530914}, {'token': 'The world has few people.', 'score': 0.0617995485663414}, {'token': 'The world has two people.', 'score': 0.03924647718667984}, {'token': 'The world has its people.', 'score': 0.023465465754270554}]}
Here we see our architecture is working as expected.
Note that hosting an endpoint will incur some costs. Therefore, delete the endpoint after you complete the test:
runtime.delete_endpoint(EndpointName=endpoint)
Cost estimation
To estimate cost, assume that you have three models, but not all of them are long-running. You’re using one endpoint for each model, and the online time of each endpoint is different. Using ml.g4dn.xlarge as an example, the total cost is about $971.52/month. The following table lists the details.
Model Name |
Endpoint Running /Day |
Instance Type |
Cost/Month (us-east-1) |
ResNet |
24 hours |
ml.g4dn.xlarge |
0.736 * 24 * 30=$529.92 |
BERT |
8 hours |
ml.g4dn.xlarge |
0.736 * 8 * 30=$176.64 |
YOLOv5 |
12 hours |
ml.g4dn.xlarge |
0.736 * 12 * 30=$264.96 |
The following table shows the cost for sharing one endpoint for three models using the preceding architecture. The total cost is about $676.8/month. From this result, we can conclude that you can save 30% in costs while also having 24/7 service from your endpoint.
Model Name |
Endpoint Running /Day |
Instance Type |
Cost/Month (us-east-1) |
ResNet, YOLOv5, BERT |
24 hours |
ml.g4dn.2xlarge |
0.94 * 24 * 30 = $676.8 |
Summary
In this post, we introduced an improved architecture in which multiple models share one endpoint in SageMaker. Under some conditions, this solution can help you save costs and improve resource utilization. It is suitable for business scenarios with low concurrency and latency-insensitive requirements.
To learn more about SageMaker and AI/ML solutions, refer to Amazon SageMaker.
References
About the authors
Zheng Zhang is a Senior Specialist Solutions Architect in AWS, he focuses on helping customers accelerate model training, inference and deployment for machine learning solutions. He also has rich experience in large-scale distributed training, design AI/ML solutions.
Yinuo He is an AI/ML specialist in AWS. She has experiences in designing and developing machine learning based products to provide better user experiences. She now works to help customers succeed in their ML journey.
Read More