Targeted handling of three distinct types of “special events” dramatically reduces false-alarm rate.Read More
Your guide to AI/ML at AWS re:Invent 2022
AWS re:Invent season is upon us again! Just a few days to go until re:Invent takes place for the 11th year in Las Vegas, Nevada. The Artificial Intelligence and Machine Learning team at AWS has been working hard to offer amazing content, an outstanding AWS DeepRacer experience, and much more. In this post, we give you a sense of how the AI/ML track is organized and highlight a few sessions we think you’ll like.
The technical sessions in the AI/ML track are divided into four areas. First, there are many common use cases that you can address with a combination of AI/ML and other AWS services, such as Intelligent Document Processing, Contact Center Intelligence, and Personalization among others. Second, ML practitioners of all levels will find compelling content on the entire ML lifecycle, such as data preparation, training, inference, MLOps, AutoML, and no-code ML. This year, we have a renewed emphasis on responsible AI. Customers have been looking for more guidance and new tools in this space. And last but never least, we have exciting workshops and activities with AWS DeepRacer—they have become a signature event!
Visit the AWS Village at the Venetian Expo Hall to meet our AI/ML specialists at the AI/ML booth and learn more about AI/ML services and solutions. You can also chat with our AWS Manufacturing experts at the AWS Industries Networking Lounge, in the Caesars Forum Main Hall.
If you’re new to re:Invent, you can attend sessions of the following types:
- Keynotes – Join in-person or virtual, and learn about all the exciting announcements.
- Leadership sessions – Learn from AWS leaders about key topics in cloud computing.
- Breakout sessions – These 60-minute sessions are expected to have broad appeal, are delivered to larger audiences, and will be recorded. If you miss them, you can watch them on demand after re:Invent.
- Chalk talks – 60 minutes of content delivered to smaller audiences with an interactive whiteboarding session. Chalk talks are where discussions happen, and these offer you the greatest opportunity to ask questions or share your opinion.
- Workshops – Hands-on learning opportunities where, in the course of 2 hours, you’ll be able to build a solution to a problem, understand the inner workings of the resulting infrastructure, and cross-service interaction. Bring your laptop and be ready to learn!
- Builders’ sessions – These highly interactive 60-minute mini-workshops are conducted in small groups of less than 10 attendees. Some of these appeal to beginners, and others are on specialized topics.
If you have reserved your seat at any of the sessions, great! If not, we always set aside some spots for walk-ins, so make a plan and come to the room early.
To help you plan your agenda for this year’s re:Invent, here are some highlights of the AI/ML track. So buckle up, and start registering for your favorite sessions.
Visit the session catalog to learn about all AI/ML sessions.
AWS Data and Machine Learning Keynote
Swami Sivasubramanian, Vice President of AWS Data and Machine Learning – Keynote
Wednesday November 30 | 8:30 AM – 10:30 AM PST | The Venetian
Join Swami Sivasubramanian, Vice President of AWS Data and Machine Learning on Wednesday, as he reveals the latest AWS innovations that can help you transform your company’s data into meaningful insights and actions for your business, in person or via livestream.
AI/ML Leadership session
AIM217-L (LVL 200) Innovate with AI/ML to transform your business
Wednesday November 30 | 1:00 PM – 2:00 PM PST
Join Dr. Bratin Saha, VP of AI/ML at AWS, for the AI/ML thought-leadership session. Bratin will share how to use AI/ML to innovate in your business in order to disrupt the status quo. You learn how customers Baxter, BMW, and Alexa have used AWS AI/ML services to fuel business profitability and growth, the latest AI/ML trends, and the details of newly launched AWS capabilities.
Breakout sessions
AIM314 (LVL 300) Accelerate your ML journey with Amazon SageMaker low-code tools
Monday November 28 | 10:00 AM – 11:00 AM PST
In this session, learn how low-code tools, including Amazon SageMaker Data Wrangler, Amazon SageMaker Autopilot, and Amazon SageMaker JumpStart, make it easier to experiment faster and bring highly accurate models to production more quickly and efficiently.
AIM204 (LVL 200) Automate insurance document processing with AI
Monday November 28 | 4:00 PM – 5:00 PM PST
The rapid rate of data generation means that organizations that aren’t investing in document automation risk getting stuck with legacy processes that are slow, error-prone, and difficult to scale. In this session, learn how organizations can take advantage of the latest innovations in AI and ML from AWS to improve the efficiency of their document-intensive claims processing use case.
AIM207 (LVL 200) Make better decisions with no-code ML using SageMaker Canvas, feat. Samsung
Wednesday November 30 | 2:30 PM – 3:30 PM PSTOrganizations everywhere use ML to accurately predict outcomes and make faster business decisions. In this session, learn how you can use Amazon SageMaker Canvas to access and combine data from a variety of sources, clean data, build ML models to generate predictions with a single click, and share models across your organization to improve productivity.
AIM307 (LVL 300) JPMorganChase real-time agent assist for contact center productivity
Wednesday November 30 | 11:30 AM – 12:30 PM PST
Resolving complex customer issues is often time-consuming and requires agents to quickly gather relevant information from knowledge bases to resolve queries accurately. Join this session to learn how JPMorgan Chase built an AWS Contact Center Intelligence (CCI) real-time agent assist solution to help 75 million customers and help 8,500 servicing agents generate next best actions in the shortest time—reducing agent frustration and churn.
AIM321 (LVL 300) Productionize ML workloads using Amazon SageMaker MLOps, feat. NatWest
Wednesday November 30 | 4:45 PM – 5:45 PM PST
Amazon SageMaker provides a breadth of MLOps tools to train, test, troubleshoot, deploy, and govern ML models at scale. In this session, explore SageMaker MLOps features, including SageMaker Pipelines, SageMaker Projects, SageMaker Experiments, SageMaker Model Registry, and SageMaker Model Monitor, and learn how to increase automation and improve the quality of your ML workflows.
AIM319 (LVL 300) Build, manage, and scale ML development with a web-based visual interface
Wednesday November 30 | 3:15 PM – 4:15 PM PST
Amazon SageMaker Studio is an integrated development environment (IDE) for data science and ML. In this session, explore how to use SageMakerStudio to prepare data and build, train, deploy, and manage ML models from a single, web-based visual interface.
Chalk talks
AIM341-R (LVL 300) Transforming responsible AI from theory into practice
Thursday December 1 | 4:15 PM – 5:15 PM PST
The practices of responsible AI can help reduce biased outcomes from models and improve their fairness, explainability, robustness, privacy, and transparency. Walk away from this chalk talk with best practices and hands-on support to guide you in applying responsible AI in your project.
*This chalk talk will be repeated Wednesday November 30 | 7:00 PM – 8:00 PM PST
AIM306-R (LVL 300) Automate content moderation and compliance with AI
Monday November 28 | 12:15 PM – 1:15 PM PST
In this chalk talk, learn how to efficiently moderate high volumes of user-generated content across media types with AI. Discover how to add humans in the moderation loop to verify low-confidence decisions and continuously improve ML models to keep online communities safe and inclusive and lower content moderation costs.
*This chalk talk will be repeated Wednesday November 30 | 9:15 AM – 10:15 AM PST
AIM407-R (LVL 400) Choosing the right ML instance for training and inference on AWS
Wednesday November 30 | 11:30 AM – 12:30 PM PST
This chalk talk guides you through how to choose the right compute instance type on AWS for your deep learning projects. Explore the available options, such as the most performant instance for training, the best instance for prototyping, and the most cost-effective instance for inference deployments.
*This chalk talk will be repeated Wednesday November 30 | 8:30 AM – 9:30 AM PST
AIM328-R (LVL 300) Explain your ML models with Amazon SageMaker Clarify
Tuesday November 29 | 2:00 PM – 3:00 PM PST
Amazon SageMaker Clarify helps organizations understand their model predictions by providing real-time explanations for models deployed on SageMaker endpoints. In this chalk talk, learn how to identify the importance of various features in overall model predictions and for individual inferences using Shapley values and detect any shifts in feature importance over time after a model is deployed to production.
*This chalk talk will be repeated Monday November 28 | 2:30 PM – 3:30 PM PST
Workshops
AIM342 (LVL 300) Advancing responsible AI: Bias assessment and transparency
Wednesday November 30 | 2:30 PM – 4:30 PM PST
Building and operating ML applications responsibly requires an active, consistent approach to prevent, assess, and mitigate bias. This workshop takes you through a computer vision case study in assessing unwanted bias—follow along during the workshop with a Jupyter notebook.
AIM402-R (LVL 400) Extract AI-driven customer insights using Post-Call Analytics
Monday November 28 | 4:00 PM – 6:00 PM PST
Companies are transforming existing contact centers by adding AI/ML to deliver actionable insights and improve automation with existing telephony systems. Join this workshop to learn how to use the AWS Contact Center Intelligence (CCI) Post-Call Analytics solution to derive AI-driven insights from virtually all customer conversations.
*This workshop will be repeated Wednesday November 30 | 9:15 AM – 11:15 AM PST
AIM212-R (LVL 200) Deep learning with Amazon SageMaker, AWS Trainium, and AWS Inferentia
Monday November 28 | 1:00 PM – 3:00 PM PST
Amazon EC2 Trn1 instances, powered by AWS Trainium, and Amazon EC2 Inf1 instances, powered by AWS Inferentia, deliver the best price performance for deep learning training and inference. In this workshop, walk through training a BERT model for natural language processing on Trn1 instances to save up to 50% in training costs over equivalent GPU-based EC2 instances.
*This workshop will be repeated Monday November 28 | 8:30 AM – 10:30 AM PST
AIM312-R (LVL 300) Build a custom recommendation engine in 2 hours with Amazon Personalize
Monday November 28 | 1:00 PM – 3:00 PM PST
In this workshop, learn how to build a customer-specific solution using your own data to deliver personalized experiences that can be integrated into your existing websites, applications, SMS, and email marketing systems using simple APIs.
*This workshop will be repeated Wednesday November 30 | 11:30 AM – 1:30 PM PST
Builders’ sessions
AIM325-R (LVL 300) Build applications faster with an ML-powered coding companion
Tuesday November 29 | 3:30 PM – 4:30 PM PST
Join this builders’ session to get hands-on experience with ML-powered developer tools from AWS. Learn how to accelerate application development with automatic code recommendations from Amazon CodeWhisperer and automate code reviews with Amazon CodeGuru.
*This session will be repeated Thursday December 1 | 12:30 PM – 1:30 PM PST
Make sure to check out the re:Invent content catalog or the AI/ML attendee guide for more AI/ML content at re:Invent.
AWS DeepRacer: Get hands-on with machine learning
Developers of all skill levels can get hands-on with ML at re:Invent by participating in AWS DeepRacer. Learn to build your own ML model from AWS ML experts in one of 11 workshop sessions, featuring guest speakers from JPMorgan Chase and Intel. Compete by racing your own ML model on real championship tracks in both the MGM and the Sands Expo, or hop in the driver’s seat to experience ML fundamentals through the fun of gamified learning with AWS DeepRacer Arcades. Whether in the classroom, on the track, or behind the wheel, AWS DeepRacer is the fastest way to get rolling with ML.
Developers: start your engines! Starting Monday November 28, the top 50 racers from around the world compete in the AWS DeepRacer League Championships presented by Intel, hosted at the AWS DeepRacer Championship Stadium in the Sands Expo. Watch trackside or tune in live on twitch.tv/aws at 3:00 PM PST on Tuesday, November 29, to see the top eight racers battle it out in the semifinals. Cheer on the finalists as they go for their shot at $20,000 in cash prizes and the right to hoist the Championship Cup.
Race on any AWS DeepRacer track on Thursday, December 1, to compete in the 2023 re:Invent Open, where the fastest competitor of the day will claim an all-expenses paid trip back to Vegas to compete in the 2023 AWS DeepRacer Championship Cup.
Attendees who participate in AWS DeepRacer Arcades or open track (non-competitive) racing will also have the chance to win one of six spots in the AWS DeepRacer Winner’s Circle Driving Experience Sweepstakes, where they will race real, full-size exotic cars on a closed track alongside the AWS DeepRacer 2022 Champions in Las Vegas.
Don’t forget to check out the AWS DeepRacer workshops before they fill up to reserve your spot. We can’t wait to see you in Las Vegas!
About the authors
Denis V. Batalov is a 17-year Amazon veteran and a PhD in Machine Learning, Denis worked on such exciting projects as Search Inside the Book, Amazon Mobile apps and Kindle Direct Publishing. Since 2013 he has helped AWS customers adopt AI/ML technology as a Solutions Architect. Currently, Denis is a Worldwide Tech Leader for AI/ML responsible for the functioning of AWS ML Specialist Solutions Architects globally. Denis is a frequent public speaker, you can follow him on Twitter @dbatalov.
Amelie Perkuhn is a Product Marketing Manager on the AI Services team at AWS. She has held various roles within AWS over the past 6 years, and in her current role, she is focused on driving adoption of AI Services including Amazon Kendra. In her spare time, Amelie enjoys the Pacific Northwest with her dog Moxie.
Amazon and UCLA announce fellowship recipients
The UCLA Science Hub fellows fulfill the hub’s mission of researching the societal impact of artificial intelligence.Read More
See a Sea Change: 3D Researchers Bring Naval History to Life
Museumgoers will be able to explore two sunken WWII ships as if they were scuba divers on the ocean floor, thanks to work at Curtin University in Perth, Australia.
Exhibits in development, for display in Australia and potentially further afield, will use exquisitely detailed 3D models the researchers are creating to tell the story of one of the nation’s greatest naval battles.
On Nov. 19, 1941, Australia’s HMAS Sydney (II) and Germany’s HSK Kormoran lobbed hundreds of shells in a duel that lasted less than an hour. More than 700 died, including every sailor on the Sydney. Both ships sank 8,000 feet, 130 miles off the coast of Western Australia, not to be discovered for decades.
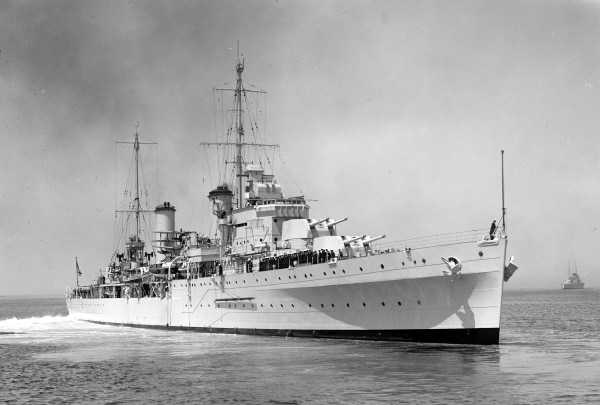
Andrew Woods, an expert in stereoscopic 3D visualization and associate professor at Curtin, built an underwater rig with more than a dozen video and still cameras to capture details of the wrecks in 2015.
Ash Doshi, a computer vision specialist and senior research officer at Curtin, is developing and running software on NVIDIA GPUs that stitches the half-million pictures and 300 hours of video they took into virtual and printed 3D models.
3D at Battleship Scale
It’s hard, pioneering work in a process called photogrammetry. Commercially available software maxes out at around 10,000 images.
“It’s highly computationally intensive — when you double the number of images, you quadruple the compute requirements,” said Woods, who manages the Curtin HIVE, a lab with four advanced visualization systems.
“It would’ve taken a thousand years to process with our existing systems, even though they are fairly fast,” he said.
When completed next year, the work will have taken less than three years, thanks to systems at the nearby Pawsey Supercomputing Centre using NVIDIA V100 and prior-generation GPUs.
Speed Enables Iteration
Accelerated computing is critical because the work is iterative. Images must be processed, manipulated and then reprocessed.
For example, Woods said a first pass on a batch of 400 images would take 10 hours on his laptop. By contrast, he could run a first pass in 10 minutes on his system with two NVIDIA RTX A6000 GPUs awarded through NVIDIA’s Applied Research Accelerator Program.
It would take a month to process 8,000 images on the lab’s fast PCs, work the supercomputer could handle in a day. “Rarely would anyone in industry wait a month to process a dataset,” said Woods.
From Films to VR
Local curators can’t wait to get the Sydney and Kormoran models on display. Half the comments on their Tripadvisor page already celebrate 3D films the team took of the wrecks.
The digital models will more deeply engage museumgoers with interactive virtual and augmented reality exhibits and large-scale 3D prints.
“These 3D models really help us unravel the story, so people can appreciate the history,” Woods said.
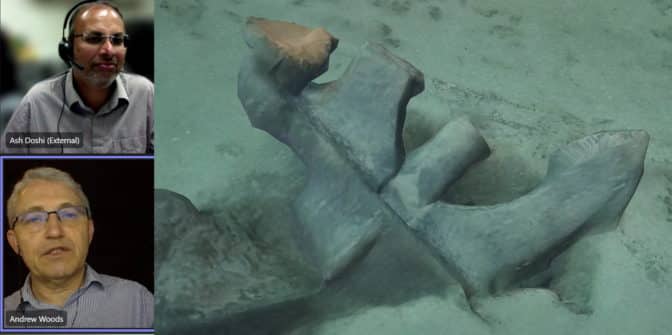
The exhibits are expected to tour museums in Perth and Sydney, and potentially cities in Germany and the U.K., where the ships were built.
When the project is complete, the researchers aim to make their code available so others can turn historic artifacts on the seabed into rare museum pieces. Woods expects the software could also find commercial uses monitoring undersea pipelines, oil and gas rigs and more.
A Real-Time Tool
On the horizon, the researchers want to try Instant NeRF, an inverse rendering tool NVIDIA researchers developed to turn 2D images into 3D models in real time.
Woods imagines using it on future shipwreck surveys, possibly running on an NVIDIA DGX System on the survey vessel. It could provide previews in near real time based on images gathered by remotely operated underwater vehicles on the ocean floor, letting the team know when it has enough data to take back for processing on a supercomputer.
“We really don’t want to return to base to find we’ve missed a spot,” said Woods.
Woods’ passion for 3D has its roots in the sea.
“I saw the movie Jaws 3D when I was a teenager, and the images of sharks exploding out of the screen are in part responsible for taking me down this path,” he said.
The researchers released the video below to commemorate the 81st anniversary of the sinking of the WWII ships.
The post See a Sea Change: 3D Researchers Bring Naval History to Life appeared first on NVIDIA Blog.
Optimizing Production PyTorch Models’ Performance with Graph Transformations
1. Introduction
PyTorch supports two execution modes [1]: eager mode and graph mode. In eager mode, operators in a model are immediately executed as they are encountered. In contrast, in graph mode, operators are first synthesized into a graph, which will then be compiled and executed as a whole. Eager mode is easier to use, more suitable for ML researchers, and hence is the default mode of execution. On the other hand, graph mode typically delivers higher performance and hence is heavily used in production.
Specifically, graph mode enables operator fusion [2], wherein one operator is merged with another to reduce/localize memory reads as well as total kernel launch overhead. Fusion can be horizontal—taking a single operation (e.g., BatchNorm) that is independently applied to many operands and merging those operands into an array; and vertical—merging a kernel with another kernel that consumes the output of the first kernel (e.g., Convolution followed by ReLU).
Torch.FX [3, 4] (abbreviated as FX) is a publicly available toolkit as part of the PyTorch package that supports graph mode execution. In particular, it (1) captures the graph from a PyTorch program and (2) allows developers to write transformations on the captured graph. It is used inside Meta to optimize the training throughput of production models. By introducing a number of FX-based optimizations developed at Meta, we demonstrate the approach of using graph transformation to optimize PyTorch’s performance for production.
2. Background
Embedding tables are ubiquitous in recommendation systems. Section 3 will discuss three FX transformations that optimize accesses to embedding tables. In this section, we provide some background on FX (Section 2.1) and embedding tables (Section 2.2).
2.1 FX
Figure 1 is a simple example adopted from [3] which illustrates using FX to transform a PyTorch program. It contains three steps: (1) capturing the graph from a program, (2) modifying the graph (in this example, all uses of RELU are replaced by GELU), and (3) generating a new program from the modified graph.
Figure 1: A FX example which replaces all uses of RELU by GELU in a PyTorch module.
The FX API [4] provides many more functionalities for inspecting and transforming PyTorch program graphs.
2.2 Embedding Tables
Figure 2: Illustration of an embedding table for a sparse feature with batch size = 1
In a recommendation system, sparse features (e.g., User ID, Story ID) are represented by embedding tables. An embedding table E is an HxD matrix, where H is the hash size, D is the embedding dimension. Each row of E is a vector of floats. Feature hashing [5] is used to map a sparse feature to a list of indices to E, say [S1,S2, …, Sk], where 0<=Si<H. Its output value is computed as f(E[S1], E[S2], …, E[Sk]), where E[Si] is the vector at row Si, and f is called the pooling function, which is typically one of the following functions: sum, average, maximum. See Figure 2 for an illustration.
To fully utilize the GPU, sparse features are usually processed in a batch. Each entity in a batch has its own list of indices. If a batch has B entities, a naive representation has B lists of indices. A more compact representation is to combine the B lists of indices into a single list of indices and add a list of the lengths of indices (one length for each entity in the batch). For example, if a batch has 3 entities whose lists of indices are as follows:
- Entity 1: indices = [10, 20]
- Entity 2: indices = [5, 9, 77, 81]
- Entity 3: indices = [15, 20, 45]
Then the indices and lengths for the entire batch will be:
- Indices = [10, 20, 5, 9, 77, 81, 15, 20, 45]
- Lengths = [2, 4, 3]
And the output of the embedding table lookup for the whole batch is a BxD matrix.
3. Three FX Transformations
We have developed three FX transformations that accelerate accesses to embedding tables. Section 3.1 discusses a transformation that combines multiple small input tensors into a single big tensor; Section 3.2 a transformation that fuses multiple, parallel compute chains into a single compute chain; and Section 3.3 a transformation that overlaps communication with computation.
3.1 Combining Input Sparse Features
Recall that an input sparse feature in a batch is represented by two lists: a list of indices and a list of B lengths, where B is the batch size. In PyTorch, these two lists are implemented as two tensors. When a PyTorch model is run on a GPU, embedding tables are commonly stored in the GPU memory (which is closer to the GPU and has much higher read/write bandwidth than the CPU memory). To use an input sparse feature, its two tensors need to be first copied from CPU to GPU. Nevertheless, per host-to-device memory copying requires a kernel launch, which is relatively expensive compared to the actual data transfer time. If a model uses many input sparse features, this copying could become a performance bottleneck (e.g., 1000 input sparse features would require copying 2000 tensors from host to device).
An optimization that reduces the number of host-to-device memcpy is to combine multiple input sparse features before sending them to the device. For instance, given the following three input features:
- Feature_A: indices = [106, 211, 7], lengths = [2, 1]
- Feature_B: indices = [52, 498, 616, 870, 1013], lengths = [3, 2]
- Feature_C: indices = [2011, 19, 351, 790], lengths = [1, 3]
The combined form is:
- Features_A_B_C: indices = [106, 211, 7, 52, 498, 616, 870, 1013, 2011, 19, 351, 790], lengths = [2, 1, 3, 2, 1, 3]
So, instead of copying 3×2=6 tensors from host to device, we only need to copy 2 tensors.
Figure 3(b) describes an implementation of this optimization, which has two components:
- On the CPU side: The input pipeline is modified to combine all the indices of sparse features into a single tensor and similarly all the lengths into another tensor. Then the two tensors are copied to the GPU.
- On the GPU side: Using FX, we insert a Permute_and_Split op into the model graph to recover the indices and lengths tensors of individual features from the combined tensors, and route them to the corresponding nodes downstream.
(a). Without the optimization
(b). With the optimization
Figure 3: Combining input sparse features
3.2 Horizontal fusion of computation chains started with accesses to embedding tables
In a production model, it is fairly common to have 10s of embedding tables residing on each GPU. For performance reasons, lookups to these tables are grouped together so that their outputs are concatenated in a single big tensor (see the red part in Figure 4(a)). To apply computations to individual feature outputs, a Split op is used to divide the big tensors into N smaller tensors (where N is the number of features) and then the desired computations are applied to each tensor. This is shown in Figure 4(a), where the computation applied to each feature output O is Tanh(LayerNorm(O)). All the computation results are concatenated back to a big tensor, which is then passed to downstream ops (Op1 in Figure 4(a)).
The main runtime cost here is the GPU kernel launch overhead. For instance, the number of GPU kernel launches in Figure 4(a) is 2*N + 3 (each oval in the figure is a GPU kernel). This could become a performance issue because execution times of LayerNorm and Tanh on the GPU are short compared to their kernel launch times. In addition, the Split op may create an extra copy of the embedding output tensor, consuming additional GPU memory.
We use FX to implement an optimization called horizontal fusion which dramatically reduces the number of GPU kernel launches (in this example, the optimized number of GPU kernel launches is 5, see Figure 4(b)). Instead of doing an explicit Split, we use the Add_middle_dim op to reshape the 2D embedding tensor of shape (B, NxD) to a 3D tensor of shape (B, N, D). Then a single LayerNorm is applied to the last dimension of it. Then a single Tanh is applied to the result of the LayerNorm. At the end, we use the Remove_middle_dim op to reshape the Tanh’s result back to a 2D tensor. In addition, since Add_middle_dim and Remove_middle_dim only reshape the tensor without creating an extra copy, the amount of GPU memory consumption could be reduced as well.
(a). Without the optimization
(b). With the optimization
Figure 4: Horizontal fusion
3.3 Overlapping Computation with Communication
Training of a production recommendation model is typically done on a distributed GPU system. Since the capacity of the device memory per GPU is not big enough to hold all the embedding tables in the model, they need to be distributed among the GPUs.
Within a training step, a GPU needs to read/write feature values from/to the embedding tables on the other GPUs. This is known as all-to-all communication [6] and can be a major performance bottleneck.
We use FX to implement a transformation that can overlap computation with all-to-all communication. Figure 5(a) shows the example of a model graph which has embedding table accesses (EmbeddingAllToAll) and other ops. Without any optimization, they are sequentially executed on a GPU stream, as shown in Figure 5(b). Using FX, we break EmbeddingAllToAll into EmbeddingAllToAll_Request and EmbeddingAllToAll_Wait, and schedule independent ops in between them.
(a) Model graph
(b) Original execution order
(c)Optimized execution order
Figure 5: Overlapping Computation with Communication
3.4 Summary
Table 1 summarizes the optimizations discussed in this section and the corresponding performance bottlenecks addressed.
Optimization | Performance Bottleneck Addressed |
Combining Input Sparse Features | Host-to-device memory copy |
Horizontal fusion | GPU kernel launch overhead |
Overlapping Computation with Communication | Embedding all-to-all access time |
Table 1: Summary of the optimizations and the performance bottlenecks addressed
We have also developed other FX transformations which are not discussed in this section due to space limitations.
To discover which models would benefit from these transformations, we analyzed the performance data collected by MAIProf [7] from the models that run at Meta’s data centers. Altogether, these transformations provide up to 2-3x of speedups compared to eager mode on a set of production models.
4. Concluding Remarks
The graph mode in PyTorch is preferred over the eager mode for production use for performance reasons. FX is a powerful tool for capturing and optimizing the graph of a PyTorch program. We demonstrate three FX transformations that are used to optimize production recommendation models inside Meta. We hope that this blog can motivate other PyTorch model developers to use graph transformations to boost their models’ performance.
References
[1] End-to-end Machine Learning Framework [2] DNNFusion: Accelerating Deep Neural Networks Execution with Advanced Operator Fusion [3] Torch.FX: Practical Program Capture and Transformation for Deep Learning In Python, MLSys 2022. [4] Torch.fx—PyTorch 1.12 documentation [5] Feature Hashing for Large Scale Multitask Learning [6] NVIDIA Collective Communication Library Documentation [7] Performance Debugging of Production PyTorch Models at MetaAlexaTM 20B is now available in Amazon SageMaker JumpStart
Today, we announce the public availability of Amazon’s state-of-the-art Alexa Teacher Model with 20 billion parameters (AlexaTM 20B) through Amazon SageMaker JumpStart, SageMaker’s machine learning hub. AlexaTM 20B is a multilingual large-scale sequence-to-sequence (seq2seq) language model developed by Amazon. You can use AlexaTM 20B for a wide range of industry use-cases, from summarizing financial reports to question answering for customer service chatbots. It can be applied even when there are only a few available training examples, or even none at all. AlexaTM 20B outperforms a 175 billion GPT-3 model on zero-shot learning tasks such as SuperGLUE and shows state-of-the-art performance for multilingual zero-shot tasks such as XNLI.
In this post, we provide an overview of how to deploy and run inference with the AlexaTM 20B model programmatically through JumpStart APIs, available in the SageMaker Python SDK. We exemplify how you can use this model to translate between multiple languages, summarize long-form text, answer questions based on a given context and generate text that appears indistinguishable from human-written text.
AlexaTM 20B and in-context learning
The Alexa Teacher Model (AlexaTM) program by Amazon Alexa AI is designed to build large-scale, multilingual deep learning models (primarily Transformer-based), aiming to improve generalization and handling data scarcity for downstream tasks. With large-scale pre-training, teacher models can generalize well to learn new tasks from sparse data and help developers improve performance on downstream tasks. AlexaTM 20B has shown competitive performance on common natural language processing (NLP) benchmarks and tasks, such as machine translation, data generation and summarization.
Using foundation models such as AlexaTM 20B reduces the need for expensive model pre-training and provides a state-of-the-art starting point to develop task models with less effort and less task-specific training data. One of the key abilities of foundation models is that we can teach a model to perform new tasks such as question and answering in different languages, with very small amounts of input examples and no fine-tuning or gradient updates required. This is known as in-context learning. With only a few examples of a new task provided as context for inference, the AlexaTM 20B model can transfer knowledge from what has been learned during large-scale pre-training, even across languages. This is called few-shot learning. In some cases, the model can perform well without any training data at all, with only an explanation of what should be predicted. This is called zero-shot learning. For example, let’s say we are using AlexaTM 20B for one-shot natural language generation. The input passed to the model is the training example in the form of attribute-value pairs, along with its corresponding output text narrative. The test example is then appended to form the full input prompt, as shown in the following figure.
To learn more about the model, check out 20B-parameter Alexa model sets new marks in few-shot learning or the original paper.
Use of AlexaTM 20B is made available for non-commercial use and is covered under the Alexa Teacher Model License agreement.
Solution overview
The following sections provide a step-by-step demo on how to deploy the model, run inference, and do in-context-learning to solve few-shot learning tasks.
Note that the following section contains code snippets; the full code with all the steps in this demo is available in the accompanying notebook: In-context-learning with AlexaTM 20B in SageMaker JumpStart.
Deploy the model
To use a large language model in SageMaker, you need an inferencing script specific for the model, which includes steps like model loading, parallelization and more. You also need to create end-to-end tests for scripts, model and the desired instance types to validate that all three can work together. JumpStart removes this effort by providing ready-to-use scripts that have been robustly tested.
SageMaker gives you the ability to run Docker containers extensively for training and inferencing. JumpStart uses these available framework-specific SageMaker Deep Learning Containers (DLCs). We start by fetching the optimized DLC (deploy_image_uri
) using the model_id
. Then we fetch the model_uri
containing the model parameters, along with inference handling scripts and any associated dependencies. Next, we create a model instance in SageMaker and deploy it to a real-time endpoint. See the following code:
Deploying AlexaTM 20B requires a GPU-backed instance with at least 50 GB of CPU memory and at least 42 GB of GPU memory. SageMaker provides many such instances that support real-time inference. We tested this solution on three instances: ml.g4dn.12xlarge, ml.p3.8xlarge, ml.p3.16xlarge. See the following code:
Next, we deploy the model to a SageMaker real-time endpoint:
AlexaTM 20B requires 40 GB of disk space in the inference container. An ml.g4dn.12xlarge instance fulfills this requirement. For instance types ml.p3.8xlarge and ml.p3.16xlarge, we attach an Amazon Elastic Block Store (Amazon EBS) volume to handle the large model size. Therefore, we set volume_size = None
when deploying on ml.g4dn.12xlarge and volume_size=256
when deploying on ml.p3.8xlarge or ml.p3.16xlarge.
Deploying the model may take up to 10 minutes. After the model is deployed, we can get predictions from it in real time!
Run inference
AlexaTM 20B is a text generation model which, given a partial sequence (a sentence or piece of text), generates the next set of words. The following code snippet gives you a glimpse of how to query the endpoint we deployed and parse the outputs for auto-completion task. To send requests to a deployed model, we use a JSON dictionary encoded in UTF-8 format. The endpoint response is a JSON object containing a list of generated texts.
Next, we query the endpoint and parse the response on a sample input text:
AlexaTM 20B currently supports 10 text generation parameters during inference: max_length
, num_return_sequences
, num_beams
, no_repeat_ngram_size
, temperature
, early_stopping
, do_sample
, top_k
, top_p
, and seed
. For detailed information on valid values for each parameter and their impact on the output, see the accompanying notebook: In-context-learning with AlexaTM 20B in SageMaker JumpStart.
In-context learning
In-context learning refers to the following: we provide the language model with a prompt, which consists of training input-output pairs that demonstrate the task. We append a test input to the prompt and allow the language model to make predictions by conditioning on the prompt and predicting the next tokens or words. This is a highly effective technique to solve few shot-learning problems, in which we learn a task from a few training samples.
Next, we show how you can use AlexaTM 20B for several 1-shot and zero-shot tasks via in-context learning. Unlike prior sequence-to-sequence models, AlexaTM 20B was trained on causal language modeling in addition to denoising, which makes it a good model for in-context learning.
1-shot text summarization
Text summarization is the task of shortening the data and creating a summary that represents the most important information present in the original text. 1-shot text summarization refers to the setting where we learn to summarize the text based on a single training sample. The following code is a text summarization sample from the XSUM dataset:
We use the following prompt for summarization when only one training sample is provided. The generated text from the model is interpreted as the predicted summary of the test article.
The output is as follows:
1-shot natural language generation
Natural language generation is the task of producing text narratives given the input text. The following sample shows a training sample from the E2E dataset:
We use the following prompt for natural language generation when only one training sample (1-shot) is provided. The generated text from the model is interpreted as the predicted text narrative for the test input (test_inp
).
The output is as follows:
1-shot machine translation
Machine translation is the task of translating text from one language to another. The following example shows a training sample from the WMT19 dataset in which we need to translate from German to English:
We use the following prompt for machine translation when only one training sample (1-shot) is provided. Generated text from the model is interpreted as the translation of the test input (test_inp
).
The output is as follows:
Zero-shot extractive question answering
Extractive question answering is the task of finding the answer to a question from the context paragraph. The following is an example of a context and a question from the SQuAD v2 dataset:
Note that we don’t have any training samples for our task. Instead, we create a dummy question about the last word in the prompt , based on the test_context
(dummy-shot). Therefore, we’re actually doing zero-shot extractive question answering.
We use the following prompt for extractive question answering when no training sample is provided. Generated text from the model is interpreted as the answer to the test question.
The output is as follows:
Prompt Engineering
Prompt engineering can sometimes be an art. Even small changes to the prompt template can result in significant changes to the model’s performance on a specific task. The following are a few pieces of advice for writing good prompt templates. First, it’s important to remember that the model was trained to learn the structure of real sentences (causal language modeling). As such, it’s best to ensure that your prompt template is grammatically and structurally correct in natural language. Second, this particular model benefits from dummy shots to help teach it the structure expected in the answer, as demonstrated above. Third, it’s always advised to examine task performance over a variety of candidate prompt templates. Promptsource and Natural Instructions are two open-source frameworks for standardizing prompt templates, and they provide a variety of example prompts used for existing modeling tasks. Additionally, Appendix B of the AlexaTM 20B paper provides the prompt templates used to generate the results presented in the paper. There is a growing sub-field dedicated to the automatic creation and learning of the best prompts for a task, including both natural language and continuous prompts. This is beyond the scope of this tutorial.
Conclusion
In this post, we showed how to deploy the AlexaTM 20B model on a SageMaker endpoint and run inference. You can use the AlexaTM 20B model for in-context-learning for a variety of few-shot learning tasks. To learn more about AlexaTM 20B, refer to 20B-parameter Alexa model sets new marks in few-shot learning or the original paper.
The authors would like to acknowledge the technical contributions of Maciej Rudnicki, Jakub Debski, Ashish Khetan, Anastasiia Dubinina, Vitaliy Korolev, Karl Albertsen, Saleh Soltan, and Mariusz Momotko toward making this launch possible.
About JumpStart
JumpStart is the machine learning (ML) hub of Amazon SageMaker that offers over 350 pre-trained models, built-in algorithms, and pre-built solution templates to help you get started with ML fast. JumpStart hosts state-of-the-art models from popular model hubs such as TensorFlow, PyTorch, Hugging Face, and MXNet, which support popular ML tasks such as object detection, text classification, and text generation. The ML research community has put a large amount of effort into making a majority of recently developed models publicly available for use. JumpStart aims to help you find right the ML models and algorithms, and immediately start building models. Specifically, JumpStart provides the following benefits:
- Easy access with the UI and SDK – You can access models and algorithms in JumpStart programmatically using the SageMaker Python SDK or through the JumpStart UI in Amazon SageMaker Studio. Currently, AlexaTM 20B is only accessible through the SageMaker Python SDK.
- SageMaker built-in algorithms – JumpStart provides over 350 built-in algorithms and pre-trained models, along with corresponding training scripts (if supported), inferencing scripts, and example notebooks. Scripts are optimized for each framework and task, and provide features such as GPU support, automatic model tuning and incremental training. Scripts are also tested against SageMaker instances and features so that you don’t run into compatibility issues.
- Pre-built solutions – JumpStart provides a set of 23 solutions for common ML use cases, such as demand forecasting and industrial and financial applications, which you can deploy with just a few clicks. Solutions are end-to-end ML applications that string together various AWS services to solve a particular business use case. They use AWS CloudFormation templates and reference architectures for quick deployment, which means they’re fully customizable.
- Support – SageMaker provides a range of support, such as maintaining up-to-date versions when new SageMaker features or Deep Learning Container versions are released, and creating documentation on how to use JumpStart contents in a SageMaker environment.
To learn more about JumpStart and how you can use open-source pre-trained models for a variety of other ML tasks, check out the following AWS re:Invent 2020 video.
About the Authors
Dr. Vivek Madan is an Applied Scientist with the Amazon SageMaker JumpStart team. He got his PhD from University of Illinois at Urbana-Champaign and was a Post Doctoral Researcher at Georgia Tech. He is an active researcher in machine learning and algorithm design and has published papers in EMNLP, ICLR, COLT, FOCS, and SODA conferences.
Jack FitzGerald is a senior applied scientist with Alexa AI, where he currently focuses on large language modeling, multilingual text modeling, and machine learning operations.
João Moura is an AI/ML Specialist Solutions Architect at Amazon Web Services. He is mostly focused on NLP use cases and helping customers optimize deep learning model training and deployment. He is also an active proponent of low-code ML solutions and ML-specialized hardware.
June Won is a product manager with SageMaker JumpStart and Built-in Algorithms. He focuses on making ML contents easily discoverable and usable for SageMaker customers.
Pulkit Kapur is the product lead for the Alexa Teacher Model program with Alexa AI, focusing on generalized intelligence and applications of Alexa’s multitask multimodal foundation models.
How Yara is using MLOps features of Amazon SageMaker to scale energy optimization across their ammonia plants
Yara is the world’s leading crop nutrition company and a provider of environmental and agricultural solutions. Yara’s ambition is focused on growing a nature-positive food future that creates value for customers, shareholders, and society at large, and delivers a more sustainable food value chain. Supporting our vision of a world without hunger and a planet respected, Yara pursues a strategy of sustainable value growth, promoting climate-friendly crop nutrition and zero-emission energy solutions. Yara is also the world’s largest producer of ammonia, nitrates, and NPK fertilizers. Their production segment is therefore an integral building block for delivering on their mission—with a clearly stated ambition to become world-leading on metrics such as safety, environmental footprint, quality, and production costs. Yara’s long-term target is the “Plant of the Future” with zero emissions and low costs.
Building on a lean transformation, Yara ramps up their focus on digital solutions to help them achieve their ambitions. To lead this effort, Yara established a global unit called Digital Production. The success of Digital Production and its solutions is a key priority for Yara, and Yara significantly grew their efforts within this field. A critical focus area is to take advantage of the vast quantity of data generated as part of their operations. Therefore, Yara is building data-driven products that help them optimize production, increase the quality of products, increase reliability of production sites, reduce emissions, increase the safety and productivity of workers, automate manual processes, and more.
Energy is a major cost component for many production plants; hence, energy efficiency has a substantial impact on profitability. However, there is often a lack of solid references for what good performance looks like and how to get there. Yara’s Energy Load Curve (ELC) is a solution that uses the best historical performance on energy consumption held up against current performance. If the current consumption deviates too much from the historical best, the tool gives recommendations to the operators in order to steer the energy consumption.
To deploy ELC to production plants and scale it to multiple sites across the globe, Yara needed to build an MLOps platform. This would ensure Yara would train, deploy, and maintain models reliably and efficiently. Additionally, to scale this to multiple sites, Yara needed to automate the deployment and maintenance processes. In this post, we discuss how Yara is using Amazon SageMaker features, including the model registry, Amazon SageMaker Model Monitor, and Amazon SageMaker Pipelines to streamline the machine learning (ML) lifecycle by automating and standardizing MLOps practices. We provide an overview of the setup, showcasing the process of building, training, deploying, and monitoring ML models for plants around the globe.
Overview of solution
ELC uses Internet of Things (IoT) sensors data from a plant. These sensors measure metrics like production throughput, ambient conditions, and raw material conditions, etc. This data is used to train an energy prediction model which is then used to generate hourly predictions. Plant operators monitor the actual energy consumption and compare it with the optimal consumption as predicted by ELC. If the current energy consumption deviates too much from the optimal point, ELC provides an action to adjust internal process variables to optimize energy efficiency based on analytical models.
ELC is hosted in the cloud. In order to stream sensor data from a plant in real time, Yara uses AWS IoT Greengrass to communicate securely with AWS IoT Core and export IoT data to the AWS cloud. AWS IoT SiteWise is a managed service that can collect, organize, search, and consume equipment data from industrial equipment at scale. Yara has built APIs using Amazon API Gateway to expose the sensor data to applications such as ELC.
The ELC application backend is deployed via Amazon ECS and powers ELC dashboards on the front end that are used by plant operators. The ELC application is responsible for providing hourly predictive energy consumption metrics to plant operators. Each plant is fitted with its own model, because their energy consumption characteristics differ. Furthermore, plants are clustered into different AWS Regions based on their location.
The following diagram illustrates this architecture.
For building ELC and scaling to multiple plants, we needed an MLOps solution that supports the following:
- Scalability – It can scale in response to data volumes. Some plants produce more data than others; each plant can produce several gigabytes of data per day.
- Extendibility – It can deploy to new Regions and accounts.
- Repeatability – It has common templates that we can use to onboard a new plant.
- Flexibility – It can change the deployment configuration based on each plant’s needs.
- Reliability and monitoring – It can run tests and have a clear visibility into the status of all active plants. In case of failure, it can roll back to the previous stable state.
- Maintenance – The solution should have a low maintenance overhead. It should use serverless services where possible to reduce the infrastructure footprint.
For ML, Yara decided to use SageMaker. SageMaker is a fully-managed service that covers the entire ML workflow. The following features were critical in selecting SageMaker:
- SageMaker framework containers – Yara had trained ELC predictive models on TensorFlow, and with SageMaker framework containers, Yara was able to lift and shift these models with minimal code changes into SageMaker.
- SageMaker Pipelines – SageMaker Pipelines offer a Python interface for data scientists to write ML pipelines. A big portion of ELC code consists of a training and an inference pipeline, which are defined in Python.
- SageMaker model registry – The SageMaker model registry makes it possible to catalog and version control models. Additionally, it makes it easy to manage model metadata, such as training metrics.
- SageMaker Model Monitor – Yara wanted to monitor the quality and distribution of the incoming data as well as the ELC model performance. SageMaker Model Monitor APIs offer data and model quality monitoring.
To manage the continuous integration and continuous delivery (CI/CD) for the ML pipelines, Yara uses Amazon Deployment Framework (ADF). ADF is an open-source framework developed by AWS to manage and deploy resources across multiple AWS accounts and Regions within an AWS Organization. ADF allows for staged, parallel, multi-account, and cross-Region deployments of applications or resources via the structure defined in AWS Organizations, while taking advantage of services such as AWS CodePipeline, AWS CodeBuild, AWS CodeCommit, and AWS CloudFormation to alleviate the heavy lifting and management compared to a traditional CI/CD setup.
Solution overview
The entire solution for the MLOps platform was built within two months in a collaborative effort with AWS Professional Services. The team working on the project consisted of data scientists, data engineers, and DevOps specialists. To facilitate faster development in a multi-team environment, Yara chose to use AWS Landing Zone and Organizations to centrally create, manage, and govern different AWS accounts. For example, Yara has a central deployment account, and uses workload accounts to host business applications. ELC is a process optimization use case and is deployed to optimize workload accounts. The Yara Digital Production team also works on ML use cases in areas other than optimization. The MLOps framework supports deploying to any workload accounts as long as the accounts are created via Organizations.
The following diagram illustrates this architecture.
Using a central deployment account makes it easy to manage common artifacts and CI/CD pipelines. In terms of access management and security of these common artifacts, it’s a simpler design because permission boundaries and encryption keys are managed centrally in one place. In the following sections, we walk you through the steps required to onboard a new use case to Yara’s MLOps platform.
In terms of account strategy, Yara has a sandbox, DEV, TEST, and PROD setup. The sandbox account is used for experimentation and trying out new ideas. The DEV account is the starting point of the CI/CD pipelines, and all development starts here. The deployment account contains the CI/CD pipeline definition and is capable of deploying to the DEV, TEST, and PROD accounts. This account setup is depicted in the following figure.
Onboarding a new use case
For this post, we assume we have a working prototype of a use case, and now we want to operationalize it. In case this use case belongs to a new product area, we first need to provision the accounts using Organizations, which automatically triggers ADF to bootstrap these accounts for deployment. Yara follows a DEV>TEST>PROD account strategy; however, this configuration isn’t mandatory. Data accounts expose APIs for data access, and for a new use case, roles need to be granted the necessary AWS Identity and Access Management (IAM) permissions so they can access the Data APIs.
Next, we need to define which accounts this use case is deployed to. This is done using a deployment map in ADF. The deployment map is a configuration file that contains the mapping of stages and targets for the pipeline. To run the deployment map, ADF uses CodePipeline. ADF provides the flexibility to manage parameters per target environment the stack is deployed to. This makes it easy to manage deployments and test with smaller instances.
For encrypting all artifacts, such as code, data, and model files, we generate an AWS Key Management Service (AWS KMS) key. You can also use server-side encryption. However, because some of the generated artifacts are accessed across accounts, we need to generate our own key and manage its permission policies to grant cross-account access.
Finally, we need to create a model package group to group different versions of a model using the SageMaker model registry, which is the SageMaker capability to track and manage models as they move through the ML lifecycle.
Model training pipeline
For each new plant onboarded for ELC, we create a new SageMaker training pipeline. This pipeline consists of data preprocessing and model training steps. SageMaker pipelines are a good fit for Yara because they offer a Python interface for defining an ML workflow. Furthermore, different steps of the workflow can be configured to scale differently. For example, you can define a much bigger instance for training than for the model evaluation step. Input and output parameters for each step of the pipeline are stored, which makes it easy to track each run and its outputs. The high-level outline of the training workflow is as follows.
As part of the model evaluation stage, an evaluation dataset is used to generate metrics, such as accuracy and root-mean-squared error (RMSE) deviation on the trained model. These metrics are added to the model metadata before registering the model to the model registry. Currently, models are manually promoted to higher environments, and the model approver can view the model metrics to ensure the new version performs better than the current model.
Models are version controlled with the model registry, with each plant having its own model package group. Additionally, you can use the model registry to track which model versions are deployed to which environments. A model can be in a Rejected, Pending Manual Approval, or Approved state, and only models that are in the Approved state can be deployed. This also offers protection from accidentally deploying a non-approved version of the model.
Model inference and monitoring pipeline
To deploy the model and set up model monitoring, we set up a second SageMaker pipeline. The ELC application provides plant operators predictions on demand, therefore the models are accessed via API calls made from the ELC backend. SageMaker inference endpoints provide a fully managed model hosting solution with an API layer; endpoints take model input as payload and return predictions. Because latency is also a crucial factor for the end-users that don’t want to wait long before getting updated predictions, Yara opted for SageMaker real-time inference endpoints, which are particularly suitable for workloads with very low latency requirements. Finally, because the ELC application can’t have downtime while updated models are being deployed, it relies on the blue/green deployment capability of SageMaker real-time endpoints to ensure that the old model version continues to serve prediction until the new version is deployed.
The following diagram illustrates the deployment and monitoring setup.
For model monitoring, Yara runs SageMaker data quality, model quality, and model explainability monitoring. The data quality monitoring checks for consistency and generates data distribution statistics. Model quality monitoring checks the model performance and compares model accuracy against the training metrics. Model monitoring reports are generated on an hourly basis. These reports are used to monitor model performance in production. Model explainability monitoring is used to understand what features contribute most towards a prediction.
This results of model explainability are shared on the ELC dashboard to provide plant operators with more context on what drives the energy consumption. This also supports determining the action to adjust the internal process in case the energy consumption deviates from the optimal point.
CI/CD flow
The CI/CD flow for the training pipelines starts in the DEV account. Yara follows a feature-based development model and when a new feature is developed, the feature branch is merged into the trunk, which starts the deployment. ELC models are trained in the DEV account and after the model is trained and evaluated, it’s registered in the model registry. A model approver performs sanity checks before updating the model status to Approved. This action generates an event that triggers the deployment of the model inference pipeline. The model inference pipeline deploys the new model version to a SageMaker endpoint in DEV.
After the deployment of the endpoint, tests to check the behavior of the setup are started. For testing, Yara uses CodeBuild test reports. This feature allows developers to run unit tests, configuration tests, and functional tests pre- and post-deployment. In this case, Yara runs functional tests by passing test payloads to SageMaker endpoints and evaluating the response. After these tests are passed, the pipeline proceeds to deploy the SageMaker endpoints to TEST. The ELC backend is also deployed to TEST, which makes end-to-end testing for the app possible in this environment. Additionally, Yara runs user-acceptance testing in TEST. The trigger from TEST to PROD deployment is a manual approval action. After the new model version has passed both functional and user acceptance testing in TEST, the engineering team approves the model deployment to PROD.
The following figure illustrates this workflow.
Common components
For ELC, we use several components that are common for all deployment stages (DEV, TEST, PROD) and models. These components reside in our deployment account, and include model version control, a container image repository, an encryption key, and a bucket to store common artifacts.
There are several advantages of using common artifacts. For example, the resources don’t have to be created for every account, which enforces compatibility between the accounts. That means we build container images once and reuse them in all target accounts, reducing build time.
This pipeline stores the different model versions in a common model registry in the deployment account. From this central location, models can be deployed in all accounts without transferring them. Similarly, the use of a centrally stored encryption key makes it easier to manage the key and cross-account permissions.
One disadvantage of using common artifacts is that the onboarding step of a new use case can become more elaborate. To onboard a new use case, a new model registry must be created and if required a new container image repository. We also recommend creating a new encryption key to strictly separate resources and stored data.
Conclusion
In this post, we demonstrated how Yara used SageMaker and ADF to build a highly scalable MLOps platform. ML is a cross-functional capability, and teams deploy models to different business unit accounts. Therefore, ADF, which offers native integration with Organizations, makes it an ideal candidate to bootstrap accounts to set up CI/CD pipelines. Operationally, ADF pipelines run in the central deployment account, which makes it easy to get an overall health view of deployments. Finally, ADF uses AWS managed services like CodeBuild, CodeDeploy, CodePipeline, and CloudFormation, making it easy to configure and maintain.
SageMaker provides a broad spectrum of ML capabilities, which enables teams to focus more on solving business problems and less on building and maintaining infrastructure. Additionally, SageMaker Pipelines provides a rich set of APIs to create, update, and deploy ML workflows, making it a great fit for MLOps.
Lastly, MLOps provides the best practices to deploy and maintain ML models in production reliably and efficiently. It’s critical for teams who create and deploy ML solutions at scale to implement MLOps. In Yara’s case, MLOps significantly reduces the effort required to onboard a new plant, roll out updates to ELC, and ensure the models are monitored for quality.
For more information on how to deploy applications using ADF, see the examples.
About the authors
Shaheer Mansoor is a Data Scientist at AWS. His focus is on building machine learning platforms that can host AI solutions at scale. His interest areas are MLOps, feature stores, model hosting, and model monitoring.
Tim Becker is a Senior Data Scientist at Yara International. Within Digital Production, his focus is on process optimization of ammonia and nitric acid production. He holds a PhD in Thermodynamics and is passionate about bringing together process engineering and machine learning.
Yongyos Kaewpitakkun is a senior data scientist in the Digital Production team at Yara International. He has a PhD in AI/machine learning and many years of hands-on experience leveraging machine learning, computer vision, and natural language processing models to solve challenging business problems.
A Force to Be Reckoned With: Lucid Group Reveals Gravity SUV, Built on NVIDIA DRIVE
Meet the electric SUV with magnetic appeal.
Lucid Group unveiled its next act, the Gravity SUV, during the AutoMobility Los Angeles auto show. The automaker also launched additional versions of the hit Lucid Air sedan — Air Pure and Air Touring.
Both models offer the future-ready DreamDrive Pro driver-assistance system, powered by the NVIDIA DRIVE platform.
Lucid launched the Air late last year to widespread acclaim. The luxury sedan won MotorTrend’s Car of the Year for 2022, with a chart-topping battery range of up to 516 miles and fast charging.
The newly introduced variants provide updated features for a wider audience. Air Pure is designed for agility, with a lightweight, compact battery and industry-leading aerodynamics.
Air Touring is the heart of the lineup, featuring more horsepower and battery range than the Pure and greater flexibility in customer options.
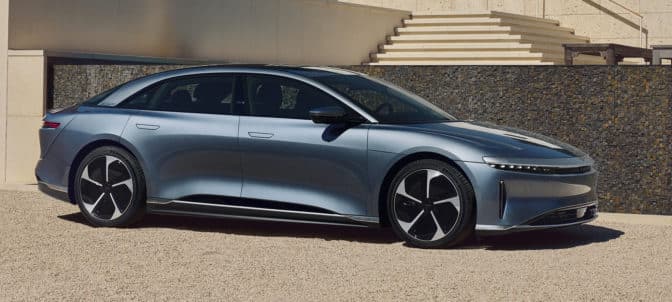
Gravity builds on this stellar reputation with an aerodynamic, spacious and intelligent design, all backed by the high-performance, centralized compute of NVIDIA DRIVE.
“Just as Lucid Air redefined the sedan category, so too will Gravity impact the world of luxury SUVs, setting new benchmarks across the board,” said Lucid Group CEO and CTO Peter Rawlinson.
Capable and Enjoyable
DreamDrive Pro is software defined, continuously improving via over-the-air software updates.
It uses a rich suite of 14 cameras, one lidar, five radars and 12 ultrasonics running on NVIDIA DRIVE for robust automated driving and intelligent cockpit features, including surround-view monitoring, blind-spot display and highway assist.
In addition to a diversity of sensors, Lucid’s dual-rail power system and proprietary Ethernet Ring offer a high degree of redundancy for key systems, such as braking and steering.
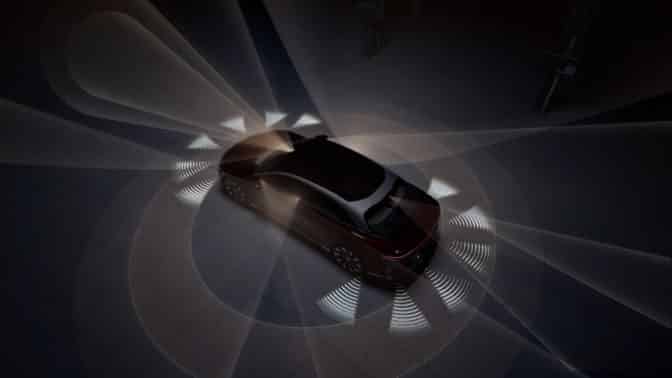
“The Lucid Air is at its core a software-defined vehicle, meaning a large part of the experience is delivered by the software,” Rawlinson said. “This makes the Lucid Air more capable and enjoyable with every passing update.”
Prepare to Launch
These new Lucid vehicles are nearly ready for liftoff.
The Lucid Air Touring has already begun production, and Air Pure will start in December, with customer deliveries soon to follow.
The automaker will open reservations for the Lucid Gravity in the spring, slating deliveries to begin in 2024.
The post A Force to Be Reckoned With: Lucid Group Reveals Gravity SUV, Built on NVIDIA DRIVE appeared first on NVIDIA Blog.
The Data Cards Playbook: A Toolkit for Transparency in Dataset Documentation
As machine learning (ML) research moves toward large-scale models capable of numerous downstream tasks, a shared understanding of a dataset’s origin, development, intent, and evolution becomes increasingly important for the responsible and informed development of ML models. However, knowledge about datasets, including use and implementations, is often distributed across teams, individuals, and even time. Earlier this year at the ACM Conference on Fairness, Accountability, and Transparency (ACM FAccT), we published Data Cards, a dataset documentation framework aimed at increasing transparency across dataset lifecycles. Data Cards are transparency artifacts that provide structured summaries of ML datasets with explanations of processes and rationale that shape the data and describe how the data may be used to train or evaluate models. At minimum, Data Cards include the following: (1) upstream sources, (2) data collection and annotation methods, (3) training and evaluation methods, (4) intended use, and (5) decisions affecting model performance.
In practice, two critical factors determine the success of a transparency artifact, the ability to identify the information decision-makers use and the establishment of processes and guidance needed to acquire that information. We started to explore this idea in our paper with three “scaffolding” frameworks designed to adapt Data Cards to a variety of datasets and organizational contexts. These frameworks helped us create boundary infrastructures, which are the processes and engagement models that complement technical and functional infrastructure necessary to communicate information between communities of practice. Boundary infrastructures enable dataset stakeholders to find common ground used to provide diverse input into decisions for the creation, documentation, and use of datasets.
Today, we introduce the Data Cards Playbook, a self-guided toolkit for a variety of teams to navigate transparency challenges with their ML datasets. The Playbook applies a human-centered design approach to documentation — from planning a transparency strategy and defining the audience to writing reader-centric summaries of complex datasets — to ensure that the usability and utility of the documented datasets are well understood. We’ve created participatory activities to navigate typical obstacles in setting up a dataset transparency effort, frameworks that can scale data transparency to new data types, and guidance that researchers, product teams and companies can use to produce Data Cards that reflect their organizational principles.
![]() |
The Data Cards Playbook incorporates the latest in fairness, accountability, and transparency research. |
The Data Cards Playbook
We created the Playbook using a multi-pronged approach that included surveys, artifact analysis, interviews, and workshops. We studied what Googlers wanted to know about datasets and models, and how they used that information in their day-to-day work. Over the past two years, we deployed templates for transparency artifacts used by fifteen teams at Google, and when bottlenecks arose, we partnered with these teams to determine appropriate workarounds. We then created over twenty Data Cards that describe image, language, tabular, video, audio, and relational datasets in production settings, some of which are now available on GitHub. This multi-faceted approach provided insights into the documentation workflows, collaborative information-gathering practices, information requests from downstream stakeholders, and review and assessment practices for each Google team.
Moreover, we spoke with design, policy, and technology experts across the industry and academia to get their unique feedback on the Data Cards we created. We also incorporated our learnings from a series of workshops at ACM FAccT in 2021. Within Google, we evaluated the effectiveness and scalability of our solutions with ML researchers, data scientists, engineers, AI ethics reviewers, product managers, and leadership. In the Data Cards Playbook, we’ve translated successful approaches into repeatable practices that can easily be adapted to unique team needs.
Activities, Foundations, and Transparency Patterns
The Data Cards Playbook is modeled after sprints and co-design practices, so cross-functional teams and their stakeholders can work together to define transparency with an eye for real-world problems they experience when creating dataset documentation and governance solutions. The thirty-three available Activities invite broad, critical perspectives from a wide variety of stakeholders, so Data Cards can be useful for decisions across the dataset lifecycle. We partnered with researchers from the Responsible AI team at Google to create activities that can reflect considerations of fairness and accountability. For example, we’ve adapted Evaluation Gaps in ML practices into a worksheet for more complete dataset documentation.
![]() |
Download readily-available activity templates to use the Data Cards Playbook in your organization. |
We’ve formed Transparency Patterns with evidence-based guidance to help anticipate challenges faced when producing transparent documentation, offer best practices that improve transparency, and make Data Cards useful for readers from different backgrounds. The challenges and their workarounds are based on data and insights from Googlers, industry experts, and academic research.
![]() |
Patterns help unblock teams with recommended practices, caution against common pitfalls, and suggested alternatives to roadblocks. |
The Playbook also includes Foundations, which are scalable concepts and frameworks that explore fundamental aspects of transparency as new contexts of data modalities and ML arise. Each Foundation supports different product development stages and includes key takeaways, actions for teams, and handy resources.
Playbook Modules
The Playbook is organized into four modules: (1) Ask, (2) Inspect, (3) Answer, and (3) Audit. Each module contains a growing compendium of materials teams can use within their workflows to tackle transparency challenges that frequently co-occur. Since Data Cards were created with scalability and extensibility in mind, modules leverage divergence-converge thinking that teams may already use, so documentation isn’t an afterthought. The Ask and Inspect modules help create and evaluate Data Card templates for organizational needs and principles. The Answer and Audit modules help data teams complete the templates and evaluate the resulting Data Cards.
In Ask, teams define transparency and optimize their dataset documentation for cross-functional decision-making. Participatory activities create opportunities for Data Card readers to have a say in what constitutes transparency in the dataset’s documentation. These address specific challenges and are rated for different intensities and durations so teams can mix-and-match activities around their needs.
The Inspect module contains activities to identify gaps and opportunities in dataset transparency and processes from user-centric and dataset-centric perspectives. It supports teams in refining, validating, and operationalizing Data Card templates across an organization so readers can arrive at reasonable conclusions about the datasets described.
The Answer module contains transparency patterns and dataset-exploration activities to answer challenging and ambiguous questions. Topics covered include preparing for transparency, writing reader-centric summaries in documentation, unpacking the usability and utility of datasets, and maintaining a Data Card over time.
The Audit module helps data teams and organizations set up processes to evaluate completed Data Cards before they are published. It also contains guidance to measure and track how a transparency effort for multiple datasets scales within organizations.
In Practice
A data operations team at Google used an early version of the Lenses and Scopes Activities from the Ask modules to create a customized Data Card template. Interestingly, we saw them use this template across their workflow till datasets were handed off. They used Data Cards to take dataset requests from research teams, tracked the various processes to create the datasets, collected metadata from vendors responsible for annotations, and managed approvals. Their experiences of iterating with experts and managing updates are reflected in our Transparency Patterns.
Another data governance group used a more advanced version of the activities to interview stakeholders for their ML health-related initiative. Using these descriptions, they identified stakeholders to co-create their Data Card schema. Voting on Lenses was used to rule out typical documentation questions, and identify atypical documentation needs specific to their data type, and important for decisions frequently made by ML leadership and tactical roles within their team. These questions were then used to customize existing metadata schemas in their data repositories.
Conclusion
We present the Data Cards Playbook, a continuous and contextual approach to dataset transparency that deliberately considers all relevant materials and contexts. With this, we hope to establish and promote practice-oriented foundations for transparency to pave the path for researchers to develop ML systems and datasets that are responsible and benefit society.
In addition to the four Playbook modules described, we’re also open-sourcing a card builder, which generates interactive Data Cards from a Markdown file. You can see the builder in action in the GEM Benchmark project’s Data Cards. The Data Cards created were a result of activities from this Playbook, in which the GEM team identified improvements across all dimensions, and created an interactive collection tool designed around scopes.
We acknowledge that this is not a comprehensive solution for fairness, accountability, or transparency in itself. We’ll continue to improve the Playbook using lessons learned. We hope the Data Cards Playbook can become a robust platform for collaboratively advancing transparency research, and invite you to make this your own.
Acknowledgements
This work was done in collaboration with Reena Jana, Vivian Tsai, and Oddur Kjartansson. We want to thank Donald Gonzalez, Dan Nanas, Parker Barnes, Laura Rosenstein, Diana Akrong, Monica Caraway, Ding Wang, Danielle Smalls, Aybuke Turker, Emily Brouillet, Andrew Fuchs, Sebastian Gehrmann, Cassie Kozyrkov, Alex Siegman, and Anthony Keene for their immense contributions; and Meg Mitchell and Timnit Gebru for championing this work.
We also want to thank Adam Boulanger, Lauren Wilcox, Roxanne Pinto, Parker Barnes, and Ayça Çakmakli for their feedback; Tulsee Doshi, Dan Liebling, Meredith Morris, Lucas Dixon, Fernanda Viegas, Jen Gennai, and Marian Croak for their support. This work would not have been possible without our workshop and study participants, and numerous partners, whose insights and experiences have shaped this Playbook.
Build high performing image classification models using Amazon SageMaker JumpStart
Image classification is a computer vision-based machine learning (ML) technique that allows you to classify images. Some well-known examples of image classification include classifying handwritten digits, medical image classification, and facial recognition. Image classification is a useful technique with several business applications, but building a good image classification model isn’t trivial.
Several considerations can play a role when evaluating an ML model. Beyond model accuracy, other potential metrics of importance are model training time and inference time. Given the iterative nature of ML model development, faster training times allow data scientists to quickly test various hypotheses. Faster inferencing can be critical in real-time applications.
Amazon SageMaker JumpStart provides one-click fine-tuning and deployment of a wide variety of pre-trained models across popular ML tasks, as well as a selection of end-to-end solutions that solve common business problems. These features remove the heavy lifting from each step of the ML process, making it easier to develop high-quality models and reducing time to deployment. JumpStart APIs allow you to programmatically deploy and fine-tune a vast selection of JumpStart-supported pre-trained models on your own datasets.
You can incrementally train and tune the ML models offered in JumpStart before deployment. At the time of writing, 87 deep-learning based image classification models are available in JumpStart.
But which model will give you the best results? In this post, we present a methodology to easily run multiple models and compare their outputs on three dimensions of interest: model accuracy, training time, and inference time.
Solution overview
JumpStart allows you to train, tune, and deploy models either from the JumpStart console using its UI or with its API. In this post, we use the API route, and present a notebook with various helper scripts. You can run this notebook and get results for easy comparison of these models against each other, and then pick a model that best suits your business need in terms of model accuracy, training time, and inference time.
The public dataset used in this post consists of nearly 55,000 images of diseased and healthy plant leaves collected under controlled conditions, with class labels ranging from 0–38. This dataset is divided into train and validation datasets, with approximately 44,000 under training and 11,000 images under validation. The following are a few sample images.
For this exercise, we selected models from two frameworks—PyTorch and TensorFlow—as offered by JumpStart. The following 15 model algorithms cover a wide range of popular neural network architectures from these frameworks:
pytorch-ic-alexnet-FT
pytorch-ic-densenet121-FT
pytorch-ic-densenet201-FT
pytorch-ic-googlenet-FT
pytorch-ic-mobilenet-v2-FT
pytorch-ic-resnet152-FT
pytorch-ic-resnet34-FT
tensorflow-ic-bit-s-r101x1-ilsvrc2012-classification-1-FT
tensorflow-ic-imagenet-inception-resnet-v2-classification 4-FT
tensorflow-ic-imagenet-inception-v3-classification-4-FT
tensorflow-ic-imagenet-mobilenet-v2-050-224-classification-4-FT
tensorflow-ic-imagenet-mobilenet-v2-075-224-classification-4-FT
tensorflow-ic-imagenet-mobilenet-v2-140-224-classification-4-FT
tensorflow-ic-imagenet-resnet-v2-152-classification-4-FT
tensorflow-ic-tf2-preview-mobilenet-v2-classification-4-FT
We use the model tensorflow-ic-imagenet-inception-v3-classification-4-FT
as a base against which results from other models are compared. This base model was picked arbitrarily.
The code used to run this comparison is available on the AWS Samples GitHub repo.
Results
In this section, we present the results from these 15 runs. For all these runs, the hyperparameters used were epochs = 5, learning rate = 0.001, batch size = 16.
Model accuracy, training time, and inference time from model tensorflow-ic-imagenet-inception-v3-classification-4-FT
were taken as the base, and results from all other models are presented relative to this base model. Our intention here is not to show which model is the best but to rather show how, through the JumpStart API, you can compare results from various models and then choose a model that best fits your use case.
The following screenshot highlights the base model against which all other models were compared.
The following plot shows a detailed view of relative accuracy vs. relative training time. PyTorch models are color coded in red and TensorFlow models in blue.
The models highlighted with a green ellipse in the preceding plot seem to have a good combination of relative accuracy and low relative training time. The following table provides more details on these three models.
Model Name | Relative Accuracy | Relative Training Time |
tensorflow-ic-imagenet-mobilenet-v2-050-224-classification-4-FT | 1.01 | 0.74 |
tensorflow-ic-imagenet-mobilenet-v2-140-224-classification-4-FT | 1.02 | 0.74 |
tensorflow-ic-bit-s-r101x1-ilsvrc2012-classification-1-FT | 1.04 | 1.16 |
The following plot compares relative accuracy vs. relative inference time. PyTorch models are color coded in red and TensorFlow models in blue.
The following table provides details on the three models in the green ellipse.
Model Name | Relative Accuracy | Relative Inference Time |
tensorflow-ic-imagenet-mobilenet-v2-050-224-classification-4-FT | 1.01 | 0.94 |
tensorflow-ic-imagenet-mobilenet-v2-140-224-classification-4-FT | 1.02 | 0.90 |
tensorflow-ic-bit-s-r101x1-ilsvrc2012-classification-1-FT | 1.04 | 1.43 |
The two plots clearly demonstrate that certain model algorithms performed better than others on the three dimensions that were selected. The flexibility offered through this exercise can help you pick the right algorithm, and by using the provided notebook, you can easily run this type of experiment on any of the 87 available models.
Conclusion
In this post, we showed how to use JumpStart to build high performing image classification models on multiple dimensions of interest, such as model accuracy, training time, and inference latency. We also provided the code to run this exercise on your own dataset; you can pick any models of interest from the 87 models that are presently available for image classification in the JumpStart model hub. We encourage you to give it a try today.
For more details on JumpStart, refer to SageMaker JumpStart.
About the Authors
Dr. Raju Penmatcha is an AI/ML Specialist Solutions Architect in AI Platforms at AWS. He received his PhD from Stanford University. He works closely on the low/no-code suite of services in SageMaker, which help customers easily build and deploy machine learning models and solutions. When not helping customers, he likes traveling to new places.
Dr. Ashish Khetan is a Senior Applied Scientist with Amazon SageMaker built-in algorithms and helps develop machine learning algorithms. He got his PhD from University of Illinois Urbana-Champaign. He is an active researcher in machine learning and statistical inference, and has published many papers in NeurIPS, ICML, ICLR, JMLR, ACL, and EMNLP conferences.