In today’s highly competitive market, performing data analytics using machine learning (ML) models has become a necessity for organizations. It enables them to unlock the value of their data, identify trends, patterns, and predictions, and differentiate themselves from their competitors. For example, in the healthcare industry, ML-driven analytics can be used for diagnostic assistance and personalized medicine, while in health insurance, it can be used for predictive care management.
However, organizations and users in industries where there is potential health data, such as in healthcare or in health insurance, must prioritize protecting the privacy of people and comply with regulations. They are also facing challenges in using ML-driven analytics for an increasing number of use cases. These challenges include a limited number of data science experts, the complexity of ML, and the low volume of data due to restricted Protected Health Information (PHI) and infrastructure capacity.
Organizations in the healthcare, clinical, and life sciences are facing several challenges in using ML for data analytics:
- Low volume of data – Due to restrictions on private, protected, and sensitive health information, the volume of usable data is often limited, reducing the accuracy of ML models
- Limited talent – Hiring ML talent is hard enough, but hiring talent that has not only ML experience but also deep medical knowledge is even harder
- Infrastructure management – Provisioning infrastructure specialized for ML is a difficult and time-consuming task, and companies would rather focus on their core competencies than manage complex technical infrastructure
- Prediction of multi-modal issues – When predicting the likelihood of multi-faceted medical events, such as a stroke, different factors such as medical history, lifestyle, and demographic information must be combined
A possible scenario is that you are a healthcare technology company with a team of 30 non-clinical physicians researching and investigating medical cases. This team has the knowledge and intuition in healthcare but not the ML skills to build models and generate predictions. How can you deploy a self-service environment that allows these clinicians to generate predictions themselves for multivariate questions like, “How can I access useful data while being compliant with health regulations and without compromising privacy?” And how can you do that without exploding the number of servers the SysOps folks need to manage?
This post addresses all these problems simultaneously in one solution. First, it automatically anonymizes the data from Amazon HealthLake. Then, it uses that data with serverless components and no-code self-service solutions like Amazon SageMaker Canvas to eliminate the ML modeling complexity and abstract away the underlying infrastructure.
A modern data strategy gives you a comprehensive plan to manage, access, analyze, and act on data. AWS provides the most complete set of services for the entire end-to-end data journey for all workloads, all types of data, and all desired business outcomes.
Solution overview
This post shows that by anonymizing sensitive data from Amazon HealthLake and making it available to SageMaker Canvas, organizations can empower more stakeholders to use ML models that can generate predictions for multi-modal problems, such as stroke prediction, without writing ML code, while limiting access to sensitive data. And we want to automate that anonymization to make this as scalable and amenable to self-service as possible. Automating also allows you to iterate the anonymization logic to meet your compliance requirements, and provides the ability to re-run the pipeline as your population’s health data changes.
The dataset used in this solution is generated by Synthea
, a Synthetic Patient Population Simulator and open-source project under the Apache License 2.0.
The workflow includes a hand-off between cloud engineers and domain experts. The former can deploy the pipeline. The latter can verify if the pipeline is correctly anonymizing the data and then generate predictions without code. At the end of the post, we’ll look at additional services to verify the anonymization.
The high-level steps involved in the solution are as follows:
- Use AWS Step Functions to orchestrate the health data anonymization pipeline.
- Use Amazon Athena queries for the following:
- Extract non-sensitive structured data from Amazon HealthLake.
- Use natural language processing (NLP) in Amazon HealthLake to extract non-sensitive data from unstructured blobs.
- Perform one-hot encoding with Amazon SageMaker Data Wrangler.
- Use SageMaker Canvas for analytics and predictions.
The following diagram illustrates the solution architecture.
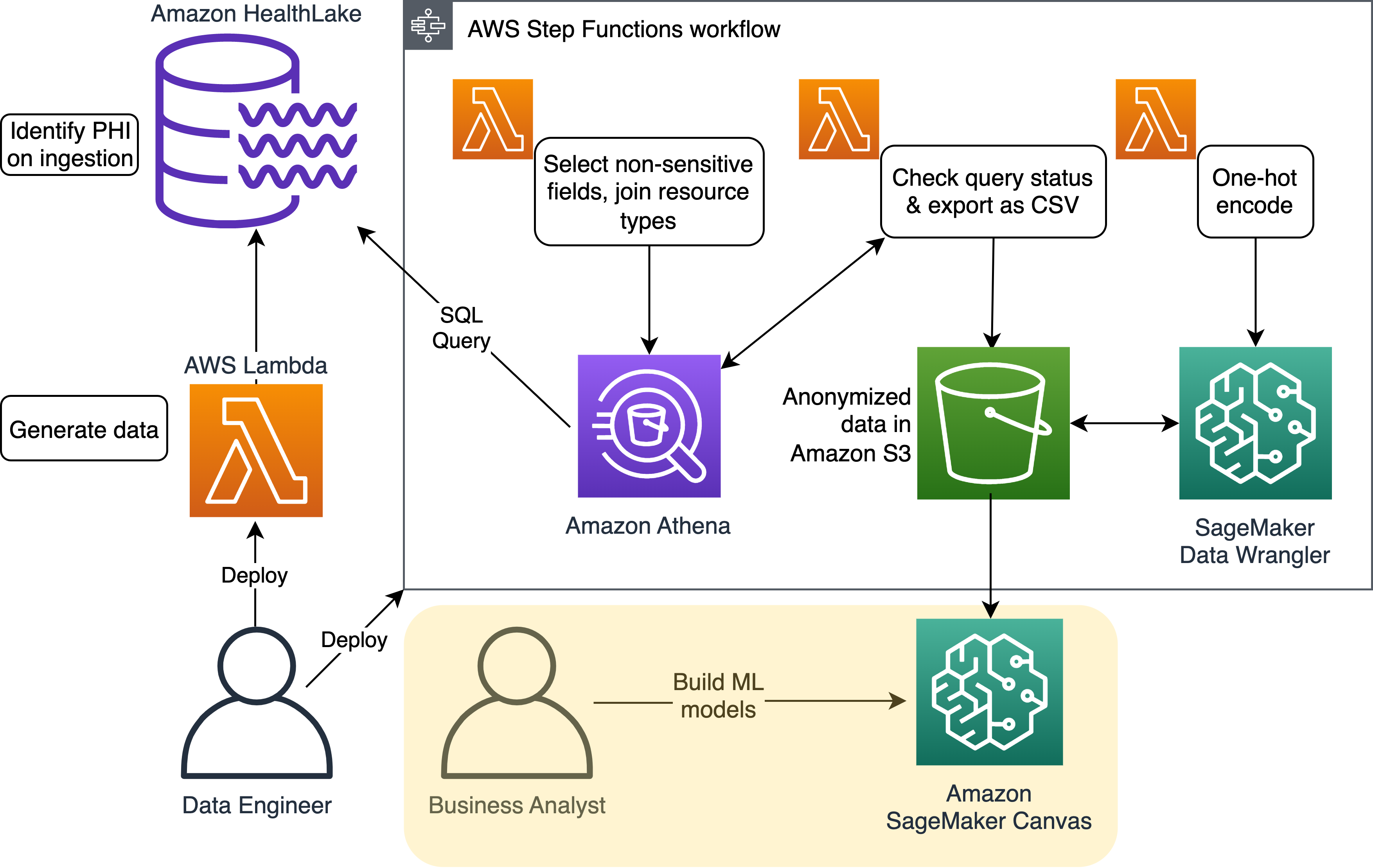
Prepare the data
First, we generate a fictional patient population using Synthea
and import that data into a newly created Amazon HealthLake data store. The result is a simulation of the starting point from where a healthcare technology company could run the pipeline and solution described in this post.
When Amazon HealthLake ingests data, it automatically extracts meaning from unstructured data, such as doctors notes, into separate structured fields, such as patient names and medical conditions. To accomplish this on the unstructured data in DocumentReference
FHIR resources, Amazon HealthLake transparently triggers Amazon Comprehend Medical, where entities, ontologies, and their relationships are extracted and added back to Amazon HealthLake as discreet data within the extension segment of records.
We can use Step Functions to streamline the collection and preparation of the data. The entire workflow is visible in one place, with any errors or exceptions highlighted, allowing for a repeatable, auditable, and extendable process.
Query the data using Athena
By running Athena SQL queries directly on Amazon HealthLake, we are able to select only those fields that are not personally identifying; for example, not selecting name and patient ID, and reducing birthdate to birth year. And by using Amazon HealthLake, our unstructured data (the text field in DocumentReference
) automatically comes with a list of detected PHI, which we can use to mask the PHI in the unstructured data. In addition, because generated Amazon HealthLake tables are integrated with AWS Lake Formation, you can control who gets access down to the field level.
The following is an excerpt from an example of unstructured data found in a synthetic DocumentReference
record:
# History of Present Illness
Marquis
is a 45 year-old. Patient has a history of hypertension, viral sinusitis (disorder), chronic obstructive bronchitis (disorder), stress (finding), social isolation (finding).
# Social History
Patient is married. Patient quit smoking at age 16.
Patient currently has UnitedHealthcare.
# Allergies
No Known Allergies.
# Medications
albuterol 5 mg/ml inhalation solution; amlodipine 2.5 mg oral tablet; 60 actuat fluticasone propionate 0.25 mg/actuat / salmeterol 0.05 mg/actuat dry powder inhaler
# Assessment and Plan
Patient is presenting with stroke.
We can see that Amazon HeathLake NLP interprets this as containing the condition “stroke” by querying for the condition record that has the same patient ID and displays “stroke.” And we can take advantage of the fact that entities found in DocumentReference are automatically labeled SYSTEM_GENERATED
:
SELECT
code.coding[1].code,
code.coding[1].display
FROM
condition
WHERE
split(subject.reference, '/')[2] = 'fbfe46b4-70b1-8f61-c343-d241538ebb9b'
AND
meta.tag[1].display = 'SYSTEM_GENERATED'
AND regexp_like(code.coding[1].display, 'Cerebellar stroke syndrome')
The result is as follows:
G46.4, Cerebellar stroke syndrome
The data collected in Amazon HealthLake can now be effectively used for analytics thanks to the ability to select specific condition codes, such as G46.4, rather than having to interpret entire notes. This data is then stored as a CSV file in Amazon Simple Storage Service (Amazon S3).
Note: When implementing this solution, please follow the instructions on turning on HealthLake’s integrated NLP feature via a support case before ingesting data into your HealthLake data store.
Perform one-hot encoding
To unlock the full potential of the data, we use a technique called one-hot encoding to convert categorical columns, like the condition column, into numerical data.
One of the challenges of working with categorical data is that it is not as amenable to being used in many machine learning algorithms. To overcome this, we use one-hot encoding, which converts each category in a column to a separate binary column, making the data suitable for a wider range of algorithms. This is done using Data Wrangler, which has built-in functions for this:
The built-in function for one-hot encoding in SageMaker Data Wrangler
One-hot encoding transforms each unique value in the categorical column into a binary representation, resulting in a new set of columns for each unique value. In the example below, the condition column is transformed into six columns, each representing one unique value. After one-hot encoding, the same rows would turn into a binary representation.
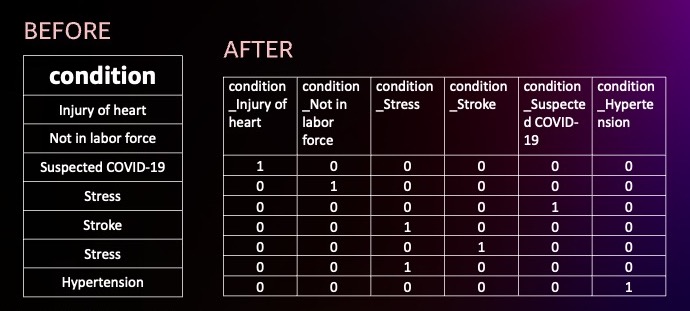
With the data now encoded, we can move on to using SageMaker Canvas for analytics and predictions.
Use SageMaker Canvas for analytics and predictions
The final CSV file then becomes the input for SageMaker Canvas, which healthcare analysts (business users) can use to generate predictions for multivariate problems like stroke prediction without needing to have expertise in ML. No special permissions are required because the data doesn’t contain any sensitive information.
In the example of stroke prediction, SageMaker Canvas was able to achieve an accuracy rate of 99.829% through the use of advanced ML models, as shown in the following screenshot.
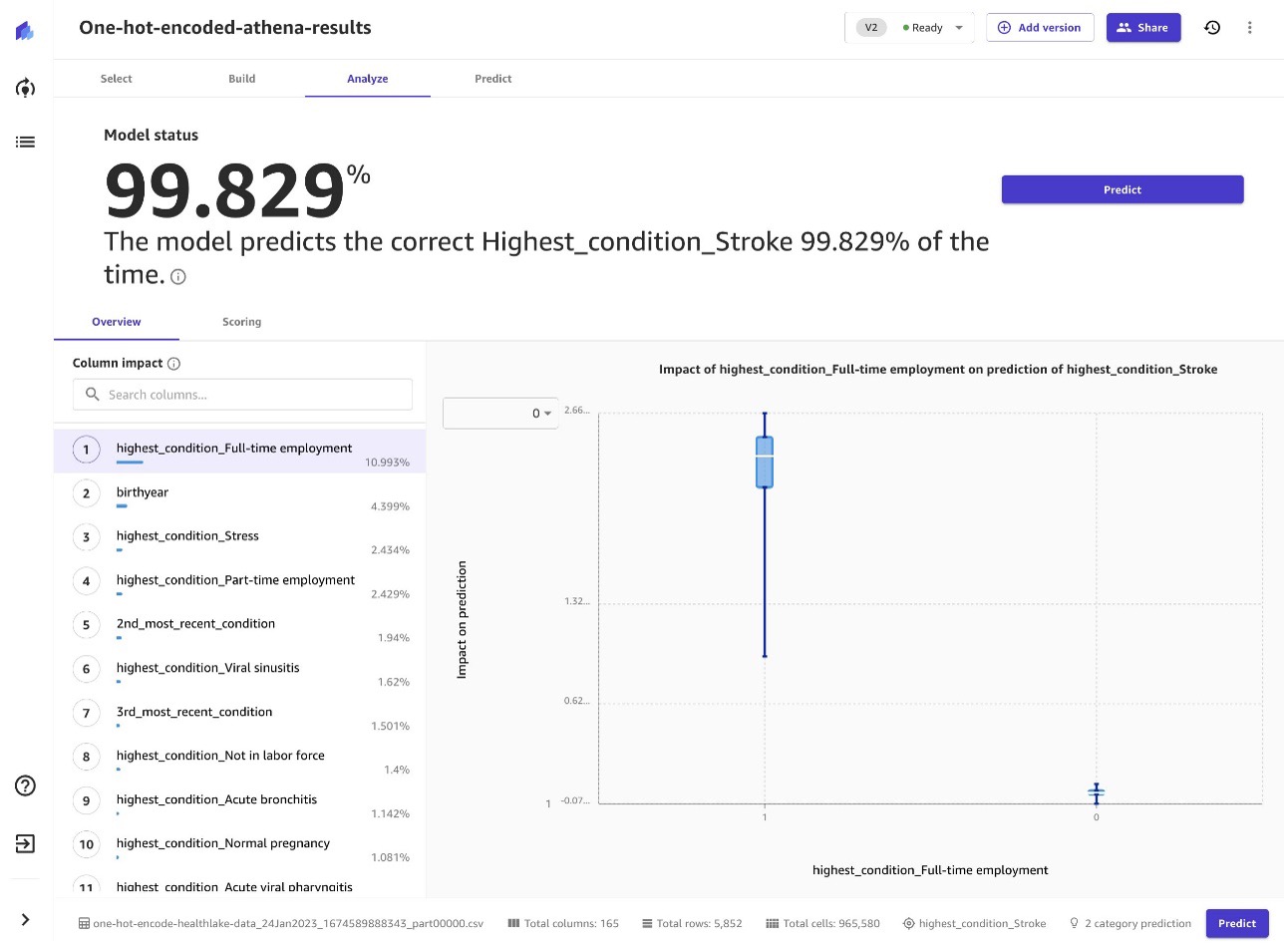
In the next screenshot, you can see that, according to the model’s prediction, this patient has a 53% chance of not having a stroke.
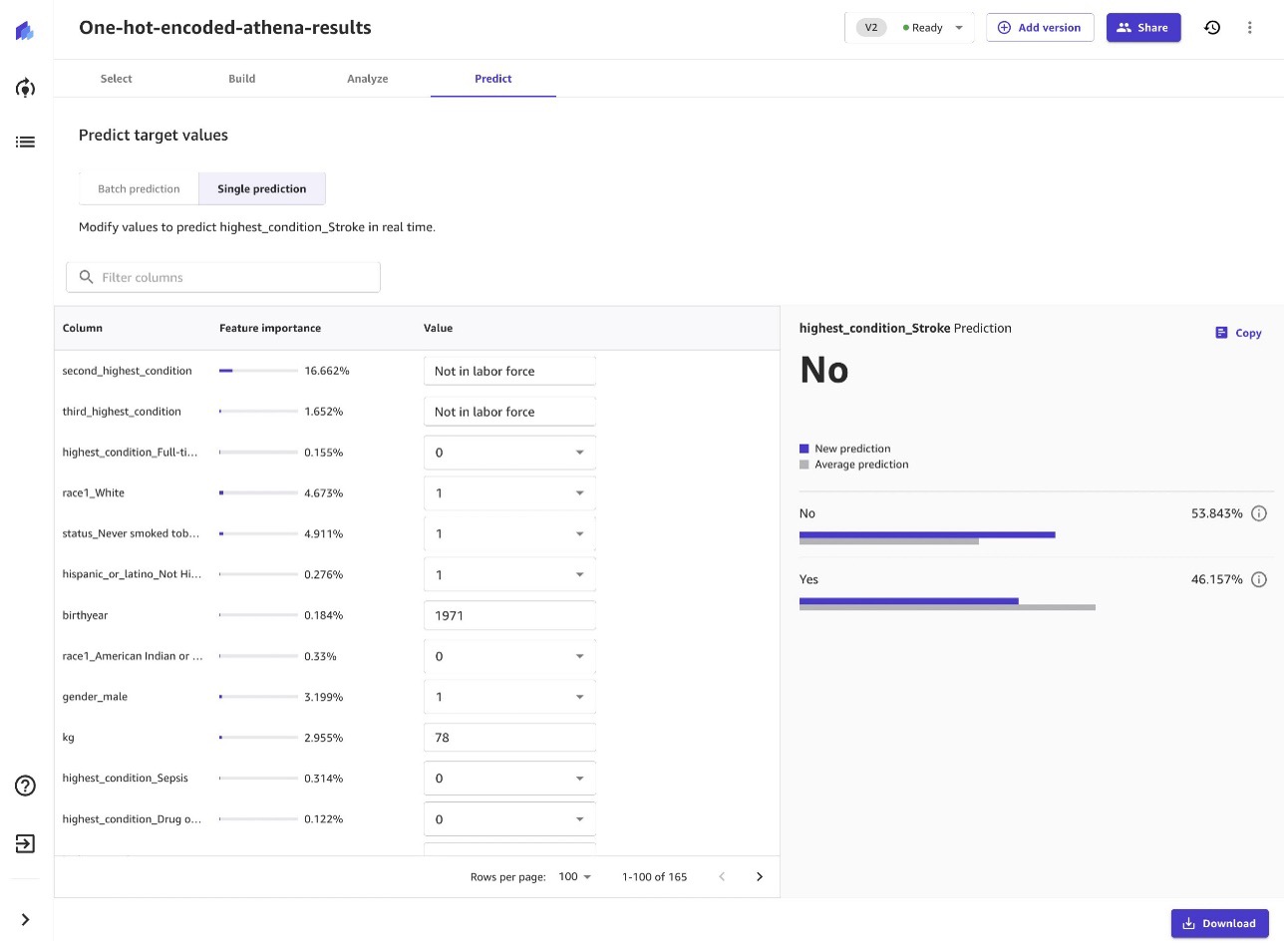
You might posit that you can create this prediction using rule-based logic in a spreadsheet. But do those rules tell you the feature importance—for example, that 4.9% of the prediction is based on whether or not they have ever smoked tobacco? And what if, in addition to the current columns like smoking status and blood pressure, you add 900 more columns (features)? Would you still be able to use a spreadsheet to maintain and manage the combinations of all those dimensions? Real-life scenarios lead to many combinations, and the challenge is to manage this at scale with the right level of effort.
Now that we have this model, we can start making batch or single predictions, asking what-if questions. For example, what if this person keeps all variables the same but, as of the previous two encounters with the medical system, is classified as Full-time employment instead of Not in labor force?
According to our model, and the synthetic data we fed it from Synthea, the person is at a 62% risk of having a stroke.
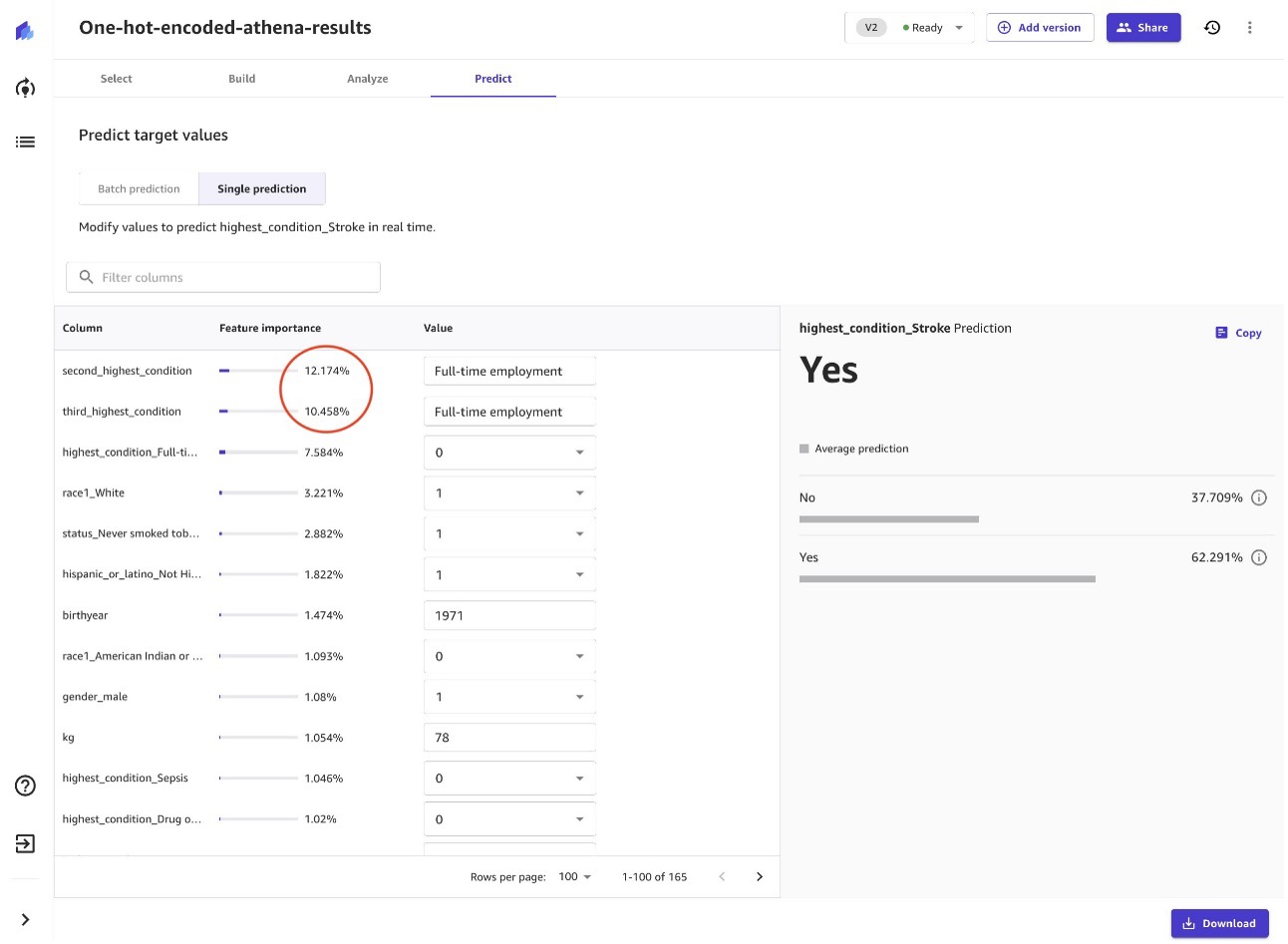
As we can tell from the circled 12% and 10% feature importance of the conditions from the two most recent encounters with the medical system, whether they are full-time employed or not has a big impact on their risk of stroke. Beyond the findings of this model, there is research that demonstrates a similar link:
These studies have used large population-based samples and controlled for other risk factors, but it’s important to note that they are observational in nature and do not establish causality. Further research is needed to fully understand the relationship between full-time employment and stroke risk.
Enhancements and alternate methods
To further validate compliance, we can use services like Amazon Macie, which will scan the CSV files in the S3 bucket and alert us if there is any sensitive data. This helps increase the confidence level of the anonymized data.
In this post, we used Amazon S3 as the input data source for SageMaker Canvas. However, we can also import data into SageMaker Canvas directly from Amazon RedShift and Snowflake—popular enterprise data warehouse services used by many customers to organize their data and popular third-party solutions. This is especially important for customers who already have their data in either Snowflake or Amazon Redshift being used for other BI analytics.
By using Step Functions to orchestrate the solution, the solution is more extensible. Instead of a separate trigger to invoke Macie, you can add another step to the end of the pipeline to call Macie to double-check for PHI. If you want to add rules to monitor your data pipeline’s quality over time, you can add a step for AWS Glue Data Quality.
And if you want to add more bespoke integrations, Step Functions lets you scale out to handle as much data or as little data as you need in parallel and only pay for what you use. The parallelization aspect is useful when you are processing hundreds of GB of data, because you don’t want to try to jam all that into one function. Instead, you want to break it up and run it in parallel so you’re not waiting for it to process in a single queue. This is similar to a check-out line at the store—you don’t want to have a single cashier.
Clean up
To avoid incurring future session charges, log out of SageMaker Canvas.
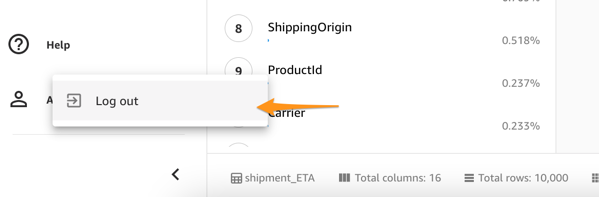
Conclusion
In this post, we showed that predictions for critical health issues like stroke can be done by medical professionals using complex ML models but without the need for coding. This will vastly increase the pool of resources by including people who have specialized domain knowledge but no ML experience. Also, using serverless and managed services allows existing IT people to manage infrastructure challenges like availability, resilience, and scalability with less effort.
You can use this post as a starting point to investigate other complex multi-modal predictions, which are key to guiding the health industry toward better patient care. Coming soon, we will have a GitHub repository to help engineers more quickly launch the kind of ideas we presented in this post.
Experience the power of SageMaker Canvas today, and build your models using a user-friendly graphical interface, with the 2-month Free Tier that SageMaker Canvas offers. You don’t need any coding knowledge to get started, and you can experiment with different options to see how your models perform.
Resources
To learn more about SageMaker Canvas, refer to the following:
To learn more about other use cases that you can solve with SageMaker Canvas, check out the following:
To learn more about Amazon HealthLake, refer to the following:
About the Authors
Yann Stoneman is a Solutions Architect at AWS based out of Boston, MA and is a member of the AI/ML Technical Field Community (TFC). Yann earned his Bachelors at The Juilliard School. When he’s not modernizing workloads for global enterprises, Yann plays piano, tinkers in React and Python, and regularly YouTubes about his cloud journey.
Ramesh Dwarakanath is a Principal Solutions Architect at AWS based out of Boston, MA. He works with Enterprises in the Northeast area on their Cloud journey. His areas of interest are Containers and DevOps. In his spare time, Ramesh enjoys tennis, racquetball.
Bakha Nurzhanov is an Interoperability Solutions Architect at AWS, and is a member of the Healthcare and Life Sciences technical field community at AWS. Bakha earned his Masters in Computer Science from the University of Washington and in his spare time Bakha enjoys spending time with family, reading, biking, and exploring new places.
Scott Schreckengaust has a degree in biomedical engineering and has been inventing devices alongside scientists on the bench since the beginning of his career. He loves science, technology, and engineering with decades of experiences in startups to large multi-national organizations within the Healthcare and Life Sciences domain. Scott is comfortable scripting robotic liquid handlers, programming instruments, integrating homegrown systems into enterprise systems, and developing complete software deployments from scratch in regulatory environments. Besides helping people out, he thrives on building — enjoying the journey of hashing out customer’s scientific workflows and their issues then converting those into viable solutions.
Read More