Recent developments in deep learning have led to increasingly large models such as GPT-3, BLOOM, and OPT, some of which are already in excess of 100 billion parameters. Although larger models tend to be more powerful, training such models requires significant computational resources. Even with the use of advanced distributed training libraries like FSDP and DeepSpeed, it’s common for training jobs to require hundreds of accelerator devices for several weeks or months at a time.
In late 2022, AWS announced the general availability of Amazon EC2 Trn1 instances powered by AWS Trainium—a purpose-built machine learning (ML) accelerator optimized to provide a high-performance, cost-effective, and massively scalable platform for training deep learning models in the cloud. Trn1 instances are available in a number of sizes (see the following table), with up to 16 Trainium accelerators per instance.
Instance Size |
Trainium Accelerators |
Accelerator Memory (GB) |
vCPUs |
Instance Memory (GiB) |
Network Bandwidth (Gbps) |
trn1.2xlarge |
1 |
32 |
8 |
32 |
Up to 12.5 |
trn1.32xlarge |
16 |
512 |
128 |
512 |
800 |
trn1n.32xlarge (coming soon) |
16 |
512 |
128 |
512 |
1600 |
Trn1 instances can either be deployed as standalone instances for smaller training jobs, or in highly scalable ultraclusters that support distributed training across tens of thousands of Trainium accelerators. All Trn1 instances support the standalone configuration, whereas Trn1 ultraclusters require trn1.32xlarge or trn1n.32xlarge instances. In an ultracluster, multiple Trn1 instances are co-located in a given AWS Availability Zone and are connected with high-speed, low-latency, Elastic Fabric Adapter (EFA) networking that provides 800 Gbps of nonblocking network bandwidth per instance for collective compute operations. The trn1n.32xlarge instance type, launching in early 2023, will increase this bandwidth to 1600 Gbps per instance.
Many enterprise customers choose to deploy their deep learning workloads using Kubernetes—the de facto standard for container orchestration in the cloud. AWS customers often deploy these workloads using Amazon Elastic Kubernetes Service (Amazon EKS). Amazon EKS is a managed Kubernetes service that simplifies the creation, configuration, lifecycle, and monitoring of Kubernetes clusters while still offering the full flexibility of upstream Kubernetes.
Today, we are excited to announce official support for distributed training jobs using Amazon EKS and EC2 Trn1 instances. With this announcement, you can now easily run large-scale containerized training jobs within Amazon EKS while taking full advantage of the price-performance, scalability, and ease of use offered by Trn1 instances.
Along with this announcement, we are also publishing a detailed tutorial that guides you through the steps required to run a multi-instance distributed training job (BERT phase 1 pre-training) using Amazon EKS and Trn1 instances. In this post, you will learn about the solution architecture and review several key steps from the tutorial. Refer to the official tutorial repository for the complete end-to-end workflow.
To follow along, a broad familiarity with core AWS services such as Amazon Elastic Compute Cloud (Amazon EC2) and Amazon EKS is implied, and basic familiarity with deep learning and PyTorch would be helpful.
Solution architecture
The following diagram illustrates the solution architecture.
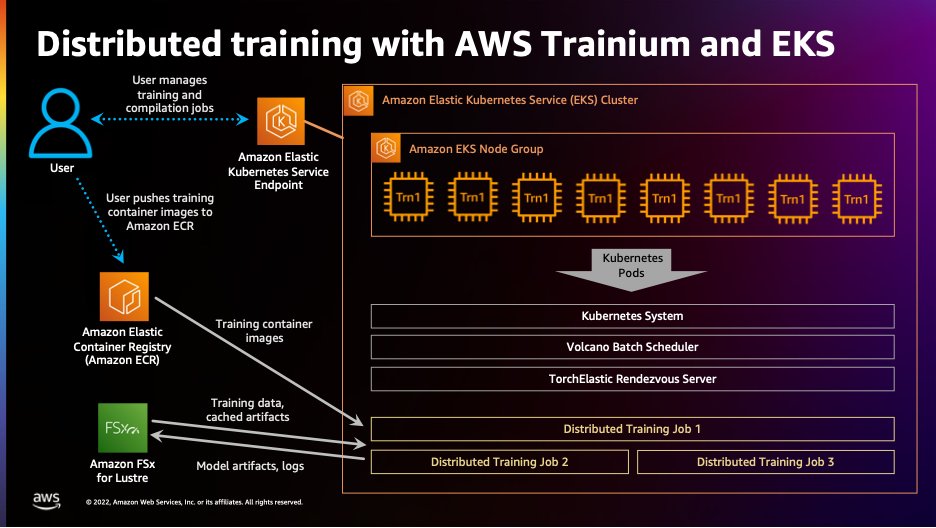
The solution consists of the following main components:
- An EKS cluster
- An EKS node group consisting of trn1.32xlarge instances
- The AWS Neuron SDK
- EKS plugins for Neuron and EFA
- An Amazon Elastic Container Registry (Amazon ECR) Rrepository
- A training container image
- An Amazon FSx for Lustre file system
- A Volcano batch scheduler and etcd server
- The TorchX universal job launcher
- The TorchX DDP module for Trainium
At the heart of the solution is an EKS cluster that provides you with core Kubernetes management functionality via an EKS service endpoint. One of the benefits of Amazon EKS is that the service actively monitors and scales the control plane based on load, which ensures high performance for large workloads such as distributed training. Inside the EKS cluster is a node group consisting of two or more trn1.32xlarge Trainium-based instances residing in the same Availability Zone.
The Neuron SDK is the software stack that provides the driver, compiler, runtime, framework integration (for example, PyTorch Neuron), and user tools that allow you to access the benefits of the Trainium accelerators. The Neuron device driver runs directly on the EKS nodes (Trn1 instances) and provides access to the Trainium chips from within the training containers that are launched on the nodes. Neuron and EFA plugins are installed within the EKS cluster to provide access to the Trainium chips and EFA networking devices required for distributed training.
An ECR repository is used to store the training container images. These images contain the Neuron SDK (excluding the Neuron driver, which runs directly on the Trn1 instances), PyTorch training script, and required dependencies. When a training job is launched on the EKS cluster, the container images are first pulled from Amazon ECR onto the EKS nodes, and the PyTorch worker containers are then instantiated from the images.
Shared storage is provided using a high-performance FSx for Lustre file system that exists in the same Availability Zone as the trn1.32xlarge instances. Creation and attachment of the FSx for Lustre file system to the EKS cluster is mediated by the Amazon FSx for Lustre CSI driver. In this solution, the shared storage is used to store the training dataset and any logs or artifacts created during the training process.
The solution uses the TorchX universal job launcher to launch distributed training jobs within Amazon EKS. TorchX has two important dependencies: the Volcano batch scheduler and the etcd server. Volcano handles the scheduling and queuing of training jobs, while the etcd server is a key-value store used by TorchElastic for synchronization and peer discovery during job startup.
When a training job is launched using TorchX, the launch command uses the provided TorchX distributed DDP module for Trainium to configure the overall training job and then run the appropriate torchrun commands on each of the PyTorch worker pods. When a job is running, it can be monitored using standard Kubernetes tools (such as kubectl) or via standard ML toolsets such as TensorBoard.
Solution overview
Let’s look at the important steps of this solution. Throughout this overview, we refer to the Launch a Multi-Node PyTorch Neuron Training Job on Trainium Using TorchX and EKS tutorial on GitHub.
Create an EKS cluster
To get started with distributed training jobs in Amazon EKS with Trn1 instances, you first create an EKS cluster as outlined in the tutorial on GitHub. Cluster creation can be achieved using standard tools such as eksctl
and AWS CloudFormation.
Create an EKS node group
Next, we need to create an EKS node group containing two or more trn1.32xlarge instances in a supported Region. In the tutorial, AWS CloudFormation is used to create a Trainium-specific EC2 launch template, which ensures that the Trn1 instances are launched with an appropriate Amazon Machine Image (AMI) and the correct EFA network configuration needed to support distributed training. The AMI also includes the Neuron device driver that provides support for the Trainium accelerator chips. With the eksctl
Amazon EKS management tool, you can easily create a Trainium node group using a basic YAML manifest that references the newly created launch template. For example:
apiVersion: eksctl.io/v1alpha5
kind: ClusterConfig
metadata:
name: my-trn1-cluster
region: us-west-2
version: "1.23"
iam:
withOIDC: true
availabilityZones: ["us-west-xx","us-west-yy"]
managedNodeGroups:
- name: trn1-ng1
launchTemplate:
id: TRN1_LAUNCH_TEMPLATE_ID
minSize: 2
desiredCapacity: 2
maxSize: 2
availabilityZones: ["us-west-xx"]
privateNetworking: true
efaEnabled: true
In the preceding manifest, several attributes are configured to allow for the use of Trn1 instances in the EKS cluster. First, metadata.region
is set to one of the Regions that supports Trn1 instances (currently us-east-1
and us-west-2
). Next, for availabilityZones, Amazon EKS requires that two Availability Zones be specified. One of these Availability Zones must support the use of Trn1 instances, while the other can be chosen at random. The tutorial shows how to determine which Availability Zones will allow for Trn1 instances within your AWS account. The same Trn1-supporting Availability Zone must also be specified using the availabiltyZones
attribute associated with the EKS node group. efaEnabled
is set to true
to configure the nodes with the appropriate EFA network configuration that is required for distributed training. Lastly, the launchTemplate.id
attribute associated with the node group points to the EC2 launch template created via AWS CloudFormation in an earlier step.
Assuming that you have already applied the CloudFormation template and installed the eksctl
management tool, you can create a Trainium-capable EKS node group by running the following code:
> eksctl create nodegroup -f TEMPLATE.yaml
Install Kubernetes plugins for Trainium and EFA devices
With the node group in place, the next step is to install Kubernetes plugins that provide support for the Trainium accelerators (via the Neuron plugin) and the EFA devices (via the EFA plugin). These plugins can easily be installed on the cluster using the standard kubectl
management tool as shown in the tutorial.
To use the TorchX universal PyTorch launcher to launch distributed training jobs, two prerequisites are required: the Volcano batch scheduler, and the etcd server. Much like the Neuron and EFA plugins, we can use the kubectl
tool to install Volcano and the etcd server on the EKS cluster.
Attach shared storage to the EKS cluster
In the tutorial, FSx for Lustre is used to provide a high-performance shared file system that can be accessed by the various EKS worker pods. This shared storage is used to host the training dataset, as well as any artifacts and logs creating during the training process. The tutorial describes how to create and attach the shared storage to the cluster using the Amazon FSx for Lustre CSI driver.
Create a training container image
Next, we need to create a training container image that includes the PyTorch training script along with any dependencies. An example Dockerfile is included in the tutorial, which incorporates the BERT pre-training script along with its software dependencies. The Dockerfile is used to build the training container image, and the image is then pushed to an ECR repository from which the PyTorch workers are able to pull the image when a training job is launched on the cluster.
Set up the training data
Before launching a training job, the training data is first copied to the shared storage volume on FSx for Lustre. The tutorial outlines how to create a temporary Kubernetes pod that has access to the shared storage volume, and shows how to log in to the pod in order to download and extract the training dataset using standard Linux shell commands.
With the various infrastructure and software prerequisites in place, we can now focus on the Trainium aspects of the solution.
Precompile your model
The Neuron SDK supports PyTorch through an integration layer called PyTorch Neuron. By default, PyTorch Neuron operates with just-in-time compilation, where the various neural network compute graphs within a training job are compiled as they are encountered during the training process. For larger models, it can be more convenient to use the provided neuron_parallel_compile
tool to precompile and cache the various compute graphs in advance so as to avoid graph compilation at training time. Before launching the training job on the EKS cluster, the tutorial shows how to first launch a precompilation job via TorchX using the neuron_parallel_compile
tool. Upon completion of the precompilation job, the Neuron compiler will have identified and compiled all of the neural network compute graphs, and cached them to the shared storage volume for later use during the actual BERT pre-training job.
Launch the distributed training job
With precompilation complete, TorchX is then used to launch a 64-worker distributed training job across two trn1.32xlarge instances, with 32 workers per instance. We use 32 workers per instance because each trn1.32xlarge instance contains 16 Trainium accelerators, with each accelerator providing 2 NeuronCores. Each NeuronCore can be accessed as a unique PyTorch XLA device in the training script. An example TorchX launch command from the tutorial looks like the following code:
torchx run
-s kubernetes --workspace="file:///$PWD/docker"
-cfg queue=test,image_repo=$ECR_REPO
lib/trn1_dist_ddp.py:generateAppDef
--name berttrain
--script_args "--batch_size 16 --grad_accum_usteps 32
--data_dir /data/bert_pretrain_wikicorpus_tokenized_hdf5_seqlen128
--output_dir /data/output"
--nnodes 2
--nproc_per_node 32
--image $ECR_REPO:bert_pretrain
--script dp_bert_large_hf_pretrain_hdf5.py
--bf16 True
--cacheset bert-large
The various command line arguments in the preceding TorchX command are described in detail in the tutorial. However, the following arguments are most important in configuring the training job:
- -cfg queue=test – Specifies the Volcano queue to be used for the training job
- -cfg image_repo – Specifies the ECR repository to be used for the TorchX container images
- –script_args – Specifies any arguments that should be passed to the PyTorch training script
- –nnodes and –nproc_per_node – The number of instances and workers per instance to use for the training job
- –script – The name of the PyTorch training script to launch within the training container
- –image – The path to the training container image in Amazon ECR
- –bf16 – Whether or not to enable BF16 data type
Monitor the training job
After the training job has been launched, there are various ways in which the job can be monitored. The tutorial shows how to monitor basic training script metrics on the command line using kubectl
, how to visually monitor training script progress in TensorBoard (see the following screenshot), and how to monitor Trainium accelerator utilization using the neuron-top
tool from the Neuron SDK.
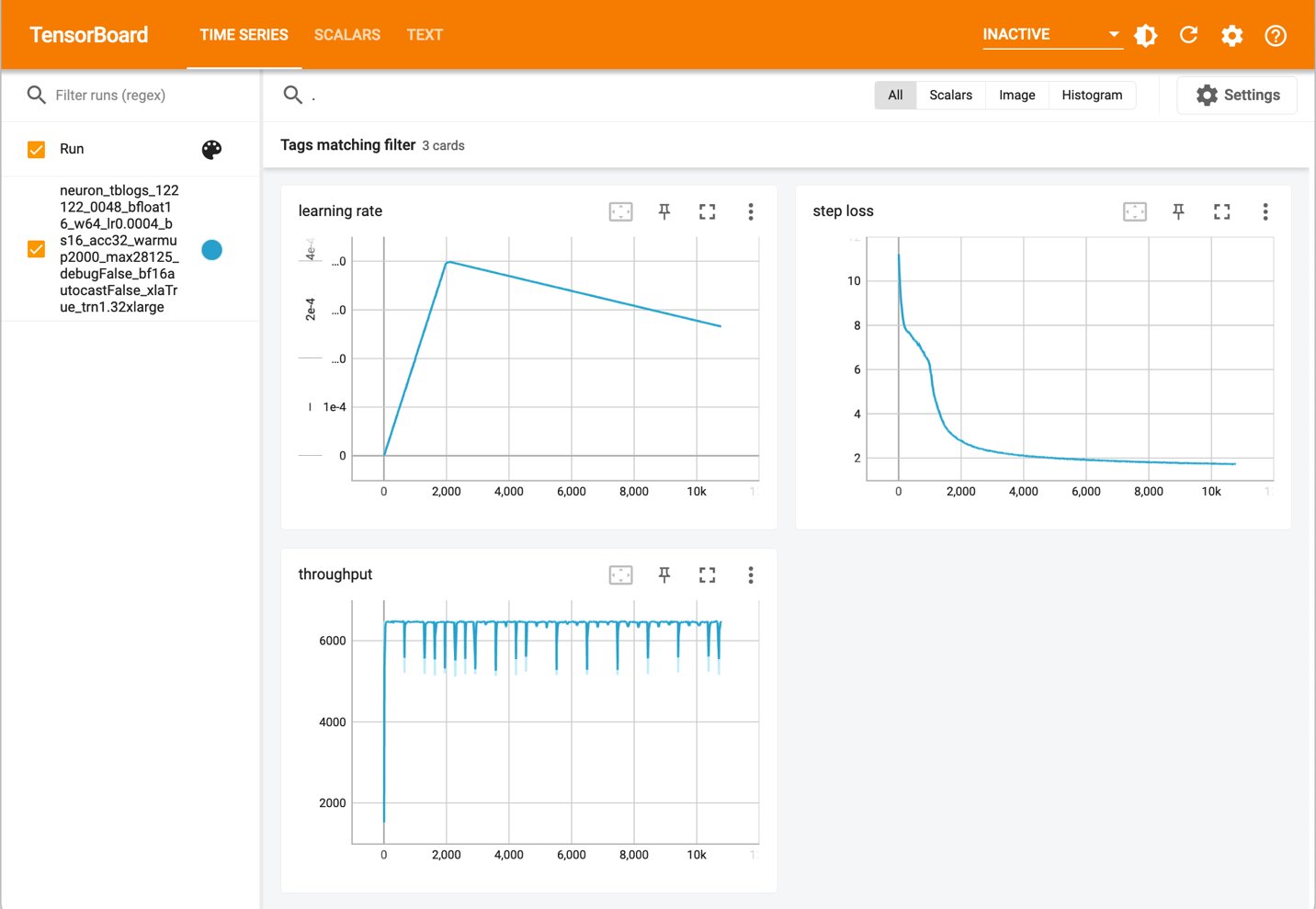
Clean up or reuse the environment
When the training job is complete, the cluster can then be reused or re-configured for additional training jobs. For example, the EKS node group can quickly be scaled up using the eksctl
command in order to support training jobs that require additional Trn1 instances. Similarly, the provided Dockerfile and TorchX launch commands can easily be modified to support additional deep learning models and distributing training topologies.
If the cluster in no longer required, the tutorial also includes all steps required to remove the EKS infrastructure and related resources.
Conclusion
In this post, we explored how Trn1 instances and Amazon EKS provide a managed platform for high-performance, cost-effective, and massively scalable distributed training of deep learning models. We also shared a comprehensive tutorial showing how to run a real-world multi-instance distributed training job in Amazon EKS using Trn1 instances, and highlighted several of the key steps and components in the solution. This tutorial content can easily be adapted for other models and workloads, and provides you with a foundational solution for distributed training of deep learning models in AWS.
To learn more about how to get started with Trainium-powered Trn1 instances, refer to the Neuron documentation.
About the Authors
Scott Perry is a Solutions Architect on the Annapurna ML accelerator team at AWS. Based in Canada, he helps customers deploy and optimize deep learning training and inference workloads using AWS Inferentia and AWS Trainium. His interests include large language models, deep reinforcement learning, IoT, and genomics.
Lorea Arrizabalaga is a Solutions Architect aligned to the UK Public Sector, where she helps customers design ML solutions with Amazon SageMaker. She is also part of the Technical Field Community dedicated to hardware acceleration and helps with testing and benchmarking AWS Inferentia and AWS Trainium workloads.
Read More