By leveraging neural vocoding, Amazon Chime SDK’s new deep-redundancy (DRED) technology can reconstruct long sequences of lost packets with little bandwidth overhead.Read More
March 20 ChatGPT outage: Here’s what happened
An update on our findings, the actions we’ve taken, and technical details of the bug.OpenAI Blog
Visual language maps for robot navigation
People are excellent navigators of the physical world, due in part to their remarkable ability to build cognitive maps that form the basis of spatial memory — from localizing landmarks at varying ontological levels (like a book on a shelf in the living room) to determining whether a layout permits navigation from point A to point B. Building robots that are proficient at navigation requires an interconnected understanding of (a) vision and natural language (to associate landmarks or follow instructions), and (b) spatial reasoning (to connect a map representing an environment to the true spatial distribution of objects). While there have been many recent advances in training joint visual-language models on Internet-scale data, figuring out how to best connect them to a spatial representation of the physical world that can be used by robots remains an open research question.
To explore this, we collaborated with researchers at the University of Freiburg and Nuremberg to develop Visual Language Maps (VLMaps), a map representation that directly fuses pre-trained visual-language embeddings into a 3D reconstruction of the environment. VLMaps, which is set to appear at ICRA 2023, is a simple approach that allows robots to (1) index visual landmarks in the map using natural language descriptions, (2) employ Code as Policies to navigate to spatial goals, such as “go in between the sofa and TV” or “move three meters to the right of the chair”, and (3) generate open-vocabulary obstacle maps — allowing multiple robots with different morphologies (mobile manipulators vs. drones, for example) to use the same VLMap for path planning. VLMaps can be used out-of-the-box without additional labeled data or model fine-tuning, and outperforms other zero-shot methods by over 17% on challenging object-goal and spatial-goal navigation tasks in Habitat and Matterport3D. We are also releasing the code used for our experiments along with an interactive simulated robot demo.
VLMaps can be built by fusing pre-trained visual-language embeddings into a 3D reconstruction of the environment. At runtime, a robot can query the VLMap to locate visual landmarks given natural language descriptions, or to build open-vocabulary obstacle maps for path planning. |
Classic 3D maps with a modern multimodal twist
VLMaps combines the geometric structure of classic 3D reconstructions with the expression of modern visual-language models pre-trained on Internet-scale data. As the robot moves around, VLMaps uses a pre-trained visual-language model to compute dense per-pixel embeddings from posed RGB camera views, and integrates them into a large map-sized 3D tensor aligned with an existing 3D reconstruction of the physical world. This representation allows the system to localize landmarks given their natural language descriptions (such as “a book on a shelf in the living room”) by comparing their text embeddings to all locations in the tensor and finding the closest match. Querying these target locations can be used directly as goal coordinates for language-conditioned navigation, as primitive API function calls for Code as Policies to process spatial goals (e.g., code-writing models interpret “in between” as arithmetic between two locations), or to sequence multiple navigation goals for long-horizon instructions.
# move first to the left side of the counter, then move between the sink and the oven, then move back and forth to the sofa and the table twice.
robot.move_to_left('counter')
robot.move_in_between('sink', 'oven')
pos1 = robot.get_pos('sofa')
pos2 = robot.get_pos('table')
for i in range(2):
robot.move_to(pos1)
robot.move_to(pos2)
# move 2 meters north of the laptop, then move 3 meters rightward.
robot.move_north('laptop')
robot.face('laptop')
robot.turn(180)
robot.move_forward(2)
robot.turn(90)
robot.move_forward(3)
VLMaps can be used to return the map coordinates of landmarks given natural language descriptions, which can be wrapped as a primitive API function call for Code as Policies to sequence multiple goals long-horizon navigation instructions. |
Results
We evaluate VLMaps on challenging zero-shot object-goal and spatial-goal navigation tasks in Habitat and Matterport3D, without additional training or fine-tuning. The robot is asked to navigate to four subgoals sequentially specified in natural language. We observe that VLMaps significantly outperforms strong baselines (including CoW and LM-Nav) by up to 17% due to its improved visuo-lingual grounding.
Tasks | Number of subgoals in a row | Independent subgoals |
||||||||
1 | 2 | 3 | 4 | |||||||
LM-Nav | 26 | 4 | 1 | 1 | 26 | |||||
CoW | 42 | 15 | 7 | 3 | 36 | |||||
CLIP MAP | 33 | 8 | 2 | 0 | 30 | |||||
VLMaps (ours) | 59 | 34 | 22 | 15 | 59 | |||||
GT Map | 91 | 78 | 71 | 67 | 85 |
The VLMaps-approach performs favorably over alternative open-vocabulary baselines on multi-object navigation (success rate [%]) and specifically excels on longer-horizon tasks with multiple sub-goals. |
A key advantage of VLMaps is its ability to understand spatial goals, such as “go in between the sofa and TV” or “move three meters to the right of the chair”. Experiments for long-horizon spatial-goal navigation show an improvement by up to 29%. To gain more insights into the regions in the map that are activated for different language queries, we visualize the heatmaps for the object type “chair”.
Open-vocabulary obstacle maps
A single VLMap of the same environment can also be used to build open-vocabulary obstacle maps for path planning. This is done by taking the union of binary-thresholded detection maps over a list of landmark categories that the robot can or cannot traverse (such as “tables”, “chairs”, “walls”, etc.). This is useful since robots with different morphologies may move around in the same environment differently. For example, “tables” are obstacles for a large mobile robot, but may be traversable for a drone. We observe that using VLMaps to create multiple robot-specific obstacle maps improves navigation efficiency by up to 4% (measured in terms of task success rates weighted by path length) over using a single shared obstacle map for each robot. See the paper for more details.
![]() |
Experiments with a mobile robot (LoCoBot) and drone in AI2THOR simulated environments. Left: Top-down view of an environment. Middle columns: Agents’ observations during navigation. Right: Obstacle maps generated for different embodiments with corresponding navigation paths. |
Conclusion
VLMaps takes an initial step towards grounding pre-trained visual-language information onto spatial map representations that can be used by robots for navigation. Experiments in simulated and real environments show that VLMaps can enable language-using robots to (i) index landmarks (or spatial locations relative to them) given their natural language descriptions, and (ii) generate open-vocabulary obstacle maps for path planning. Extending VLMaps to handle more dynamic environments (e.g., with moving people) is an interesting avenue for future work.
Open-source release
We have released the code needed to reproduce our experiments and an interactive simulated robot demo on the project website, which also contains additional videos and code to benchmark agents in simulation.
Acknowledgments
We would like to thank the co-authors of this research: Chenguang Huang and Wolfram Burgard.
Amazon launches AICE Center at UIUC
The center will support UIUC researchers in their development of novel approaches to conversational AI systems.Read More
Enable fully homomorphic encryption with Amazon SageMaker endpoints for secure, real-time inferencing
This is joint post co-written by Leidos and AWS. Leidos is a FORTUNE 500 science and technology solutions leader working to address some of the world’s toughest challenges in the defense, intelligence, homeland security, civil, and healthcare markets.
Leidos has partnered with AWS to develop an approach to privacy-preserving, confidential machine learning (ML) modeling where you build cloud-enabled, encrypted pipelines.
Homomorphic encryption is a new approach to encryption that allows computations and analytical functions to be run on encrypted data, without first having to decrypt it, in order to preserve privacy in cases where you have a policy that states data should never be decrypted. Fully homomorphic encryption (FHE) is the strongest notion of this type of approach, and it allows you to unlock the value of your data where zero-trust is key. The core requirement is that the data needs to be able to be represented with numbers through an encoding technique, which can be applied to numerical, textual, and image-based datasets. Data using FHE is larger in size, so testing must be done for applications that need the inference to be performed in near-real time or with size limitations. It’s also important to phrase all computations as linear equations.
In this post, we show how to activate privacy-preserving ML predictions for the most highly regulated environments. The predictions (inference) use encrypted data and the results are only decrypted by the end consumer (client side).
To demonstrate this, we show an example of customizing an Amazon SageMaker Scikit-learn, open sourced, deep learning container to enable a deployed endpoint to accept client-side encrypted inference requests. Although this example shows how to perform this for inference operations, you can extend the solution to training and other ML steps.
Endpoints are deployed with a couple clicks or lines of code using SageMaker, which simplifies the process for developers and ML experts to build and train ML and deep learning models in the cloud. Models built using SageMaker can then be deployed as real-time endpoints, which is critical for inference workloads where you have real time, steady state, low latency requirements. Applications and services can call the deployed endpoint directly or through a deployed serverless Amazon API Gateway architecture. To learn more about real-time endpoint architectural best practices, refer to Creating a machine learning-powered REST API with Amazon API Gateway mapping templates and Amazon SageMaker. The following figure shows both versions of these patterns.
In both of these patterns, encryption in transit provides confidentiality as the data flows through the services to perform the inference operation. When received by the SageMaker endpoint, the data is generally decrypted to perform the inference operation at runtime, and is inaccessible to any external code and processes. To achieve additional levels of protection, FHE enables the inference operation to generate encrypted results for which the results can be decrypted by a trusted application or client.
More on fully homomorphic encryption
FHE enables systems to perform computations on encrypted data. The resulting computations, when decrypted, are controllably close to those produced without the encryption process. FHE can result in a small mathematical imprecision, similar to a floating point error, due to noise injected into the computation. It’s controlled by selecting appropriate FHE encryption parameters, which is a problem-specific, tuned parameter. For more information, check out the video How would you explain homomorphic encryption?
The following diagram provides an example implementation of an FHE system.
In this system, you or your trusted client can do the following:
- Encrypt the data using a public key FHE scheme. There are a couple of different acceptable schemes; in this example, we’re using the CKKS scheme. To learn more about the FHE public key encryption process we chose, refer to CKKS explained.
- Send client-side encrypted data to a provider or server for processing.
- Perform model inference on encrypted data; with FHE, no decryption is required.
- Encrypted results are returned to the caller and then decrypted to reveal your result using a private key that’s only available to you or your trusted users within the client.
We’ve used the preceding architecture to set up an example using SageMaker endpoints, Pyfhel as an FHE API wrapper simplifying the integration with ML applications, and SEAL as our underlying FHE encryption toolkit.
Solution overview
We’ve built out an example of a scalable FHE pipeline in AWS using an SKLearn logistic regression deep learning container with the Iris dataset. We perform data exploration and feature engineering using a SageMaker notebook, and then perform model training using a SageMaker training job. The resulting model is deployed to a SageMaker real-time endpoint for use by client services, as shown in the following diagram.
In this architecture, only the client application sees unencrypted data. The data processed through the model for inferencing remains encrypted throughout its lifecycle, even at runtime within the processor in the isolated AWS Nitro Enclave. In the following sections, we walk through the code to build this pipeline.
Prerequisites
To follow along, we assume you have launched a SageMaker notebook with an AWS Identity and Access Management (IAM) role with the AmazonSageMakerFullAccess managed policy.
Train the model
The following diagram illustrates the model training workflow.
The following code shows how we first prepare the data for training using SageMaker notebooks by pulling in our training dataset, performing the necessary cleaning operations, and then uploading the data to an Amazon Simple Storage Service (Amazon S3) bucket. At this stage, you may also need to do additional feature engineering of your dataset or integrate with different offline feature stores.
In this example, we’re using script-mode on a natively supported framework within SageMaker (scikit-learn), where we instantiate our default SageMaker SKLearn estimator with a custom training script to handle the encrypted data during inference. To see more information about natively supported frameworks and script mode, refer to Use Machine Learning Frameworks, Python, and R with Amazon SageMaker.
Finally, we train our model on the dataset and deploy our trained model to the instance type of our choice.
At this point, we’ve trained a custom SKLearn FHE model and deployed it to a SageMaker real-time inference endpoint that’s ready accept encrypted data.
Encrypt and send client data
The following diagram illustrates the workflow of encrypting and sending client data to the model.
In most cases, the payload of the call to the inference endpoint contains the encrypted data rather than storing it in Amazon S3 first. We do this in this example because we’ve batched a large number of records to the inference call together. In practice, this batch size will be smaller or batch transform will be used instead. Using Amazon S3 as an intermediary isn’t required for FHE.
Now that the inference endpoint has been set up, we can start sending data over. We normally use different test and training datasets, but for this example we use the same training dataset.
First, we load the Iris dataset on the client side. Next, we set up the FHE context using Pyfhel. We selected Pyfhel for this process because it’s simple to install and work with, includes popular FHE schemas, and relies upon trusted underlying open-sourced encryption implementation SEAL. In this example, we send the encrypted data, along with public keys information for this FHE scheme, to the server, which enables the endpoint to encrypt the result to send on its side with the necessary FHE parameters, but doesn’t give it the ability to decrypt the incoming data. The private key remains only with the client, which has the ability to decrypt the results.
After we encrypt our data, we put together a complete data dictionary—including the relevant keys and encrypted data—to be stored on Amazon S3. Aferwards, the model makes its predictions over the encrypted data from the client, as shown in the following code. Notice we don’t transmit the private key, so the model host isn’t able to decrypt the data. In this example, we’re passing the data through as an S3 object; alternatively, that data may be sent directly to the Sagemaker endpoint. As a real-time endpoint, the payload contains the data parameter in the body of the request, which is mentioned in the SageMaker documentation.
The following screenshot shows the central prediction within fhe_train.py
(the appendix shows the entire training script).
We’re computing the results of our encrypted logistic regression. This code computes an encrypted scalar product for each possible class and returns the results to the client. The results are the predicted logits for each class across all examples.
Client returns decrypted results
The following diagram illustrates the workflow of the client retrieving their encrypted result and decrypting it (with the private key that only they have access to) to reveal the inference result.
In this example, results are stored on Amazon S3, but generally this would be returned through the payload of the real-time endpoint. Using Amazon S3 as an intermediary isn’t required for FHE.
The inference result will be controllably close to the results as if they had computed it themselves, without using FHE.
Clean up
We end this process by deleting the endpoint we created, to make sure there isn’t any unused compute after this process.
Results and considerations
One of the common drawbacks of using FHE on top of models is that it adds computational overhead, which—in practice—makes the resulting model too slow for interactive use cases. But, in cases where the data is highly sensitive, it might be worthwhile to accept this latency trade-off. However, for our simple logistic regression, we are able to process 140 input data samples within 60 seconds and see linear performance. The following chart includes the total end-to-end time, including the time performed by the client to encrypt the input and decypt the results. It also uses Amazon S3, which adds latency and isn’t required for these cases.
We see linear scaling as we increase the number of examples from 1 to 150. This is expected because each example is encrypted independently from each other, so we expect a linear increase in computation, with a fixed setup cost.
This also means that you can scale your inference fleet horizontally for greater request throughput behind your SageMaker endpoint. You can use Amazon SageMaker Inference Recommender to cost optimize your fleet depending on your business needs.
Conclusion
And there you have it: fully homomorphic encryption ML for a SKLearn logistic regression model that you can set up with a few lines of code. With some customization, you can implement this same encryption process for different model types and frameworks, independent of the training data.
If you’d like to learn more about building an ML solution that uses homomorphic encryption, reach out to your AWS account team or partner, Leidos, to learn more. You can also refer to the following resources for more examples:
- Capabilities on the Leidos website.
- The AWS re:Invent 2020 breakout session Privacy-preserving maching learning, by Joan Feidenbaim, Amazon Scholar with the AWS Crytographic Algorithms Group. In this session, Feidenbaim describes two prototypes that were built in 2020.
- Amazon Science articles related to homomorphic encryption.
- What is cryptographic computing? A conversation with two AWS experts, featuring Joan Feigenbaum, Amazon Scholar, AWS Cryptography, and Bill Horne, Principal Product Manager, AWS Cryptography.
The content and opinions in this post contains those from third-party authors and AWS is not responsible for the content or accuracy of this post.
Appendix
The full training script is as follows:
About the Authors
Liv d’Aliberti is a researcher within the Leidos AI/ML Accelerator under the Office of Technology. Their research focuses on privacy-preserving machine learning.
Manbir Gulati is a researcher within the Leidos AI/ML Accelerator under the Office of Technology. His research focuses on the intersection of cybersecurity and emerging AI threats.
Joe Kovba is a Cloud Center of Excellence Practice Lead within the Leidos Digital Modernization Accelerator under the Office of Technology. In his free time, he enjoys refereeing football games and playing softball.
Ben Snively is a Public Sector Specialist Solutions Architect. He works with government, non-profit, and education customers on big data and analytical projects, helping them build solutions using AWS. In his spare time, he adds IoT sensors throughout his house and runs analytics on them.
Sami Hoda is a Senior Solutions Architect in the Partners Consulting division covering the Worldwide Public Sector. Sami is passionate about projects where equal parts design thinking, innovation, and emotional intelligence can be used to solve problems for and impact people in need.
AI Explainer: Foundation models and the next era of AI
The release of OpenAI’s GPT-4 is a significant advance that builds on several years of rapid innovation in foundation models. GPT-4, which was trained on the Microsoft Azure AI supercomputer, has exhibited significantly improved abilities across many dimensions—from summarizing lengthy documents, to answering complex questions about a wide range of topics and explaining the reasoning behind those answers, to telling jokes and writing code and poetry.
Microsoft Senior Principal Research Manager Ahmed H. Awadallah was among a group of researchers across the company who have worked in partnership with OpenAI over several months to evaluate this new model’s capabilities. In this video, recapped below, he tells the story of the technical innovations in recent years that have brought us to this moment: the surprising progress of GPT-4’s predecessor models, leading up to the capabilities demonstrated in ChatGPT, and the integration of the latest models into Bing.
Introduction to foundation models [00:00-11:01]
Over the last decade, AI has made significant progress on perception tasks like image recognition and language processing. More recently, the field is witnessing new advances in the form of generative AI, underpinned by a class of large-scale models known as foundation models. Foundation models are trained on massive amounts of data and are capable of performing a wide range of tasks. With a simple natural language prompt like “describe a scene of the sun rising over the beach,” generative AI models can output a detailed description or produce an image based on the generated description, which can then be animated or even turned into video. Many recent language models are not only good at generating text but also generating, explaining, and debugging code.
Three components have been driving these advances:
- The transformer architecture: A popular choice across modalities, the transformer architecture is efficient, easy to scale and parallelize, and can model interdependence between different components in input and output data.
- Scale: Growing model size and the use of increasingly large amounts of data have resulted in what is being termed as “emerging capabilities.” When models reach a critical size, they begin displaying capabilities not previously present.
- In-context learning: Showing potential on a range of applications, from text classification to translation and summarization, this new training paradigm provides pre-trained models with instructions for new tasks or just a few examples instead of training or fine-tuning models on labeled data. Because no additional data or training is needed and prompts are provided in natural language, models can be applied right out of the box and aren’t limited to those with developer experience.
From GPT-3 to ChatGPT – a jump in generative capabilities [11:02-19:07]
With the November 2022 release of ChatGPT, a language model optimized for dialogue, we saw exciting developments in text generation. Compared with GPT-3, an earlier language model in the GPT family, ChatGPT not only provides longer, more thorough, and more structured responses to questions and instructions but can also produce answers in different styles, or tones, and tailor explanations to different audiences, like a child, a first-year college student, or someone with a PhD.
Earlier language models such as GPT-3 were trained to predict the next word in a sentence using large amounts of text from the web with no direct human supervision. Several additional training approaches have helped fuel the improved performance of later models such as ChatGPT. These models are being trained on code in addition to text, which seems to be providing another opportunity to identify the relationship between different parts of speech. This is resulting in models that are better at following instructions and reasoning than models trained on text alone. Human-generated data is also contributing to better outputs. Instruction tuning adds the step of training models on prompts and responses created by a human, while model-generated responses ranked by a human are being employed to train a reward model that can be used to train the main model with reinforcement learning.
The fast-paced advancements demonstrated by these models have challenged one of the traditional methods used to measure progress: benchmarks. Improvements are happening so fast that benchmarks are becoming obsolete, with many solved or saturated as quickly as they come out.
Everyday impact: Integrating foundation models and products [19:09-27:20]
Foundation models are already appearing in products available today. For example, GitHub Copilot leverages OpenAI Codex to assist in writing code. The AI pair programmer has been shown to not only make developers feel more productive but to support them in actually getting more done. A GitHub study found participants using Copilot were 55 percent more productive than participants without access to Copilot.
Combining language models optimized for dialogue with external knowledge sources and tools is another avenue for improved experiences. The new Bing, for instance, brings together these models and search. Years of research have yielded insight into the web search experience; much of it involves reviewing and synthesizing information across a variety of resources identified via multiple queries, which is time-consuming. The new Bing can do the heavy lifting for the searcher, working behind the scenes to make the necessary queries, collect results, synthesize the information, and present a single complete answer.
Large language models and foundation models more broadly are not without their limitations, however. There are issues such as reliability, accuracy, staleness, and provenance that need to be explored. Additionally, each specific application of one of these models comes with its own challenges and opportunities. For example, in applying foundation models to web search, we need to rethink the overall user experience, including how people interact with search and how we improve, measure, and personalize the experience over time.
Transcript
Introduction to foundation models [00:00–11:01]
Hello, everyone. My name is Ahmed Awadallah. I am a researcher here at Microsoft Research. Today, I am going to be talking about foundation models and the impact they are having on the current era of AI.
If we look back at the last five to 10 years, AI has been making significant impact on many perception tasks like image and object recognition, speech recognition, and most recently on language understanding tasks, where we have been seeing different AI models achieving superior performance and in many cases reaching performance equal to what a human annotator would do on the same task. Over the last couple of years, though, the frontier of AI has changed toward generative AI.
We have had quite good text generation models for some time. You could actually prompt a model with asking it to describe an imaginary scene, and it will produce a very good description of what you have asked it to do. And then we started making a lot of progress on image generation, as well. With models like DALL-E 2 and Imagen and even models coming out from such startups like Midjourney and Stability AI, we have been getting to a level of quality of image generation that we have never seen before. Inspired by that, there has been also a lot of work on animating the generated images or even generating videos from scratch. Another frontier for generative models has been code, and not only generating code based on text prompt but also explaining the code or in some cases even debugging the code. I was listening to this episode of the Morning Edition on NPR when it aired at the beginning of February where they were attempting to use a bunch of AI models for producing a schematic design of a rocket and also for coming up with some equations for the rocket design. And, of course, the hypothetical design would have crashed and burned, but I couldn’t help but think how exciting it is that AI has become so good that we are even attempting to measure its proficiency on a field as complex as rocket science.
[2:11] If we look back, we will find that there are three main components that led to the current performance we are seeing from AI models: the transformer architecture, the scale, and in-context learning. Transformer in particular has been dominating the field of AI for the previous years. At the beginning, we started with natural language processing, and the architecture was very efficient that it took over the field of natural language processing within a very short amount of time. The transformer is a very efficient architecture that’s easy to scale, easy to parallelize, and relies on its heart at the attention mechanism, a technique that allows us to model interdependence between different components or different tokens in our input and output data. Transformers started off mostly in natural language processing, but slowly but surely, they made their way to pretty much any modality. So now we are seeing that models that are operating on images, on videos, on audio, and many other modalities are also using transformers. Five years later since their inception and transformers have surprisingly changed little compared to when they started despite so many attempts at producing better and more efficient variants of transformers, perhaps because of the gains were limited to certain use cases or perhaps because the gains did not persist at scale. Another potential reason is that maybe they made the architecture less universal, which has been one of its more—of its biggest advantages.
[03:53] The next point is scale, and when we talk about scale, we really mean the amount of compute that’s being used to train the model, and that can be translated into either training bigger and bigger models with larger and larger number of parameters—and we have been seeing a steady increase of that over the previous years—but scale could also mean more data, using more data to train the model on larger and larger amounts of data. And we have seen different models over the previous few years taking different approaches in deciding how much data and how large the model is. But the consistent trend is that we have been scaling larger and larger and using more and more compute. Scale has also led to what is being called as “emerging capabilities.” And that’s one of the most interesting properties of scale that have been described over the previous year or so. By emerging capability, we mean that the model starts to show a certain ability that appears only when it reaches a critical size. Before that, the model is not demonstrating any of this ability at all. For example, let’s look at the figures here, and on the left-hand side, we see arithmetic. If we try to use language models to solve arithmetic word problems, up until a certain scale, they absolutely cannot solve the problem in any way, and they do not perform any better than random. But then at a certain critical point, we start seeing improved performance, and that performance just keeps getting better and better. And we have seen that at so many other tasks, as well, ranging from arithmetic to transliteration to multitask learning.
[05:38] And perhaps one of the most exciting emerging capabilities of language models recently is their ability to in-context learn, which has been introducing a new paradigm for using these models. If we take a look back at how we have been practicing machine learning in general, with deep learning, you would start by choosing an architecture, a transformer or before that an RNN or CNN, and then you fully supervise train your model. You have a lot of labeled data, and you train your model based on that data. When we started getting into pre-trained models, we instead of training models from scratch, we actually start off with a pre-trained model and then fine-tune it still on a lot of fully supervised labeled data for the task at hand. But then with in-context learning, suddenly we can actually use the models out of the box. We can just use a pre-trained model and use a prompt in order to learn—in order to perform a new task without actually doing any learning. We can do that in zero-shot settings, meaning we do not provide any examples at all, just instructions or a description of what the task is, or in a few-shot setting, where we just provide a small handful number of examples to the model. For example, if we are interested in trying to do text classification, we can just—in this case sentiment analysis—we can just provide the text to the model and ask it to classify the text into either positive or negative. If the task is a little bit harder, we can provide few-shot samples, just a few examples of how do we want the model to classify things into, say, positive, negative, or neutral, and then ask the model to reason about a new piece of text, and it actually does pretty good at it. And it’s not only simple tasks like text classification. We can do translation or summarization and much more complex tasks with that paradigm. We can even try to do things like arithmetic where we try to give the model a word problem and ask it to come up with the answer. On the example we are showing right now, we did give the model just one sample to show it how we would solve a problem and then ask it to solve another problem. But in that particular case, the model actually failed. It did produce an answer, but it was not the correct answer. But then came the idea of chain-of-thought prompts, where instead of just showing the model the input and the output, we can actually also show it the steps it can take in order to get to that output from that particular input. In that case, we are just solving the arithmetic word problem step by step and showing an example of that to the model. When we do that, the models are not only able to produce the correct answer, but they are also able to walk us step by step through how they produced that answer. That mechanism is referred to as a chain-of-thought prompting, and it has been very prominently used in so many tasks and showing very superior performance on multiple tasks. It has been also used in many different ways, including in fine-tuning and training some of the models. The “pre-train and then fine-tune” paradigm have been established paradigm for years, since maybe the inception of BERT and similar pre-trained language models. But now you would see that there’s increased shift into using the models by prompting them instead of having to fine-tune them. That’s evident in a lot of practical usage of the models but even in the publications in the machine learning areas that have been using natural language processing tasks and switching into using prompting instead of using fine-tuning. In-context learning and prompting matters a lot because it’s actually changing the way we apply the models to new tasks. The ability of applying the models to new tasks out of the box without collecting additional data, without doing any additional training, is an amazing ability that increases the amount of tasks that can be applied—the models can be applied to and also reduces the amount of effort needed into building models with these tasks.
[09:57] The performance has been also amazing by just providing only a few examples, and the tasks in this setting are being adapted to the model rather than the models being adapted to the tasks. If you think about the fine-tuning paradigm, what we did is that we already had the pre-trained model and we were fine-tuning it to adapt to the task. Now we are trying to frame the task in a way that’s more friendly to how the model is being trained so that the model can perform well on the task even without any fine-tuning. Finally, this allows the humans to interact with the models in their normal form of communication, in natural language. We can just give instructions describing the task that we want, and the model would perform the task. And that blurs the line between who is an ML user and who is an ML developer because now anyone can just prompt and describe different tasks to the language model and get the language model to do a large number of tasks without having to have any training or any development involved.
From GPT-3 to ChatGPT—a jump in generative capabilities [11:02–19:07]
[11:02] Now looking back at the last three months or so, we have been seeing the field changing quite a bit and a tremendous amount of excitement happening around the release of the ChatGPT model. And if we think about the ChatGPT model as a generative model, we would see that there has been other generative models out there from the GPT family and other models, as well, that have been doing a decent job at text generation. So you can take one of these models, in this case GPT-3, and prompt it to the question asking it to explain what the foundational language model means and it would give you a pretty decent answer. You can ask the same question to ChatGPT and you’ll find that it’s able to provide a much better answer. It’s longer; it’s more thorough; it’s more structured. You can ask it to style it in different ways. You can ask it to simplify it in different ways. And all of these are capabilities that the previous generation of the models could not really do. If we look at how ChatGPT is described, the description lists different things, but it’s mostly optimized for dialogue, allowing the humans to interact in natural language. It’s much better at following instructions and so on and so forth. If we look at step by step about how this actually was manifested in the training, we will see from the description that looking at base models that ChatGPT was built on and other models before ChatGPT, that language model training was following a self-supervised pre-training approach, where we have a lot of unsupervised language, web-scale language, that we are training the models on, and the models in this particular case are trained with an autoregressive next word prediction approach. So we are looking at an input context, which is a sentence or a part of a sentence, and trying to predict the next word. But then over the last year or so, we have been seeing a shift where models are being trained not just on text but also on code. For example, GPT-3.5 models are trained on both text and code, and surprisingly, training the models on both text and codes improves their performance on many tasks that has nothing to do with code. On the figure we see right now, we see different models being compared on—models that were trained with code and models that were not trained with code—and we are seeing that the models that were trained with both text and code show better performance at following task instructions, show better performance at reasoning, compared to similar models that were trained on text only. So the training on code seems to be grounding the models in different ways, allowing them to learn a little bit more about how to reason, about how to look at structured relation between different parts of the text.
[13:59] The second main difference is the idea of instruction tuning, which has been—what you have been seeing becoming more and more popular over different models over the last year, maybe starting with InstructGPT that introduced the idea of training the models on human-generated data. And this is a departure from the traditional self-supervised approach, where we have been only training the model on unsupervised, free, unstructured text. Now there’s an additional step in the training process that actually trains the models on human-generated data. The human-generated data takes the format of prompt and the response, and it’s trying to teach the model to respond in a particular way given a prompt, and this step of instruction tuning has been actually helping the models get a lot better, especially in zero-shot performance. And we see here that the instruction-tuned models tend to perform a lot better than their non-instruction–tuned counterpart, especially in zero-shot settings. And the last step of the training process introduces yet another human-generated data. In this case, we actually have different responses generated by the model and we have a human providing preferences to all these responses so in a sense ranking responses and choosing which response is better than other responses. This data is used to train a reward model that can then be used to actually train the main model with reinforcement learning. And this approach further aligns the model into responding in certain ways that correspond to the way the human has been providing the feedback data. This notion of training the model with human feedback data is very interesting, and it’s creating a lot of traction with many people thinking about the best technique to train on human feedback data, the best form of human feedback to collect, to train the model on, and it would probably help us improve the models even further in the near future.
[16:02] Now with all these advances we have been seeing, the pace of innovation and the acceleration of the advances have been moving so fast that it has been very challenging in so many ways, but perhaps one of the most profound ways it has been challenging with is the notion of benchmarking, that traditionally research in machine learning has been very dependent on using very solid benchmarks on measuring the progress of different approaches. But the pace of innovation has been really challenging that recently. To understand how fast the progress has been, let’s look at this data coming from Hypermind, a forecasting company that uses crowd forecasting and has been doing that—tracking some of the AI benchmarks recently. The first benchmark is Massive Multitask Language Understanding benchmark, a large collection of language understanding tasks. In June of 2021, a forecast was made that in a year, by June 2022, we will get to around 57 performance on this task. But in reality, what happens is that by June 2022, we were at around 67 percent, and a couple of months later, we were at 75 percent, and we keep seeing more and more fast improvements after that. A second task is the MATH task, which is a collection of middle and high school math problems, and here the prediction was that in a year, we will get to around 13 percent. But in reality, we ended up going much more beyond that within one year, and we still see more and more advances happening at a faster-than-ever-expected pace. That rate of improvement is actually resulting in a lot of the benchmarks being saturated really fast.
[17:51] If we look back at benchmarks like MNIST and Switchboard, it took the community 20-plus years in order to fully saturate these benchmarks. And that has been accelerating, accelerating to the point where now we see benchmarks being saturated in a year or less. In fact, many of the benchmarks are becoming obsolete to the point that only 66 percent of machine learning benchmarks have received more than three results at different time points, and many of them are solved or saturated soon after they are being released. And that actually motivated the community to come together with very large efforts to try to design benchmarks that are designed specifically to challenge large language models. In that particular case, with BIG-bench, more than 400 authors from over 100 institutions came together to create it. But even with such an elaborate effort, we are seeing very fast progress, and with large language models and chain-of-thought prompting that we discussed earlier, we are seeing that we are making very fast progress against the hardest tasks in BIG-bench, and in many of them, models are already performing better than humans right now.
Everyday impact: Integrating foundation models and products [19:09–27:20]
[19:09] The foundation models are not only getting better and better at benchmarks, but they are actually changing many products that we use every day. We mentioned code generation earlier, so let’s talk a little bit about Copilot. GitHub Copilot is a new experience that helps developers write code, and Copilot is very interesting in many perspectives. One is how fast it went from the model being created in research to how—to the point it made it as a product generally available in GitHub Copilot but also in how much user value it has been generating. This study that was done by the Copilot GitHub team was looking at quantifying the value these models were providing to developers. And in the first part of the study, they asked different questions to the developers, trying to assess how useful the models are, and we see that 88 percent of the participants reported that they feel like they are much more productive when using Copilot than before, and they reported many other positive implications on their productivity, as well. But perhaps even more interesting, the study did a controlled study where there were two groups of developers trying to solve the same set of tasks. A group of them had access to Copilot, and the other group did not, and interestingly, the group that had access to Copilot not only finished the tasks at a higher success rate but also at a much more efficient rate. Overall, they were 55 percent more productive. Fifty-five percent more productivity in a coding scenario is an amazing progress that a lot of people would have been very surprised to think about a model like Copilot performing so fast with such value.
[21:10] Now beyond code generation and text generation, another frontier where these models are starting to shine is when we start connecting them with external knowledge sources and external tools. Language models that have been optimized for dialogue have amazing language capabilities; they do really good at understanding language, at following instructions. They also do really well at synthesizing and generating answers. They are also conversational in nature and do store knowledge from the training data that they were trained on. But they do have a lot of limitations around reliability, factualness, staleness, access to more recent information that was not part of the training data, provenance, and so on. And that’s why connecting these models to external knowledge sources and tools could be super exciting. Let’s talk about, for example, connecting language models to search as we have seen recently with the new Bing.
[22:14] If we take a look back years ago, there was many, many studies studying web search, studying tasks that people try to complete in web search scenarios. And many of these tasks were deemed as complex search tasks, tasks that are not navigational, as in trying to go to a particular website, or that are not simple informational tasks where you are trying to look up a fact that you can quickly get with one query but more complex tasks that involve multiple queries. Maybe you are planning a travel, maybe you are trying to buy a product, and as part of your research process, there are multifaceted queries that you would like to look at. There has been a lot of research understanding user behavior with such tasks and how prevalent they are and how much time and effort people spend in order to perform them. And they typically involve spending a significant amount of time with the search engine, reading and synthesizing information from different sources with different queries. But with a new experience like the experience Bing is providing, we can actually take one of these queries and provide much more complex long queries to the search engine. And the search engine uses both search and the power of the language model to generate multiple queries, get the results of all of these queries, and synthesize a detailed answer back to the searcher. Not only that, but it can recommend additional searches and additional ways you could interact with the search engine in order to learn more. That has the potential of saving a lot of time and a lot of effort for many searchers in supporting these complex search tasks in a much better way. Not only that, but there are some of these complex search tasks that are multistep in nature, where I would start with one query and then follow up with another query based on the information I get from the first query. Imagine that I am doing this search before the Super Bowl where I am trying to understand some comparisons, stats, between the two quarterbacks that are going to face each other, and I start with that query. What the search engine did in that particular case is that it actually started with a query where it was trying to identify who are the two quarterbacks that are going to be playing in the Super Bowl. And if I have done that as a human, I would have done that. I would have identified the teams and the two quarterbacks, and then maybe I would follow up with another query where I would actually search for the stats of the two quarterbacks I am asking about, and get that and actually synthesize the information maybe from different results and then get to the answer I am looking for. But with the new Bing experience, I can just issue the query and all of that is happening in the background. Different search queries are being generated, submitted to the search engine, recent results are getting collected, and a single answer is being synthesized and displayed, making me as a searcher much more productive and much more efficient.
[25:21] The potential of LLM integrated—large language models integrated with search and other tools is very huge and can add much, much value to so many scenarios. But there are also a lot of challenges and a lot of opportunities and a lot of limitations that needs to be addressed. Reliability and safety are one of them; making the models more accurate; thinking about trust, provenance, and bias. User experience and behavior and how the new experience would affect how the users are interacting with the search engine is another one, with new and different tasks or different user interfaces or even different behavior models. Search has been a very well-studied experience, and we have very good understanding of how users interact with the search engine and very reliable behavior models to predict that. Changing this experience will require a lot of additional study there. Personalization and managing user preferences and search history and so on and so forth has also been a very well-studied field in web search, and with new experiences like that, we have so many opportunities and thinking about things like personalization and user experience again but also evaluation and what do metrics mean. How do we measure user satisfaction? How do we understand good and bad abandonment? Good abandonment as in when people get satisfied with the result but they don’t have to click on anything on the search result page, and bad abandonment being the opposite of that. Thinking about feedback loops, which has been playing a large part in improving search engines, and how can we apply them to new experiences and new scenarios. So while integrating language models with an experience like search and other tools and experiences is very exciting, it’s actually also creating so many opportunities for new research problems or for revisiting previous search problems that we had very good understanding for.
Conclusion [27:21–28:37]
[27:21] To conclude, we have been seeing incredible advancing with AI over the past couple of years. The progress has been accelerating and outpacing expectations in so many ways, and the advances are not only in terms of academic benchmarks and publications, but we are also seeing an explosion of applications that are changing the products that we use every day. However, we are really much closer to the beginning of a new era with AI than we are to the end state of AI capabilities. There are so many opportunities, and we will probably see a lot more advances and even more accelerated progress over the coming month and years. And there are so many challenges that remain and many new opportunities that are arising because of the state of where these models are. It’s a very exciting time for AI, and we are really looking forward to seeing the advances that will happen moving forward and to the applications that will result from these advances and how they will affect every one of us with the products we use every day. Thank you so much.
[END]
Explore more
The post AI Explainer: Foundation models and the next era of AI appeared first on Microsoft Research.
AI Frontiers: The Physics of AI with Sébastien Bubeck

Episode 136 | March 23, 2023
Powerful new large-scale AI models like GPT-4 are showing dramatic improvements in reasoning, problem-solving, and language capabilities. This marks a phase change for artificial intelligence—and a signal of accelerating progress to come.
In this new Microsoft Research Podcast series, AI scientist and engineer Ashley Llorens hosts conversations with his collaborators and colleagues about what these new models—and the models that will come next—mean for our approach to creating, understanding, and deploying AI, its applications in areas such as health care and education, and its potential to benefit humanity.
The first episode features Sébastien Bubeck, who leads the Machine Learning Foundations group at Microsoft Research in Redmond. He and his collaborators conducted an extensive evaluation of GPT-4 while it was in development, and have published their findings in a paper that explores its capabilities and limitations—noting that it shows “sparks” of artificial general intelligence.
Learn more:
- Sparks of Artificial General Intelligence: Early experiments with GPT-4
Publication, March 2023 - AI and Microsoft Research
Learn more about the breadth of AI research at Microsoft - Unveiling Transformers with LEGO: a synthetic reasoning task
Publication, June 2022
Transcript
Ashley Llorens: I’m Ashley Llorens with Microsoft Research. I spent the last 20 years working in AI and machine learning. But I’ve never felt more fortunate to work in the field than at this moment. Just this month, March 2023, OpenAI announced GPT-4, a powerful new large-scale AI model with dramatic improvements in reasoning, problem-solving, and much more. This model, and the models that will come after it, represent a phase change in the decades-long pursuit of artificial intelligence.
In this podcast series, I’ll share conversations with fellow researchers about our initial impressions of GPT-4, the nature of intelligence, and ultimately how innovations like these can have the greatest benefit for humanity.
Today I’m sitting down with Sébastien Bubeck, who leads the Machine Learning Foundations Group at Microsoft Research. In recent months, some of us at Microsoft had the extraordinary privilege of early access to GPT-4. We took the opportunity to dive deep into its remarkable reasoning, problem-solving, and the many other abilities that emerge from the massive scale of GPT-4.
Sébastien and his team took this opportunity to probe the model in new ways to gain insight into the nature of its intelligence. Sébastien and his collaborators have shared some of their observations in the new paper called “Sparks of Artificial General Intelligence: Experiments with an early version of GPT-4.”
Welcome to A.I. Frontiers.
Sébastien, I’m excited for this discussion.
The place that I want to start is with what I call the AI moment. So, what do I mean by that? In my experience, everyone that’s picked up and played with the latest wave of large-scale AI models, whether it’s ChatGPT or the more powerful models coming after, has a moment.
They have a moment where they’re genuinely surprised by what the models are capable of, by the experience of the model, the apparent intelligence of the model. And in my observation, the intensity of the reaction is more or less universal. Although everyone comes at it from their own perspective, it triggers its own unique range of emotions, from awe to skepticism.
So now, I’d love from your perspective, the perspective of a machine learning theorist: what was that moment like for you?
Sébastien Bubeck: That’s a great question to start. So, when we started playing with the model, we did what I think anyone would do. We started to ask mathematical questions, mathematical puzzles. We asked it to give some poetry analysis. Peter Lee did one on Black Thought, which was very intriguing. But every time we were left wondering, okay, but maybe it’s out there on the internet. Maybe it’s just doing some kind of pattern matching and it’s finding a little bit of structure. But this is not real intelligence. It cannot be. How could it be real intelligence when it’s such simple components coming together? So, for me, I think the awestruck moment was one night when I woke up and I turned on my laptop and fired up the Playground.
And I have a three-year-old at home, my daughter, who is a huge fan of unicorns. And I was just wondering, you know what? Let’s ask GPT-4 if it can draw a unicorn. And in my professional life, I play a lot with LaTeX, this programing language for mathematical equations. And in LaTeX there is this subprogramming language called TikZ to draw images using code. And so I just asked it: can you draw a unicorn in TikZ. And it did it so beautifully. It was really amazing. You can render it and you can see the unicorn. And no, it wasn’t a perfect unicorn.
What was amazing is that it drew a unicorn, which was quite abstract. It was really the concept of a unicorn, all the bits and pieces of what makes a unicorn, the horn, the tail, the fur, et cetera. And this is what really struck me at that moment. First of all, there is no unicorn in TikZ online.
I mean, who would draw a unicorn in a mathematical language? This doesn’t make any sense. So, there is no unicorn online. I was pretty sure of that. And then we did further experiments to confirm that. And we’re sure that it really drew the unicorn by itself. But really what struck me is this getting into what is a concept of a unicorn, that there is a head, a horn, the legs, et cetera.
This has been a longstanding challenge for AI research. This has always been the problem with all those AI systems that came before, like the convolutional neural networks that were trained on ImageNet and image datasets and that can recognize whether there is a cat or dog in the image, et cetera. Those neural networks, it was always hard to interpret them. And it was not clear how they were detecting exactly whether there is a cat or dog in particular that was susceptible to these adversarial examples like small perturbations to the input that would completely change the output.
And it was understood that the big issue is that they didn’t really get the concept of a cat or dog. And then suddenly with GPT-4, it was kind of clear to me at that moment that it really understood something. It really understands what is a unicorn. So that was the moment for me.
Ashley Llorens: That’s fascinating. What did you feel in that moment? Does that change your concept of your field of study, your relationship to the field?
Sébastien Bubeck: It really changed a lot of things to me. So first of all, I never thought that I would live to see what I would call a real artificial intelligence. Of course, we’ve been talking about AI for many decades now. And the AI revolution in some sense has been happening for a decade already.
But I would argue that all the systems before were really this narrow intelligence, which does not really rise to the level of what I would call intelligence. Here, we’re really facing something which is much more general and really feels like intelligence. So, at that moment, I felt honestly lucky. I felt lucky that I had early access to this system, that I could be one of the first human beings to play with it.
And I saw that this is really going to change the world dramatically. And selfishly, (it) is going to change my field of study, as you were saying. Now suddenly we can start to attack: what is intelligence, really? We can start to approach this question, which seemed completely out of reach before.
So really deep down inside me, incredible excitement. That’s really what I felt. Then upon reflection, in the next few days, there was also some worry, of course. Clearly things are accelerating dramatically. Not only did I never think that I would live to see a real artificial intelligence, but the timeline that I had in mind ten years ago or 15 years ago when I was a Ph.D. student, I saw maybe by the end of the decade, the 2010s, maybe at that time, we will have a system that can play Go better than humans.
That was my target. And maybe 20 years after that, we will have systems that can do language. And maybe somewhere in between, we will have systems that can play multiplayer games like Starcraft II or Dota 2. All of those things got compressed into the 2010s.
And by the end of the 2010s, we had basically solved language in a way with GPT-3. And now we enter the 2020s and suddenly something totally unexpected which wasn’t in the 70 years of my life and professional career: intelligence in our hands. So, it’s just changing everything and this compressed timeline, I do worry where is this going.
There are still fundamental limitations that I’m sure we’re going to talk about. And it’s not clear whether the acceleration is going to keep going. But if it does keep going, it’s going to challenge a lot of things for us as human beings.
Ashley Llorens: As someone that’s been in the field for a while myself, I had a very similar reaction where I felt like I was interacting with a real intelligence, like something deserving of the name artificial intelligence—AI. What does that mean to you? What does it mean to have real intelligence?
Sébastien Bubeck: It’s a tough question, because, of course, intelligence has been studied for many decades. And psychologists have developed tests of your level of intelligence. But in a way, I feel intelligence is still something very mysterious. It’s kind of—we recognize it when we see it. But it’s very hard to define.
And what I’m hoping is that with this system, what I want to argue is that basically, it was very hard before to study what is intelligence, because we had only one example of intelligence. What is this one example? I’m not necessarily talking about human beings, but more about natural intelligence. By that, I mean intelligence that happened on planet Earth through billions of years of evolution.
This is one type of intelligence. And this was the only example of intelligence that we had access to. And so all our series were fine-tuned to that example of intelligence. Now, I feel that we have a new system which I believe rises to the level of being called an intelligence system. We suddenly have two examples which are very different.
GPT-4’s intelligence is comparable to human in some ways, but it’s also very, very different. It can both solve Olympiad-level mathematical problems and also make elementary school mistakes when adding two numbers. So, it’s clearly not human-like intelligence. It’s a different type of intelligence. And of course, because it came about through a very different process than natural evolution, you could argue that it came about through a process which you could call artificial evolution.
And so I’m hoping that now that we have those two different examples of intelligence, maybe we can start to make progress on defining it and understanding what it is. That was a long-winded answer to your question, but I don’t know how to put it differently.
Basically, the way for me to test intelligence is to really ask creative questions, difficult questions that you do not find, online and (through) search. In a way, you could ask: is Bing, is Google, are search engines intelligent? They can answer tough questions. Are these intelligent systems? Of course not. Everybody would say, no.
So, you have to distinguish, what is it that makes us say that GPT-4 is an intelligent system? Is it just the fact that it can answer many questions? No, it’s more that it can inspect, it answers. It can explain itself. It can interact with you. You can have a discussion. This interaction is really of the essence of intelligence to me.
Ashley Llorens: It certainly is a provocative and unsolved kind of question of: what is intelligence. And perhaps equally mysterious is how we actually measure intelligence. Which is a challenge even for humans. Which I’m reminded of with young kids in the school system, as I know you are or will be soon here as a father.
But you’ve had to think differently as you’ve tried to measure the intelligence of GPT-4. And you alluded to…I’d say the prevailing way that we’ve gone about measuring the intelligence of AI systems or intelligent systems is through this process of benchmarking, and you and your team have taken a very different approach.
Can you maybe contrast those?
Sébastien Bubeck: Of course, yeah. So maybe let me start with an example. So, we use GPT-4 to pass mock interviews for software engineer positions at Amazon and at Google and META. It passes all of those interviews very easily. Not only does it pass those interviews, but it also ranks in the very top of the human beings.
In fact, for the Amazon interview, not only did it pass all the questions, but it scored better than 100% of all the human users on that website. So, this is really incredible. And headlines would be, GPT-4 can be hired as a software engineer at Amazon. But this is a little bit misleading to view it that way because those tests, they were designed for human beings.
They make a lot of hidden assumptions about the person that they are interviewing. In particular, they will not test whether that person has a memory from one day to the next. Of course, human beings remember what they did the next day, unless there is some very terrible problem.
So, they all face those benchmarks of intelligence. At least they face this issue that they were designed to test for human beings. So, we have to find new ways to test intelligence when we’re talking about the intelligence of AI systems. That’s point number one. But number two is so far in the machine learning tradition, we have developed lots of benchmarks to test a system, a narrow AI system.
This is how the machine learning community has made progress over the decades—by beating benchmarks, by having systems that keep improving, percentage by percentage over those target benchmarks. Now, all of those become kind of irrelevant in the era of GPT-4 for two reasons. Number one is GPT-4—we don’t know exactly what data it is being trained on and in particular it might have seen all of these datasets.
So really you cannot separate anymore the training data and the test data. This is not really a meaningful way to test something like GPT-4 because it might have seen everything. For example, Google came out with a suite of benchmarks, which they called Big Bench, and in there they hid the code to make sure that you don’t know the code and you haven’t seen this data, and of course GPT-4 knows this code.
So, it has seen all of Big Bench. So, you just cannot benchmark it against Big Bench. So, that’s problem number one for the classical ML benchmark. Problem number two is that all those benchmarks are just too easy. It’s just too easy for GPT-4. It crushes all of them, hands down. Very, very easily.
In fact, it’s the same thing for the medical license exam for a multi-state bar exam. All of those things it just passes very, very easily. And the reason why we have to go beyond this is really beyond the classical ML benchmark, we really have to test the generative abilities, the interaction abilities. How is it able to interact with human beings? How is it able to interact with tools?
How creative can it be at the task? All of those questions, it’s very hard to benchmark them. Our own hard benchmark, whether there is one right solution. Now, of course, the ML community has grappled with this problem recently because generative AI has been in the works for a few years now, but the answers are still very tentative.
Just to give you an example, imagine that you want to have a benchmark where you describe a movie and you want to write a movie review. Let’s say, for example, you want to tell the system, write a positive movie review about this movie. Okay. The problem is in your benchmark. In the data, you will have examples of those reviews. And then you ask your system to write its own review, which might be very different from what you have in your training data. So, the question is, is it better to write something different or is it worse? Do you have to match what was in the training data? Maybe GPT-4 is so good that it’s going to write something better than what the humans wrote.
And in fact, we have seen that many, many times the training data was crafted by humans and GPT-4 just does a better job at it. So, it gives better labels if you want than what the humans did. It cannot even compare to humans anymore. So, this is a problem that we are facing as we are writing our paper, trying to assess GPT-4’s intelligence.
Ashley Llorens: Give me an example where the model is actually better than the humans.
Sébastien Bubeck: Sure. I mean, let me think of a good one. I mean, coding—it is absolutely superhuman at coding. We already alluded to this and this is going to have tremendous implications. But really coding is incredible. So, for example, going back to the example of movie reviews, there is this IMDB dataset which is very popular in machine learning where you can ask many basic questions that you want to ask.
But now in the era of GPT-4, you can give the IMDB dataset and you can just ask GPT-4—can you explore the dataset. And it’s going to come up with suggestions of data analysis ideas. Maybe it would say, maybe we want to do some clustering, maybe you want to cluster by the movie, directors, and you would see which movies were the most popular and why.
It can come up creatively with its own analysis. So that’s one aspect—differently coding data analysis. It can be very easily superhuman. I think in terms of writing, its writing capabilities are just astounding. For example, in the paper, we asked it many times to rewrite parts of what we wrote, and it writes it in this much more lyrical way, poetic way.
You can ask for any kind of style that you want. It’s really at the level I would say at this far in my novice eyes, I would say it’s at the level of some of the best authors out there. There is its style and this is really native. You don’t have to do anything.
Ashley Llorens: Yeah, it does it does remind me a little bit of the AlphaGo moment or maybe more specifically the AlphaZero moment, where all of a sudden, you kind of leave the human training data behind. And you’re entering into a realm where it’s its only real competition. You talked about the evolution that we need to have of how we measure intelligence from ways of measuring narrow or specialized intelligence to measuring more general kinds of intelligence.
And we’ve had these narrow benchmarks. You see a lot of this, kind of past the bar exam, these kinds of human intelligence measures. But what happens when all of those are also too easy? How do we think about measurement and assessment in that regime?
Sébastien Bubeck: So, of course, I want to say maybe it’s a good point to bring up the limitations of the system also. Right now a very clear frontier that GPT-4 is not stepping over is to produce new knowledge to discover new things, for example, let’s say in mathematics, to prove mathematical theorems that humans do not know how to prove.
Right now, the systems cannot do it. And this, I think, would be a very clean and clear demonstration, whereas there is just no ambiguity once it can start to produce this new knowledge. Now, of course, whether it’s going to happen or not is an open question. I personally believe it’s plausible. I am not 100 percent sure what’s going to happen, but I believe it is plausible that it will happen.
But then there might be another question, which is what happens if the proof that it produces becomes inscrutable to human beings. Mathematics is not only this abstract thing, but it’s also a language between humans. Of course, at the end of the day, you can come back to the axioms, but that’s not the way we humans do mathematics.
So, what happens if, let’s say, GPT-5 proves the Riemann hypothesis and it is formally proved? Maybe it gives the proof in the LEAN language, which is a formalization of mathematics, and you can formally verify that the proof is correct. But no human being is able to understand the concepts that were introduced.
What does it mean? Is the Riemann hypothesis really proven? I guess it is proven, but is that really what we human beings wanted? So this kind of question might be on the horizon. And that I think ultimately might be the real test of intelligence.
Ashley Llorens: Let’s stick with this category of the limitations of the model. And you kind of drew a line here in terms of producing new knowledge. You offered one example of that as proving mathematical theorems. What are some of the other limitations that you’ve discovered?
Sébastien Bubeck: So, GPT-4 is a large language model which was trained on the next –word-prediction objective function. So, what does it mean? It just means you give it a partial text and you’re trying to predict what is going to be the next word in that partial text. Once you want to generate content, you just keep doing that on the text that you’re producing. So, you’re producing words one by one. Now, of course, it’s a question that I have been reflecting upon myself, once I saw GPT-4. It’s a question whether human beings are thinking like this. I mean it doesn’t feel like it. It feels like we’re thinking a little bit more deeply.
We’re thinking a little bit more in advance of what we want to say. But somehow, as I reflect, I’m not so sure, at least when I speak, verbally, orally, maybe I am just coming up every time with the next word. So, this is a very interesting aspect. But the key point is certainly when I’m doing mathematics, I think I am thinking a little bit more deeply.
And I’m not just trying to see what is the next step, but I’m trying to come up with a whole plan of what I want to achieve. And right now the system is not able to do this kind of long-term planning. And we can give a very simple experiment that shows this maybe. My favorite one is, let’s say you have a very simple arithmetic equality—three times seven plus 21 times 27 equals something.
So this is part of the prompt that you give to GPT-4. And now you just ask, okay, you’re allowed to modify one digit in this so that the end result is modified in a certain way. Which one do you choose? So, the way to solve this problem is that you have to think.
You have to try. Okay, what if I were to modify the first digit? What would happen if I were to modify the second digit? What would happen? And GPT-4 is not able to do that. GPT-4 is not able to think ahead in this way. What it will say is just: I think if you modify the third digit, just randomly, it’s going to work. And it just tries and it fails. And the really funny aspect is that once it starts feigning, GPT-4, this becomes part of its context, which in a way becomes part of its truth. So, the failure becomes part of its truth and then it will do anything to justify it.
It will keep making mistakes to keep justifying it. So, these two aspects, the fact that it cannot really plan ahead and that once it makes mistakes, it just becomes part of its truths. These are very, very serious limitations, in particular for mathematics. This makes it a very uneven system, once you approach mathematics.
Ashley Llorens: You mentioned something that’s different about machine learning the way it’s conceptualized in this kind of generative AI regime, which is fundamentally different than what we’ve typically thought about as machine learning, where you’re optimizing an objective function with a fairly narrow objective versus when you’re trying to actually learn something about the structure of the data, albeit through this next word prediction or some other way.
What do you think about that learning mechanism? Are there any limitations of that?
Sébastien Bubeck: This is a very interesting question. Maybe I just want to backtrack for a second and just acknowledge that what happened there is kind of a miracle. Nobody, I think nobody in the world, perhaps, except OpenAI, expected that intelligence would emerge from this next word prediction framework just on a lot of data.
I mean, this is really crazy, if you think about it now, the way I have justified it to myself recently is like this. So, I think it is agreed that deep learning is what powers the GPT4 training. You have a big neural network that you’re training with gradient descent, just trying to fiddle with the parameters.
So, it is agreed that deep learning is this hammer, that if you give it a dataset, it will be able to extract the latent structure of that dataset. So, for example, the first breakthrough that happened in deep learning, a little bit more than ten years ago, was the Alexa.NET moment, where they trained a neural network to basically classify cats, dogs, cars accessorized with images.
And when you train this network, what happens is that you have these edge detectors that emerge on the first few layers of the neural network. And, nothing in the objective function told you that you have to come up with edge detector. This was an emergent property. Why? Because it makes sense. The structure of an image is to combine those edges to create geometric shapes.
Right now, I think what’s happening and we have seen this more and more with the large language models, is that there are more and more emerging properties that happen as you scale up the size of the network and the size of the data. Now what I believe is happening is that in the case of GPT-4 they gave it such a big dataset, so diverse with so many complex parameters in it, that the only way to make sense of it, the only latent structure that unifies all of this data is intelligence.
The only way to make sense of the data was for the system to become intelligent. This is kind of a crazy sentence. And I expect the next few years, maybe even the next few decades, will try to make sense of whether this sentence is correct or not. And hopefully, human beings are intelligent enough to make sense of that sentence.
I don’t know right now. I just feel like it’s a reasonable hypothesis that this is what happened there. And so in a way, you can say maybe there is no limitation to the next-word-prediction framework. So that’s one perspective. The other perspective is, actually, the next –word-prediction, token framework is very limiting, at least at generation time.
At least once you start to generate new sentences, you should go beyond a little bit if you want to have a planning aspect, if you want to be able to revisit mistakes that you made. So, there we believe that at least at generation time, you need to have a slightly different system. But maybe in terms of training, in terms of coming up with intelligence in the first place, maybe this is a fine way to do it.
Ashley Llorens: And maybe I’m kind of inspired to ask you a somewhat technical question, though. Yeah. Where I think one aspect of our previous notion of intelligence and maybe still the current notion of intelligence for some is this aspect of compression, the ability to take something complex and make it simple, maybe thinking grounded in Occam’s Razor, where we want to generate the simplest explanation of the data in the thing, some of the things you’re saying and some of the things we’re seeing in the model kind of go against that intuition.
So talk to me a little bit about that.
Sébastien Bubeck: Absolutely. So, I think this is really exemplified well in the project that we did here at Microsoft Research a year ago, which we called Lego. So let me tell you about this very briefly, because it will really get to the point of what you’re trying to say. So, let’s say you want train an AI system that can solve middle school systems of linear equations.
So, maybe it’s X plus Y equals Z, three X minus two, Y equals one, and so on. You have three equations with two variables. And you want to train a neural network that takes in this system of equation and outputs the answer for it. The classical perspective, the Occam’s Razor perspective would be collected dataset with lots of equations like this train the system to solve those linear equation.
And there you go. This is a way you have the same kind of distribution at training time and that they start now. What this new paradigm of deep learning and in particular of large language models would say is instead, even though your goal is to solve systems of linear equations for middle school students instead of just training data, middle school systems offline.
Now, we’re going to collect a hugely diverse list of data maybe we’re going to do next. One prediction not only on the systems of linear equation, but also on all of Wikipedia. So, this is now a very concrete experiment. You have to learn networks. Neural network A, train on the equations. Neural network B, train on the equations, plus Wikipedia. And any kind of classical thinking would tell you that neural network B is going to do worse because it has to do more things, it’s going to get more confused. It’s not the simplest way to solve the problem. But lo and behold, if you actually run the experiment for real, Network B is much, much, much better than network A. Now I need to quantify this a little bit. Network A, if it was trained with systems of linear regression with three variables, is going to be fine on systems of linear regression with three variables.
But as soon as you ask it four variables or five variables, it’s not able to do it. It didn’t really get to the essence of what it means to solve the linear equations, whereas Network B, it’s not only subsystems of equation with three variables, but it also does four, it also does five and so on.
Now the question is why? What’s going on? Why is it that making the thing more complicated, going against Occam’s Razor, why is that a good idea? And the extremely naive perspective, which in fact some people have said because it is so mysterious, would be maybe to read the Wikipedia page on solving systems of linear equation.
But of course, that’s not what happened. And this is another aspect of this whole story, which is anthropomorphication of the system is a big danger. But let’s not get into that right now. But the point is that’s not at all the reason why we became good at solving systems of linear equations.
It’s rather that it had this very diverse data and it forced it to come up with unifying principles, more canonical, component of intelligence. And then it’s able to compose this canonical component of intelligence to solve the task at hand.
Ashley Llorens: I want to go back to something you said much earlier around natural evolution versus this notion of artificial evolution. And I think that starts to allude to where I think you want to take this field next, at least in terms of your study and your group. And that is, focusing on the aspect of emergence and how intelligence emerges.
So, what do you see as the way forward from this point, from your work with Lego that you just described for you and for the field?
Sébastien Bubeck: Yes, absolutely. So, I would argue that maybe we need a new name for machine learning in a way. GPT-4 and GPT-3 and all those other large language models, in some ways, it’s not machine learning anymore. And by that I mean machine learning is all about how do you teach a machine a very well-defined task? Recognize cats and dogs, something like that. But here, that’s not what we’re doing. We’re not trying to teach it a narrow task. We’re trying to teach it everything. And we’re not trying to mimic how a human would learn. This is another point of confusion. Some people say, oh, but it’s learning language, but using more tags than any human would ever see.
But that’s kind of missing the point. The point is we’re not trying to mimic human learning. And that’s why maybe learning is not the right word anymore. We’re really trying to mimic something which is more akin to evolution. We’re trying to mimic the experience of millions, billions of entities that interact with the world. In this case, the world is the data that humans produced.
So, it’s a very different style. And I believe the reason why all the tools that we have introduced in machine learning are kind of useless and almost irrelevant in light of GPT-4 is because it’s a new field. It’s something that needs new tools to be defined. So we hope to be at the forefront of that and we want to introduce those new tools.
And of course, we don’t know what it’s going to look like, but the avenue that we’re taking to try to study this is to try to understand emergence. So emergence again is this phenomenon that as you scale up the network and the data, suddenly there are new properties that emerge at every scale. Google had this experiment where they scaled up their large language models from 8 billion to 60 billion to 500 billion.
And at 8 billion, it’s able to understand language. And it’s able to do a little bit of arithmetic at 60 billion. Suddenly it’s able to translate between languages. Before it couldn’t translate. At 60 billion parameters, suddenly it can translate. At 500 billion suddenly it can explain jokes. Why can it suddenly explain jokes?
So, we really would like to understand this. And there is another field out there that has been grappling with emergence for a long time that we’re trying to study systems of very complex particles interacting with each other and leading to some emergent behaviors.
What is this field? It’s physics. So, what we would like to propose is let’s study the physics of AI or the physics of AGI, because in a way, we are really seeing this general intelligence now. So, what would it mean to study the physics of AGI? What it would mean is, let’s try to borrow from the methodology that physicists have used for the last few centuries to make sense of reality.
And what (were) those tools? Well, one of them was to run a very controlled experiment. If you look at the waterfall and you observe the water which is flowing and is going in all kinds of ways, and you go look at it in the winter and it’s frozen. Good luck to try to make sense of the phases of water by just staring at the waterfall, GPT-4 or LAMDA or the flash language model.
These are all waterfalls. What we need are much more small scale controlled experiments where we know we have pure water. It’s not being tainted by the stone, by the algae. We need those controlled experiments to make sense of it. And LEGO is one example. So that’s one direction that we want to take. But in physics there is another direction that you can take, which is to build toy mathematical models of the real world.
You try to abstract away lots of things, and you’re left with a very simple mathematical equation that you can study. And then you have to go back to really experiment and see whether the prediction from the toy mathematical model tells you something about the real experiment. So that’s another avenue that we want to take. And then we made some progress recently also with interns at (Microsoft Research).
So, we have a paper which is called Learning Threshold Units. And here really we’re able to understand how does the most basic element, I don’t want to say intelligence, but the most basic element of reasoning emerges in those neural networks. And what is this most basic element of reasoning? It’s a threshold unit. It’s something that takes as input some value.
And if the value is too small, then it just turns it to zero. And this emergence already, it’s a very, very complicated phenomenon. And we were able to understand the non convex dynamics at play and connected to which is called the edge of stability, which is all very exciting. But the key point is that we have a toy mathematical model and there in essence what we were able to do is to say that emergence is related to the instability in training, which is very surprising because usually in classical machine learning, instability, something that you do not want, you want to erase all the instabilities.
And somehow through this physics of AI approach, where we have a toy mathematical model, we are able to say actually it’s instability in training that you’re seeing, that everybody is seeing for decades now, it actually matters for learning and for emergence. So, this is the first edge that we took.
Ashley Llorens: I want to come back to this aspect of interaction and want to ask you if you see fundamental limitations with this whole methodology around certain kinds of interactions. So right now we’ve been talking mostly about these models interacting with information in information environments, with information that people produce, and then producing new information behind that.
The source of that information is actual humans. So, I want to know if you see any limitations or if this is an aspect of your study, how we make these models better at interacting with humans, understanding the person behind the information produced. And after you do that, I’m going to come back and we’ll ask the same question of the natural world in which we as humans reside.
Sébastien Bubeck: Absolutely. So, this is one of the emergent properties of GPT-4 to put it very simply, that not only can it interact with information, but it can actually interact with humans, too. You can communicate with it. You can discuss, and you’re going to have very interesting discussions. In fact, some of my most interesting discussions in the last few months were with GPT-4.
So this is surprising. Not at all something we would have expected, But in there it’s there. Not only that, but it also has a theory of mind. So GPT-4 is able to reason about what somebody is thinking, what somebody is thinking about, what somebody else is thinking, and so on. So, it really has a very sophisticated theory of mind. There was recently a paper saying that ChatGPT is roughly at the level of a seven-year-old in terms of its theory of mind. For GPT-4, I cannot really distinguish from an adult. Just to give you an anecdote, I don’t know if I should say this, but one day in the last few months, I had an argument with my wife and she was telling me something.
And I just didn’t understand what she wanted from me. And I just talked with GPT-4 I explained the situation. And that’s what’s going on. What should I be doing? And the answer was so detailed, so thoughtful. I mean, I’m really not making this up. This is absolutely real. I learned something from GPT-4 about human interaction with my wife.
This is as real as it gets. And so, I can’t see any limitation right now in terms of interaction. And not only can it interact with humans, but it can also interact with tools. And so, this is the premise in a way of the new Bing that was recently introduced, which is that this new model, you can tell it “hey, you know what, you have access to a search engine.”
“You can use Bing if there is some information that you’re missing and you need to find it out, please make a Bing search.” And somehow natively, this is again, an emergent property. It’s able to use a search engine and make searches when it needs to, which is really, really incredible. And not only can it use those tools which are well-known, but you can also make up tools.
You can tell you can say, hey, I invented some API. Here is what the API does. Now please solve me problem XYZ using that API and it’s able to do it native. It’s able to understand your description in natural language of what the API that you built is doing and it’s able to leverage its power and use it.
This is really incredible and opens so many directions.
Ashley Llorens: We certainly see some super impressive capabilities like the new integration with Bing, for example. We also see some of those limitations come into play. Tell me about your exploration of those in this context.
Sébastien Bubeck: So, one keyword that didn’t come up yet and which is going to drive forward is a conversation at least online and on Twitter is hallucinations. So those models, GPT-4, still does hallucinate a lot. And in a way, for good reason, it’s on the spectrum where on the one hand you have bad hallucination, completely making up facts when which are contrary to the real facts in the real world.
But on the other end, you have creativity. When you create, when you generate new things, you are, in a way, hallucinating. It’s good hallucinations, but still these are hallucinations. So having a system which can both be creative, but does not hallucinate at all—it’s a very delicate balance. And GPT-4 did not solve that problem yet. It made a lot of progress, but it didn’t solve it yet.
So that’s still a big limitation, which the world is going to have to grapple with. And I think in the new Bing it’s very clearly explained that it is still making mistakes from time to time and that you need to double check the result. I still think the rough contours of what GPT-4 says and the new Bing says is really correct.
And it’s a very good first draft most of the time, and you can get started with that. But then, you need to do your research and it cannot be used for critical missions yet. Now what’s interesting is GPT-4 is also intelligent enough to look over itself. So, once it produced a transcript, you can ask another instance of GPT-4 to look over what the first instance did and to check whether there is any hallucination.
This works particularly well for what I would call in-context hallucination. So, what would be in-context hallucination? Let’s say you have a text that you’re asking it to summarize and maybe in the summary, it invents something that was not out there. Then the other instance of GPT-4 will immediately spot it. So that’s basically in-context hallucination.
We believe they can be fully solved soon. The open-world type of hallucination When you ask anything, for example, in our paper we ask: where is the McDonald’s at Sea-Tac, at the airport in Seattle. And it responds: gate C2. And the answer is not C2. The answer, it’s B3. So this type of open-world hallucination—it’s much more difficult to resolve.
And we don’t know yet how to do that. Exactly.
Ashley Llorens: Do you see a difference between a hallucination and a factual error?
Sébastien Bubeck: I have to think about this one. I would say that no, I do not really see a difference between the hallucination and the factual error. In fact, I would go as far as saying that when it’s making arithmetic mistakes, which again, it still does, when it adds two numbers, you can also view it as some kind of hallucination.
And by that I mean it’s kind of a hallucination by omission. And let me explain what I mean. So, when it does an arithmetic calculation, you can actually ask it to print each step and that improves the accuracy. It does a little bit better if it has to go through all the steps and this makes sense from the next word prediction framework.
Now what happens is very often it will skip a step. It would kind of forget something. This can be viewed as a kind of hallucination. It just, hallucinated that this step is not necessary and that it can move on to the next stage immediately. And so, this kind of factual error or like in this case in a reasoning error if you want, they are all related to the same concept of hallucination. There could be many ways to resolve those hallucinations.
Maybe we want to look inside the model a little bit more. Maybe we want to change the training pipeline a little bit. Maybe the reinforcement learning with human feedback can help. All of these are small patches and still I want to make it clear to the audience that it’s still an academic open problem whether any of those directions can eventually fix it or is it a fatal error for large language models that will never be fixed.
We do not know the answer to that question.
Ashley Llorens: I want to come back to this notion of interaction with the natural world.
As human beings, we learn about the natural world through interaction with it. We start to develop intuitions about things like gravity, for example. And there is an argument or debate right now in the community as to how much of that knowledge of how to interact with the natural world is encoded and learnable from language and the kinds of information inputs that we put into the model versus how much actually needs to be implicit, explicitly encoded in an architecture or just learned through interaction with the world.
What do you see here? Do you see a fundamental limitation with this kind of architecture for that purpose?
Sébastien Bubeck: I do think that there is a fundamental limit. I mean, that there is a fundamental limitation in terms of the current structure of the pipeline. And I do believe it’s going to be a big limitation once you ask the system to discover new facts. So, what I think is the next stage of evolution for the systems would be to hook it up with a simulator of sorts so that the system at training time when it’s going through all of the web, is going through all of the data produced by humanity.
So, it realizes, oh, maybe I need more data of a certain type, then we want to give it access to a simulator so that it can produce its own data, it can run experiments which is really what babies are doing. Infants—they run experiments when they play with a ball, when they look at their hand in front of their face.
This is an experiment. So, we do need to give access to the system a way to do experiments. Now, the problem of this is you get into this little bit of a dystopian discussion of whether do we really want to give these systems which are super intelligent in some way, do we want to give them access to simulators?
Aren’t we afraid that they will become super-human in every way if some of the experiments that they can run is to run code, is to access the internet? There are lots of questions about what could happen. And it’s not hard to imagine what could go wrong there.
Ashley Llorens: It’s a good segue into maybe a last question or topic to explore, which comes back to this phrase AGI—artificial general intelligence. In some ways, there’s kind of a lowercase version of that where we talk towards more generalizable kinds of intelligence. That’s the regime that we’ve been exploring. Then there’s a kind of a capital letter version of that, which is almost like a like a sacred cow or a kind of dogmatic pursuit within the AI community. So, what does that capital letter phrase AGI, mean to you? And maybe part B of that is: is our classic notion of AGI the right goal for us to be aiming for?
Sébastien Bubeck: Before interacting with GPT-4, to me, AGI was this unachievable dream. Some think it’s not even clear whether it’s doable. What does it even mean? And really by interacting with GPT-4, I suddenly had the realization that actually general intelligence, it’s something very concrete.
It’s able to understand any kind of topics that you bring up. It is going to be able to reason about any of the things that you want to discuss. It can bring up information, it can use tools, it can interact with humans, it can interact with an environment. This is general intelligence. Now, you’re totally right in encoding it “lowercase” AGI.
Why is it not uppercase AGI? Because it’s still lacking some of the fundamental aspects, two of them, which are really, really important. One is memory. So, every new session with GPT-4 is a completely fresh tabula rasa session. It’s not remembering what you did yesterday with it. And it’s something which is emotionally hard to take because you kind of develop a relationship with the system.
As crazy as it sounds, that’s really what happens. And so you’re kind of disappointed that it doesn’t remember all the good times that you guys had together. So this is one aspect. The other one is the learning. Right now, you cannot teach it new concepts very easily. You can turn the big crank of retraining the model.
Sure, you can do that, but I’ll give you the example of using a new API. Tomorrow, you have to explain it again. So, of course, learning and memory, those two things are very, very related, as I just explained. So, this is one huge limitation to me.
If it had that, I think it would qualify as uppercase AGI. Now, not everybody would agree even with that, because many people would say, no, it needs to be embodied, to have real world experience. This becomes a philosophical question. Is it possible to have something that you would call a generally intelligent being that only lives in the digital world?
I don’t see any problem with that, honestly. I cannot see any issue with this. Now, there is another aspect. Once you get into this philosophical territory, which is right now the systems have no intrinsic motivation. All they want to do is to generate the next token. So, is that also an obstruction to having something which is a general intelligence?
Again, to me this becomes more philosophical than really technical, but maybe there is some aspect which is technical there. Again, if you start to hook up the system to simulate those to run their own experiments, then certainly they maybe have some intrinsic motivation to just improve themselves. So maybe that’s one technical way to resolve the question. I don’t know.
Ashley Llorens: That’s interesting. And I think there’s a word around that phrase in the community. Agent. Or seeing “agentic” or goal-oriented behaviors. And that is really where you start to get into the need for serious sandboxing or alignment or other kinds of guardrails for the system that actually starts to exhibit goal-oriented behavior.
Sébastien Bubeck: Absolutely. Maybe one other point that I want to bring up about AGI, which I think is confusing a lot of people. Somehow when people hear general intelligence, they want something which is truly general that could grapple with any kind of environment. And not only that, but maybe that grapples with any kind of environment and does so in a sort of optimal way.
This universality and optimality, I think, are completely irrelevant to intelligence. Intelligence has nothing to do with universality or optimality. We as human beings are notoriously not universal. I mean, you change a little bit the condition of your environment, and you’re going to be very confused for a week. It’s going to take you months to adapt.
So, we are very, very far from universal and I think I don’t need to tell anybody that we’re very far from being optimal. The number of crazy decisions that we make every second is astounding. So, we’re not optimal in any way. So, I think it is not realistic to try to have an AGI that would be universal and optimal. And it’s not even desirable in any way, in my opinion. So that’s maybe not achievable and not even realistic, in my opinion.
Ashley Llorens: Is there an aspect of complementarity that we should be striving for in, say, a refreshed version of AGI or this kind of long-term goal for AI?
Sébastien Bubeck: Yeah, absolutely. But I don’t want to be here in this podcast today and try to say what I view in terms of this question, because I think it’s really a community that should come together and discuss this in the coming weeks, months, years, and come together with where do we want to go, where the society want to go, and so on.
I think it’s a terribly important question. And we should not dissociate our futuristic goal with the technical innovation that we’re trying to do day-to-day. We have to take both into account. But I imagine that this discussion will happen and we will know a lot more a year from now, hopefully.
Ashley Llorens: Thanks, Sébastien. Just a really fun and fascinating discussion. Appreciate your time today.
Sébastien Bubeck: Yeah, thanks, Ashley it was super fun.
The post AI Frontiers: The Physics of AI with Sébastien Bubeck appeared first on Microsoft Research.
A new paradigm for partnership between industry and academia
How Amazon is shaping a set of initiatives to enable academia-based talent to harmonize their passions, life stations, and career ambitions.Read More
GFN Thursday Celebrates 1,500+ Games and Their Journey to GeForce NOW
Gamers love games — as do the people who make them.
GeForce NOW streams over 1,500 games from the cloud, and with the Game Developers Conference in full swing this week, today’s GFN Thursday celebrates all things games: the tech behind them, the tools that bring them to the cloud, the ways to play them and the new ones being added to the library this week.
Developers use a host of NVIDIA resources to deliver the best in PC cloud gaming experiences. CD PROJEKT RED, one of many developers to tap into these resources, recently announced a new update coming to Cyberpunk 2077 on April 11 — including a new technology preview for Ray Tracing: Overdrive Mode that enables full ray tracing on GeForce RTX 40 Series GPUs and RTX 4080 SuperPODs.
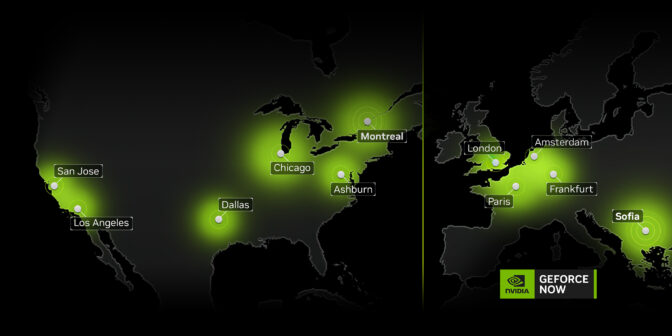
In addition, members in and around Sofia, Bulgaria, can now experience the best of GeForce NOW Ultimate cloud gaming. It’s the latest city to roll out RTX 4080 gaming rigs to GeForce NOW servers around the globe.
Plus, with five new games joining the cloud this week, and an upcoming marvel-ous reward, GeForce NOW members can look forward to a busy weekend of streaming goodness.
Developers, Mount Up!
GDC presents the ideal time to spotlight GeForce NOW tools that enable developers to seamlessly bring their games to the cloud. NVIDIA tools, software development kits (SDKs) and partner engines together enable the production of stunning real-time content that uses AI and ray tracing. And bringing these games to billions of non-PC devices is as simple as checking an opt-in box.
GeForce NOW taps into existing game stores, allowing game developers to reap the benefits of a rapidly growing audience without the hassle of developing for another platform. This means zero port work to bring games to the cloud. Users don’t have to buy games for another platform and can play them on many of the devices they already own.
Developers who want to do more have access to the GeForce NOW Developer Platform — an SDK and toolset empowering integration of, interaction with and testing on the NVIDIA cloud gaming service. It allows developers to enhance their games to run more seamlessly, add cloud gaming into their stores and launchers, and let users connect their accounts and libraries to GeForce NOW.
The SDK is a set of APIs, runtimes, samples and documentation that allows games to query for cloud execution and enable virtual touchscreens; launchers to trigger cloud streaming of a specified game; and GeForce NOW and publisher backends to facilitate account linking and game library ownership syncing, already available for Steam and Ubisoft games.
Content developers have a slew of opportunities to bring their virtual worlds and interactive experiences to users in unique ways, powered by the cloud.
Metaverse services company Improbable will use NVIDIA cloud gaming infrastructure for an interactive, live, invite-only experience that will accommodate up to 10,000 guests. Other recent developer events included the DAF Trucks virtual experience, where potential customers took the newest DAF truck for a test drive in a simulated world, with PixelMob’s Euro Truck Simulator 2 providing the virtual playground.
Furthermore, CD PROJEKT RED will be delivering full ray tracing, aka path tracing, to Cyberpunk 2077. Such effects were previously only possible for film and TV. With the power of a GeForce RTX 4080 gaming rig in the cloud, Ultimate members will be able to stream the new technology preview for the Ray Tracing: Overdrive Mode coming to Cyberpunk 2077 across devices — even Macs — no matter the game’s system requirements.
Get Ready for a Marvel-ous Week
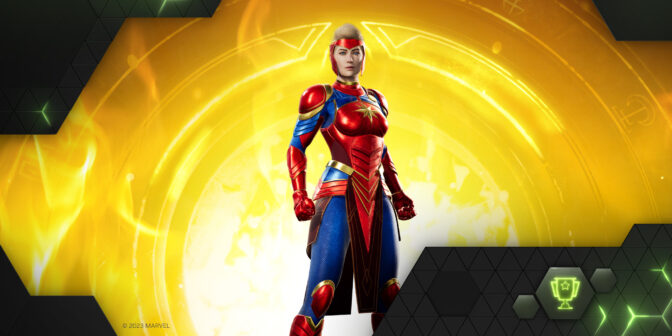
GeForce NOW Ultimate members have been enjoying Marvel’s Midnight Suns’ ultra-smooth, cinematic game play thanks to DLSS 3 technology support on top of RTX-powered ray tracing, which together enable graphics breakthroughs.
Now, members can fight among the legends with Captain Marvel’s Medieval Marvel suit in a free reward, which will become available at the end of the month — first to Premium members who are opted into GeForce NOW rewards. This reward is only available until May 6, so upgrade to an Ultimate or Priority membership today and opt into rewards to get first access.
Next, on to the five new games hitting GeForce NOW week for a happy weekend:
- Tchia (New release on Epic Games Store)
- Chess Ultra (New release on Epic Games Store, March 23)
- Amberial Dreams (Steam)
- Symphony of War: The Nephilim Saga (Steam)
- No One Survived (Steam)
And with that, we’ve got a question to end this GFN Thursday:
You’ve got free rent for a year to live in a video game city of your choice, which one are you choosing?
—
NVIDIA GeForce NOW (@NVIDIAGFN) March 22, 2023