Large language models (LLMs) with billions of parameters are currently at the forefront of natural language processing (NLP). These models are shaking up the field with their incredible abilities to generate text, analyze sentiment, translate languages, and much more. With access to massive amounts of data, LLMs have the potential to revolutionize the way we interact with language. Although LLMs are capable of performing various NLP tasks, they are considered generalists and not specialists. In order to train an LLM to become an expert in a particular domain, fine-tuning is usually required.
One of the major challenges in training and deploying LLMs with billions of parameters is their size, which can make it difficult to fit them into single GPUs, the hardware commonly used for deep learning. The sheer scale of these models requires high-performance computing resources, such as specialized GPUs with large amounts of memory. Additionally, the size of these models can make them computationally expensive, which can significantly increase training and inference times.
In this post, we demonstrate how we can use Amazon SageMaker JumpStart to easily fine-tune a large language text generation model on a domain-specific dataset in the same way you would train and deploy any model on Amazon SageMaker. In particular, we show how you can fine-tune the GPT-J 6B language model for financial text generation using both the JumpStart SDK and Amazon SageMaker Studio UI on a publicly available dataset of SEC filings.
JumpStart helps you quickly and easily get started with machine learning (ML) and provides a set of solutions for the most common use cases that can be trained and deployed readily with just a few steps. All the steps in this demo are available in the accompanying notebook Fine-tuning text generation GPT-J 6B model on a domain specific dataset.
Solution overview
In the following sections, we provide a step-by-step demonstration for fine-tuning an LLM for text generation tasks via both the JumpStart Studio UI and Python SDK. In particular, we discuss the following topics:
- An overview of the SEC filing data in the financial domain that the model is fine-tuned on
- An overview of the LLM GPT-J 6B model we have chosen to fine-tune
- A demonstration of two different ways we can fine-tune the LLM using JumpStart:
- Use JumpStart programmatically with the SageMaker Python SDK
- Access JumpStart using the Studio UI
- An evaluation of the fine-tuned model by comparing it with the pre-trained model without fine-tuning
Fine-tuning refers to the process of taking a pre-trained language model and training it for a different but related task using specific data. This approach is also known as transfer learning, which involves transferring the knowledge learned from one task to another. LLMs like GPT-J 6B are trained on massive amounts of unlabeled data and can be fine-tuned on smaller datasets, making the model perform better in a specific domain.
As an example of how performance improves when the model is fine-tuned, consider asking it the following question:
“What drives sales growth at Amazon?”
Without fine-tuning, the response would be:
“Amazon is the world’s largest online retailer. It is also the world’s largest online marketplace. It is also the world”
With fine tuning, the response is:
“Sales growth at Amazon is driven primarily by increased customer usage, including increased selection, lower prices, and increased convenience, and increased sales by other sellers on our websites.”
The improvement from fine-tuning is evident.
We use financial text from SEC filings to fine-tune a GPT-J 6B LLM for financial applications. In the next sections, we introduce the data and the LLM that will be fine-tuned.
SEC filing dataset
SEC filings are critical for regulation and disclosure in finance. Filings notify the investor community about companies’ business conditions and the future outlook of the companies. The text in SEC filings covers the entire gamut of a company’s operations and business conditions. Because of their potential predictive value, these filings are good sources of information for investors. Although these SEC filings are publicly available to anyone, downloading parsed filings and constructing a clean dataset with added features is a time-consuming exercise. We make this possible in a few API calls in the JumpStart Industry SDK.
Using the SageMaker API, we downloaded annual reports (10-K filings; see How to Read a 10-K for more information) for a large number of companies. We select Amazon’s SEC filing reports for years 2021–2022 as the training data to fine-tune the GPT-J 6B model. In particular, we concatenate the SEC filing reports of the company in different years into a single text file except for the “Management Discussion and Analysis” section, which contains forward-looking statements by the company’s management and are used as the validation data.
The expectation is that after fine-tuning the GPT-J 6B text generation model on the financial SEC documents, the model is able to generate insightful financial related textual output, and therefore can be used to solve multiple domain-specific NLP tasks.
GPT-J 6B large language model
GPT-J 6B is an open-source, 6-billion-parameter model released by Eleuther AI. GPT-J 6B has been trained on a large corpus of text data and is capable of performing various NLP tasks such as text generation, text classification, and text summarization. Although this model is impressive on a number of NLP tasks without the need for any fine-tuning, in many cases you will need to fine-tune the model on a specific dataset and NLP tasks you are trying to solve for. Use cases include custom chatbots, idea generation, entity extraction, classification, and sentiment analysis.
Access LLMs on SageMaker
Now that we have identified the dataset and the model we are going to fine-tune on, JumpStart provides two avenues to get started using text generation fine-tuning: the SageMaker SDK and Studio.
Use JumpStart programmatically with the SageMaker SDK
We now go over an example of how you can use the SageMaker JumpStart SDK to access an LLM (GPT-J 6B) and fine-tune it on the SEC filing dataset. Upon completion of fine-tuning, we will deploy the fine-tuned model and make inference against it. All the steps in this post are available in the accompanying notebook: Fine-tuning text generation GPT-J 6B model on domain specific dataset.
In this example, JumpStart uses the SageMaker Hugging Face Deep Learning Container (DLC) and DeepSpeed library to fine-tune the model. The DeepSpeed library is designed to reduce computing power and memory use and to train large distributed models with better parallelism on existing computer hardware. It supports single node distributed training, utilizing gradient checkpointing and model parallelism to train large models on a single SageMaker training instance with multiple GPUs. With JumpStart, we integrate the DeepSpeed library with the SageMaker Hugging Face DLC for you and take care of everything under the hood. You can easily fine-tune the model on your domain-specific dataset without manually setting it up.
Fine-tune the pre-trained model on domain-specific data
To fine-tune a selected model, we need to get that model’s URI, as well as the training script and the container image used for training. To make things easy, these three inputs depend solely on the model name, version (for a list of the available models, see Built-in Algorithms with pre-trained Model Table), and the type of instance you want to train on. This is demonstrated in the following code snippet:
from sagemaker import image_uris, model_uris, script_uris, hyperparameters
model_id, model_version = "huggingface-textgeneration1-gpt-j-6b", "*"
training_instance_type = "ml.g5.12xlarge"
# Retrieve the docker image
train_image_uri = image_uris.retrieve(
region=None,
framework=None,
model_id=model_id,
model_version=model_version,
image_scope="training",
instance_type=training_instance_type,
)
# Retrieve the training script
train_source_uri = script_uris.retrieve(
model_id=model_id, model_version=model_version, script_scope="training"
)
# Retrieve the pre-trained model tarball to further fine-tune
train_model_uri = model_uris.retrieve(
model_id=model_id, model_version=model_version, model_scope="training"
)
We retrieve the model_id
corresponding to the same model we want to use. In this case, we fine-tune huggingface-textgeneration1-gpt-j-6b
.
Defining hyperparameters involves setting the values for various parameters used during the training process of an ML model. These parameters can affect the model’s performance and accuracy. In the following step, we establish the hyperparameters by utilizing the default settings and specifying custom values for parameters such as epochs
and learning_rate
:
from sagemaker import hyperparameters
# Retrieve the default hyper-parameters for fine-tuning the model
hyperparameters = hyperparameters.retrieve_default(model_id=model_id, model_version=model_version)
# [Optional] Override default hyperparameters with custom values
hyperparameters["epochs"] = "6"
hyperparameters["learning_rate"] = "2e-04"
print(hyperparameters)
JumpStart provides an extensive list of hyperparameters available to tune. The following list provides an overview of part of the key hyperparameters utilized in fine-tuning the model. For a full list of hyperparameters, see the notebook Fine-tuning text generation GPT-J 6B model on domain specific dataset.
- epochs – Specifies at most how many epochs of the original dataset will be iterated.
- learning_rate – Controls the step size or learning rate of the optimization algorithm during training.
- eval_steps – Specifies how many steps to run before evaluating the model on the validation set during training. The validation set is a subset of the data that is not used for training, but instead is used to check the performance of the model on unseen data.
- weight_decay – Controls the regularization strength during model training. Regularization is a technique that helps prevent the model from overfitting the training data, which can result in better performance on unseen data.
- fp16 – Controls whether to use fp16 16-bit (mixed) precision training instead of 32-bit training.
- evaluation_strategy – The evaluation strategy used during training.
- gradient_accumulation_steps – The number of updates steps to accumulate the gradients for, before performing a backward/update pass.
For further details regarding hyperparameters, refer to the official Hugging Face Trainer documentation.
You can now fine-tune this JumpStart model on your own custom dataset using the SageMaker SDK. We use the SEC filing data we described earlier. The train and validation data is hosted under train_dataset_s3_path
and validation_dataset_s3_path
. The supported format of the data includes CSV, JSON, and TXT. For the CSV and JSON data, the text data is used from the column called text
or the first column if no column called text is found. Because this is for text generation fine-tuning, no ground truth labels are required. The following code is an SDK example of how to fine-tune the model:
from sagemaker.estimator import Estimator
from sagemaker.utils import name_from_base
from sagemaker.tuner import HyperparameterTuner
from sagemaker.huggingface import HuggingFace
train_dataset_s3_path = "s3://jumpstart-cache-prod-us-west-2/training-datasets/tc/data.csv"
validation_dataset_s3_path = "s3://jumpstart-cache-prod-us-west-2/training-datasets/tc/data.csv"
training_job_name = name_from_base(f"jumpstart-example-{model_id}")
metric_definitions=[
{'Name': 'train:loss', 'Regex': "'loss': ([0-9]+.[0-9]+)"},
{'Name': 'eval:loss', 'Regex': "'eval_loss': ([0-9]+.[0-9]+)"},
{'Name': 'eval:runtime', 'Regex': "'eval_runtime': ([0-9]+.[0-9]+)"},
{'Name': 'eval:samples_per_second', 'Regex': "'eval_samples_per_second': ([0-9]+.[0-9]+)"},
{'Name': 'eval:eval_steps_per_second', 'Regex': "'eval_steps_per_second': ([0-9]+.[0-9]+)"},
]
# # Create SageMaker Estimator instance
tg_estimator = Estimator(
role=aws_role,
image_uri=train_image_uri,
source_dir=train_source_uri,
model_uri=train_model_uri,
entry_point="transfer_learning.py",
instance_count=1,
instance_type=training_instance_type,
hyperparameters=hyperparameters,
output_path=s3_output_location,
base_job_name=training_job_name,
enable_network_isolation=True,
metric_definitions=metric_definitions
)
# Launch a SageMaker Training job by passing s3 path of the training data
tg_estimator.fit({"train": train_dataset_s3_path, "validation": validation_dataset_s3_path}, logs=True)
After we have set up the SageMaker Estimator with the required hyperparameters, we instantiate a SageMaker estimator and call the .fit
method to start fine-tuning our model, passing it the Amazon Simple Storage Service (Amazon S3) URI for our training data. As you can see, the entry_point
script provided is named transfer_learning.py
(the same for other tasks and models), and the input data channel passed to .fit
must be named train and validation.
JumpStart also supports hyperparameter optimization with SageMaker automatic model tuning. For details, see the example notebook.
Deploy the fine-tuned model
When training is complete, you can deploy your fine-tuned model. To do so, all we need to obtain is the inference script URI (the code that determines how the model is used for inference once deployed) and the inference container image URI, which includes an appropriate model server to host the model we chose. See the following code:
from sagemaker.predictor import Predictor
from sagemaker import image_uris
from sagemaker.utils import name_from_base
import boto3
sagemaker_session = sagemaker.Session(boto_session=boto3.Session(region_name="us-west-2"))
#Retrieve the inference docker container uri
deploy_image_uri = image_uris.retrieve(
region=None,
framework=None,
image_scope="inference",
model_id=model_id,
model_version=model_version,
instance_type=inference_instance_type,
)
endpoint_name = name_from_base(f"jumpstart-example-{model_id}")
# Use the estimator from the previous step to deploy to a SageMaker endpoint
finetuned_predictor = tg_estimator.deploy(
initial_instance_count=1,
instance_type="ml.g5.12xlarge",
image_uri=image_uri,
endpoint_name=endpoint_name,
)
After a few minutes, our model is deployed and we can get predictions from it in real time!
Access JumpStart through the Studio UI
Another way to fine-tune and deploy JumpStart models is through the Studio UI. This UI provides a low-code/no-code solution to fine-tuning LLMs.
On the Studio console, choose Models, notebooks, solutions under SageMaker JumpStart in the navigation pane.
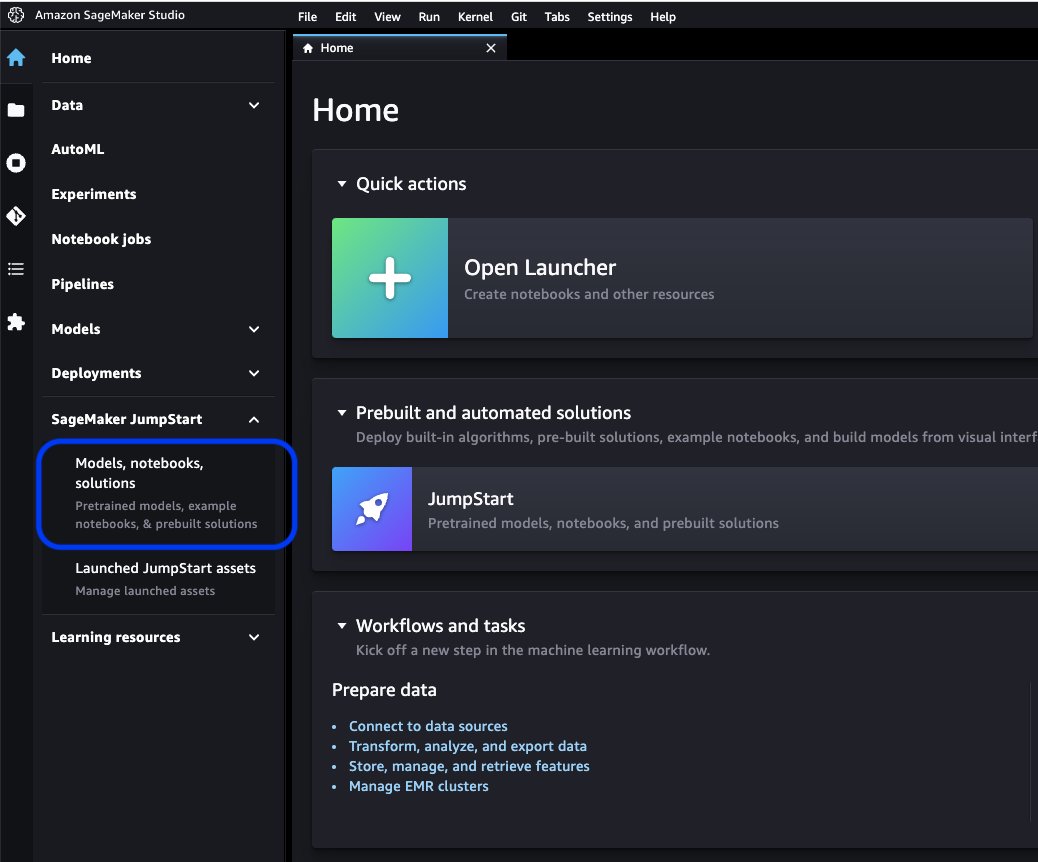
In the search bar, search for the model you want to fine-tune and deploy.
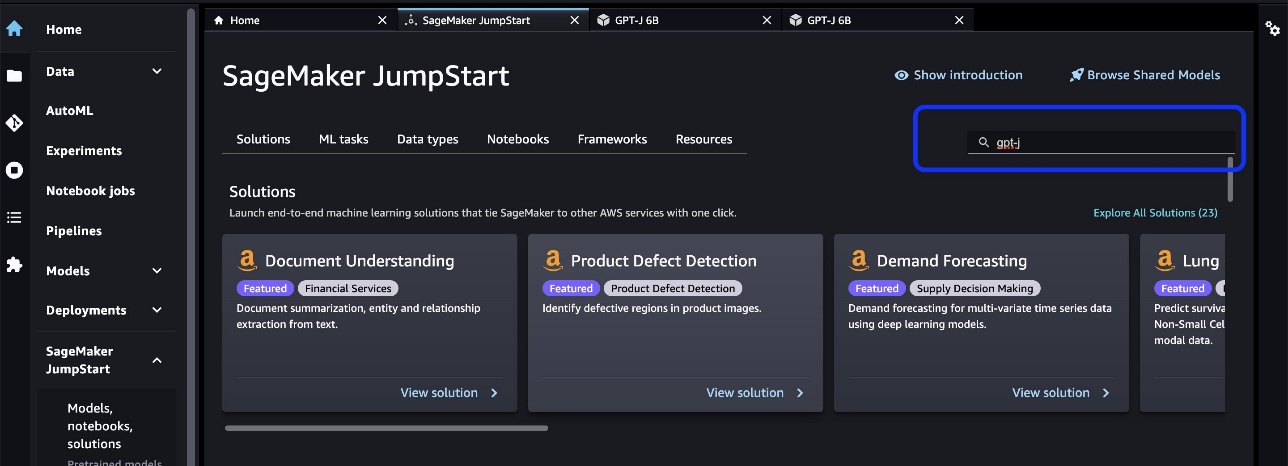
In our case, we chose the GPT-J 6B model card. Here we can directly fine-tune or deploy the LLM.
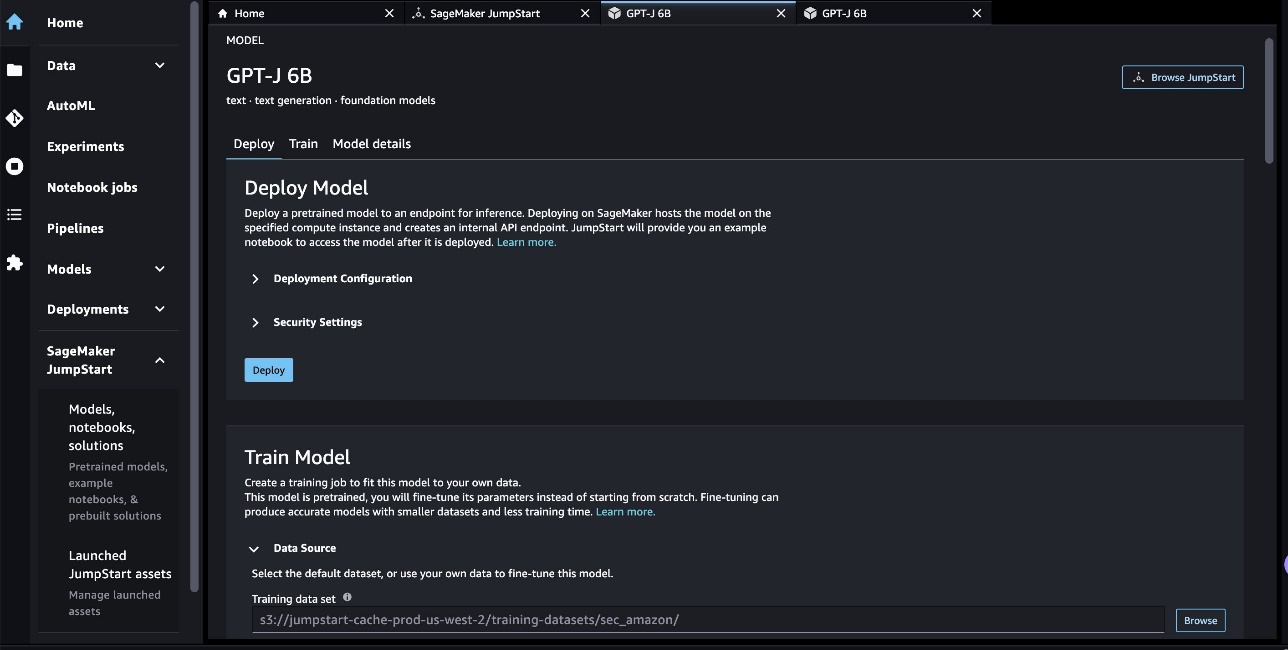
Model evaluation
When evaluating an LLM, we can use perplexity (PPL). PPL is a common measure of how well a language model is able to predict the next word in a sequence. In simpler terms, it’s a way to measure how well the model can understand and generate human-like language.
A lower perplexity score means that the model is shown to perform better at predicting the next word. In practical terms, we can use perplexity to compare different language models and determine which one performs better on a given task. We can also use it to track the performance of a single model over time. For more details, refer to Perplexity of fixed-length models.
We evaluate the model’s performance through a comparison of its pre- and post-fine-tuning performance. PPL is emitted in the training job’s Amazon CloudWatch logs. In addition, we look at the output generated by the model in response to specific test prompts.
Evaluation metric on the validation data |
Before fine-tuning |
After fine-tuning |
Perplexity (PPL) |
8.147 |
1.437 |
The model’s enhanced performance is demonstrated by two experiments:
- Text generation – We show that the output the fine-tuned GPT-J generates text that has a resemblance to the SEC dataset, indicating that it has learned to emulate the language patterns and conventions of the financial regulatory domain
- Zero-shot learning – We demonstrate that the fine-tuned GPT-J shows significantly better performance than the non-fine-tuned GPT-J on zero-shot question answering
For the text generation experiment, we use the following inference parameters to achieve our results: max_length
of 400 (number of tokens returned), num_return_sequences
of 1 (number of response text examples), top_k
of 250 (pick from one of the top 250 possible continuations), top_p
of 0.8 (select only from continuations that are at least 80% probable), do_sample
is True
(sampling), and temperature
of 1 (high temperature is greater randomness in responses). For more details about the parameters, refer to GenerationConfig. The following table compares the fine-tuned GPT-J and non-fine-tuned one on a text completion task.
Inference example |
Output from GPT-J 6B Before Fine-Tuning |
Output from GPT-J 6B After Fine-Tuning |
This Form 10-K report shows that |
This Form 10-K report shows that:
The Companys net income attributable to the Company for the year ended December 31, 2016 was $3,923,000, or $0.21 per diluted share, compared to $3,818,000, or $0.21 per diluted share, for the year ended December 31, 2015.
The Companys net income attributable to the Company for the year ended December 31, 2016 was $4,816,000, or $0.28 per diluted share, compared to $4,726,000, or $0.27 per diluted share, for the year ended December 31, 2015.
The Companys net income attributable to the Company for the year ended December 31, 2016 was $5,828,000, or $0.34 per diluted share, compared to $5,716,000, or $0.33 per diluted share, for the year ended December 31, 2015.
On August 21, 2009, the Company filed a Form 10-Q for the quarter ended December 31, 2008.
On August 21, 2009, the Company filed a Form 10-Q for the quarter ended September 30, 2008.
On August 21, 2009, the Company filed a Form 10-Q for the quarter ended March 31, 2009.
|
This Form 10-K report shows that:(1) The registrant’s name, address, and telephone number, are as follows:Amazon.com, Inc. c/o Ernst & Young LLPSeattle, Washington Attention: Corporate Secretary (2) For the purpose of Article III of the Securities Exchange Act of 1934, the registrant’s name and address are as follows:(3) The registrant’s Exchange Act reportable time period is from and includingJanuary 1, 2020 to the present.(4) The registrant’s subjective assessment is that the financial statements, and other financial information included in this report, fairlypresent in all material respects the financial condition, results of operations and cashflows of the registrant as of, and for, the periods presented in this report.(5) The registrant’s management’s assessment is that the financial statements, and other financial information included in this report, fairlypresent in all material respects the financial condition, results of operations and cashflows of the registrant as of, and for, the periods presented in this report./s/ Ernst & Young LLPSeattle, WashingtonJanuary 29, 2020EX-31.1 5 amzn-20201231xex311.htm EX-31.1 DocumentExhibit 31.1CERTIFICATIONSI, Andrew R. Jassy, certify that:1. I have reviewed this Form 10-K of Amazon.com, Inc.;2. Based on my knowledge, this report does not contain any untrue statementof a material fact or omit to state a material fact necessary to make thestatements made, in light of the circumstances under which such statementswere made, not misleading with respect to the period covered by this report; |
We serve consumers through |
We serve consumers through:
Our Blog
Are you a business owner looking to grow your business? Do you want to create a more effective and efficient business? Do you want to learn how to grow your business? If you answered “yes” to any of these questions, then you need to read this article.
The first step to growing your business is to understand your business. You must be able to answer the following questions about your business:
Who are your customers?
What is your product or service?
What is your unique selling proposition?
What is your marketing strategy?
What is your sales forecast?
What is your sales forecast?
What is your sales forecast?
What is your sales forecast?
What is your sales forecast?
|
We serve consumers through: Amazon.com, a retailer of physical and digital products; Amazon Web Services, which provides technology infrastructure services that enable virtually any type of business; Amazon Enterprise Solutions, which provides companies with custom technology and services to enable better business processes and improve efficiency and productivity; and Audible, a digital audiobook publisher. We serve developers and enterprises of all sizes through AWS, which offers a broad set of global compute, storage, database, and other service offerings. We also manufacture and sell electronic devices. We have organized our operations into two principal segments: North America and International. See Item 8 of Part II, “”Financial Statements and Supplementary Data-Note 12-Segment Information.”” See Item 7 of Part II, “”Management’s Discussion and Analysis of Financial Condition and Results ofOperations-Results of Operations-Supplemental Information”” for supplemental informationabout our net sales.ConsumersWe serve consumers through our online and physical stores and focus on selection,price, and convenience. We design our stores to enable hundreds of millions ofunique products to be sold by us and by third parties across dozens of productcategories. Customers access our offerings through our websites, mobile apps,Alexa, and physically visiting our stores. We also manufacture and sell Kindledevices. We strive to offer our customers the lowest prices possible throughlow everyday product pricing and shipping offers, and to improve ouroperating efficiencies so that we can continue to lower prices for ourcustomers. We also provide easy-to-use functionality, fast and reliablefulfillment, and timely customer service.In addition, we offer Amazon Prime, a membership program that includes unlimitedfree shipping on over 100 million items, access to unlimited streaming of tens ofthousands of movies and TV episodes, and other benefits. |
This year we particularly focus on |
This year we particularly focus on:
The role of the health care system in the prevention of obesity and diabetes
The role of the health care system in the prevention of obesity and diabetes
The role of the health care system in the prevention of obesity and diabetes
The role of the health care system in the prevention of obesity and diabetes
The role of the health care system in the prevention of obesity and diabetes
The role of the health care system in the prevention of obesity and diabetes
The role of the health care system in the prevention of obesity and diabetes
|
This year we particularly focus on: -Continued international expansion, including the development of newbusinesses in existing international market segments, as well as thedevelopment of new international market segments; -Expanding our customer base, including increasing sales to existingcustomers and attracting new customers; -Developing new business opportunities and entering into commercialagreements, including through licensing and other arrangements, with othercompanies, or through the acquisition of other companies; -Developing technology that provides for enhanced customer service andefficiently delivers our products and services; -Developing new product and service offerings and incorporating these intoexisting and new product and service offerings; -Developing efficient and effective systems for supporting our business;and -Other business and strategic initiatives. We believe that offering low prices to our customers is fundamental to ourfuture success. One way we offer lower prices is through free-shipping offersthat result in a net cost to us in delivering products, and through membershipin Amazon Prime, which provides free-shipping on millions of items andaccess to movies and other content. We also offer other promotions that enableus to turn a lower net cost of sales.We have organized our operations into two principal segments: North Americaand International. See Item 8 of Part II, “”Financial Statements andSupplementary Data-Note 12-Segment Information.”” See Item 7 of Part II,””Management’s Discussion and Analysis of Financial Condition and Results ofOperations-Results of Operations-Supplemental Information”” for supplementalinformation about our net sales.ConsumersWe serve consumers through our retail websites and physical stores and focuson selection, price, and convenience. |
For the second experiment with a zero-shot question answering task, we set the max_length
to be a different value (40, 200, and 40, respectively) based on the output we expect from each question. The parameters of top_k
, top_p
, do_sample
, temperature
are set as 250, 0.8, False
, and 0, respectively. We set temperature close to 0 to control the randomness of the output, whereas in the text generation task we set it close to 1 to make the model more creative. The following table compares the results. For each question, the output from fine-tuned GPT-J is more accurate than the non-fine-tuned one.
Input |
Output from GPT-J 6B Before Fine-Tuning |
Output from GPT-J 6B After Fine-Tuning |
What is Item 7 of the 10-K SEC filing about?: |
What is Item 7 of the 10-K SEC filing about?:
Item 7 of the 10-K SEC filing is the following:
“The Company has not received any material adverse
|
Item 7. Management’s Discussion and Analysis of Financial Condition and Results ofOperations Forward-Looking Statements |
How did the COVID-19 pandemic impact Amazon’s business?: |
How did the COVID-19 pandemic impact Amazon’s business?:
Amazon’s business is being impacted by the COVID-19 pandemic in a number of ways.
The company is seeing a decline in the number of Prime memberships, which is a big part of its business.
The company is also seeing a decline in the number of Prime memberships.
Amazon is also seeing a decline in the number of orders placed on its website.
The company is also seeing a decline in the number of people who are shopping on its website.
Amazon is also seeing a decline in the number of people who are shopping on its website.
Amazon is also seeing a decline in the number of people who are shopping on its website.
Amazon is also seeing a decline in the number of people who are shopping on its website.
Amazon is also seeing a decline in the number of people who are shopping
|
How did the COVID-19 pandemic impact Amazon’s business?:The COVID-19 pandemic has impacted our business in several ways. First, it has disrupted our supply chain, and it has affected customer demand, which in turn has affected our sales and operating results. Second, it has increased our spending on advertising and marketing, which may not be effective in the long run. Third, it has increased our spending on technology infrastructure and computing capacity, which may not be effective in the long run. Fourth, it has increased our spending on fulfillment and customer service, which may not be effective in the long run. Finally, it has increased our spending on content, which may not be effective in the long run. See Item 8 of Part II, “Financial Statements and Supplementary Data — Note 1 — Description of Business and Accounting Policies. |
What drives sales growth at Amazon?: |
Amazon is the world’s largest online retailer. It is also the world’s largest online marketplace. It is also the world’ |
Sales growth at Amazon is driven primarily by increased customer usage, including increased selection, lower prices, and increased convenience, and increased sales by other sellers on our websites. |
Clean up
To avoid ongoing charges, delete the SageMaker inference endpoints. You can delete the endpoints via the SageMaker console or from the notebook using the following commands:
# Delete the SageMaker endpoint and the attached resources
finetuned_predictor.delete_model()
finetuned_predictor.delete_endpoint()
Conclusion
JumpStart is a capability in SageMaker that allows you to quickly get started with ML. JumpStart uses open-source, pre-trained models to solve common ML problems like image classification, object detection, text classification, sentence pair classification, and question answering.
In this post, we showed you how to fine-tune and deploy a pre-trained LLM (GPT-J 6B) for text generation based on the SEC filling dataset. We demonstrated how the model transformed into a finance domain expert by undergoing the fine-tuning process on just two annual reports of the company. This fine-tuning enabled the model to generate content with an understanding of financial topics and greater precision. Try out the solution on your own and let us know how it goes in the comments.
Important: This post is for demonstrative purposes only. It is not financial advice and should not be relied on as financial or investment advice. The post used models pre-trained on data obtained from the SEC EDGAR database. You are responsible for complying with EDGAR’s access terms and conditions if you use SEC data.
To learn more about JumpStart, check out the following posts:
About the Authors
Dr. Xin Huang is a Senior Applied Scientist for Amazon SageMaker JumpStart and Amazon SageMaker built-in algorithms. He focuses on developing scalable machine learning algorithms. His research interests are in the area of natural language processing, explainable deep learning on tabular data, and robust analysis of non-parametric space-time clustering. He has published many papers in ACL, ICDM, KDD conferences, and Royal Statistical Society: Series A.
Marc Karp is an ML Architect with the Amazon SageMaker Service team. He focuses on helping customers design, deploy, and manage ML workloads at scale. In his spare time, he enjoys traveling and exploring new places.
Dr. Sanjiv Das is an Amazon Scholar and the Terry Professor of Finance and Data Science at Santa Clara University. He holds post-graduate degrees in Finance (M.Phil and PhD from New York University) and Computer Science (MS from UC Berkeley), and an MBA from the Indian Institute of Management, Ahmedabad. Prior to being an academic, he worked in the derivatives business in the Asia-Pacific region as a Vice President at Citibank. He works on multimodal machine learning in the area of financial applications.
Arun Kumar Lokanatha is a Senior ML Solutions Architect with the Amazon SageMaker Service team. He focuses on helping customers build, train, and migrate ML production workloads to SageMaker at scale. He specializes in deep learning, especially in the area of NLP and CV. Outside of work, he enjoys running and hiking.
Read More