Open-source large language models (LLMs) have become popular, allowing researchers, developers, and organizations to access these models to foster innovation and experimentation. This encourages collaboration from the open-source community to contribute to developments and improvement of LLMs. Open-source LLMs provide transparency to the model architecture, training process, and training data, which allows researchers to understand how the model works and identify potential biases and address ethical concerns. These open-source LLMs are democratizing generative AI by making advanced natural language processing (NLP) technology available to a wide range of users to build mission-critical business applications. GPT-NeoX, LLaMA, Alpaca, GPT4All, Vicuna, Dolly, and OpenAssistant are some of the popular open-source LLMs.
OpenChatKit is an open-source LLM used to build general-purpose and specialized chatbot applications, released by Together Computer in March 2023 under the Apache-2.0 license. This model allows developers to have more control over the chatbot’s behavior and tailor it to their specific applications. OpenChatKit provides a set of tools, base bot, and building blocks to build fully customized, powerful chatbots. The key components are as follows:
- An instruction-tuned LLM, fine-tuned for chat from EleutherAI’s GPT-NeoX-20B with over 43 million instructions on 100% carbon negative compute. The
GPT-NeoXT-Chat-Base-20B
model is based on EleutherAI’s GPT-NeoX model, and is fine-tuned with data focusing on dialog-style interactions.
- Customization recipes to fine-tune the model to achieve high accuracy on your tasks.
- An extensible retrieval system enabling you to augment bot responses with information from a document repository, API, or other live-updating information source at inference time.
- A moderation model, fine-tuned from GPT-JT-6B, designed to filter which questions the bot responds to.
The increasing scale and size of deep learning models present obstacles to successfully deploy these models in generative AI applications. To meet the demands for low latency and high throughput, it becomes essential to employ sophisticated methods like model parallelism and quantization. Lacking proficiency in the application of these methods, numerous users encounter difficulties in initiating the hosting of sizable models for generative AI use cases.
In this post, we show how to deploy OpenChatKit models (GPT-NeoXT-Chat-Base-20B and GPT-JT-Moderation-6B
) models on Amazon SageMaker using DJL Serving and open-source model parallel libraries like DeepSpeed and Hugging Face Accelerate. We use DJL Serving, which is a high-performance universal model serving solution powered by the Deep Java Library (DJL) that is programming language agnostic. We demonstrate how the Hugging Face Accelerate library simplifies deployment of large models into multiple GPUs, thereby reducing the burden of running LLMs in a distributed fashion. Let’s get started!
Extensible retrieval system
An extensible retrieval system is one of the key components of OpenChatKit. It enables you to customize the bot response based on a closed domain knowledge base. Although LLMs are able to retain factual knowledge in their model parameters and can achieve remarkable performance on downstream NLP tasks when fine-tuned, their capacity to access and predict closed domain knowledge accurately remains restricted. Therefore, when they’re presented with knowledge-intensive tasks, their performance suffers to that of task-specific architectures. You can use the OpenChatKit retrieval system to augment knowledge in their responses from external knowledge sources such as Wikipedia, document repositories, APIs, and other information sources.
The retrieval system enables the chatbot to access current information by obtaining pertinent details in response to a specific query, thereby supplying the necessary context for the model to generate answers. To illustrate the functionality of this retrieval system, we provide support for an index of Wikipedia articles and offer example code demonstrating how to invoke a web search API for information retrieval. By following the provided documentation, you can integrate the retrieval system with any dataset or API during the inference process, allowing the chatbot to incorporate dynamically updated data into its responses.
Moderation model
Moderation models are important in chatbot applications to enforce content filtering, quality control, user safety, and legal and compliance reasons. Moderation is a difficult and subjective task, and depends a lot on the domain of the chatbot application. OpenChatKit provides tools to moderate the chatbot application and monitor input text prompts for any inappropriate content. The moderation model provides a good baseline that can be adapted and customized to various needs.
OpenChatKit has a 6-billion-parameter moderation model, GPT-JT-Moderation-6B
, which can moderate the chatbot to limit the inputs to the moderated subjects. Although the model itself does have some moderation built in, TogetherComputer trained a GPT-JT-Moderation-6B model with Ontocord.ai’s OIG-moderation dataset. This model runs alongside the main chatbot to check that both the user input and answer from the bot don’t contain inappropriate results. You can also use this to detect any out of domain questions to the chatbot and override when the question is not part of the chatbot’s domain.
The following diagram illustrates the OpenChatKit workflow.
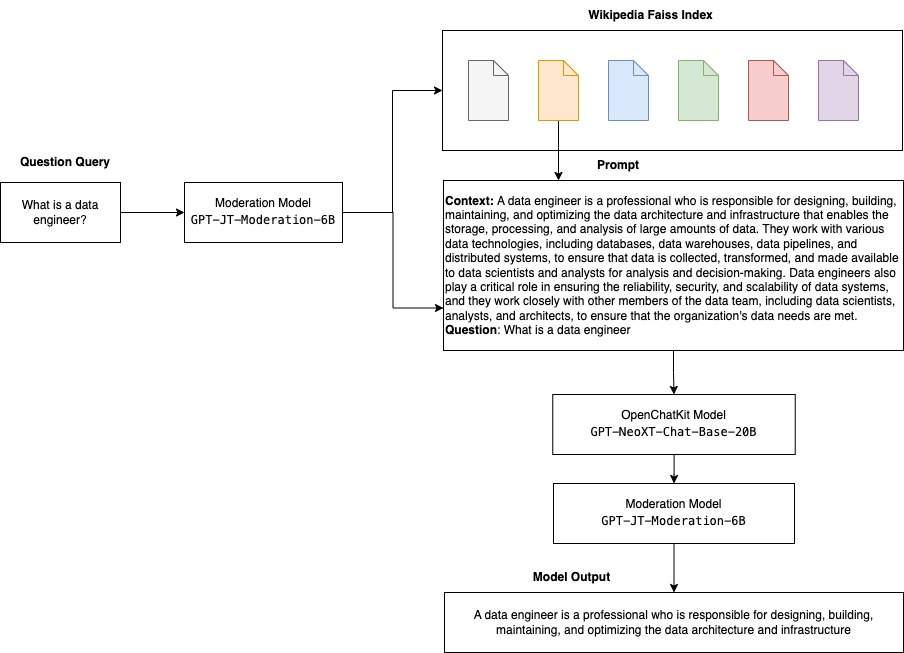
Extensible retrieval system use cases
Although we can apply this technique in various industries to build generative AI applications, for this post we discuss use cases in the financial industry. Retrieval augmented generation can be employed in financial research to automatically generate research reports on specific companies, industries, or financial products. By retrieving relevant information from internal knowledge bases, financial archives, news articles, and research papers, you can generate comprehensive reports that summarize key insights, financial metrics, market trends, and investment recommendations. You can use this solution to monitor and analyze financial news, market sentiment, and trends.
Solution overview
The following steps are involved to build a chatbot using OpenChatKit models and deploy them on SageMaker:
- Download the chat base
GPT-NeoXT-Chat-Base-20B
model and package the model artifacts to be uploaded to Amazon Simple Storage Service (Amazon S3).
- Use a SageMaker large model inference (LMI) container, configure the properties, and set up custom inference code to deploy this model.
- Configure model parallel techniques and use inference optimization libraries in DJL serving properties. We will use Hugging Face Accelerate as the engine for DJL serving. Additionally, we define tensor parallel configurations to partition the model.
- Create a SageMaker model and endpoint configuration, and deploy the SageMaker endpoint.
You can follow along by running the notebook in the GitHub repo.
Download the OpenChatKit model
First, we download the OpenChatKit base model. We use huggingface_hub
and use snapshot_download to download the model, which downloads an entire repository at a given revision. Downloads are made concurrently to speed up the process. See the following code:
from huggingface_hub import snapshot_download
from pathlib import Path
import os
# - This will download the model into the current directory where ever the jupyter notebook is running
local_model_path = Path("./openchatkit")
local_model_path.mkdir(exist_ok=True)
model_name = "togethercomputer/GPT-NeoXT-Chat-Base-20B"
# Only download pytorch checkpoint files
allow_patterns = ["*.json", "*.pt", "*.bin", "*.txt", "*.model"]
# - Leverage the snapshot library to donload the model since the model is stored in repository using LFS
chat_model_download_path = snapshot_download(
repo_id=model_name,#A user or an organization name and a repo name
cache_dir=local_model_path, #Path to the folder where cached files are stored.
allow_patterns=allow_patterns, #only files matching at least one pattern are downloaded.
)
DJL Serving properties
You can use SageMaker LMI containers to host large generative AI models with custom inference code without providing your own inference code. This is extremely useful when there is no custom preprocessing of the input data or postprocessing of the model’s predictions. You can also deploy a model using custom inference code. In this post, we demonstrate how to deploy OpenChatKit models with custom inference code.
SageMaker expects the model artifacts in tar format. We create each OpenChatKit model with the following files: serving.properties
and model.py
.
The serving.properties
configuration file indicates to DJL Serving which model parallelization and inference optimization libraries you would like to use. The following is a list of settings we use in this configuration file:
openchatkit/serving.properties
engine = Python
option.tensor_parallel_degree = 4
option.s3url = {{s3url}}
This contains the following parameters:
- engine – The engine for DJL to use.
- option.entryPoint – The entry point Python file or module. This should align with the engine that is being used.
- option.s3url – Set this to the URI of the S3 bucket that contains the model.
- option.modelid – If you want to download the model from huggingface.co, you can set
option.modelid
to the model ID of a pretrained model hosted inside a model repository on huggingface.co (https://huggingface.co/models). The container uses this model ID to download the corresponding model repository on huggingface.co.
- option.tensor_parallel_degree – Set this to the number of GPU devices over which DeepSpeed needs to partition the model. This parameter also controls the number of workers per model that will be started up when DJL Serving runs. For example, if we have an 8 GPU machine and we are creating eight partitions, then we will have one worker per model to serve the requests. It’s necessary to tune the parallelism degree and identify the optimal value for a given model architecture and hardware platform. We call this ability inference-adapted parallelism.
Refer to Configurations and settings for an exhaustive list of options.
OpenChatKit models
The OpenChatKit base model implementation has the following four files:
- model.py – This file implements the handling logic for the main OpenChatKit GPT-NeoX model. It receives the inference input request, loads the model, loads the Wikipedia index, and serves the response. Refer to
model.py
(created part of the notebook) for additional details. model.py
uses the following key classes:
- OpenChatKitService – This handles passing the data between the GPT-NeoX model, Faiss search, and conversation object.
WikipediaIndex
and Conversation
objects are initialized and input chat conversations are sent to the index to search for relevant content from Wikipedia. This also generates a unique ID for each invocation if one is not supplied for the purpose of storing the prompts in Amazon DynamoDB.
- ChatModel – This class loads the model and tokenizer and generates the response. It handles partitioning the model across multiple GPUs using
tensor_parallel_degree
, and configures the dtypes
and device_map
. The prompts are passed to the model to generate responses. A stopping criteria StopWordsCriteria
is configured for the generation to only produce the bot response on inference.
- ModerationModel – We use two moderation models in the
ModerationModel
class: the input model to indicate to the chat model that the input is inappropriate to override the inference result, and the output model to override the inference result. We classify the input prompt and output response with the following possible labels:
- casual
- needs caution
- needs intervention (this is flagged to be moderated by the model)
- possibly needs caution
- probably needs caution
- wikipedia_prepare.py – This file handles downloading and preparing the Wikipedia index. In this post, we use a Wikipedia index provided on Hugging Face datasets. To search the Wikipedia documents for relevant text, the index needs to be downloaded from Hugging Face because it’s not packaged elsewhere. The
wikipedia_prepare.py
file is responsible for handling the download when imported. Only a single process in the multiple that are running for inference can clone the repository. The rest wait until the files are present in the local file system.
- wikipedia.py – This file is used for searching the Wikipedia index for contextually relevant documents. The input query is tokenized and embeddings are created using
mean_pooling
. We compute cosine similarity distance metrics between the query embedding and the Wikipedia index to retrieve contextually relevant Wikipedia sentences. Refer to wikipedia.py
for implementation details.
#function to create sentence embedding using mean_pooling
def mean_pooling(token_embeddings, mask):
token_embeddings = token_embeddings.masked_fill(~mask[..., None].bool(), 0.0)
sentence_embeddings = token_embeddings.sum(dim=1) / mask.sum(dim=1)[..., None]
return sentence_embeddings
#function to compute cosine similarity distance between 2 embeddings
def cos_sim_2d(x, y):
norm_x = x / np.linalg.norm(x, axis=1, keepdims=True)
norm_y = y / np.linalg.norm(y, axis=1, keepdims=True)
return np.matmul(norm_x, norm_y.T)
- conversation.py – This file is used for storing and retrieving the conversation thread in DynamoDB for passing to the model and user.
conversation.py
is adapted from the open-source OpenChatKit repository. This file is responsible for defining the object that stores the conversation turns between the human and the model. With this, the model is able to retain a session for the conversation, allowing a user to refer to previous messages. Because SageMaker endpoint invocations are stateless, this conversation needs to be stored in a location external to the endpoint instances. On startup, the instance creates a DynamoDB table if it doesn’t exist. All updates to the conversation are then stored in DynamoDB based on the session_id
key, which is generated by the endpoint. Any invocation with a session ID will retrieve the associated conversation string and update it as required.
Build an LMI inference container with custom dependencies
The index search uses Facebook’s Faiss library for performing the similarity search. Because this isn’t included in the base LMI image, the container needs to be adapted to install this library. The following code defines a Dockerfile that installs Faiss from the source alongside other libraries needed by the bot endpoint. We use the sm-docker
utility to build and push the image to Amazon Elastic Container Registry (Amazon ECR) from Amazon SageMaker Studio. Refer to Using the Amazon SageMaker Studio Image Build CLI to build container images from your Studio notebooks for more details.
The DJL container doesn’t have Conda installed, so Faiss needs to be cloned and compiled from the source. To install Faiss, the dependencies for using the BLAS APIs and Python support need to be installed. After these packages are installed, Faiss is configured to use AVX2 and CUDA before being compiled with the Python extensions installed.
pandas
, fastparquet
, boto3
, and git-lfs
are installed afterwards because these are required for downloading and reading the index files.
FROM 763104351884.dkr.ecr.us-east-1.amazonaws.com/djl-inference:0.21.0-deepspeed0.8.0-cu117
ARG FAISS_URL=https://github.com/facebookresearch/faiss.git
RUN apt-get update && apt-get install -y git-lfs wget cmake pkg-config build-essential apt-utils
RUN apt search openblas && apt-get install -y libopenblas-dev swig
RUN git clone $FAISS_URL &&
cd faiss &&
cmake -B build . -DFAISS_OPT_LEVEL=avx2 -DCMAKE_CUDA_ARCHITECTURES="86" &&
make -C build -j faiss &&
make -C build -j swigfaiss &&
make -C build -j swigfaiss_avx2 &&
(cd build/faiss/python && python -m pip install )
RUN pip install pandas fastparquet boto3 &&
git lfs install --skip-repo &&
apt-get clean all
Create the model
Now that we have the Docker image in Amazon ECR, we can proceed with creating the SageMaker model object for the OpenChatKit models. We deploy GPT-NeoXT-Chat-Base-20B
input and output moderation models using GPT-JT-Moderation-6B
. Refer to create_model for more details.
from sagemaker.utils import name_from_base
chat_model_name = name_from_base(f"gpt-neoxt-chatbase-ds")
print(chat_model_name)
create_model_response = sm_client.create_model(
ModelName=chat_model_name,
ExecutionRoleArn=role,
PrimaryContainer={
"Image": chat_inference_image_uri,
"ModelDataUrl": s3_code_artifact,
},
)
chat_model_arn = create_model_response["ModelArn"]
print(f"Created Model: {chat_model_arn}")
Configure the endpoint
Next, we define the endpoint configurations for the OpenChatKit models. We deploy the models using the ml.g5.12xlarge instance type. Refer to create_endpoint_config for more details.
chat_endpoint_config_name = f"{chat_model_name}-config"
chat_endpoint_name = f"{chat_model_name}-endpoint"
chat_endpoint_config_response = sm_client.create_endpoint_config(
EndpointConfigName=chat_endpoint_config_name,
ProductionVariants=[
{
"VariantName": "variant1",
"ModelName": chat_model_name,
"InstanceType": "ml.g5.12xlarge",
"InitialInstanceCount": 1,
"ContainerStartupHealthCheckTimeoutInSeconds": 3600,
},
],
)
Deploy the endpoint
Finally, we create an endpoint using the model and endpoint configuration we defined in the previous steps:
chat_create_endpoint_response = sm_client.create_endpoint(
EndpointName=f"{chat_endpoint_name}", EndpointConfigName=chat_endpoint_config_name
)
print(f"Created Endpoint: {chat_create_endpoint_response['EndpointArn']},")
Run inference from OpenChatKit models
Now it’s time to send inference requests to the model and get the responses. We pass the input text prompt and model parameters such as temperature
, top_k
, and max_new_tokens
. The quality of the chatbot responses is based on the parameters specified, so it’s recommended to benchmark model performance against these parameters to find the optimal setting for your use case. The input prompt is first sent to the input moderation model, and the output is sent to ChatModel
to generate the responses. During this step, the model uses the Wikipedia index to retrieve contextually relevant sections to the model as the prompt to get domain-specific responses from the model. Finally, the model response is sent to the output moderation model to check for classification, and then the responses are returned. See the following code:
def chat(prompt, session_id=None, **kwargs):
if session_id:
chat_response_model = smr_client.invoke_endpoint(
EndpointName=chat_endpoint_name,
Body=json.dumps(
{
"inputs": prompt,
"parameters": {
"temperature": 0.6,
"top_k": 40,
"max_new_tokens": 512,
"session_id": session_id,
"no_retrieval": True,
},
}
),
ContentType="application/json",
)
else:
chat_response_model = smr_client.invoke_endpoint(
EndpointName=chat_endpoint_name,
Body=json.dumps(
{
"inputs": prompt,
"parameters": {
"temperature": 0.6,
"top_k": 40,
"max_new_tokens": 512,
},
}
),
ContentType="application/json",
)
response = chat_response_model["Body"].read().decode("utf8")
return response
prompts = "What does a data engineer do?"
chat(prompts)
Refer to sample chat interactions below.
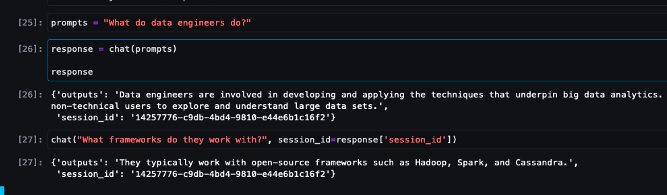
Clean up
Follow the instructions in the cleanup section of the to delete the resources provisioned as part of this post to avoid unnecessary charges. Refer to Amazon SageMaker Pricing for details about the cost of the inference instances.
Conclusion
In this post, we discussed the importance of open-source LLMs and how to deploy an OpenChatKit model on SageMaker to build next-generation chatbot applications. We discussed various components of OpenChatKit models, moderation models, and how to use an external knowledge source like Wikipedia for retrieval augmented generation (RAG) workflows. You can find step-by-step instructions in the GitHub notebook. Let us know about the amazing chatbot applications you’re building. Cheers!
About the Authors
Dhawal Patel is a Principal Machine Learning Architect at AWS. He has worked with organizations ranging from large enterprises to mid-sized startups on problems related to distributed computing, and Artificial Intelligence. He focuses on Deep learning including NLP and Computer Vision domains. He helps customers achieve high performance model inference on SageMaker.
Vikram Elango is a Sr. AIML Specialist Solutions Architect at AWS, based in Virginia, US. He is currently focused on generative AI, LLMs, prompt engineering, large model inference optimization, and scaling ML across enterprises. Vikram helps financial and insurance industry customers with design and thought leadership to build and deploy machine learning applications at scale. In his spare time, he enjoys traveling, hiking, cooking, and camping with his family.
Andrew Smith is a Cloud Support Engineer in the SageMaker, Vision & Other team at AWS, based in Sydney, Australia. He supports customers using many AI/ML services on AWS with expertise in working with Amazon SageMaker. Outside of work, he enjoys spending time with friends and family as well as learning about different technologies.
Read More