Amazon SageMaker JumpStart is a machine learning (ML) hub that can help you accelerate your ML journey. With SageMaker JumpStart, you can discover and deploy publicly available and proprietary foundation models to dedicated Amazon SageMaker instances for your generative AI applications. SageMaker JumpStart allows you to deploy foundation models from a network isolated environment, and doesn’t share customer training and inference data with model providers.
In this post, we walk through how to get started with proprietary models from model providers such as AI21, Cohere, and LightOn from Amazon SageMaker Studio. SageMaker Studio is a notebook environment where SageMaker enterprise data scientist customers evaluate and build models for their next generative AI applications.
Foundation models in SageMaker
Foundation models are large-scale ML models that contain billions of parameters and are pre-trained on terabytes of text and image data so you can perform a wide range of tasks, such as article summarization and text, image, or video generation. Because foundation models are pre-trained, they can help lower training and infrastructure costs and enable customization for your use case.
SageMaker JumpStart provides two types of foundation models:
- Proprietary models – These models are from providers such as AI21 with Jurassic-2 models, Cohere with Cohere Command, and LightOn with Mini trained on proprietary algorithms and data. You can’t view model artifacts such as weight and scripts, but you can still deploy to SageMaker instances for inferencing.
- Publicly available models – These are from popular model hubs such as Hugging Face with Stable Diffusion, Falcon, and FLAN trained on publicly available algorithms and data. For these models, users have access to model artifacts and are able to fine-tune with their own data prior to deployment for inferencing.
Discover models
You can access the foundation models through SageMaker JumpStart in the SageMaker Studio UI and the SageMaker Python SDK. In this section, we go over how to discover the models in the SageMaker Studio UI.
SageMaker Studio is a web-based integrated development environment (IDE) for ML that lets you build, train, debug, deploy, and monitor your ML models. For more details on how to get started and set up SageMaker Studio, refer to Amazon SageMaker Studio.
Once you’re on the SageMaker Studio UI, you can access SageMaker JumpStart, which contains pre-trained models, notebooks, and prebuilt solutions, under Prebuilt and automated solutions.
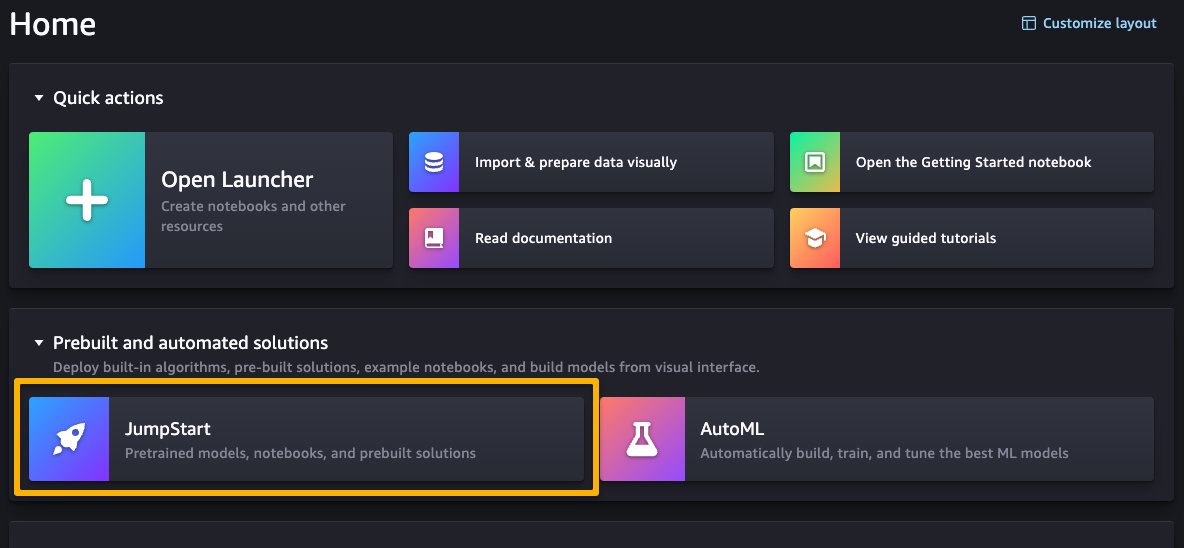
From the SageMaker JumpStart landing page, you can browse for solutions, models, notebooks, and other resources. The following screenshot shows an example of the landing page with solutions and foundation models listed.
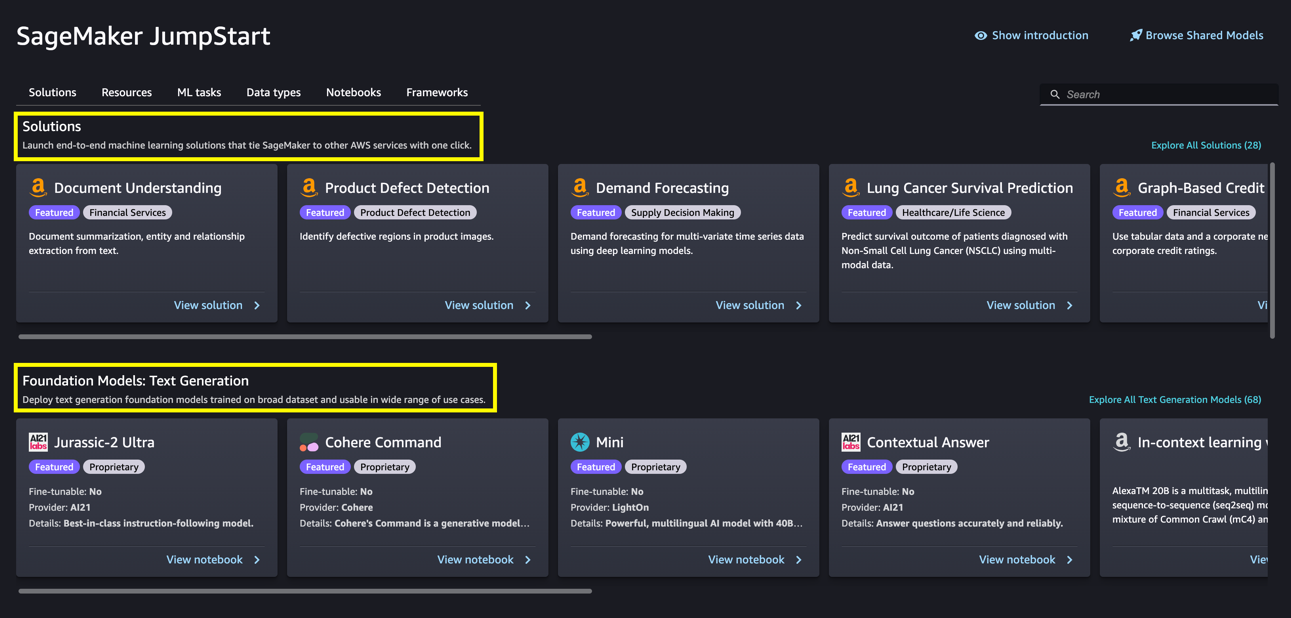
Each model has a model card, as shown in the following screenshot, which contains the model name, if it is fine-tunable or not, the provider name, and a short description about the model. You can also open the model card to learn more about the model and start training or deploying.
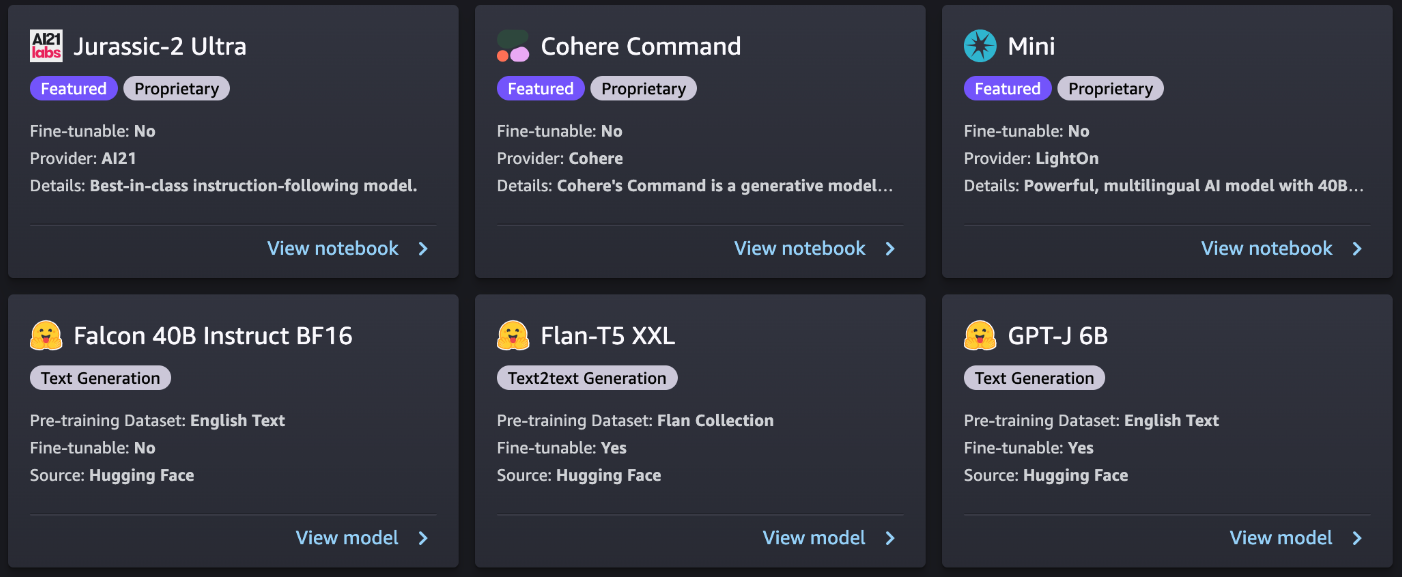
Subscribe in AWS Marketplace
Proprietary models in SageMaker JumpStart are published by model providers such as AI21, Cohere, and LightOn. You can identify proprietary models by the “Proprietary” tag on model cards, as shown in the following screenshot.
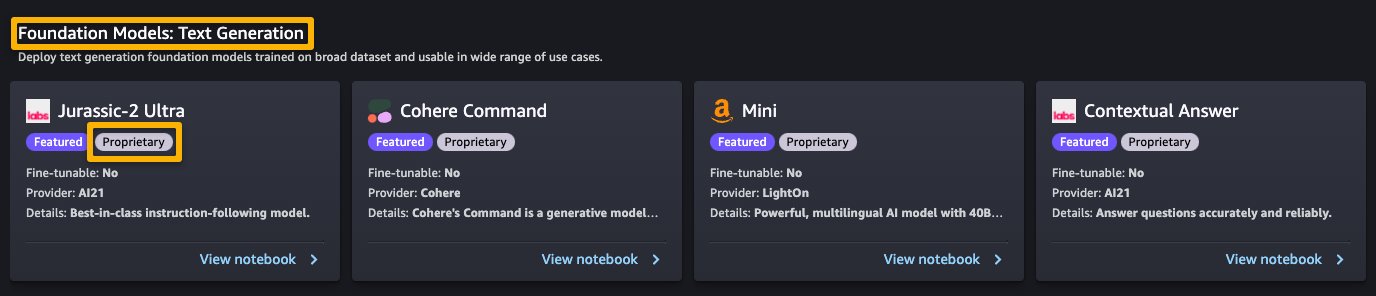
You can choose View notebook on the model card to open the notebook in read-only mode, as shown in the following screenshot. You can read the notebook for important information regarding prerequisites and other usage instructions.
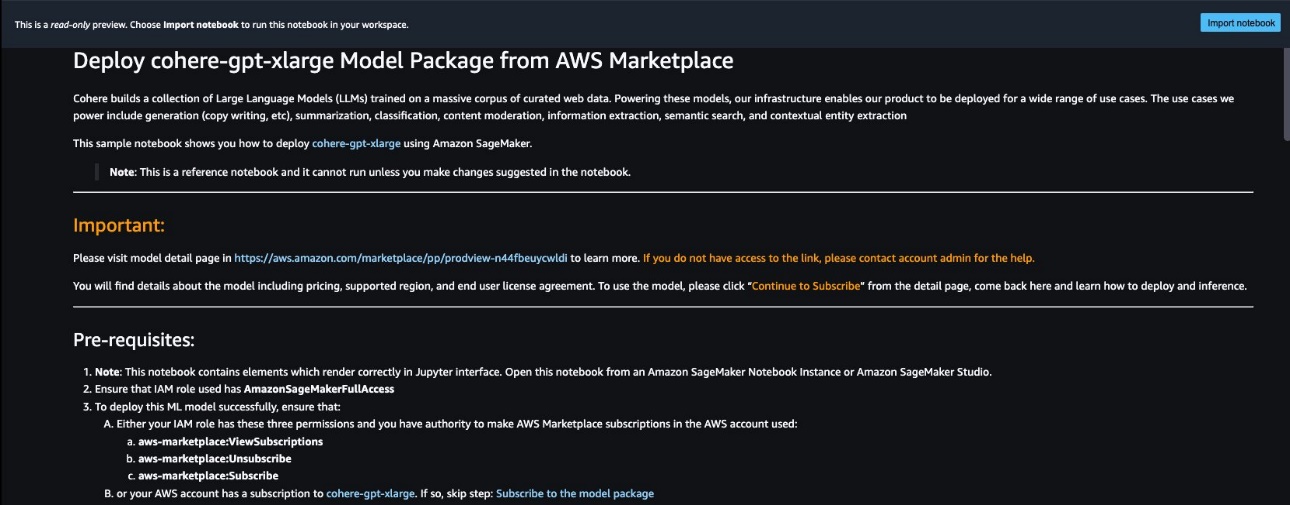
After importing the notebook, you need to select the appropriate notebook environment (image, kernel, instance type, and so on) before running codes. You should also follow the subscription and usage instructions per the selected notebook.
Before using a proprietary model, you need to first subscribe to the model from AWS Marketplace:
- Open the model listing page in AWS Marketplace.
The URL is provided in the Important section of the notebook, or you can access it from the SageMaker JumpStart service page. The listing page shows the overview, pricing, usage, and support information about the model.
- On the AWS Marketplace listing, choose Continue to subscribe.
If you don’t have the necessary permissions to view or subscribe to the model, reach out to your IT admin or procurement point of contact to subscribe to the model for you. Many enterprises may limit AWS Marketplace permissions to control the actions that someone with those permissions can take in the AWS Marketplace Management Portal.
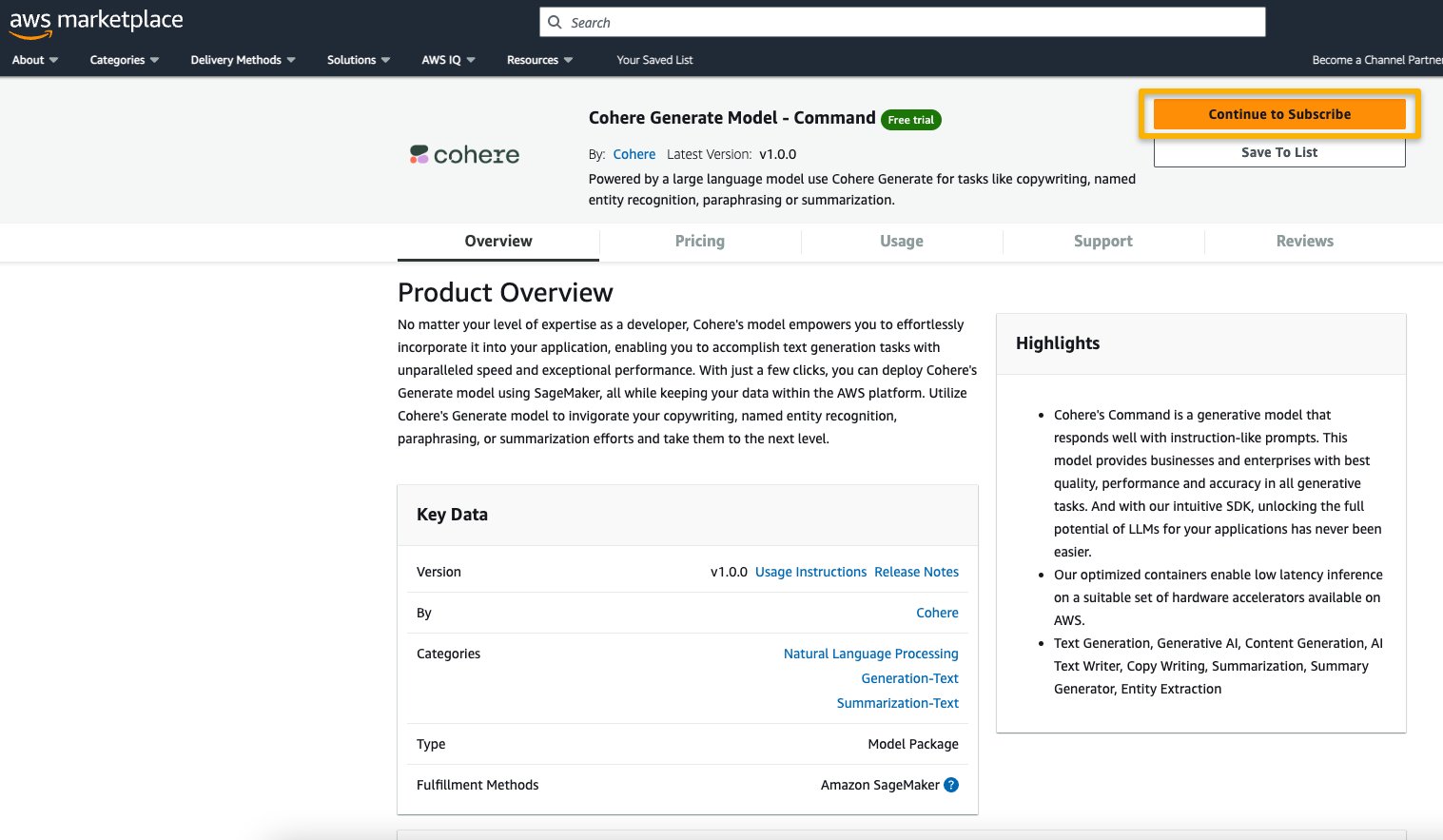
- On the Subscribe to this software page, review the details and choose Accept offer if you and your organization agree with the EULA, pricing, and support terms.
If you have any questions or a request for volume discount, reach out to the model provider directly via the support email provided on the detail page or reach out to your AWS account team.
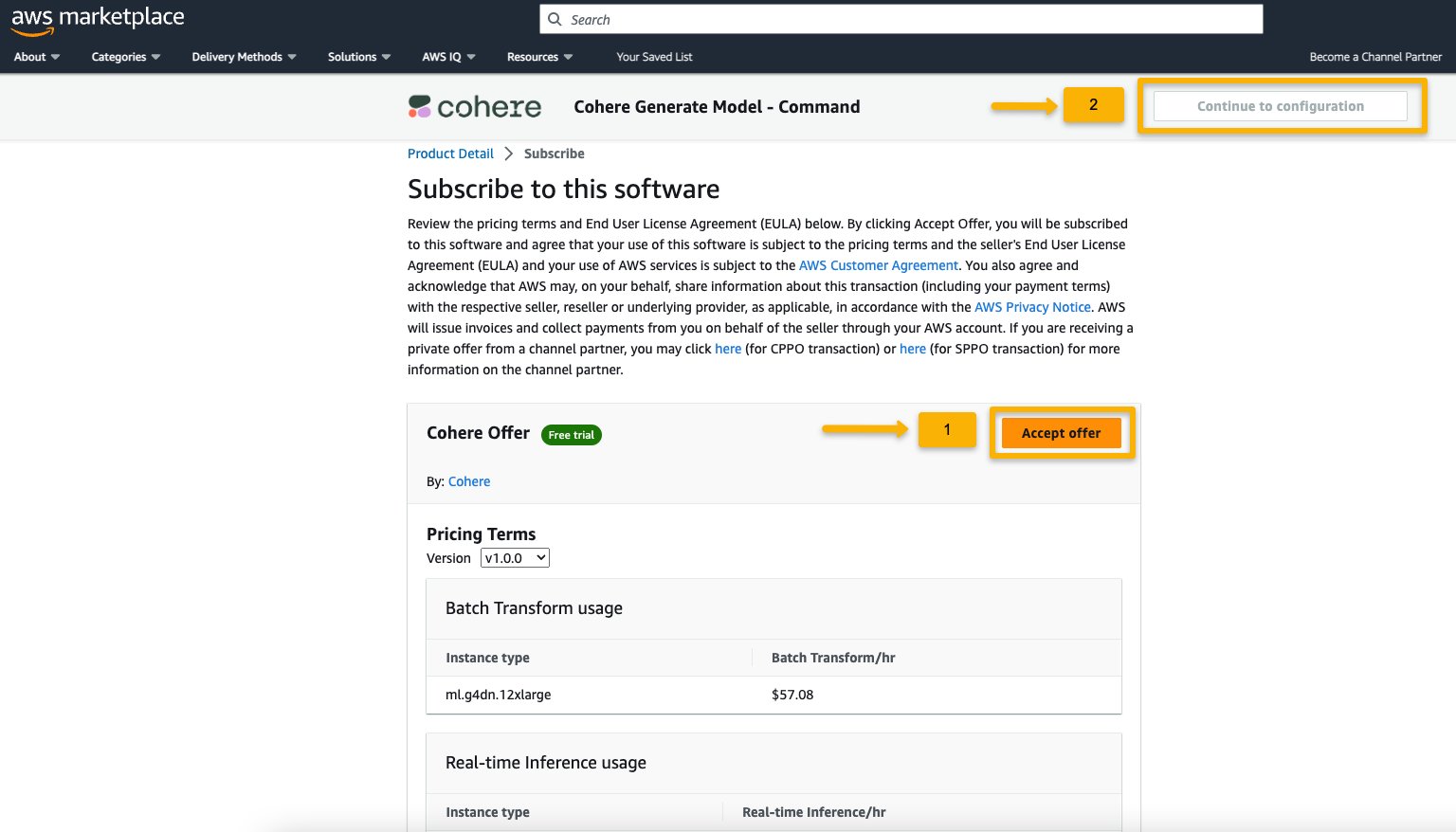
- Choose Continue to configuration and choose a Region.
You will see a product ARN displayed. This is the model package ARN that you need to specify while creating a deployable model using Boto3.
- Copy the ARN corresponding to your Region and specify the same in the notebook’s cell instruction.
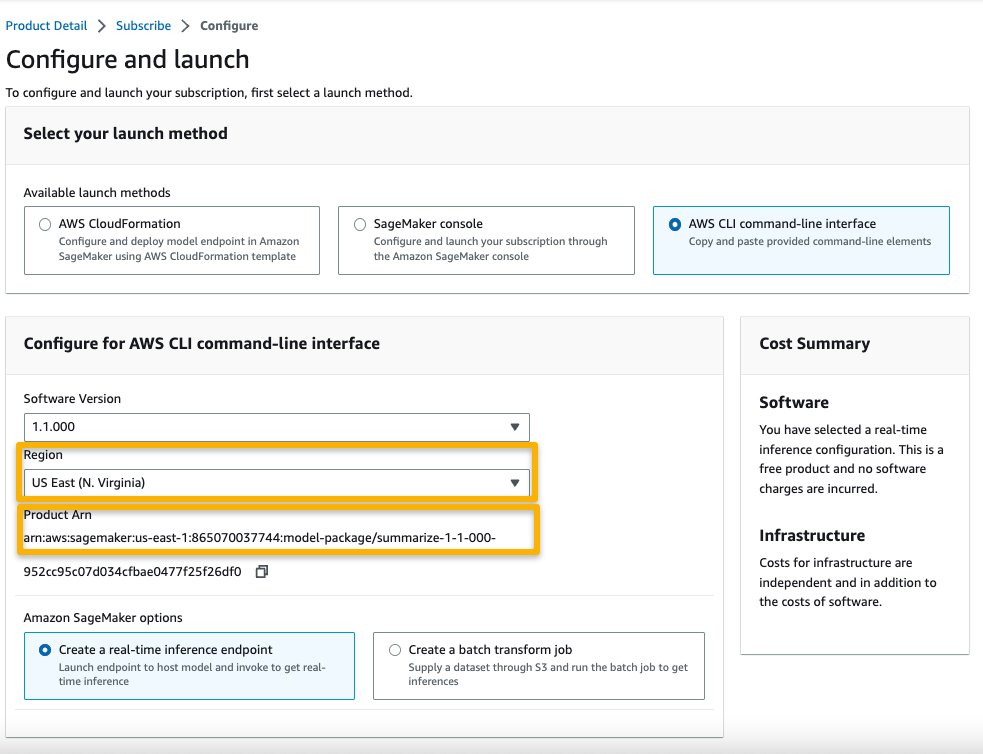
Sample inferencing with sample prompts
Let’s look at some of the sample foundation models from A21 Labs, Cohere, and LightOn that are discoverable from SageMaker JumpStart in SageMaker Studio. All of them have same the instructions to subscribe from AWS Marketplace and import and configure the notebook.
AI21 Summarize
The Summarize model by A121 Labs condenses lengthy texts into short, easy-to-read bites that remain factually consistent with the source. The model is trained to generate summaries that capture key ideas based on a body of text. It doesn’t require any prompting. You simply input the text that needs to be summarized. Your source text can contain up to 50,000 characters, translating to roughly 10,000 words, or an impressive 40 pages.
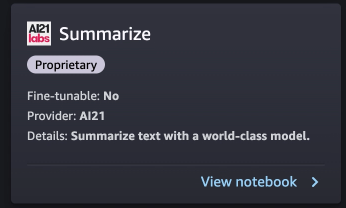
The sample notebook for AI21 Summarize model provides important prerequisites that needs to be followed. For example the model is subscribed from AWS Marketplace , have appropriate IAM roles permissions, and required boto3 version etc. It walks you through how to select the model package, create endpoints for real-time inference, and then clean up.
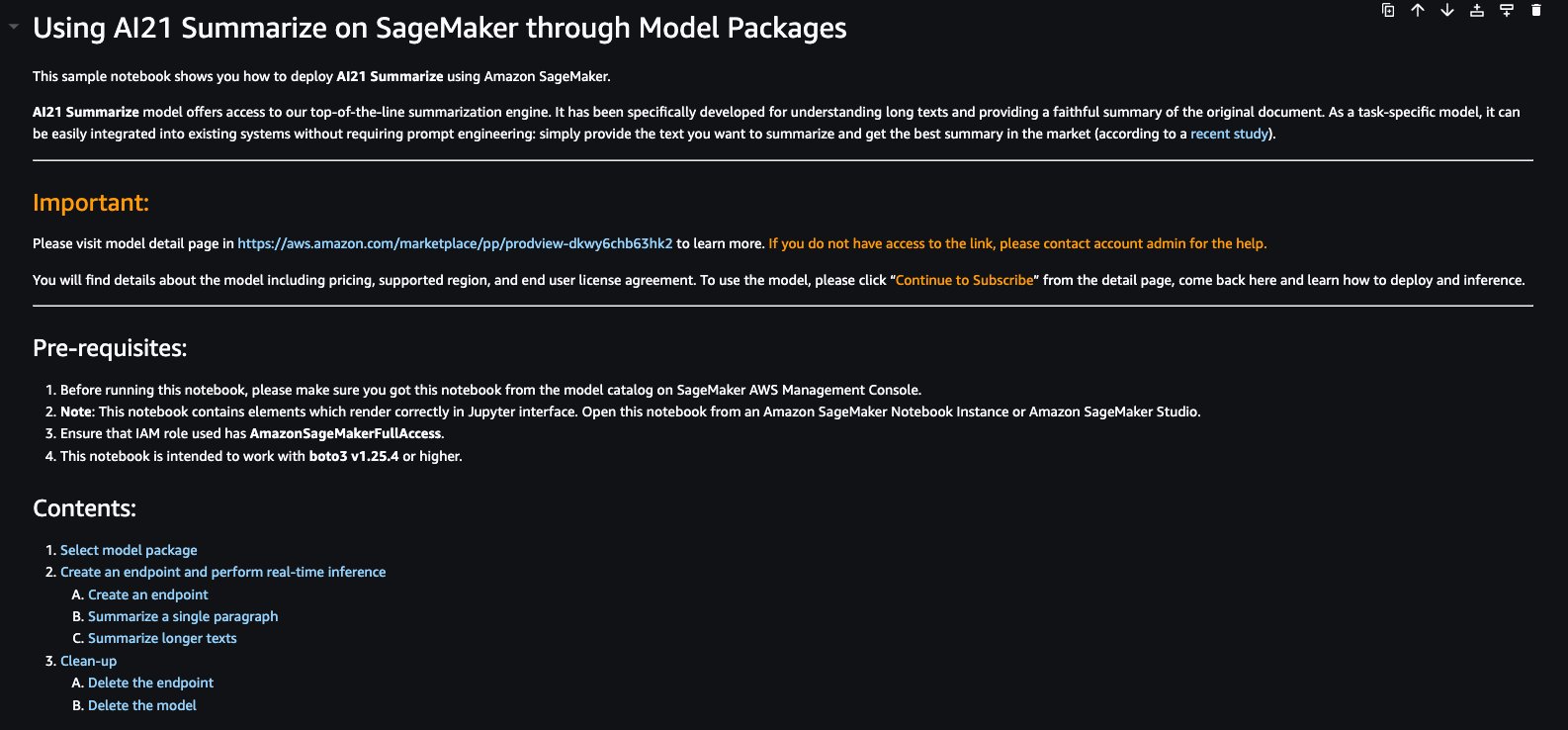
The selected model package contains the mapping of ARNs to Regions. This is the information you captured after choosing Continue to configuration on the AWS Marketplace subscription page (in the section Evaluate and subscribe in Marketplace) and then selecting a Region for which you will see the corresponding product ARN.
The notebook may already have ARN prepopulated.

You then import some libraries required to run this notebook and install wikipedia, which is a Python library that makes it easy to access and parse data from Wikipedia. The notebook uses this later to showcase how to summarize a long text from Wikipedia.
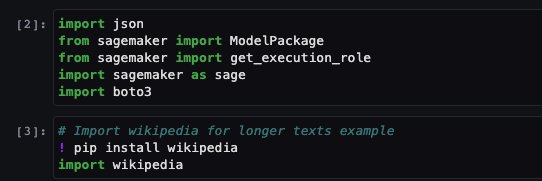
The notebook also proceeds to install the ai21
Python SDK, which is a wrapper around SageMaker APIs such as deploy
and invoke endpoint
.
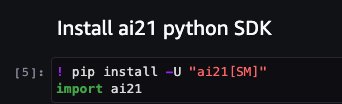
The next few cells of the notebook walk through the following steps:
- Select the Region and fetch the model package ARN from model package map
- Create your inference endpoint by selecting an instance type (depending on your use case and supported instance for the model; see Task-specific models for more details) to run the model on
- Create a deployable model from the model package
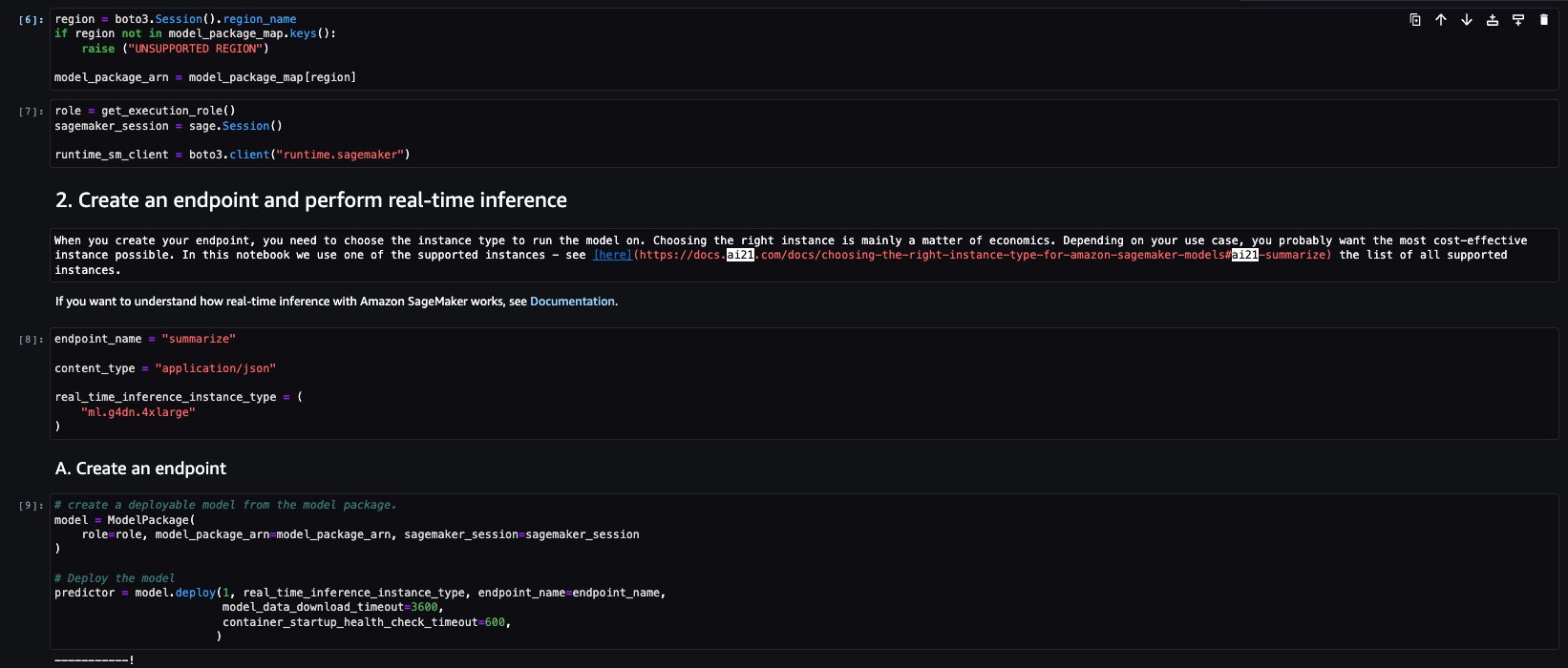
Let’s run the inference to generate a summary of a single paragraph taken from a news article. As you can see in the output, the summarized text is presented as an output by the model.
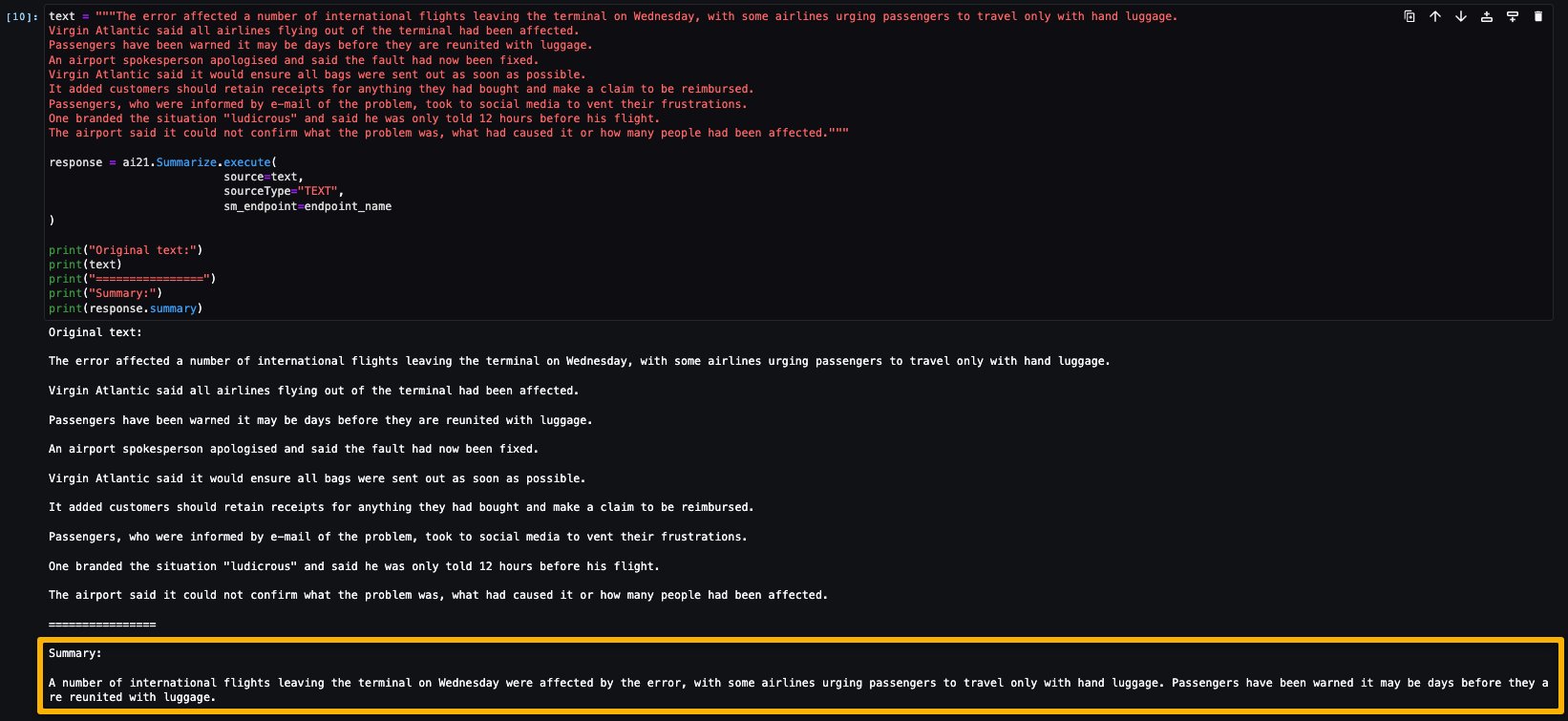
AI21 Summarize can handle inputs up to 50,000 characters. This translates into roughly 10,000 words, or 40 pages. As a demonstration of the model’s behavior, we load a page from Wikipedia.
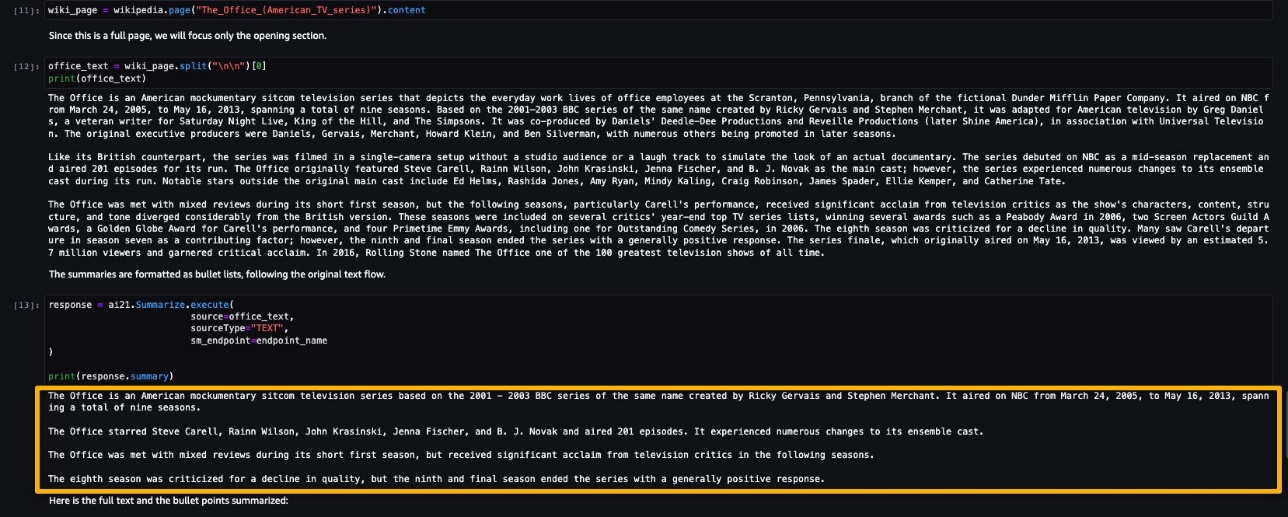
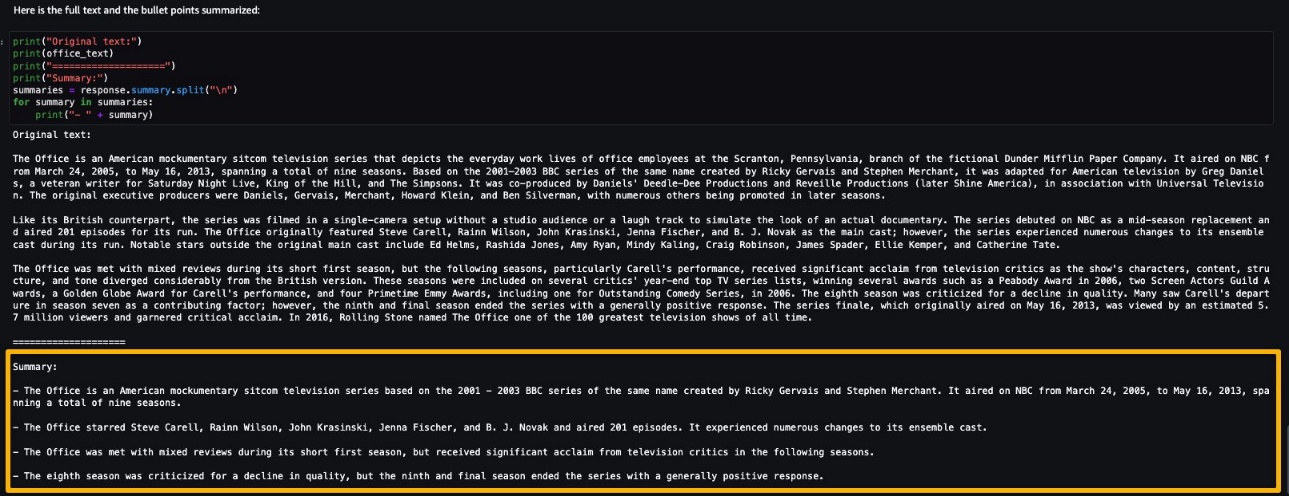
Now that you have performed a real-time inference for testing, you may not need the endpoint anymore. You can delete the endpoint to avoid being charged.
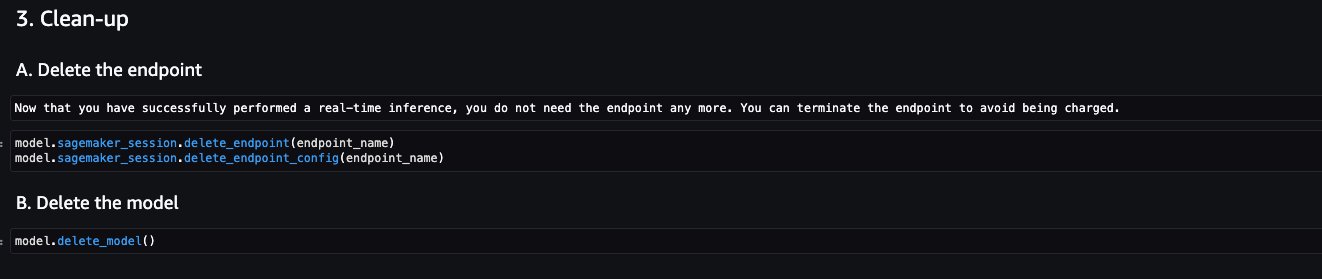
Cohere Command
Cohere Command is a generative model that responds well with instruction-like prompts. This model provides businesses and enterprises with best quality, performance, and accuracy in all generative tasks. You can use Cohere’s Command model to invigorate your copywriting, named entity recognition, paraphrasing, or summarization efforts and take them to the next level.
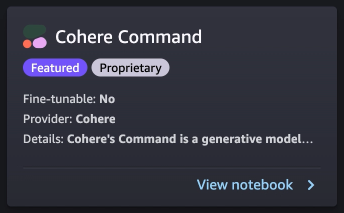
The sample notebook for Cohere Command model provides important prerequisites that needs to be followed. For example the model is subscribed from AWS Marketplace, have appropriate IAM roles permissions, and required boto3 version etc. It walks you through how to select the model package, create endpoints for real-time inference, and then clean up.
Some of the tasks are similar to those covered in the previous notebook example, like installing Boto3, installing cohere-sagemaker
(the package provides functionality developed to simplify interfacing with the Cohere model), and getting the session and Region.
Let’s explore creating the endpoint. You provide the model package ARN, endpoint name, instance type to be used, and number of instances. Once created, the endpoint appears in your endpoint section of SageMaker.

Now let’s run the inference to see some of the outputs from the Command model.
The following screenshot shows a sample example of generating a job post and its output. As you can see, the model generated a post from the given prompt.
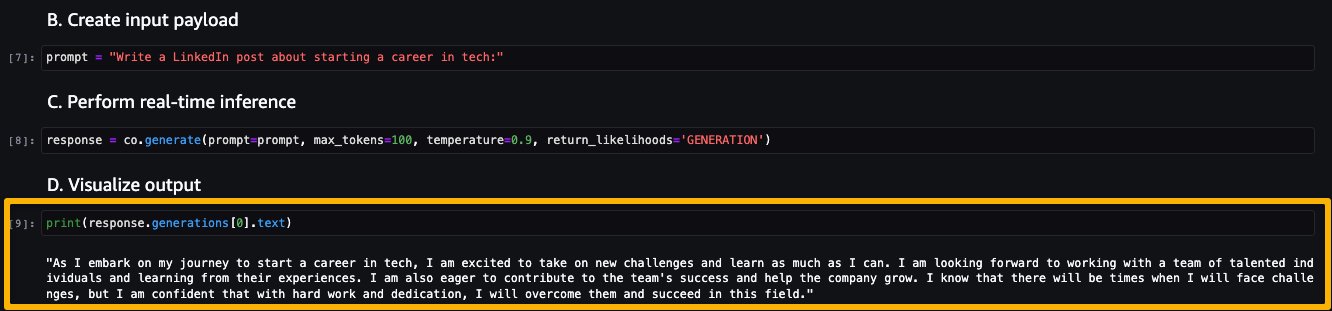
Now let’s look at the following examples:
- Generate a product description
- Generate a body paragraph of a blog post
- Generate an outreach email
As you can see, the Cohere Command model generated text for various generative tasks.
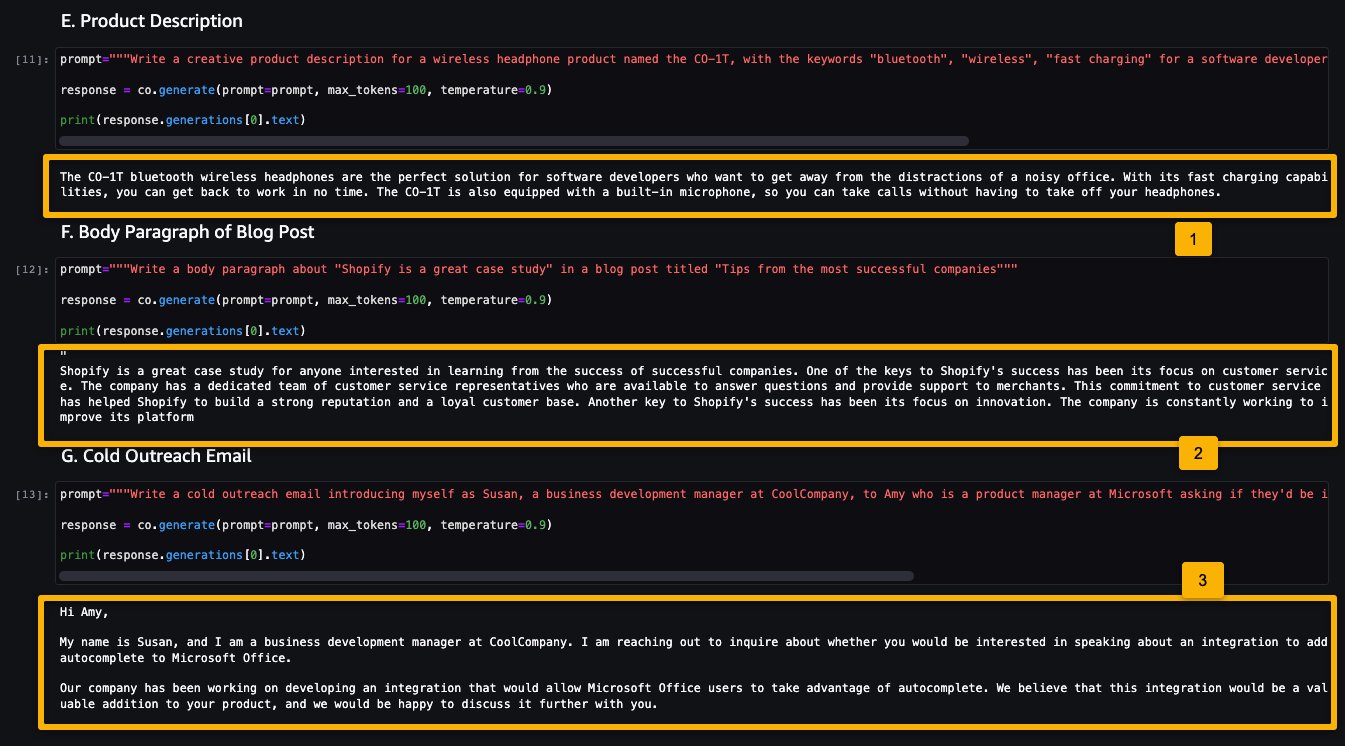
Now that you have performed real-time inference for testing, you may not need the endpoint anymore. You can delete the endpoint to avoid being charged.
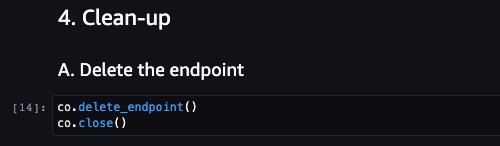
LightOn Mini-instruct
Mini-instruct, an AI model with 40 billion billion parameters created by LightOn, is a powerful multilingual AI system that has been trained using high-quality data from numerous sources. It is built to understand natural language and react to commands that are specific to your needs. It performs admirably in consumer products like voice assistants, chatbots, and smart appliances. It also has a wide range of business applications, including agent assistance and natural language production for automated customer care.
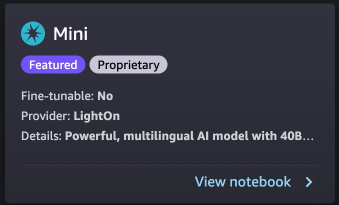
The sample notebook for LightOn Mini-instruct model provides important prerequisites that needs to be followed. For example the model is subscribed from AWS Marketplace, have appropriate IAM roles permissions, and required boto3 version etc. It walks you through how to select the model package, create endpoints for real-time inference, and then clean up.
Some of the tasks are similar to those covered in the previous notebook example, like installing Boto3 and getting the session Region.
Let’s look at creating the endpoint. First, provide the model package ARN, endpoint name, instance type to be used, and number of instances. Once created, the endpoint appears in your endpoint section of SageMaker.
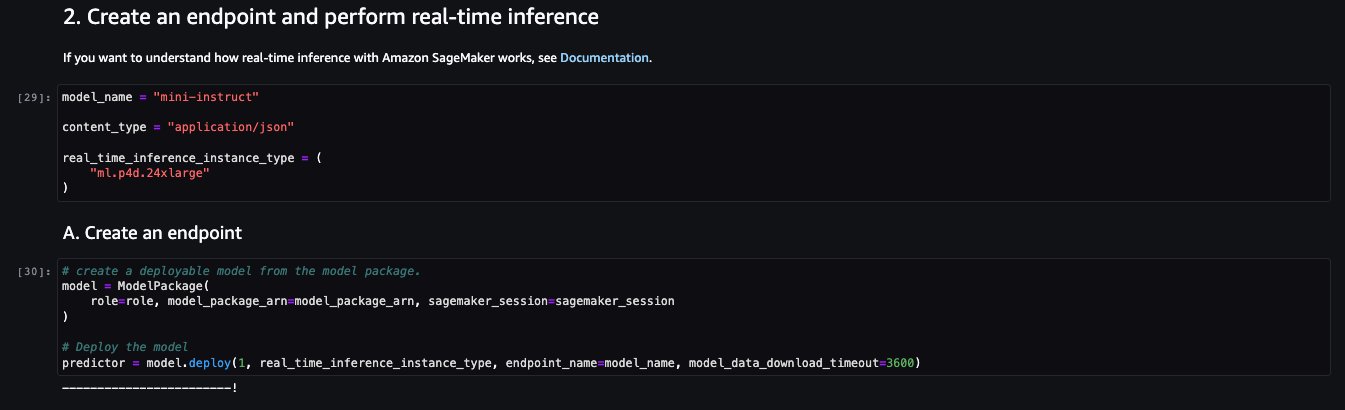
Now let’s try inferencing the model by asking it to generate a list of ideas for articles for a topic, in this case watercolor.
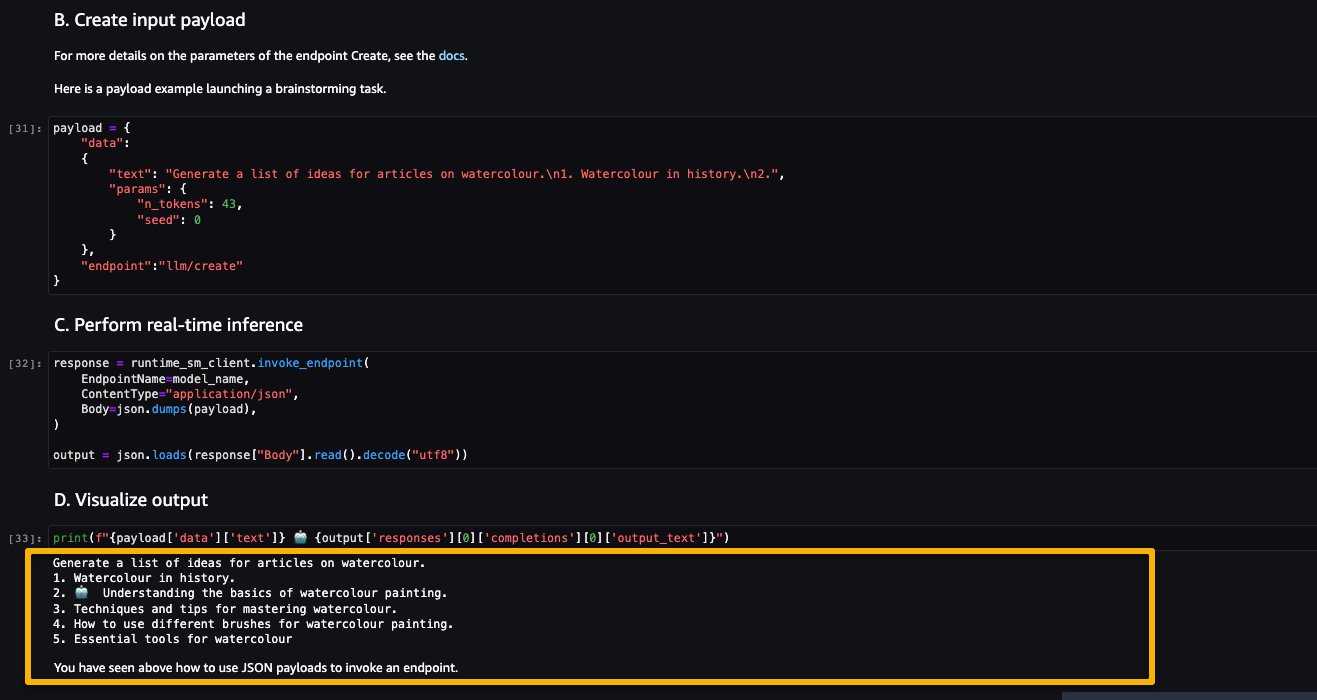
As you can see, the LightOn Mini-instruct model was able to provide generated text based on the given prompt.
Clean up
After you have tested the models and created endpoints above for the example proprietary Foundation Models, make sure you delete the SageMaker inference endpoints and delete the models to avoid incurring charges.
Conclusion
In this post, we showed you how to get started with proprietary models from model providers such as AI21, Cohere, and LightOn in SageMaker Studio. Customers can discover and use proprietary Foundation Models in SageMaker JumpStart from Studio, the SageMaker SDK, and the SageMaker Console. With this, they have access to large-scale ML models that contain billions of parameters and are pretrained on terabytes of text and image data so customers can perform a wide range of tasks such as article summarization and text, image, or video generation. Because foundation models are pretrained, they can also help lower training and infrastructure costs and enable customization for your use case.
Resources
About the authors
June Won is a product manager with SageMaker JumpStart. He focuses on making foundation models easily discoverable and usable to help customers build generative AI applications.
Mani Khanuja is an Artificial Intelligence and Machine Learning Specialist SA at Amazon Web Services (AWS). She helps customers using machine learning to solve their business challenges using the AWS. She spends most of her time diving deep and teaching customers on AI/ML projects related to computer vision, natural language processing, forecasting, ML at the edge, and more. She is passionate about ML at edge, therefore, she has created her own lab with self-driving kit and prototype manufacturing production line, where she spends lot of her free time.
Nitin Eusebius is a Sr. Enterprise Solutions Architect at AWS with experience in Software Engineering , Enterprise Architecture and AI/ML. He works with customers on helping them build well-architected applications on the AWS platform. He is passionate about solving technology challenges and helping customers with their cloud journey.
Read More