Explainability of machine learning (ML) models used in the medical domain is becoming increasingly important because models need to be explained from a number of perspectives in order to gain adoption. These perspectives range from medical, technological, legal, and the most important perspective—the patient’s. Models developed on text in the medical domain have become accurate statistically, yet clinicians are ethically required to evaluate areas of weakness related to these predictions in order to provide the best care for individual patients. Explainability of these predictions is required in order for clinicians to make the correct choices on a patient-by-patient basis.
In this post, we show how to improve model explainability in clinical settings using Amazon SageMaker Clarify.
Background
One specific application of ML algorithms in the medical domain, which uses large volumes of text, is clinical decision support systems (CDSSs) for triage. On a daily basis, patients are admitted to hospitals and admission notes are taken. After these notes are taken, the triage process is initiated, and ML models can assist clinicians with estimating clinical outcomes. This can help reduce operational overhead costs and provide optimal care for patients. Understanding why these decisions are suggested by the ML models is extremely important for decision-making related to individual patients.
The purpose of this post is to outline how you can deploy predictive models with Amazon SageMaker for the purposes of triage within hospital settings and use SageMaker Clarify to explain these predictions. The intent is to offer an accelerated path to adoption of predictive techniques within CDSSs for many healthcare organizations.
The notebook and code from this post are available on GitHub. To run it yourself, clone the GitHub repository and open the Jupyter notebook file.
Technical background
A large asset for any acute healthcare organization is its clinical notes. At the time of intake within a hospital, admission notes are taken. A number of recent studies have shown the predictability of key indicators such as diagnoses, procedures, length of stay, and in-hospital mortality. Predictions of these are now highly achievable from admission notes alone, through the use of natural language processing (NLP) algorithms [1].
Advances in NLP models, such as Bi-directional Encoder Representations from Transformers (BERT), have allowed for highly accurate predictions on a corpus of text, such as admission notes, that were previously difficult to get value from. Their prediction of the clinical indicators is highly applicable for use in a CDSS.
Yet, in order to use the new predictions effectively, how these accurate BERT models are achieving their predictions still needs to be explained. There are several techniques to explain the predictions of such models. One such technique is SHAP (SHapley Additive exPlanations), which is a model-agnostic technique for explaining the output of ML models.
What is SHAP
SHAP values are a technique for explaining the output of ML models. It provides a way to break down the prediction of an ML model and understand how much each input feature contributes to the final prediction.
SHAP values are based on game theory, specifically the concept of Shapley values, which were originally proposed to allocate the payout of a cooperative game among its players [2]. In the context of ML, each feature in the input space is considered a player in a cooperative game, and the prediction of the model is the payout. SHAP values are calculated by examining the contribution of each feature to the model prediction for each possible combination of features. The average contribution of each feature across all possible feature combinations is then calculated, and this becomes the SHAP value for that feature.
SHAP allows models to explain predictions without understanding the model’s inner workings. In addition, there are techniques to display these SHAP explanations in text, so that the medical and patient perspectives can all have intuitive visibility into how algorithms come to their predictions.
With new additions to SageMaker Clarify, and the use of pre-trained models from Hugging Face that are easily used implemented in SageMaker, model training and explainability can all be easily done in AWS.
For the purpose of an end-to-end example, we take the clinical outcome of in-hospital mortality and show how this process can be implemented easily in AWS using a pre-trained Hugging Face BERT model, and the predictions will be explained using SageMaker Clarify.
Choices of Hugging Face model
Hugging Face offers a variety of pre-trained BERT models that have been specialized for use on clinical notes. For this post, we use the bigbird-base-mimic-mortality model. This model is a fine-tuned version of Google’s BigBird model, specifically adapted for predicting mortality using MIMIC ICU admission notes. The model’s task is to determine the likelihood of a patient not surviving a particular ICU stay based on the admission notes. One of the significant advantages of using this BigBird model is its capability to process larger context lengths, which means we can input the complete admission notes without the need for truncation.
Our steps involve deploying this fine-tuned model on SageMaker. We then incorporate this model into a setup that allows for real-time explanation of its predictions. To achieve this level of explainability, we use SageMaker Clarify.
Solution overview
SageMaker Clarify provides ML developers with purpose-built tools to gain greater insights into their ML training data and models. SageMaker Clarify explains both global and local predictions and explains decisions made by computer vision (CV) and NLP models.
The following diagram shows the SageMaker architecture for hosting an endpoint that serves explainability requests. It includes interactions between an endpoint, the model container, and the SageMaker Clarify explainer.
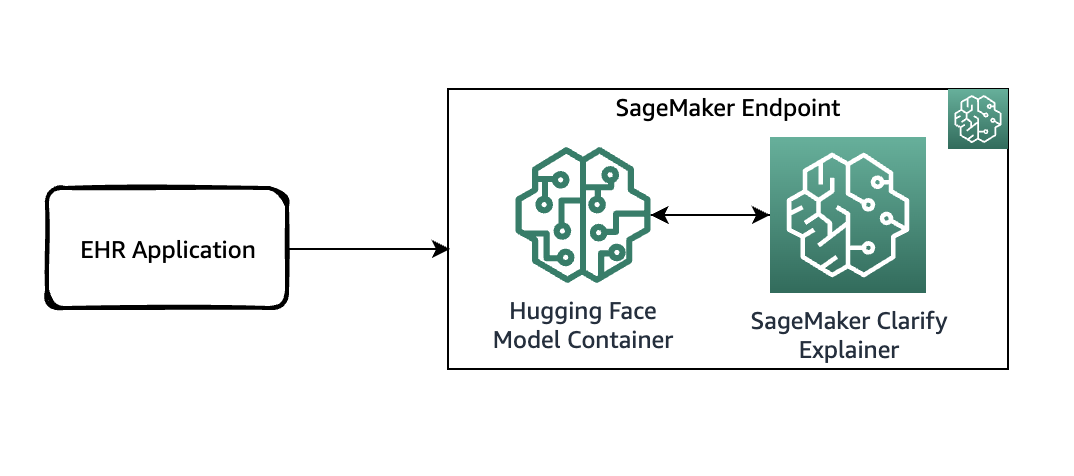
In the sample code, we use a Jupyter notebook to showcase the functionality. However, in a real-world use case, electronic health records (EHRs) or other hospital care applications would directly invoke the SageMaker endpoint to get the same response. In the Jupyter notebook, we deploy a Hugging Face model container to a SageMaker endpoint. Then we use SageMaker Clarify to explain the results that we obtain from the deployed model.
Prerequisites
You need the following prerequisites:
Access the code from the GitHub repository and upload it to your notebook instance. You can also run the notebook in an Amazon SageMaker Studio environment, which is an integrated development environment (IDE) for ML development. We recommend using a Python 3 (Data Science) kernel on SageMaker Studio or a conda_python3 kernel on a SageMaker notebook instance.
Deploy the model with SageMaker Clarify enabled
As the first step, download the model from Hugging Face and upload it to an Amazon Simple Storage Service (Amazon S3) bucket. Then create a model object using the HuggingFaceModel class. This uses a prebuilt container to simplify the process of deploying Hugging Face models to SageMaker. You also use a custom inference script to do the predictions within the container. The following code illustrates the script that is passed as an argument to the HuggingFaceModel class:
from sagemaker.huggingface import HuggingFaceModel
# create Hugging Face Model Class
huggingface_model = HuggingFaceModel(
model_data = model_path_s3,
transformers_version='4.6.1',
pytorch_version='1.7.1',
py_version='py36',
role=role,
source_dir = "./{}/code".format(model_id),
entry_point = "inference.py"
)
Then you can define the instance type that you deploy this model on:
instance_type = "ml.g4dn.xlarge"
container_def = huggingface_model.prepare_container_def(instance_type=instance_type)
container_def
We then populate ExecutionRoleArn
, ModelName
and PrimaryContainer
fields to create a Model.
model_name = "hospital-triage-model"
sagemaker_client.create_model(
ExecutionRoleArn=role,
ModelName=model_name,
PrimaryContainer=container_def,
)
print(f"Model created: {model_name}")
Next, create an endpoint configuration by calling the create_endpoint_config
API. Here, you supply the same model_name
used in the create_model
API call. The create_endpoint_config
now supports the additional parameter ClarifyExplainerConfig
to enable the SageMaker Clarify explainer. The SHAP baseline is mandatory; you can provide it either as inline baseline data (the ShapBaseline parameter) or by a S3 baseline file (the ShapBaselineUri parameter). For optional parameters, see the developer guide.
In the following code, we use a special token as the baseline:
baseline = [["<UNK>"]]
print(f"SHAP baseline: {baseline}")
The TextConfig is configured with sentence-level granularity (each sentence is a feature, and we need a few sentences per review for good visualization) and the language as English:
endpoint_config_name = "hospital-triage-model-ep-config"
csv_serializer = sagemaker.serializers.CSVSerializer()
json_deserializer = sagemaker.deserializers.JSONDeserializer()
sagemaker_client.create_endpoint_config(
EndpointConfigName=endpoint_config_name,
ProductionVariants=[
{
"VariantName": "MainVariant",
"ModelName": model_name,
"InitialInstanceCount": 1,
"InstanceType": instance_type,
}
],
ExplainerConfig={
"ClarifyExplainerConfig": {
"InferenceConfig": {"FeatureTypes": ["text"]},
"ShapConfig": {
"ShapBaselineConfig": {"ShapBaseline": csv_serializer.serialize(baseline)},
"TextConfig": {"Granularity": "sentence", "Language": "en"},
},
}
},
)
Finally, after you have the model and endpoint configuration ready, use the create_endpoint
API to create your endpoint. The endpoint_name
must be unique within a Region in your AWS account. The create_endpoint
API is synchronous in nature and returns an immediate response with the endpoint status being in the Creating state.
endpoint_name = "hospital-triage-prediction-endpoint"
sagemaker_client.create_endpoint(
EndpointName=endpoint_name,
EndpointConfigName=endpoint_config_name,
)
Explain the prediction
Now that you have deployed the endpoint with online explainability enabled, you can try some examples. You can invoke the real-time endpoint using the invoke_endpoint
method by providing the serialized payload, which in this case is some sample admission notes:
response = sagemaker_runtime_client.invoke_endpoint(
EndpointName=endpoint_name,
ContentType="text/csv",
Accept="text/csv",
Body=csv_serializer.serialize(sample_admission_note.iloc[:1, :].to_numpy())
)
result = json_deserializer.deserialize(response["Body"], content_type=response["ContentType"])
pprint.pprint(result)
In the first scenario, let’s assume that the following medical admission note was taken by a healthcare worker:
“Patient is a 25-year-old male with a chief complaint of acute chest pain. Patient reports the pain began suddenly while at work and has been constant since. Patient rates the pain as 8/10 in severity. Patient denies any radiation of pain, shortness of breath, nausea, or vomiting. Patient reports no previous history of chest pain. Vital signs are as follows: blood pressure 140/90 mmH. Heart rate 92 beats per minute. Respiratory rate 18 breaths per minute. Oxygen saturation 96% on room air. Physical examination reveals mild tenderness to palpation over the precordium and clear lung fields. EKG shows sinus tachycardia with no ST-elevations or depressions.”
The following screenshot shows the model results.
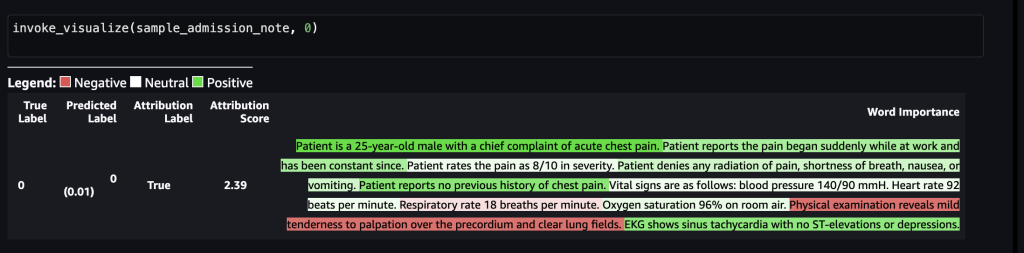
After this is forwarded to the SageMaker endpoint, the label was predicted as 0, which indicates that the risk of mortality is low. In other words, 0 implies that the admitted patient is in non-acute condition according to the model. However, we need the reasoning behind that prediction. For that, you can use the SHAP values as the response. The response includes the SHAP values corresponding to the phrases of the input note, which can be further color-coded as green or red based on how the SHAP values contribute to the prediction. In this case, we see more phrases in green, such as “Patient reports no previous history of chest pain” and “EKG shows sinus tachycardia with no ST-elevations or depressions,” as opposed to red, aligning with the mortality prediction of 0.
In the second scenario, let’s assume that the following medical admission note was taken by a healthcare worker:
“Patient is a 72-year-old female with a chief complaint of severe sepsis and septic shock. Patient reports a fever, chills, and weakness for the past 3 days, as well as decreased urine output and confusion. Patient has a history of chronic obstructive pulmonary disease (COPD) and a recent hospitalization for pneumonia. Vital signs are as follows: blood pressure 80/40 mmHg. Heart rate 130 beats per minute. Respiratory rate 30 breaths per minute. Oxygen saturation 82% on 4L of oxygen via nasal cannula. Physical examination reveals diffuse erythema and warmth over the lower extremities and positive findings for sepsis such as altered mental status, tachycardia, and tachypnea. Blood cultures were taken and antibiotic therapy was started with appropriate coverage.”
The following screenshot shows our results.
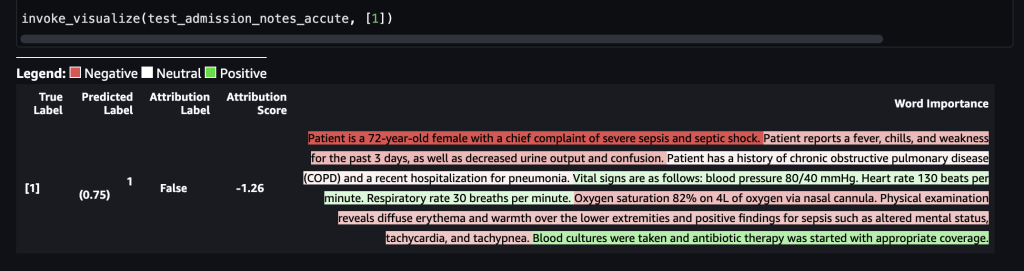
After this is forwarded to the SageMaker endpoint, the label was predicted as 1, which indicates that the risk of mortality is high. This implies that the admitted patient is in acute condition according to the model. However, we need the reasoning behind that prediction. Again, you can use the SHAP values as the response. The response includes the SHAP values corresponding to the phrases of the input note, which can be further color-coded. In this case, we see more phrases in red, such as “Patient reports a fever, chills, and weakness for the past 3 days, as well as decreased urine output and confusion” and “Patient is a 72-year-old female with a chief complaint of severe sepsis shock,” as opposed to green, aligning with the mortality prediction of 1.
The clinical care team can use these explanations to assist in their decisions on the care process for each individual patient.
Clean up
To clean up the resources that have been created as part of this solution, run the following statements:
huggingface_model.delete_model()
predictor = sagemaker.Predictor(endpoint_name="triage-prediction-endpoint")
predictor.delete_endpoint()
Conclusion
This post showed you how to use SageMaker Clarify to explain decisions in a healthcare use case based on the medical notes captured during various stages of triage process. This solution can be integrated into existing decision support systems to provide another data point to clinicians as they evaluate patients for admission into the ICU. To learn more about using AWS services in the healthcare industry, check out the following blog posts:
References
[1]
https://aclanthology.org/2021.eacl-main.75/
[2]
https://arxiv.org/pdf/1705.07874.pdf
About the authors
Shamika Ariyawansa, serving as a Senior AI/ML Solutions Architect in the Global Healthcare and Life Sciences division at Amazon Web Services (AWS), has a keen focus on Generative AI. He assists customers in integrating Generative AI into their projects, emphasizing the importance of explainability within their AI-driven initiatives. Beyond his professional commitments, Shamika passionately pursues skiing and off-roading adventures.”
Ted Spencer is an experienced Solutions Architect with extensive acute healthcare experience. He is passionate about applying machine learning to solve new use cases, and rounds out solutions with both the end consumer and their business/clinical context in mind. He lives in Toronto Ontario, Canada, and enjoys traveling with his family and training for triathlons as time permits.
Ram Pathangi is a Solutions Architect at AWS supporting healthcare and life sciences customers in the San Francisco Bay Area. He has helped customers in finance, healthcare, life sciences, and hi-tech verticals run their business successfully on the AWS Cloud. He specializes in Databases, Analytics, and Machine Learning.
Read More