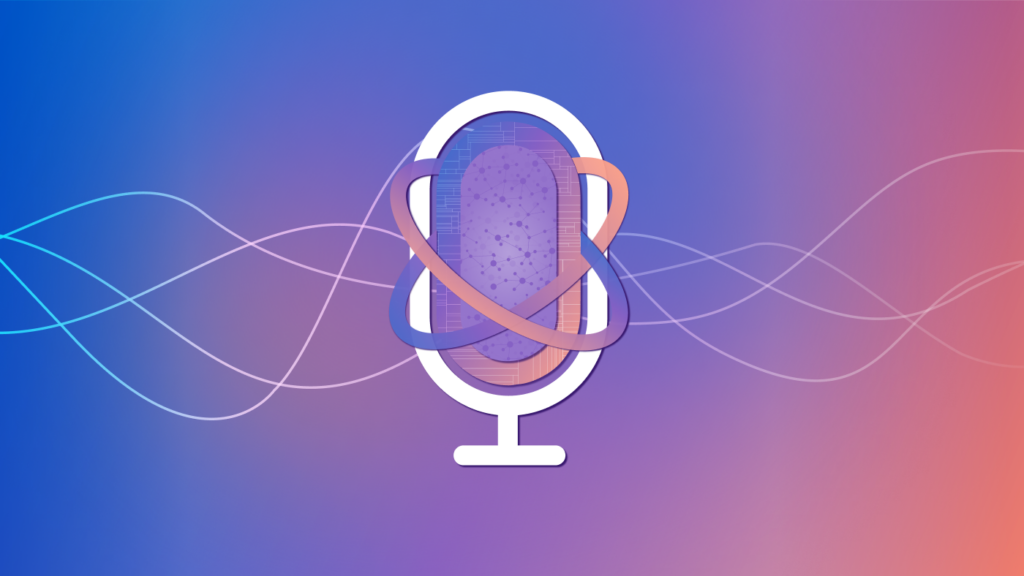
Episode 148 | September 13, 2023
Members of the research community at Microsoft work continuously to advance their respective fields. Abstracts brings its audience to the cutting edge with them through short, compelling conversations about new and noteworthy achievements.
In the inaugural episode of the series, Dr. Ava Amini and Dr. Kevin K. Yang, both Senior Researchers with Microsoft Health Futures, join host Dr. Gretchen Huizinga to discuss “Protein generation with evolutionary diffusion: Sequence is all you need.” The paper introduces EvoDiff, a suite of models that leverages evolutionary-scale protein data to help design novel proteins more efficiently. Improved protein engineering has the potential to help create new vaccines to prevent disease and new ways to recycle plastics.
Subscribe to the Microsoft Research Podcast:
Transcript
[MUSIC PLAYS]GRETCHEN HUIZINGA: Welcome to Abstracts, a Microsoft Research Podcast that puts the spotlight on world-class research in brief. I’m Dr. Gretchen Huizinga. In this series, members of the research community at Microsoft give us a quick snapshot—or a podcast abstract!—of their new and noteworthy papers.
[MUSIC FADES]Today, I’m talking to Dr. Ava Amini and Dr. Kevin Yang, both senior researchers at Microsoft Health Futures. Ava and Kevin are co-authors of a paper titled “Protein generation with evolutionary diffusion: Sequence is all you need,” and a preprint of the paper is available now on bioRxiv. Ava and Kevin, thanks for joining us on Abstracts!
KEVIN YANG: Thanks for having us.
AVA AMINI: Thank you so much.
HUIZINGA: So, Kevin, in just a couple sentences, tell us what problem this research addresses and why people should care.
YANG: Yeah, so proteins are this really big, important family of biomolecules, and they’re responsible for a lot of cellular processes. For example, hemoglobin carries oxygen in your blood, and insulin regulates your blood sugar levels. And people are interested in generating new proteins to do things that people care about—not necessarily in our bodies, but we’re interested in proteins as industrial enzymes so for catalysis and to make new chemicals or for therapeutics to make new drugs. And as a step towards this goal, we train a suite of models that we call EvoDiff that learns to generate realistic but novel proteins. So proteins do a lot of useful things in nature, but we can really expand their repertoire to do things that people care about but that nature may not really care about. One really good historical example of this is that most of our modern laundry detergents contain enzymes that break down things that stain your clothes. And these enzymes were based on natural proteins, but natural proteins don’t work under high heat. They don’t work in detergent. So somebody engineered those to work in the conditions of our washing machine. And they work really well nowadays. Looking forward, we look at some of the challenges facing our world, such as sustainability. So some really big things people are working on now are things like enzymes that can break down plastic and help us recycle plastic or enzymes that can perform photosynthesis more efficiently. And then on the other side, there’s therapeutics, and an obvious example there is vaccine design. So designing vaccines quickly and safely for new diseases as they emerge.
HUIZINGA: Ava, how does your approach build on or differ from what’s been done previously in this field?
AMINI: Yeah, so we call our approach EvoDiff, and EvoDiff has two components. The first, Evo, refers to evolutionary, and the second, Diff, refers to this notion of diffusion. And the two things that make our approach cool and powerful is the fact that we are leveraging data about proteins that is at an evolutionary scale in terms of the size and the diversity of the datasets about natural proteins that we use. And specifically, we use that data to build a type of AI model that is called a diffusion model. Now, for a little backstory on this, a few years ago, we in the AI community learned that we can do really well in generating brand-new images by taking natural images, adding small amounts of noise to them, corrupting them, and then training an AI model called a diffusion model to remove that noise. And so what we’ve done in this paper is that we have constructed and trained these diffusion models to do the same kind of process on protein data at evolutionary scale.
HUIZINGA: Kevin, back to you, let’s go a little deeper on methodology. How did you do this?
YANG: Yeah, so we really wanted to do this in a protein sequence space. So in protein biology, you have sequences of amino acids. So that’s a series of amino acid monomers that form a chain, and then that chain folds oftentimes into a 3D structure. And function is usually mediated by that 3D structure. Unfortunately, it’s difficult and can be slow and expensive to obtain experimental structures for all these proteins. And so previous diffusion models of proteins have really focused on generating a three-dimensional structure. And then you can use some other method to find a sequence that will fold to that structure. But what we really wanted to do was generate proteins directly as sequences because it’s much easier to get sequences than it is to get structure. So there’s many, many more sequences out there than there are structures. And we know that deep learning methods scale really well as you increase the size and quality of the datasets they’re trained on. And so we … and by we, it’s me and Ava but also Nitya Thakkar, who was an undergraduate intern last summer with me and Ava, and then Sarah Alamdari, our data scientist, who also did a lot of the hands-on programming for this. And then we also got a lot of help from Rianne van den Berg, who is at AI4Science, and then Alex Lu and Nicolò Fusi, also here in New England. So we went and got these large, diverse, evolutionary datasets of protein sequences, and then we used a deep learning framework called PyTorch to train these diffusion models. And then we do a lot of computational experiments to see whether they do the things we want them to do, which Ava, I think, will talk about next.
HUIZINGA: Right. Right. So, Ava, yes, what were your major findings?
AMINI: Yeah, the first question we really asked was, can our method, EvoDiff, generate proteins that are new, that are realistic, and that are diverse, meaning they’re not similar to proteins that exist in nature but still are realistic? And so what we found was that indeed, we can do this, and we can do this really well. In fact, the generated proteins from our method show a better coverage of the whole landscape of structural features, functional features, and features in sequence space that exist amongst proteins in nature. And so that was our first really exciting result, that we could generate proteins that were really of high quality using our method. The second thing we asked was, OK, now if we give some context to the model, a little bit of information, can we guide the generation to fulfill particular properties that we want to see in that protein? And so specifically here, we experimented with two types of experiments where first, we can give a part of the protein to the model, let’s say, a part of the protein that binds to another protein. And we hold that part constant and ask the model to generate the sequence around that. And we see that we can do really well on this task, as well. And why that’s important is because it means we can now design new proteins that meet some criteria that we, the users, want the protein to have. For example, the ability to bind to something else. And finally, the last really exciting result was … one point that we’ve talked about is why we want to do this generation in sequence space rather than structure—because structure is difficult, it’s expensive, and there are particular types of proteins that don’t actually end up folding into a final 3D structure. They’re what we call disordered. And these types of disordered proteins have really, really important roles in biology and in disease. And so what we show is that because we do our generation and design in protein sequence space, we can actually generate these types of disordered proteins that are completely inaccessible to methods that rely on using information about the protein’s 3D shape.
HUIZINGA: So, Kevin, building on Ava’s description there of the structure and sequence space, how is your work significant in terms of real-world impact?
YANG: Right, so there’s a lot of interest in designing or generating new proteins that do useful things as therapeutics or as industrial catalysts and for a lot of other things, as well. And what our work really does is it gives us a method that can reliably generate high-quality proteins directly in sequence space. And this is good because now we can leverage evolutionary-scale data to do this on any downstream protein engineering problem without relying on a structure-based design or structure-based data. And we’re hoping that this opens up a lot of possibilities for protein engineering, protein design, and we’re really excited about some new experimental work that we—and we hope others—will use to build on this method.
HUIZINGA: Are you guys the first to move into the evolutionary scale in this? Is that a differentiator for your work?
YANG: So there have been a few other preprints or papers that talk about applying diffusion to protein sequences. The difference here is that, yes, like I said, we’re the first ones to do this at evolutionary scale. So people will also train these models on small sets of related protein sequences. For example, you might go look for an enzyme family and find all the sequences in nature of that family and train a model to generate new examples of that enzyme. But what we’re doing is we’re looking at data that’s from all different species and all different functional classes of proteins and giving us a model that is hopefully universal or as close to universal as we can get for protein sequence space.
HUIZINGA: Wow. Ava, if there was one thing you want listeners to take away from this work, what would it be?
AMINI: If there’s one thing to take away, I think it would be this idea that we can and should do protein generation over sequence because of the generality we’re able to achieve, the scale that we’re able to achieve, and the modularity and that our diffusion framework gives us the ability to do that and also to control how we design these proteins to meet specific functional goals.
HUIZINGA: So, Kevin, to kind of wrap it up, I wonder if you could address what unanswered questions still remain, or unsolved problems in this area, and what’s next on your research agenda.
YANG: So there’s kind of two directions we want to see here. One is, we want to test better ideas for conditioner models. And what I mean there is we want to feed in text or a desired chemical reaction or some other function directly and have it generate those things that will then go work in the lab. And that’s a really big step up from just generating sequences that work and are novel. And two is, in biology and in protein engineering, models are really good, but what really matters is, do things work in the lab? So we are actually looking to do some of our own experiments to see if the proteins we generate from EvoDiff work as desired in the lab.
[MUSIC PLAYS]HUIZINGA: Ava Amini and Kevin Yang, thanks so much for joining us today, and to our listeners, thanks for tuning in. If you’re interested in learning more about the paper, you can find a link at aka.ms/abstracts or you can find a preprint of the paper on bioRxiv. See you next time on Abstracts!
The post Abstracts: September 13, 2023 appeared first on Microsoft Research.