As customers accelerate their migrations to the cloud and transform their business, some find themselves in situations where they have to manage IT operations in a multicloud environment. For example, you might have acquired a company that was already running on a different cloud provider, or you may have a workload that generates value from unique capabilities provided by AWS. Another example is independent software vendors (ISVs) that make their products and services available in different cloud platforms to benefit their end customers. Or an organization may be operating in a Region where a primary cloud provider is not available, and in order to meet the data sovereignty or data residency requirements, they can use a secondary cloud provider.
In these scenarios, as you start to embrace generative AI, large language models (LLMs) and machine learning (ML) technologies as a core part of your business, you may be looking for options to take advantage of AWS AI and ML capabilities outside of AWS in a multicloud environment. For example, you may want to make use of Amazon SageMaker to build and train ML model, or use Amazon SageMaker Jumpstart to deploy pre-built foundation or third party ML models, which you can deploy at the click of a few buttons. Or you may want to take advantage of Amazon Bedrock to build and scale generative AI applications, or you can leverage AWS’ pre-trained AI services, which don’t require you to learn machine learning skills. AWS provides support for scenarios where organizations want to bring their own model to Amazon SageMaker or into Amazon SageMaker Canvas for predictions.
In this post, we demonstrate one of the many options that you have to take advantage of AWS’s broadest and deepest set of AI/ML capabilities in a multicloud environment. We show how you can build and train an ML model in AWS and deploy the model in another platform. We train the model using Amazon SageMaker, store the model artifacts in Amazon Simple Storage Service (Amazon S3), and deploy and run the model in Azure. This approach is beneficial if you use AWS services for ML for its most comprehensive set of features, yet you need to run your model in another cloud provider in one of the situations we’ve discussed.
Key concepts
Amazon SageMaker Studio is a web-based, integrated development environment (IDE) for machine learning. SageMaker Studio allows data scientists, ML engineers, and data engineers to prepare data, build, train, and deploy ML models on one web interface. With SageMaker Studio, you can access purpose-built tools for every stage of the ML development lifecycle, from data preparation to building, training, and deploying your ML models, improving data science team productivity by up to ten times. SageMaker Studio notebooks are quick start, collaborative notebooks that integrate with purpose-built ML tools in SageMaker and other AWS services.
SageMaker is a comprehensive ML service enabling business analysts, data scientists, and MLOps engineers to build, train, and deploy ML models for any use case, regardless of ML expertise.
AWS provides Deep Learning Containers (DLCs) for popular ML frameworks such as PyTorch, TensorFlow, and Apache MXNet, which you can use with SageMaker for training and inference. DLCs are available as Docker images in Amazon Elastic Container Registry (Amazon ECR). The Docker images are preinstalled and tested with the latest versions of popular deep learning frameworks as well as other dependencies needed for training and inference. For a complete list of the pre-built Docker images managed by SageMaker, see Docker Registry Paths and Example Code. Amazon ECR supports security scanning, and is integrated with Amazon Inspector vulnerability management service to meet your organization’s image compliance security requirements, and to automate vulnerability assessment scanning. Organizations can also use AWS Trainium and AWS Inferentia for better price-performance for running ML training jobs or inference.
Solution overview
In this section, we describe how to build and train a model using SageMaker and deploy the model to Azure Functions. We use a SageMaker Studio notebook to build, train, and deploy the model. We train the model in SageMaker using a pre-built Docker image for PyTorch. Although we’re deploying the trained model to Azure in this case, you could use the same approach to deploy the model on other platforms such as on premises or other cloud platforms.
When we create a training job, SageMaker launches the ML compute instances and uses our training code and the training dataset to train the model. It saves the resulting model artifacts and other output in an S3 bucket that we specify as input to the training job. When model training is complete, we use the Open Neural Network Exchange (ONNX) runtime library to export the PyTorch model as an ONNX model.
Finally, we deploy the ONNX model along with a custom inference code written in Python to Azure Functions using the Azure CLI. ONNX supports most of the commonly used ML frameworks and tools. One thing to note is that converting an ML model to ONNX is useful if you want to want to use a different target deployment framework, such as PyTorch to TensorFlow. If you’re using the same framework on both the source and target, you don’t need to convert the model to ONNX format.
The following diagram illustrates the architecture for this approach.
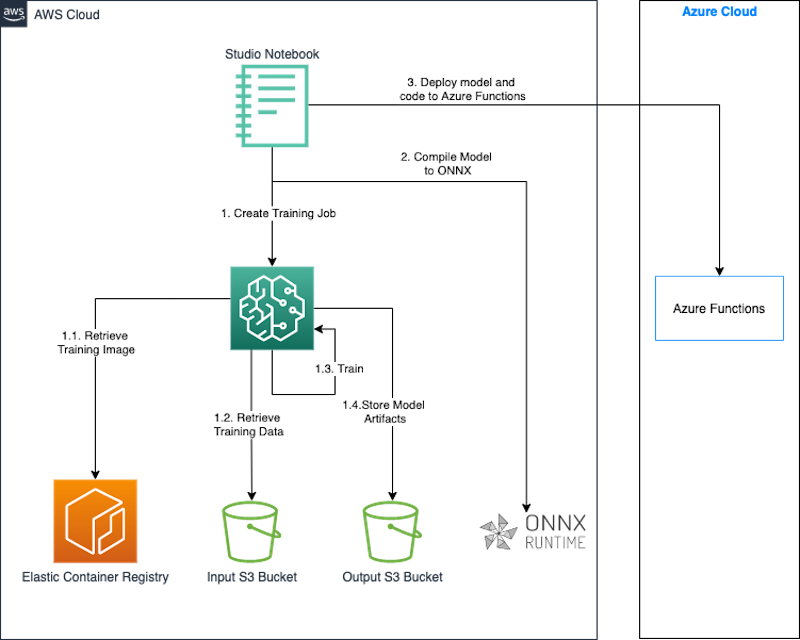
We use a SageMaker Studio notebook along with the SageMaker Python SDK to build and train our model. The SageMaker Python SDK is an open-source library for training and deploying ML models on SageMaker. For more details, refer to Create or Open an Amazon SageMaker Studio Notebook.
The code snippets in the following sections have been tested in the SageMaker Studio notebook environment using the Data Science 3.0 image and Python 3.0 kernel.
In this solution, we demonstrate the following steps:
- Train a PyTorch model.
- Export the PyTorch model as an ONNX model.
- Package the model and inference code.
- Deploy the model to Azure Functions.
Prerequisites
You should have the following prerequisites:
- An AWS account.
- A SageMaker domain and SageMaker Studio user. For instructions to create these, refer to Onboard to Amazon SageMaker Domain Using Quick setup.
- The Azure CLI.
- Access to Azure and credentials for a service principal that has permissions to create and manage Azure Functions.
Train a model with PyTorch
In this section, we detail the steps to train a PyTorch model.
Install dependencies
Install the libraries to carry out the steps required for model training and model deployment:
pip install torchvision onnx onnxruntime
Complete initial setup
We begin by importing the AWS SDK for Python (Boto3) and the SageMaker Python SDK. As part of the setup, we define the following:
- A session object that provides convenience methods within the context of SageMaker and our own account.
- A SageMaker role ARN used to delegate permissions to the training and hosting service. We need this so that these services can access the S3 buckets where our data and model are stored. For instructions on creating a role that meets your business needs, refer to SageMaker Roles. For this post, we use the same execution role as our Studio notebook instance. We get this role by calling
sagemaker.get_execution_role()
.
- The default Region where our training job will run.
- The default bucket and the prefix we use to store the model output.
See the following code:
import sagemaker
import boto3
import os
execution_role = sagemaker.get_execution_role()
region = boto3.Session().region_name
session = sagemaker.Session()
bucket = session.default_bucket()
prefix = "sagemaker/mnist-pytorch"
Create the training dataset
We use the dataset available in the public bucket sagemaker-example-files-prod-{region}
. The dataset contains the following files:
- train-images-idx3-ubyte.gz – Contains training set images
- train-labels-idx1-ubyte.gz – Contains training set labels
- t10k-images-idx3-ubyte.gz – Contains test set images
- t10k-labels-idx1-ubyte.gz – Contains test set labels
We use thetorchvision.datasets
module to download the data from the public bucket locally before uploading it to our training data bucket. We pass this bucket location as an input to the SageMaker training job. Our training script uses this location to download and prepare the training data, and then train the model. See the following code:
MNIST.mirrors = [
f"https://sagemaker-example-files-prod-{region}.s3.amazonaws.com/datasets/image/MNIST/"
]
MNIST(
"data",
download=True,
transform=transforms.Compose(
[transforms.ToTensor(), transforms.Normalize((0.1307,), (0.3081,))]
),
)
Create the training script
With SageMaker, you can bring your own model using script mode. With script mode, you can use the pre-built SageMaker containers and provide your own training script, which has the model definition, along with any custom libraries and dependencies. The SageMaker Python SDK passes our script as an entry_point
to the container, which loads and runs the train function from the provided script to train our model.
When the training is complete, SageMaker saves the model output in the S3 bucket that we provided as a parameter to the training job.
Our training code is adapted from the following PyTorch example script. The following excerpt from the code shows the model definition and the train function:
# define network
class Net(nn.Module):
def __init__(self):
super(Net, self).__init__()
self.conv1 = nn.Conv2d(1, 32, 3, 1)
self.conv2 = nn.Conv2d(32, 64, 3, 1)
self.dropout1 = nn.Dropout(0.25)
self.dropout2 = nn.Dropout(0.5)
self.fc1 = nn.Linear(9216, 128)
self.fc2 = nn.Linear(128, 10)
def forward(self, x):
x = self.conv1(x)
x = F.relu(x)
x = self.conv2(x)
x = F.relu(x)
x = F.max_pool2d(x, 2)
x = self.dropout1(x)
x = torch.flatten(x, 1)
x = self.fc1(x)
x = F.relu(x)
x = self.dropout2(x)
x = self.fc2(x)
output = F.log_softmax(x, dim=1)
return output
# train
def train(args, model, device, train_loader, optimizer, epoch):
model.train()
for batch_idx, (data, target) in enumerate(train_loader):
data, target = data.to(device), target.to(device)
optimizer.zero_grad()
output = model(data)
loss = F.nll_loss(output, target)
loss.backward()
optimizer.step()
if batch_idx % args.log_interval == 0:
print('Train Epoch: {} [{}/{} ({:.0f}%)]tLoss: {:.6f}'.format(
epoch, batch_idx * len(data), len(train_loader.dataset),
100. * batch_idx / len(train_loader), loss.item()))
if args.dry_run:
break
Train the model
Now that we have set up our environment and created our input dataset and custom training script, we can start the model training using SageMaker. We use the PyTorch estimator in the SageMaker Python SDK to start a training job on SageMaker. We pass in the required parameters to the estimator and call the fit method. When we call fit on the PyTorch estimator, SageMaker starts a training job using our script as training code:
from sagemaker.pytorch import PyTorch
output_location = f"s3://{bucket}/{prefix}/output"
print(f"training artifacts will be uploaded to: {output_location}")
hyperparameters={
"batch-size": 100,
"epochs": 1,
"lr": 0.1,
"gamma": 0.9,
"log-interval": 100
}
instance_type = "ml.c4.xlarge"
estimator = PyTorch(
entry_point="train.py",
source_dir="code", # directory of your training script
role=execution_role,
framework_version="1.13",
py_version="py39",
instance_type=instance_type,
instance_count=1,
volume_size=250,
output_path=output_location,
hyperparameters=hyperparameters
)
estimator.fit(inputs = {
'training': f"{inputs}",
'testing': f"{inputs}"
})
Export the trained model as a ONNX model
After the training is complete and our model is saved to the predefined location in Amazon S3, we export the model to an ONNX model using the ONNX runtime.
We include the code to export our model to ONNX in our training script to run after the training is complete.
PyTorch exports the model to ONNX by running the model using our input and recording a trace of operators used to compute the output. We use a random input of the right type with the PyTorch torch.onnx.export
function to export the model to ONNX. We also specify the first dimension in our input as dynamic so that our model accepts a variable batch_size
of inputs during inference.
def export_to_onnx(model, model_dir, device):
logger.info("Exporting the model to onnx.")
dummy_input = torch.randn(1, 1, 28, 28).to(device)
input_names = [ "input_0" ]
output_names = [ "output_0" ]
path = os.path.join(model_dir, 'mnist-pytorch.onnx')
torch.onnx.export(model, dummy_input, path, verbose=True, input_names=input_names, output_names=output_names,
dynamic_axes={'input_0' : {0 : 'batch_size'}, # variable length axes
'output_0' : {0 : 'batch_size'}})
ONNX is an open standard format for deep learning models that enables interoperability between deep learning frameworks such as PyTorch, Microsoft Cognitive Toolkit (CNTK), and more. This means you can use any of these frameworks to train the model and subsequently export the pre-trained models in ONNX format. By exporting the model to ONNX, you get the benefit of a broader selection of deployment devices and platforms.
Download and extract the model artifacts
The ONNX model that our training script has saved has been copied by SageMaker to Amazon S3 in the output location that we specified when we started the training job. The model artifacts are stored as a compressed archive file called model.tar.gz
. We download this archive file to a local directory in our Studio notebook instance and extract the model artifacts, namely the ONNX model.
import tarfile
local_model_file = 'model.tar.gz'
model_bucket,model_key = estimator.model_data.split('/',2)[-1].split('/',1)
s3 = boto3.client("s3")
s3.download_file(model_bucket,model_key,local_model_file)
model_tar = tarfile.open(local_model_file)
model_file_name = model_tar.next().name
model_tar.extractall('.')
model_tar.close()
Validate the ONNX model
The ONNX model is exported to a file named mnist-pytorch.onnx
by our training script. After we have downloaded and extracted this file, we can optionally validate the ONNX model using the onnx.checker
module. The check_model
function in this module checks the consistency of a model. An exception is raised if the test fails.
import onnx
onnx_model = onnx.load("mnist-pytorch.onnx")
onnx.checker.check_model(onnx_model)
Package the model and inference code
For this post, we use .zip deployment for Azure Functions. In this method, we package our model, accompanying code, and Azure Functions settings in a .zip file and publish it to Azure Functions. The following code shows the directory structure of our deployment package:
mnist-onnx
├── function_app.py
├── model
│ └── mnist-pytorch.onnx
└── requirements.txt
List dependencies
We list the dependencies for our inference code in the requirements.txt
file at the root of our package. This file is used to build the Azure Functions environment when we publish the package.
azure-functions
numpy
onnxruntime
Write inference code
We use Python to write the following inference code, using the ONNX Runtime library to load our model and run inference. This instructs the Azure Functions app to make the endpoint available at the /classify
relative path.
import logging
import azure.functions as func
import numpy as np
import os
import onnxruntime as ort
import json
app = func.FunctionApp()
def preprocess(input_data_json):
# convert the JSON data into the tensor input
return np.array(input_data_json['data']).astype('float32')
def run_model(model_path, req_body):
session = ort.InferenceSession(model_path)
input_data = preprocess(req_body)
logging.info(f"Input Data shape is {input_data.shape}.")
input_name = session.get_inputs()[0].name # get the id of the first input of the model
try:
result = session.run([], {input_name: input_data})
except (RuntimeError) as e:
print("Shape={0} and error={1}".format(input_data.shape, e))
return result[0]
def get_model_path():
d=os.path.dirname(os.path.abspath(__file__))
return os.path.join(d , './model/mnist-pytorch.onnx')
@app.function_name(name="mnist_classify")
@app.route(route="classify", auth_level=func.AuthLevel.ANONYMOUS)
def main(req: func.HttpRequest) -> func.HttpResponse:
logging.info('Python HTTP trigger function processed a request.')
# Get the img value from the post.
try:
req_body = req.get_json()
except ValueError:
pass
if req_body:
# run model
result = run_model(get_model_path(), req_body)
# map output to integer and return result string.
digits = np.argmax(result, axis=1)
logging.info(type(digits))
return func.HttpResponse(json.dumps({"digits": np.array(digits).tolist()}))
else:
return func.HttpResponse(
"This HTTP triggered function successfully.",
status_code=200
)
Deploy the model to Azure Functions
Now that we have the code packaged into the required .zip format, we’re ready to publish it to Azure Functions. We do that using the Azure CLI, a command line utility to create and manage Azure resources. Install the Azure CLI with the following code:
!pip install -q azure-cli
Then complete the following steps:
- Log in to Azure:
!az login
- Set up the resource creation parameters:
import random
random_suffix = str(random.randint(10000,99999))
resource_group_name = f"multicloud-{random_suffix}-rg"
storage_account_name = f"multicloud{random_suffix}"
location = "ukwest"
sku_storage = "Standard_LRS"
functions_version = "4"
python_version = "3.9"
function_app = f"multicloud-mnist-{random_suffix}"
- Use the following commands to create the Azure Functions app along with the prerequisite resources:
!az group create --name {resource_group_name} --location {location}
!az storage account create --name {storage_account_name} --resource-group {resource_group_name} --location {location} --sku {sku_storage}
!az functionapp create --name {function_app} --resource-group {resource_group_name} --storage-account {storage_account_name} --consumption-plan-location "{location}" --os-type Linux --runtime python --runtime-version {python_version} --functions-version {functions_version}
- Set up the Azure Functions so that when we deploy the Functions package, the
requirements.txt
file is used to build our application dependencies:
!az functionapp config appsettings set --name {function_app} --resource-group {resource_group_name} --settings @./functionapp/settings.json
- Configure the Functions app to run the Python v2 model and perform a build on the code it receives after .zip deployment:
{
"AzureWebJobsFeatureFlags": "EnableWorkerIndexing",
"SCM_DO_BUILD_DURING_DEPLOYMENT": true
}
- After we have the resource group, storage container, and Functions app with the right configuration, publish the code to the Functions app:
!az functionapp deployment source config-zip -g {resource_group_name} -n {function_app} --src {function_archive} --build-remote true
Test the model
We have deployed the ML model to Azure Functions as an HTTP trigger, which means we can use the Functions app URL to send an HTTP request to the function to invoke the function and run the model.
To prepare the input, download the test images files from the SageMaker example files bucket and prepare a set of samples to the format required by the model:
from torch.utils.data import DataLoader
from torchvision import datasets, transforms
import matplotlib.pyplot as plt
transform=transforms.Compose(
[transforms.ToTensor(), transforms.Normalize((0.1307,), (0.3081,))]
)
test_dataset = datasets.MNIST(root='../data', download=True, train=False, transform=transform)
test_loader = DataLoader(test_dataset, batch_size=16, shuffle=True)
test_features, test_labels = next(iter(test_loader))
Use the requests library to send a post request to the inference endpoint with the sample inputs. The inference endpoint takes the format as shown in the following code:
import requests, json
def to_numpy(tensor):
return tensor.detach().cpu().numpy() if tensor.requires_grad else tensor.cpu().numpy()
url = f"https://{function_app}.azurewebsites.net/api/classify"
response = requests.post(url,
json.dumps({"data":to_numpy(test_features).tolist()})
)
predictions = json.loads(response.text)['digits']
Clean up
When you’re done testing the model, delete the resource group along with the contained resources, including the storage container and Functions app:
!az group delete --name {resource_group_name} --yes
Additionally, it is recommended to shut down idle resources within SageMaker Studio to reduce costs. For more information, refer to Save costs by automatically shutting down idle resources within Amazon SageMaker Studio.
Conclusion
In this post, we showed how you can build and train an ML model with SageMaker and deploy it to another cloud provider. In the solution, we used a SageMaker Studio notebook, but for production workloads, we recommended using MLOps to create repeatable training workflows to accelerate model development and deployment.
This post didn’t show all the possible ways to deploy and run a model in a multicloud environment. For example, you can also package your model into a container image along with inference code and dependency libraries to run the model as a containerized application in any platform. For more information about this approach, refer to Deploy container applications in a multicloud environment using Amazon CodeCatalyst. The intent of the post is to show how organizations can use AWS AI/ML capabilities in a multicloud environment.
About the authors
Raja Vaidyanathan is a Solutions Architect at AWS supporting global financial services customers. Raja works with customers to architect solutions to complex problems with long-term positive impact on their business. He’s a strong engineering professional skilled in IT strategy, enterprise data management, and application architecture, with particular interests in analytics and machine learning.
Amandeep Bajwa is a Senior Solutions Architect at AWS supporting financial services enterprises. He helps organizations achieve their business outcomes by identifying the appropriate cloud transformation strategy based on industry trends and organizational priorities. Some of the areas Amandeep consults on are cloud migration, cloud strategy (including hybrid and multicloud), digital transformation, data and analytics, and technology in general.
Prema Iyer is Senior Technical Account Manager for AWS Enterprise Support. She works with external customers on a variety of projects, helping them improve the value of their solutions when using AWS.
Read More