At Amazon Web Services (AWS), not only are we passionate about providing customers with a variety of comprehensive technical solutions, but we’re also keen on deeply understanding our customers’ business processes. We adopt a third-party perspective and objective judgment to help customers sort out their value propositions, collect pain points, propose appropriate solutions, and create the most cost-effective and usable prototypes to help them systematically achieve their business goals.
This method is called working backwards at AWS. It means putting aside technology and solutions, starting from the expected results of customers, confirming their value, and then deducing what needs to be done in reverse order before finally implementing a solution. During the implementation phase, we also follow the concept of minimum viable product and strive to quickly form a prototype that can generate value within a few weeks, and then iterate on it.
Today, let’s review a case study where AWS and New Hope Dairy collaborated to build a smart farm on the cloud. From this blog post, you can have a deep understanding about what AWS can provide for building a smart farm and how to build smart farm applications on the cloud with AWS experts.
Project background
Milk is a nutritious beverage. In consideration of national health, China has been actively promoting the development of the dairy industry. According to data from Euromonitor International, the sale of dairy products in China reached 638.5 billion RMB in 2020 and is expected to reach 810 billion RMB in 2025. In addition, the compound annual growth rate in the past 14 years has also reached 10 percent, showing rapid development.
On the other hand, as of 2022, most of the revenue in the Chinese dairy industry still comes from liquid milk. Sixty percent of the raw milk is used for liquid milk and yogurt, and another 20 percent is milk powder—a derivative of liquid milk. Only a very small amount is used for highly processed products such as cheese and cream.
Liquid milk is a lightly processed product and its output, quality, and cost are closely linked to raw milk. This means that if the dairy industry wants to free capacity to focus on producing highly processed products, create new products, and conduct more innovative biotechnology research, it must first improve and stabilize the production and quality of raw milk.
As a dairy industry leader, New Hope Dairy has been thinking about how to improve the efficiency of its ranch operations and increase the production and quality of raw milk. New Hope Dairy hopes to use the third-party perspective and technological expertise of AWS to facilitate innovation in the dairy industry. With support and promotion from Liutong Hu, VP and CIO of New Hope Dairy, the AWS customer team began to organize operations and potential innovation points for the dairy farms.
Dairy farm challenges
AWS is an expert in the field of cloud technology, but to implement innovation in the dairy industry, professional advice from dairy subject matter experts is necessary. Therefore, we conducted several in-depth interviews with Liangrong Song, the Deputy Director of Production Technology Center of New Hope Dairy, the ranch management team, and nutritionists to understand some of the issues and challenges facing the farm.
First is taking inventory of reserve cows
The dairy cows on the ranch are divided into two types: dairy cows and reserve cows. Dairy cows are mature and continuously produce milk, while reserve cows are cows that have not yet reached the age to produce milk. Large and medium-sized farms usually provide reserve cows with a larger open activity area to create a more comfortable growing environment.
However, both dairy cows and reserve cows are assets of the farm and need to be inventoried monthly. Dairy cows are milked every day, and because they are relatively still during milking, inventory tracking is easy. However, reserve cows are in an open space and roam freely, which makes it inconvenient to inventory them. Each time inventory is taken, several workers count the reserve cows repeatedly from different areas, and finally, the numbers are checked. This process consumes one to two days for several workers, and often there are problems with aligning the counts or uncertainties about whether each cow has been counted.
Significant time can be saved if we have a way to inventory reserve cows quickly and accurately.
Second is identifying lame cattle
Currently, most dairy companies use a breed named Holstein to produce milk. Holsteins are the black and white cows most of us are familiar with. Despite most dairy companies using the same breed, there are still differences in milk production quantity and quality among different companies and ranches. This is because the health of dairy cows directly affects milk production.
However, cows cannot express discomfort on their own like humans can, and it isn’t practical for veterinarians to give thousands of cows physical examinations regularly. Therefore, we have to use external indicators to quickly judge the health status of cows.
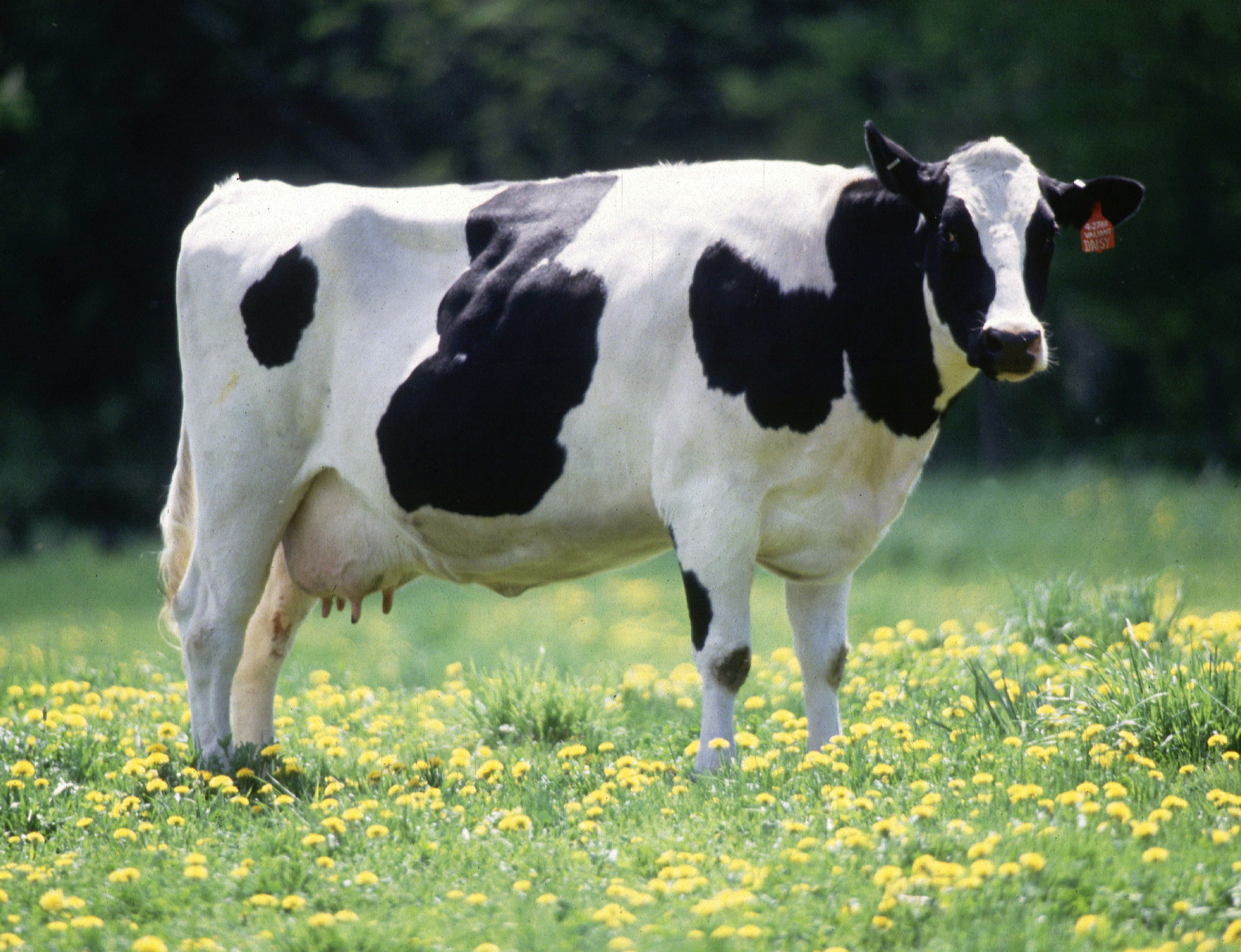
The external indicators of a cow’s health include body condition score and lameness degree. Body condition score is largely related to the cow’s body fat percentage and is a long-term indicator, while lameness is a short-term indicator caused by leg problems or foot infections and other issues that affect the cow’s mood, health, and milk production. Additionally, adult Holstein cows can weigh over 500 kg, which can cause significant harm to their feet if they aren’t stable. Therefore, when lameness occurs, veterinarians should intervene as soon as possible.
According to a 2014 study, the proportion of severely lame cows in China can be as high as 31 percent. Although the situation might have improved since the study, the veterinarian count on farms is extremely limited, making it difficult to monitor cows regularly. When lameness is detected, the situation is often severe, and treatment is time-consuming and difficult, and milk production is already affected.
If we have a way to timely detect lameness in cows and prompt veterinarians to intervene at the mild lameness stage, the overall health and milk production of the cows will increase, and the performance of the farm will improve.
Lastly, there is feed cost optimization
Within the livestock industry, feed is the biggest variable cost. To ensure the quality and inventory of feed, farms often need to purchase feed ingredients from domestic and overseas suppliers and deliver them to feed formulation factories for processing. There are many types of modern feed ingredients, including soybean meal, corn, alfalfa, oat grass, and so on, which means that there are many variables at play. Each type of feed ingredient has its own price cycle and price fluctuations. During significant fluctuations, the total cost of feed can fluctuate by more than 15 percent, causing a significant impact.
Feed costs fluctuate, but dairy product prices are relatively stable over the long term. Consequently, under otherwise unchanged conditions, the overall profit can fluctuate significantly purely due to feed cost changes.
To avoid this fluctuation, it’s necessary to consider storing more ingredients when prices are low. But stocking also needs to consider whether the price is genuinely at the trough and what quantity of feed should be purchased according to the current consumption rate.
If we have a way to precisely forecast feed consumption and combine it with the overall price trend to suggest the best time and quantity of feed to purchase, we can reduce costs and increase efficiency on the farm.
It’s evident that these issues are directly related to the customer’s goal of improving farm operational efficiency, and the methods are respectively freeing up labor, increasing production and reducing costs. Through discussions on the difficulty and value of solving each issue, we chose increasing production as the starting point and prioritized solving the problem of lame cows.
Research
Before discussing technology, research had to be conducted. The research was jointly conducted by the AWS customer team, the AWS Generative AI Innovation Center, which managed the machine learning algorithm models, and AWS AI Shanghai Lablet, which provides algorithm consultation on the latest computer vision research and the expert farming team from New Hope Dairy. The research was divided into several parts:
- Understanding the traditional paper-based identification method of lame cows and developing a basic understanding of what lame cows are.
- Confirming existing solutions, including those used in farms and in the industry.
- Conducting farm environment research to understand the physical situation and limitations.
Through studying materials and observing on-site videos, the teams gained a basic understanding of lame cows. Readers can also get a basic idea of the posture of lame cows through the animated image below.
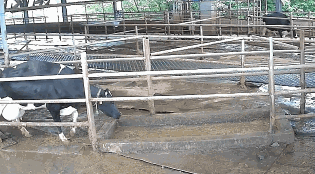
In contrast to a relatively healthy cow.
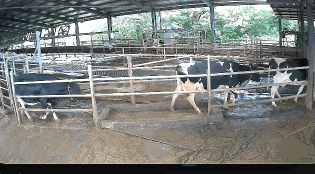
Lame cows have visible differences in posture and gait compared to healthy cows.
Regarding existing solutions, most ranches rely on visual inspection by veterinarians and nutritionists to identify lame cows. In the industry, there are solutions that use wearable pedometers and accelerometers for identification, as well as solutions that use partitioned weighbridges for identification, but both are relatively expensive. For the highly competitive dairy industry, we need to minimize identification costs and the costs and dependence on non-generic hardware.
After discussing and analyzing the information with ranch veterinarians and nutritionists, the AWS Generative AI Innovation Center experts decided to use computer vision (CV) for identification, relying only on ordinary hardware: civilian surveillance cameras, which don’t add any additional burden to the cows and reduce costs and usage barriers.
After deciding on this direction, we visited a medium-sized farm with thousands of cows on site, investigated the ranch environment, and determined the location and angle of camera placement.
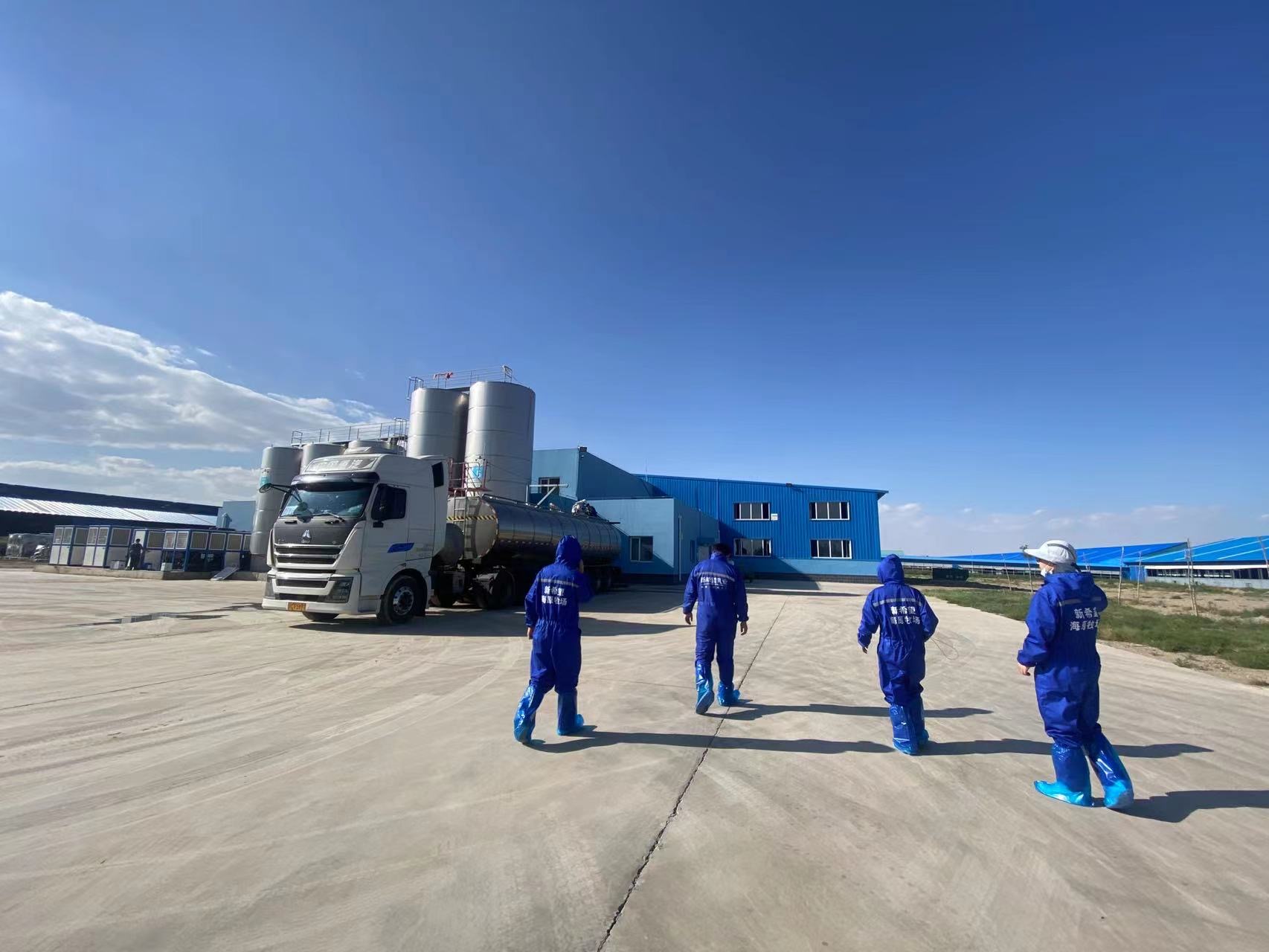
Initial proposal
Now, for the solution. The core of our CV-based solution consists of the following steps:
- Cow identification: Identify multiple cows in a single frame of video and mark the position of each cow.
- Cow tracking: While video is recording, we need to continuously track cows as the frames change and assign a unique number to each cow.
- Posture marking: Reduce the dimensionality of cow movements by converting cow images to marked points.
- Anomaly identification: Identify anomalies in the marked points’ dynamics.
- Lame cow algorithm: Normalize the anomalies to obtain a score to determine the degree of cow lameness.
- Threshold determination: Obtain a threshold based on expert inputs.
According to the judgment of the AWS Generative AI Innovation Center experts, the first few steps are generic requirements that can be solved using open-source models, while the latter steps require us to use mathematical methods and expert intervention.
Difficulties in the solution
To balance cost and performance, we chose the yolov5l model, a medium-sized pre-trained model for cow recognition, with an input width of 640 pixels, which provides good value for this scene.
While YOLOv5 is responsible for recognizing and tagging cows in a single image, in reality, videos consist of multiple images (frames) that change continuously. YOLOv5 cannot identify that cows in different frames belong to the same individual. To track and locate a cow across multiple images, another model called SORT is needed.
SORT stands for simple online and realtime tracking, where online means it considers only the current and previous frames to track without consideration of any other frames, and realtime means it can identify the object’s identity immediately.
After the development of SORT, many engineers implemented and optimized it, leading to the development of OC-SORT, which considers the appearance of the object, DeepSORT (and its upgraded version, StrongSORT), which includes human appearance, and ByteTrack, which uses a two-stage association linker to consider low-confidence recognition. After testing, we found that for our scene, DeepSORT’s appearance tracking algorithm is more suitable for humans than for cows, and ByteTrack’s tracking accuracy is slightly weaker. As a result, we ultimately chose OC-SORT as our tracking algorithm.
Next, we use DeepLabCut (DLC for short) to mark the skeletal points of the cows. DLC is a markerless model, which means that although different points, such as the head and limbs, might have different meanings, they are all just points for DLC, which only requires us to mark the points and train the model.
This leads to a new question: how many points should we mark on each cow and where should we mark them? The answer to this question affects the workload of marking, training, and subsequent inference efficiency. To solve this problem, we must first understand how to identify lame cows.
Based on our research and the inputs of our expert clients, lame cows in videos exhibit the following characteristics:
- An arched back: The neck and back are curved, forming a triangle with the root of the neck bone (arched-back).
- Frequent nodding: Each step can cause the cow to lose balance or slip, resulting in frequent nodding (head bobbing).
- Unstable gait: The cow’s gait changes after a few steps, with slight pauses (gait pattern change).
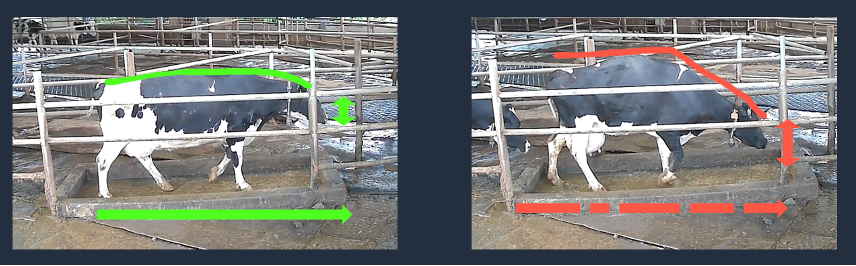
With regards to neck and back curvature as well as nodding, experts from AWS Generative AI Innovation Center have determined that marking only seven back points (one on the head, one at the base of the neck, and five on the back) on cattle can result in good identification. Since we now have a frame of identification, we should also be able to recognize unstable gait patterns.
Next, we use mathematical expressions to represent the identification results and form algorithms.
Human identification of these problems isn’t difficult, but precise algorithms are required for computer identification. For example, how does a program know the degree of curvature of a cow’s back given a set of cow back coordinate points? How does it know if a cow is nodding?
In terms of back curvature, we first consider treating the cow’s back as an angle and then we find the vertex of that angle, which allows us to calculate the angle. The problem with this method is that the spine might have bidirectional curvature, making the vertex of the angle difficult to identify. This requires switching to other algorithms to solve the problem.
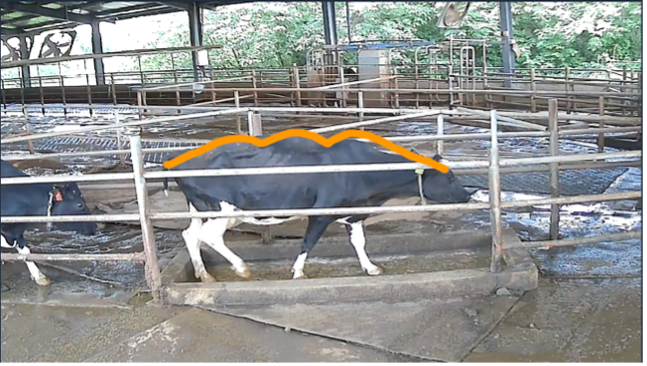
In terms of nodding, we first considered using the Fréchet distance to determine if the cow is nodding by comparing the difference in the curve of the cow’s overall posture. However, the problem is that the cow’s skeletal points might be displaced, causing significant distance between similar curves. To solve this problem, we need to take out the position of the head relative to the recognition box and normalize it.
After normalizing the position of the head, we encountered a new problem. In the image that follows, the graph on the left shows the change in the position of the cow’s head. We can see that due to recognition accuracy issues, the position of the head point will constantly shake slightly. We need to remove these small movements and find the relatively large movement trend of the head. This is where some knowledge of signal processing is needed. By using a Savitzky-Golay filter, we can smooth out a signal and obtain its overall trend, making it easier for us to identify nodding, as shown by the orange curve in the graph on the right.
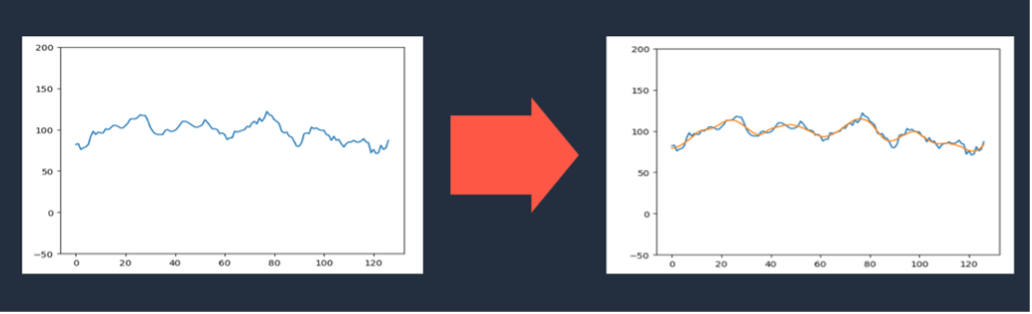
Additionally, after dozens of hours of video recognition, we found that some cows with extremely high back curvature actually did not have a hunched back. Further investigation revealed that this was because most of the cows used to train the DLC model were mostly black or black and white, and there weren’t many cows that were mostly white or close to pure white, resulting in the model recognizing them incorrectly when they had large white areas on their bodies, as shown by the red arrow in the figure below. This can be corrected through further model training.
In addition to solving the preceding problems, there were other generic problems that needed to be solved:
- There are two paths in the video frame, and cows in the distance might also be recognized, causing problems.
- The paths in the video also have a certain curvature, and the cow’s body length becomes shorter when the cow is on the sides of the path, making the posture easy to identify incorrectly.
- Due to the overlap of multiple cows or occlusion from the fence, the same cow might be identified as two cows.
- Due to tracking parameters and occasional frame skipping of the camera, it’s impossible to correctly track the cows, resulting in ID confusion issues.
In the short term, based on the alignment with New Hope Dairy on delivering a minimum viable product and then iterate on it, these problems can usually be solved by outlier judgment algorithms combined with confidence filtering, and if they cannot be solved, they will become invalid data, which requires us to perform additional training and continuously iterate our algorithms and models.
In the long term, AWS AI Shanghai Lablet provided future experiment suggestions to solve the preceding problems based on their object-centric research: Bridging the Gap to Real-World Object-Centric Learning and Self-supervised Amodal Video Object Segmentation. Besides invalidating those outlier data, the issues can also be addressed by developing more precise object-level models for pose estimation, amodal segmentation, and supervised tracking. However, traditional vision pipelines for these tasks typically require extensive labeling. Object-centric learning focuses on tackling the binding problem of pixels to objects without additional supervision. The binding process not only provides information on the location of objects but also results in robust and adaptable object representations for downstream tasks. Because the object-centric pipeline focuses on self-supervised or weakly-supervised settings, we can improve performance without significantly increasing labeling costs for our customers.
After solving a series of problems and combining the scores given by the farm veterinarian and nutritionist, we have obtained a comprehensive lameness score for cows, which helps us identify cows with different degrees of lameness such as severe, moderate, and mild, and can also identify multiple body posture attributes of cows, helping further analysis and judgment.
Within weeks, we developed an end-to-end solution for identifying lame cows. The hardware camera for this solution cost only 300 RMB, and the Amazon SageMaker batch inference, when using the g4dn.xlarge instance, took about 50 hours for 2 hours of video, totaling only 300 RMB. When it enters production, if five batches of cows are detected per week (assuming about 10 hours), and including the rolling saved videos and data, the monthly detection cost for a medium-sized ranch with several thousand cows is less than 10,000 RMB.
Currently, our machine learning model process is as follows:
- Raw video is recorded.
- Cows are detected and identified.
- Each cow is tracked, and key points are detected.
- Each cow’s movement is analyzed.
- A lameness score is determined.
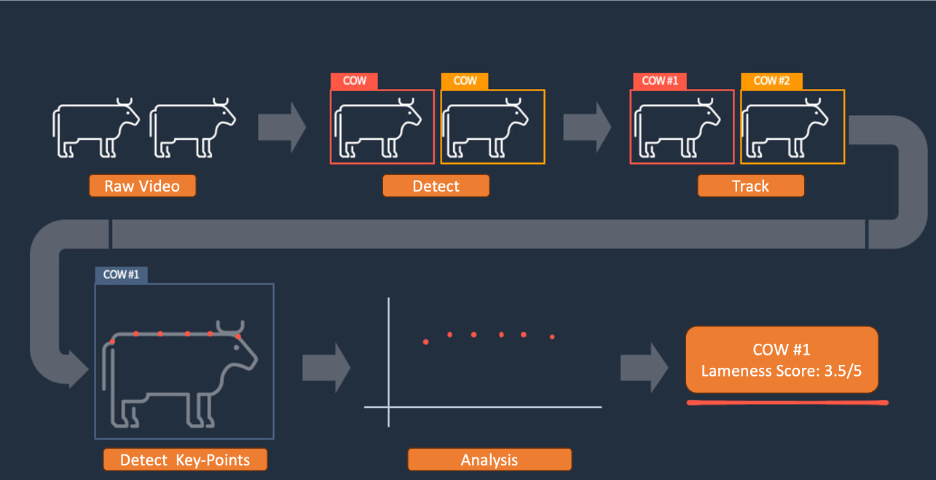
Model deployment
We’ve described the solution for identifying lame cows based on machine learning before. Now, we need to deploy these models on SageMaker. As shown in the following figure:
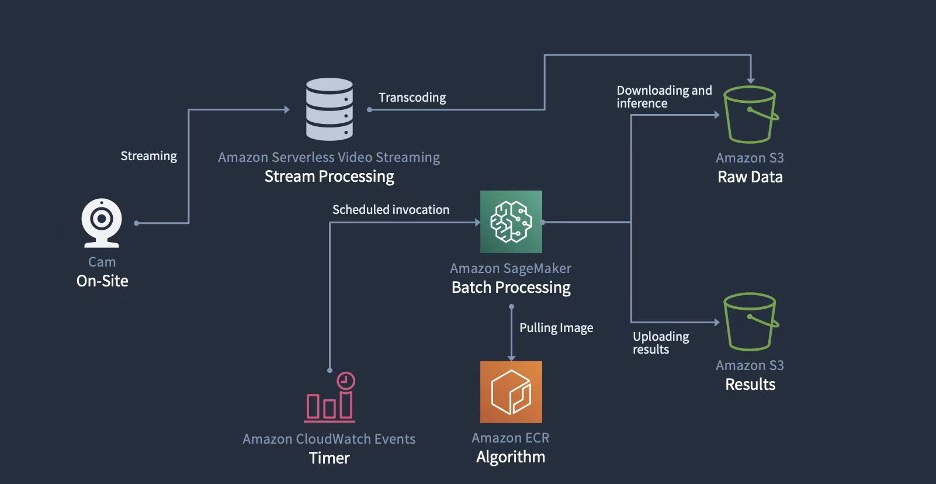
Business implementation
Of course, what we’ve discussed so far is just the core of our technical solution. To integrate the entire solution into the business process, we also must address the following issues:
- Data feedback: For example, we must provide veterinarians with an interface to filter and view lame cows that need to be processed and collect data during this process to use as training data.
- Cow identification: After a veterinarian sees a lame cow, they also need to know the cow’s identity, such as its number and pen.
- Cow positioning: In a pen with hundreds of cows, quickly locate the target cow.
- Data mining: For example, find out how the degree of lameness affects feeding, rumination, rest, and milk production.
- Data-driven: For example, identify the genetic, physiological, and behavioral characteristics of lame cows to achieve optimal breeding and reproduction.
Only by addressing these issues can the solution truly solve the business problem, and the collected data can generate long-term value. Some of these problems are system integration issues, while others are technology and business integration issues. We will share further information about these issues in future articles.
Summary
In this article, we briefly explained how the AWS Customer Solutions team innovates quickly based on the customer’s business. This mechanism has several characteristics:
- Business led: Prioritize understanding the customer’s industry and business processes on site and in person before discussing technology, and then delve into the customer’s pain points, challenges, and problems to identify important issues that can be solved with technology.
- Immediately available: Provide a simple but complete and usable prototype directly to the customer for testing, validation, and rapid iteration within weeks, not months.
- Minimal cost: Minimize or even eliminate the customer’s costs before the value is truly validated, avoiding concerns about the future. This aligns with the AWS frugality leadership principle.
In our collaborative innovation project with the dairy industry, we not only started from the business perspective to identify specific business problems with business experts, but also conducted on-site investigations at the farm and factory with the customer. We determined the camera placement on site, installed and deployed the cameras, and deployed the video streaming solution. Experts from AWS Generative AI Innovation Center dissected the customer’s requirements and developed an algorithm, which was then engineered by a solution architect for the entire algorithm.
With each inference, we could obtain thousands of decomposed and tagged cow walking videos, each with the original video ID, cow ID, lameness score, and various detailed scores. The complete calculation logic and raw gait data were also retained for subsequent algorithm optimization.
Lameness data can not only be used for early intervention by veterinarians, but also combined with milking machine data for cross-analysis, providing an additional validation dimension and answering some additional business questions, such as: What are the physical characteristics of cows with the highest milk yield? What is the effect of lameness on milk production in cows? What is the main cause of lame cows, and how can it be prevented? This information will provide new ideas for farm operations.
The story of identifying lame cows ends here, but the story of farm innovation has just begun. In subsequent articles, we will continue to discuss how we work closely with customers to solve other problems.
About the Authors
Hao Huang is an applied scientist at the AWS Generative AI Innovation Center. He specializes in Computer Vision (CV) and Visual-Language Model (VLM). Recently, he has developed a strong interest in generative AI technologies and has already collaborated with customers to apply these cutting-edge technologies to their business. He is also a reviewer for AI conferences such as ICCV and AAAI.
Peiyang He is a senior data scientist at the AWS Generative AI Innovation Center. She works with customers across a diverse spectrum of industries to solve their most pressing and innovative business needs leveraging GenAI/ML solutions. In her spare time, she enjoys skiing and traveling.
Xuefeng Liu leads a science team at the AWS Generative AI Innovation Center in the Asia Pacific and Greater China regions. His team partners with AWS customers on generative AI projects, with the goal of accelerating customers’ adoption of generative AI.
Tianjun Xiao is a senior applied scientist at the AWS AI Shanghai Lablet, co-leading the computer vision efforts. Presently, his primary focus lies in the realms of multimodal foundation models and object-centric learning. He is actively investigating their potential in diverse applications, including video analysis, 3D vision and autonomous driving.
Zhang Dai is a an AWS senior solution architect for China Geo Business Sector. He helps companies of various sizes achieve their business goals by providing consultancy on business processes, user experience and cloud technology. He is a prolific blog writer and also author of two books: The Modern Autodidact and Designing Experience.
Jianyu Zeng is a senior customer solutions manager at AWS, whose responsibility is to support customers, such as New Hope group, during their cloud transition and assist them in realizing business value through cloud-based technology solutions. With a strong interest in artificial intelligence, he is constantly exploring ways to leverage AI to drive innovative changes in our customer’s businesses.
Carol Tong Min is a senior business development manager, responsible for Key Accounts in GCR GEO West, including two important enterprise customers: Jiannanchun Group and New Hope Group. She is customer obsessed, and always passionate about supporting and accelerating customers’ cloud journey.
Nick Jiang is a senior specialist sales at AIML SSO team in China. He is focus on transferring innovative AIML solutions and helping with customer to build the AI related workloads within AWS.
Read More