Large language models (LLMs) with their broad knowledge, can generate human-like text on almost any topic. However, their training on massive datasets also limits their usefulness for specialized tasks. Without continued learning, these models remain oblivious to new data and trends that emerge after their initial training. Furthermore, the cost to train new LLMs can prove prohibitive for many enterprise settings. However, it’s possible to cross-reference a model answer with the original specialized content, thereby avoiding the need to train a new LLM model, using Retrieval-Augmented Generation (RAG).
RAG empowers LLMs by giving them the ability to retrieve and incorporate external knowledge. Instead of relying solely on their pre-trained knowledge, RAG allows models to pull data from documents, databases, and more. The model then skillfully integrates this outside information into its generated text. By sourcing context-relevant data, the model can provide informed, up-to-date responses tailored to your use case. The knowledge augmentation also reduces the likelihood of hallucinations and inaccurate or nonsensical text. With RAG, foundation models become adaptable experts that evolve as your knowledge base grows.
Today, we are excited to unveil three generative AI demos, licensed under MIT-0 license:
- Amazon Kendra with foundational LLM – Utilizes the deep search capabilities of Amazon Kendra combined with the expansive knowledge of LLMs. This integration provides precise and context-aware answers to complex queries by drawing from a diverse range of sources.
- Embeddings model with foundational LLM – Merges the power of embeddings—a technique to capture semantic meanings of words and phrases—with the vast knowledge base of LLMs. This synergy enables more accurate topic modeling, content recommendation, and semantic search capabilities.
- Foundation Models Pharma Ad Generator – A specialized application tailored for the pharmaceutical industry. Harnessing the generative capabilities of foundational models, this tool creates convincing and compliant pharmaceutical advertisements, ensuring content adheres to industry standards and regulations.
These demos can be seamlessly deployed in your AWS account, offering foundational insights and guidance on utilizing AWS services to create a state-of-the-art LLM generative AI question and answer bot and content generation.
In this post, we explore how RAG combined with Amazon Kendra or custom embeddings can overcome these challenges and provide refined responses to natural language queries.
Solution overview
By adopting this solution, you can gain the following benefits:
- Improved information access – RAG allows models to pull in information from vast external sources, which can be especially useful when the pre-trained model’s knowledge is outdated or incomplete.
- Scalability – Instead of training a model on all available data, RAG allows models to retrieve relevant information on the fly. This means that as new data becomes available, it can be added to the retrieval database without needing to retrain the entire model.
- Memory efficiency – LLMs require significant memory to store parameters. With RAG, the model can be smaller because it doesn’t need to memorize all details; it can retrieve them when needed.
- Dynamic knowledge update – Unlike conventional models with a set knowledge endpoint, RAG’s external database can undergo regular updates, granting the model access to up-to-date information. The retrieval function can be fine-tuned for distinct tasks. For example, a medical diagnostic task can source data from medical journals, ensuring the model garners expert and pertinent insights.
- Bias mitigation – The ability to draw from a well-curated database offers the potential to minimize biases by ensuring balanced and impartial external sources.
Before diving into the integration of Amazon Kendra with foundational LLMs, it’s crucial to equip yourself with the necessary tools and system requirements. Having the right setup in place is the first step towards a seamless deployment of the demos.
Prerequisites
You must have the following prerequisites:
Although it’s possible to set up and deploy the infrastructure detailed in this tutorial from your local computer, AWS Cloud9 offers a convenient alternative. Pre-equipped with tools like AWS CLI, AWS CDK, and Docker, AWS Cloud9 can function as your deployment workstation. To use this service, simply set up the environment via the AWS Cloud9 console.
With the prerequisites out of the way, let’s dive into the features and capabilities of Amazon Kendra with foundational LLMs.
Amazon Kendra with foundational LLM
Amazon Kendra is an advanced enterprise search service enhanced by machine learning (ML) that provides out-of-the-box semantic search capabilities. Utilizing natural language processing (NLP), Amazon Kendra comprehends both the content of documents and the underlying intent of user queries, positioning it as a content retrieval tool for RAG based solutions. By using the high-accuracy search content from Kendra as a RAG payload, you can get better LLM responses. The use of Amazon Kendra in this solution also enables personalized search by filtering responses according to the end-user content access permissions.
The following diagram shows the architecture of a generative AI application using the RAG approach.
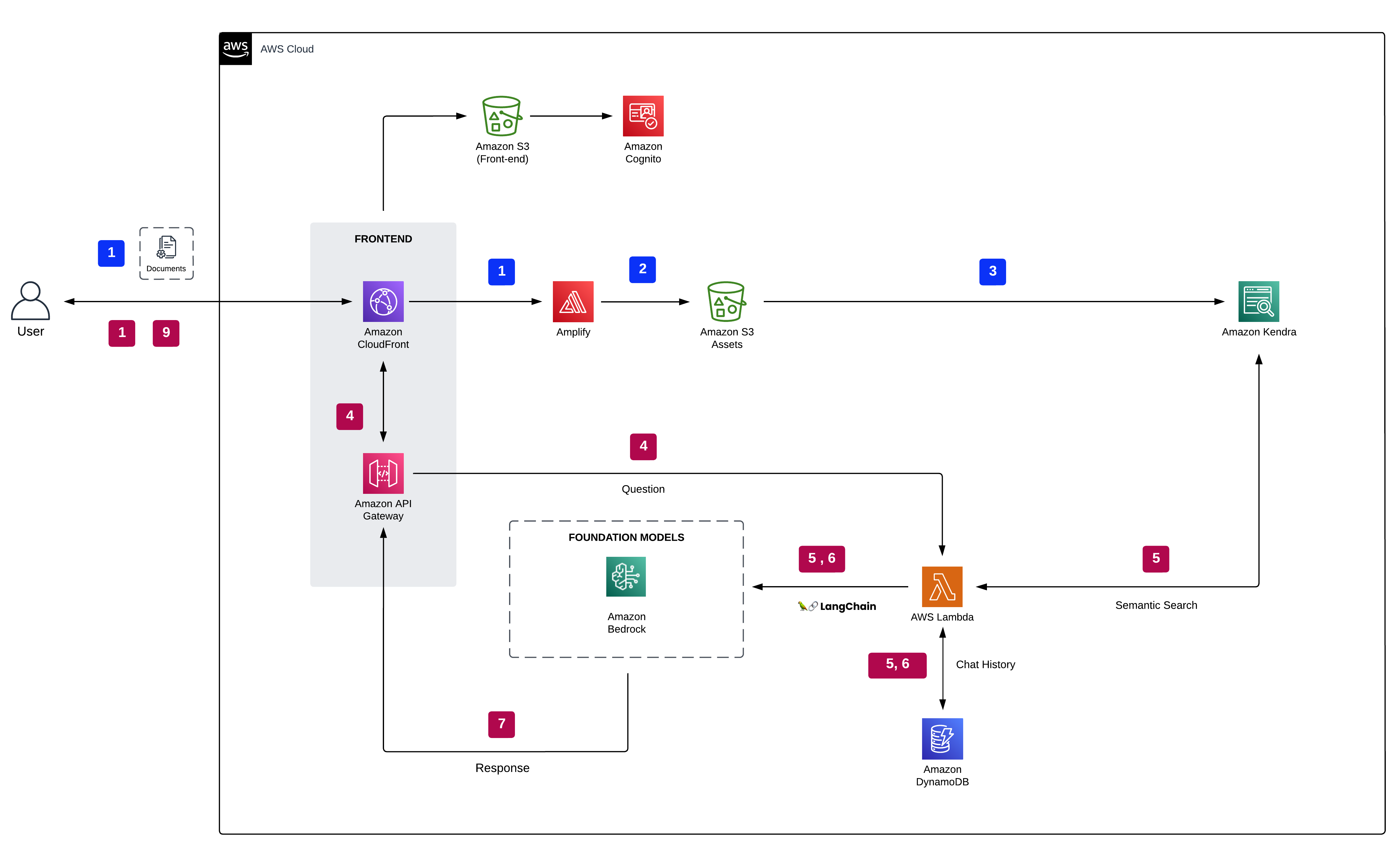
Documents are processed and indexed by Amazon Kendra through the Amazon Simple Storage Service (Amazon S3) connector. Customer requests and contextual data from Amazon Kendra are directed to an Amazon Bedrock foundation model. The demo lets you choose between Amazon’s Titan, AI21’s Jurassic, and Anthropic’s Claude models supported by Amazon Bedrock. The conversation history is saved in Amazon DynamoDB, offering added context for the LLM to generate responses.
We have provided this demo in the GitHub repo. Refer to the deployment instructions within the readme file for deploying it into your AWS account.
The following steps outline the process when a user interacts with the generative AI app:
- The user logs in to the web app authenticated by Amazon Cognito.
- The user uploads one or more documents into Amazon S3.
- The user runs an Amazon Kendra sync job to ingest S3 documents into the Amazon Kendra index.
- The user’s question is routed through a secure WebSocket API hosted on Amazon API Gateway backed by a AWS Lambda function.
- The Lambda function, empowered by the LangChain framework—a versatile tool designed for creating applications driven by AI language models—connects to the Amazon Bedrock endpoint to rephrase the user’s question based on chat history. After rephrasing, the question is forwarded to Amazon Kendra using the Retrieve API. In response, the Amazon Kendra index displays search outcomes, providing excerpts from pertinent documents sourced from the enterprise’s ingested data.
- The user’s question along with the data retrieved from the index are sent as a context in the LLM prompt. The response from the LLM is stored as chat history within DynamoDB.
- Finally, the response from the LLM is sent back to the user.
Document indexing workflow
The following is the procedure for processing and indexing documents:
- Users submit documents via the user interface (UI).
- Documents are transferred to an S3 bucket utilizing the AWS Amplify API.
- Amazon Kendra indexes new documents in the S3 bucket through the Amazon Kendra S3 connector.
Benefits
The following list highlights the advantages of this solution:
- Enterprise-level retrieval – Amazon Kendra is designed for enterprise search, making it suitable for organizations with vast amounts of structured and unstructured data.
- Semantic understanding – The ML capabilities of Amazon Kendra ensure that retrieval is based on deep semantic understanding and not just keyword matches.
- Scalability – Amazon Kendra can handle large-scale data sources and provides quick and relevant search results.
- Flexibility – The foundational model can generate answers based on a wide range of contexts, ensuring the system remains versatile.
- Integration capabilities – Amazon Kendra can be integrated with various AWS services and data sources, making it adaptable for different organizational needs.
Embeddings model with foundational LLM
An embedding is a numerical vector that represents the core essence of diverse data types, including text, images, audio, and documents. This representation not only captures the data’s intrinsic meaning, but also adapts it for a wide range of practical applications. Embedding models, a branch of ML, transform complex data, such as words or phrases, into continuous vector spaces. These vectors inherently grasp the semantic connections between data, enabling deeper and more insightful comparisons.
RAG seamlessly combines the strengths of foundational models, like transformers, with the precision of embeddings to sift through vast databases for pertinent information. Upon receiving a query, the system utilizes embeddings to identify and extract relevant sections from an extensive body of data. The foundational model then formulates a contextually precise response based on this extracted information. This perfect synergy between data retrieval and response generation allows the system to provide thorough answers, drawing from the vast knowledge stored in expansive databases.
In the architectural layout, based on their UI selection, users are guided to either the Amazon Bedrock or Amazon SageMaker JumpStart foundation models. Documents undergo processing, and vector embeddings are produced by the embeddings model. These embeddings are then indexed using FAISS to enable efficient semantic search. Conversation histories are preserved in DynamoDB, enriching the context for the LLM to craft responses.
The following diagram illustrates the solution architecture and workflow.
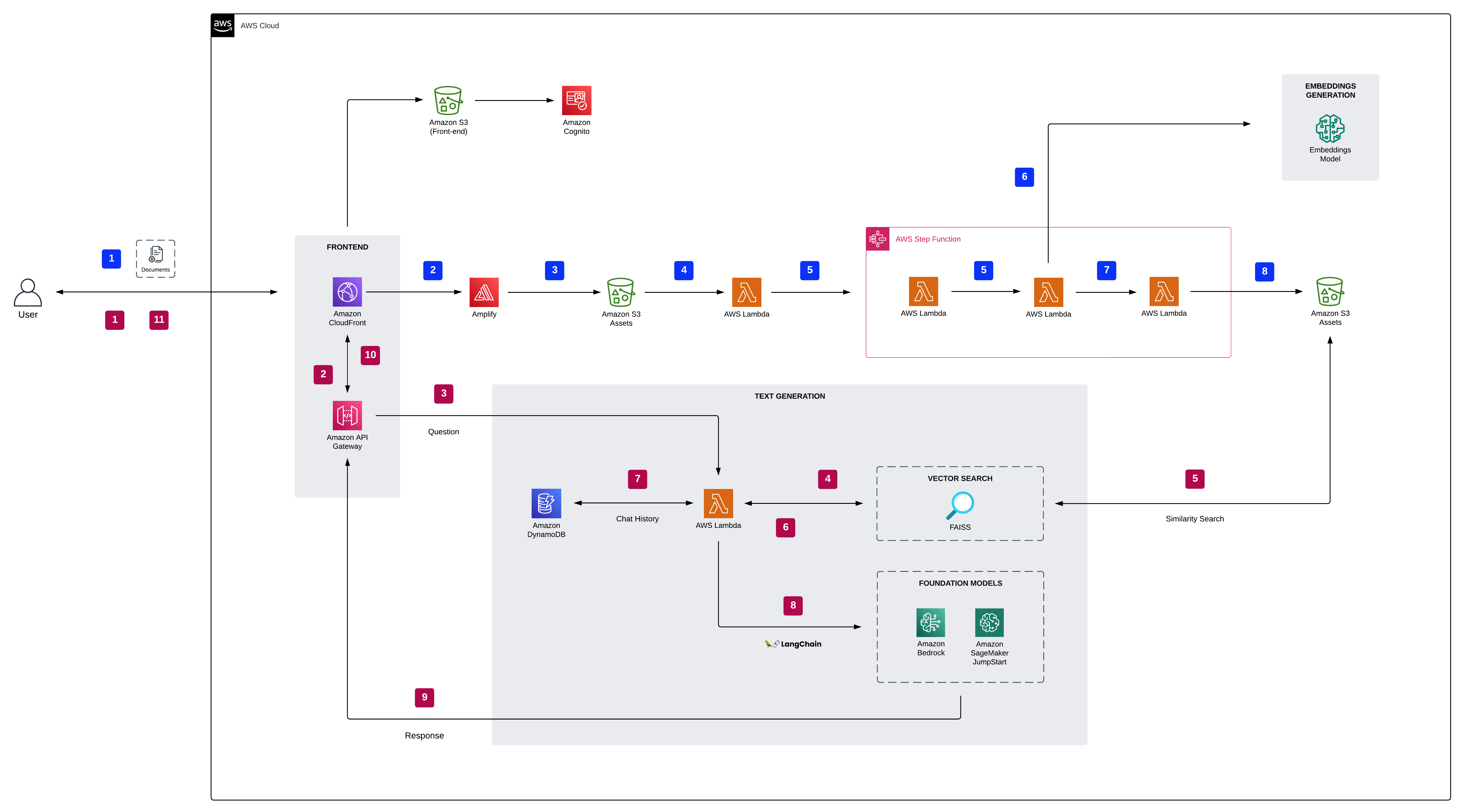
We have provided this demo in the GitHub repo. Refer to the deployment instructions within the readme file for deploying it into your AWS account.
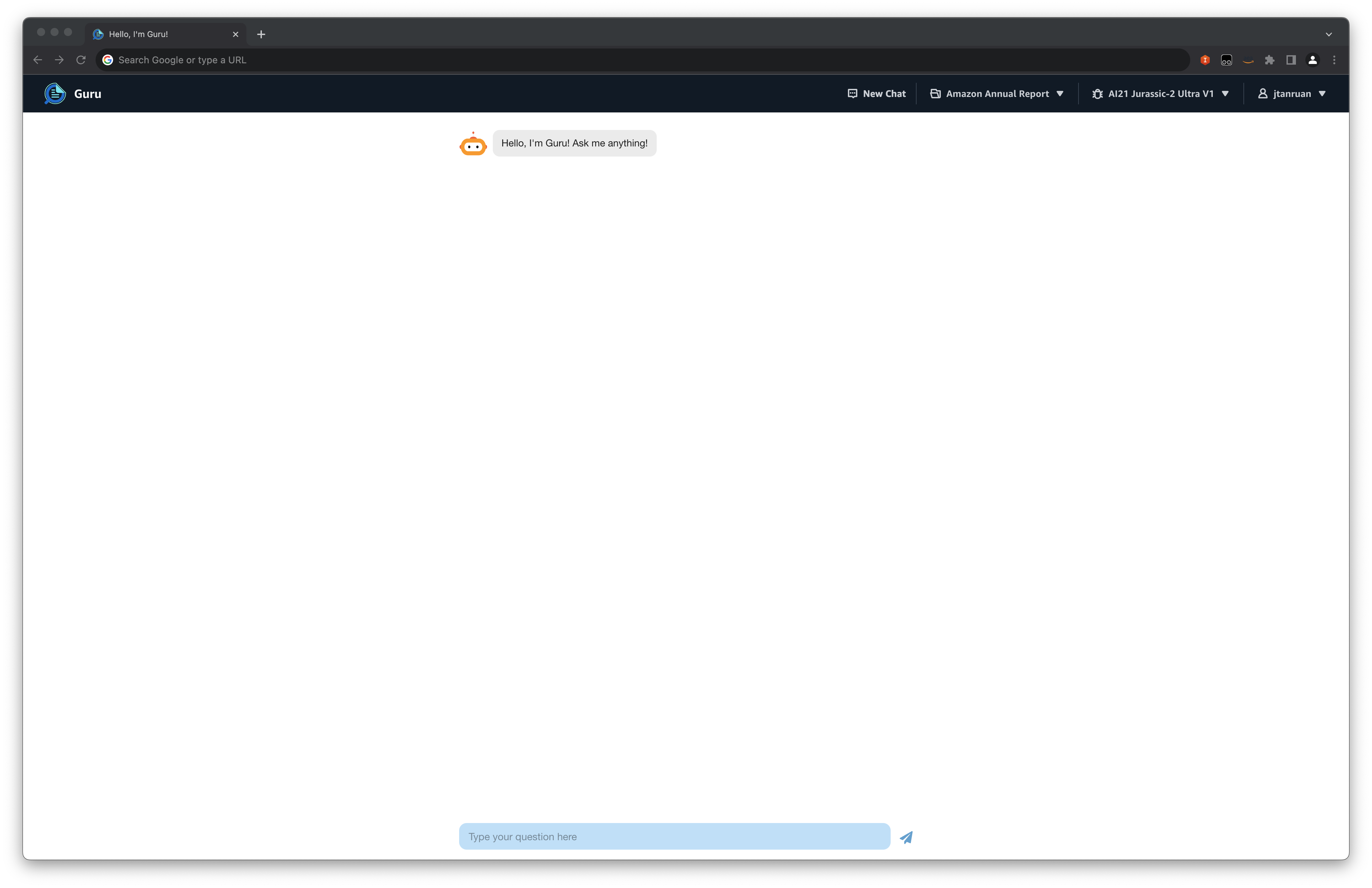
Embeddings model
The responsibilities of the embeddings model are as follows:
- This model is responsible for converting text (like documents or passages) into dense vector representations, commonly known as embeddings.
- These embeddings capture the semantic meaning of the text, allowing for efficient and semantically meaningful comparisons between different pieces of text.
- The embeddings model can be trained on the same vast corpus as the foundational model or can be specialized for specific domains.
Q&A workflow
The following steps describe the workflow of the question answering over documents:
- The user logs in to the web app authenticated by Amazon Cognito.
- The user uploads one or more documents to Amazon S3.
- Upon document transfer, an S3 event notification triggers a Lambda function, which then calls the SageMaker embedding model endpoint to generate embeddings for the new document. The embeddings model converts the question into a dense vector representation (embedding). The resulting vector file is securely stored within the S3 bucket.
- The FAISS retriever compares this question embedding with the embeddings of all documents or passages in the database to find the most relevant passages.
- The passages, along with the user’s question, are provided as context to the foundational model. The Lambda function uses the LangChain library and connects to the Amazon Bedrock or SageMaker JumpStart endpoint with a context-stuffed query.
- The response from the LLM is stored in DynamoDB along with the user’s query, the timestamp, a unique identifier, and other arbitrary identifiers for the item such as question category. Storing the question and answer as discrete items allows the Lambda function to easily recreate a user’s conversation history based on the time when questions were asked.
- Finally, the response is sent back to the user via a HTTPs request through the API Gateway WebSocket API integration response.
Benefits
The following list describe the benefits of this solution:
- Semantic understanding – The embeddings model ensures that the retriever selects passages based on deep semantic understanding, not just keyword matches.
- Scalability – Embeddings allow for efficient similarity comparisons, making it feasible to search through vast databases of documents quickly.
- Flexibility – The foundational model can generate answers based on a wide range of contexts, ensuring the system remains versatile.
- Domain adaptability – The embeddings model can be trained or fine-tuned for specific domains, allowing the system to be adapted for various applications.
Foundation Models Pharma Ad Generator
In today’s fast-paced pharmaceutical industry, efficient and localized advertising is more crucial than ever. This is where an innovative solution comes into play, using the power of generative AI to craft localized pharma ads from source images and PDFs. Beyond merely speeding up the ad generation process, this approach streamlines the Medical Legal Review (MLR) process. MLR is a rigorous review mechanism in which medical, legal, and regulatory teams meticulously evaluate promotional materials to guarantee their accuracy, scientific backing, and regulatory compliance. Traditional content creation methods can be cumbersome, often requiring manual adjustments and extensive reviews to ensure alignment with regional compliance and relevance. However, with the advent of generative AI, we can now automate the crafting of ads that truly resonate with local audiences, all while upholding stringent standards and guidelines.
The following diagram illustrates the solution architecture.
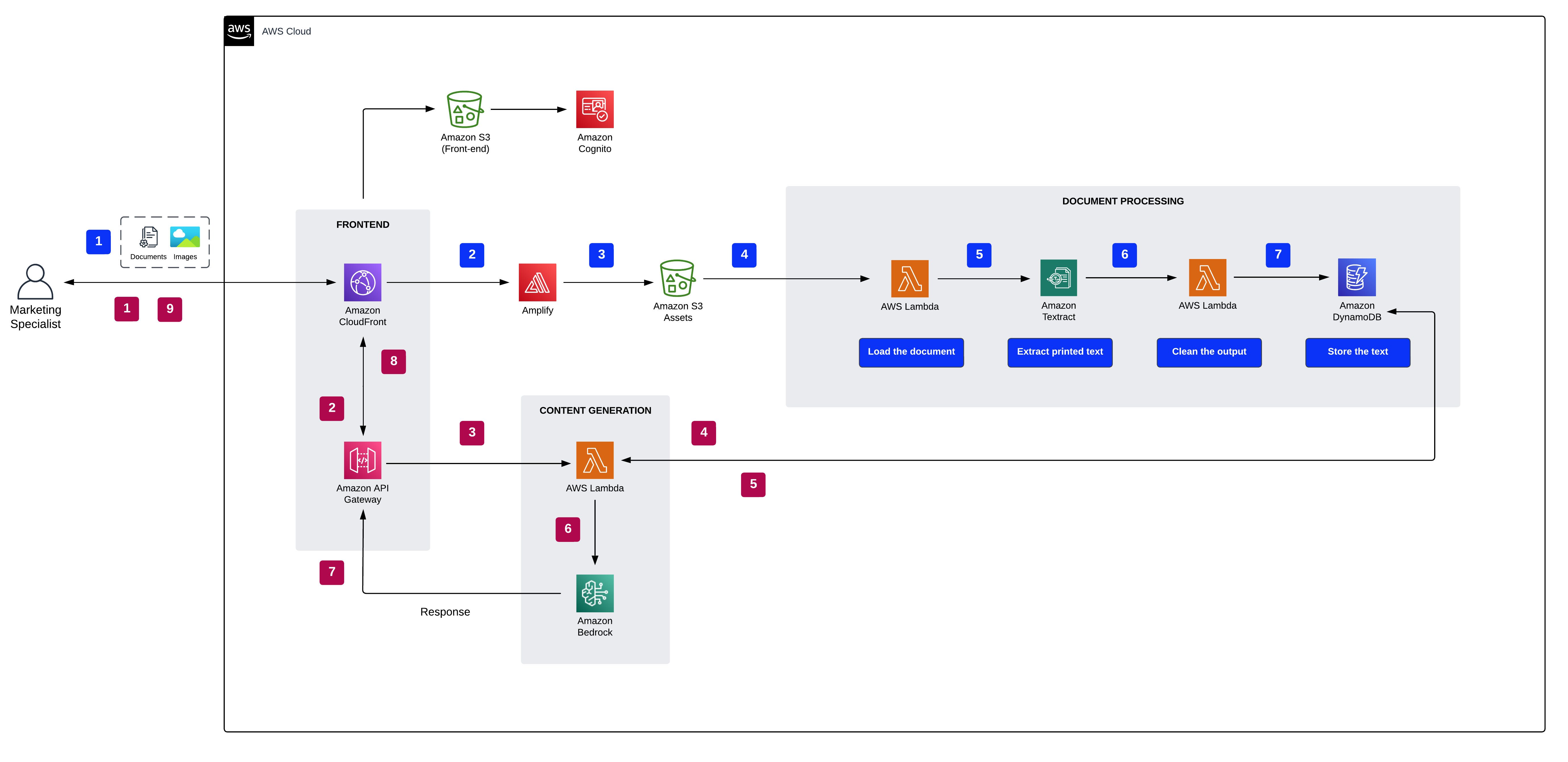
In the architectural layout, based on their selected model and ad preferences, users are seamlessly guided to the Amazon Bedrock foundation models. This streamlined approach ensures that new ads are generated precisely according to the desired configuration. As part of the process, documents are efficiently handled by Amazon Textract, with the resultant text securely stored in DynamoDB. A standout feature is the modular design for image and text generation, granting you the flexibility to independently regenerate any component as required.
We have provided this demo in the GitHub repo. Refer to the deployment instructions within the readme file for deploying it into your AWS account.
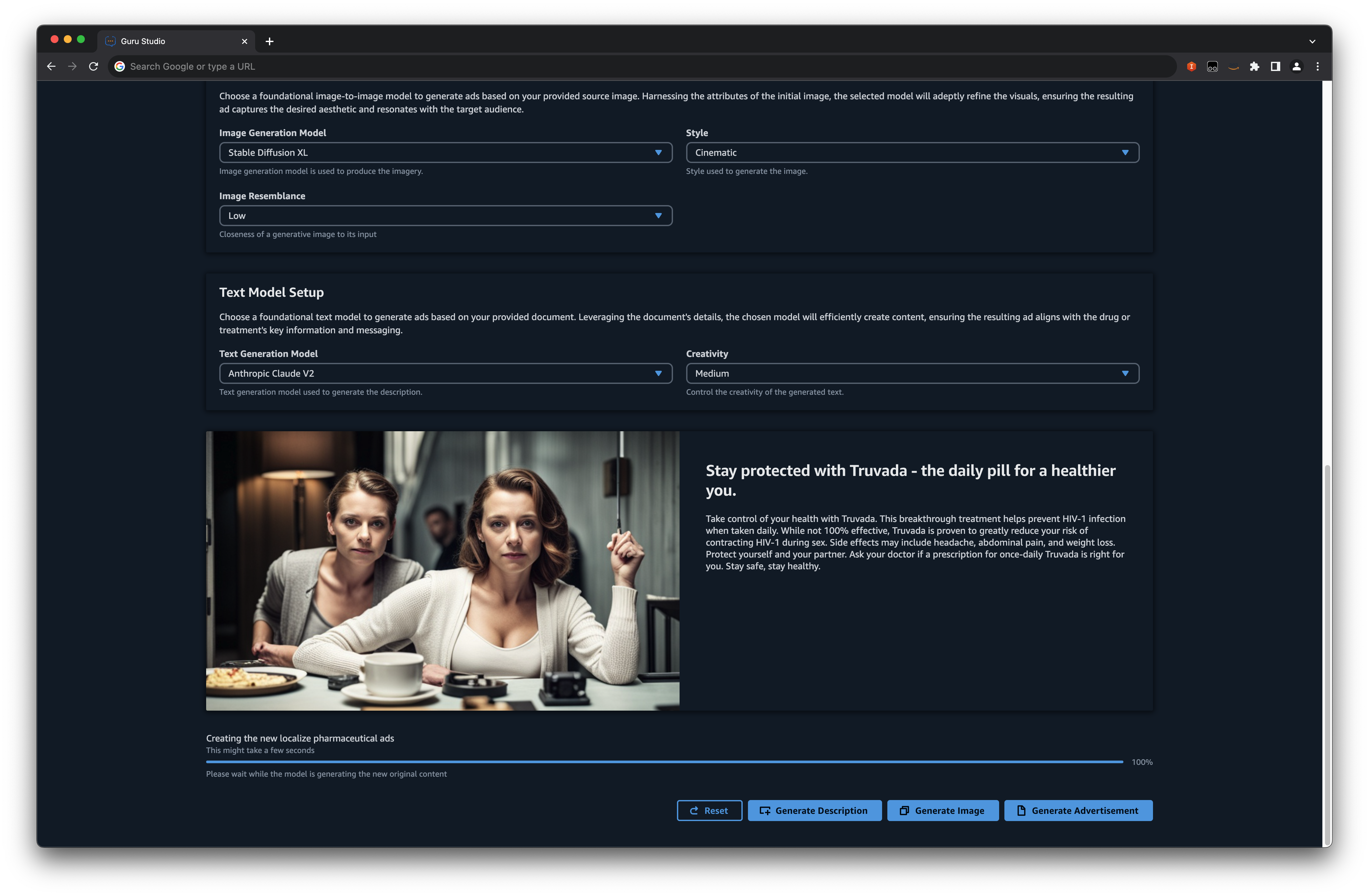
Content generation workflow
The following steps outline the process for content generation:
- The user chooses their document, source image, ad placement, language, and image style.
- Secure access to the web application is ensured through Amazon Cognito authentication.
- The web application’s front end is hosted via Amplify.
- A WebSocket API, managed by API Gateway, facilitates user requests. These requests are authenticated through AWS Identity and Access Management (IAM).
- Integration with Amazon Bedrock includes the following steps:
- A Lambda function employs the LangChain library to connect to the Amazon Bedrock endpoint using a context-rich query.
- The text-to-text foundational model crafts a contextually appropriate ad based on the given context and settings.
- The text-to-image foundational model creates a tailored image, influenced by the source image, chosen style, and location.
- The user receives the response through an HTTPS request via the integrated API Gateway WebSocket API.
Document and image processing workflow
The following is the procedure for processing documents and images:
- The user uploads assets via the specified UI.
- The Amplify API transfers the documents to an S3 bucket.
- After the asset is transferred to Amazon S3, one of the following actions takes place:
- If it’s a document, a Lambda function uses Amazon Textract to process and extract text for ad generation.
- If it’s an image, the Lambda function converts it to base64 format, suitable for the Stable Diffusion model to create a new image from the source.
- The extracted text or base64 image string is securely saved in DynamoDB.
Benefits
The following list describes the benefits of this solution:
- Efficiency – The use of generative AI significantly accelerates the ad generation process, eliminating the need for manual adjustments.
- Compliance adherence – The solution ensures that generated ads adhere to specific guidance and regulations, such as the FDA’s guidelines for marketing.
- Cost-effective – By automating the creation of tailored ads, companies can significantly reduce costs associated with ad production and revisions.
- Streamlined MLR process – The solution simplifies the MLR process, reducing friction points and ensuring smoother reviews.
- Localized resonance – Generative AI produces ads that resonate with local audiences, ensuring relevance and impact in different regions.
- Standardization – The solution maintains necessary standards and guidelines, ensuring consistency across all generated ads.
- Scalability – The AI-driven approach can handle vast databases of source images and PDFs, making it feasible for large-scale ad generation.
- Reduced manual intervention – The automation reduces the need for human intervention, minimizing errors and ensuring consistency.
You can deploy the infrastructure in this tutorial from your local computer or you can use AWS Cloud9 as your deployment workstation. AWS Cloud9 comes pre-loaded with the AWS CLI, AWS CDK, and Docker. If you opt for AWS Cloud9, create the environment from the AWS Cloud9 console.
Clean up
To avoid unnecessary cost, clean up all the infrastructure created via the AWS CloudFormation console or by running the following command on your workstation:
Additionally, remember to stop any SageMaker endpoints you initiated via the SageMaker console. Remember, deleting an Amazon Kendra index doesn’t remove the original documents from your storage.
Conclusion
Generative AI, epitomized by LLMs, heralds a paradigm shift in how we access and generate information. These models, while powerful, are often limited by the confines of their training data. RAG addresses this challenge, ensuring that the vast knowledge within these models is consistently infused with relevant, current insights.
Our RAG-based demos provide a tangible testament to this. They showcase the seamless synergy between Amazon Kendra, vector embeddings, and LLMs, creating a system where information is not only vast but also accurate and timely. As you dive into these demos, you’ll explore firsthand the transformational potential of merging pre-trained knowledge with the dynamic capabilities of RAG, resulting in outputs that are both trustworthy and tailored to enterprise content.
Although generative AI powered by LLMs opens up a new way of gaining information insights, these insights must be trustworthy and confined to enterprise content using the RAG approach. These RAG-based demos enable you to be equipped with insights that are accurate and up to date. The quality of these insights is dependent on semantic relevance, which is enabled by using Amazon Kendra and vector embeddings.
If you’re ready to further explore and harness the power of generative AI, here are your next steps:
- Engage with our demos – The hands-on experience is invaluable. Explore the functionalities, understand the integrations, and familiarize yourself with the interface.
- Deepen your knowledge – Take advantage of the resources available. AWS offers in-depth documentation, tutorials, and community support to aid in your AI journey.
- Initiate a pilot project – Consider starting with a small-scale implementation of generative AI in your enterprise. This will provide insights into the system’s practicality and adaptability within your specific context.
For more information about generative AI applications on AWS, refer to the following:
Remember, the landscape of AI is constantly evolving. Stay updated, remain curious, and always be ready to adapt and innovate.
About The Authors
Jin Tan Ruan is a Prototyping Developer within the AWS Industries Prototyping and Customer Engineering (PACE) team, specializing in NLP and generative AI. With a background in software development and nine AWS certifications, Jin brings a wealth of experience to assist AWS customers in materializing their AI/ML and generative AI visions using the AWS platform. He holds a master’s degree in Computer Science & Software Engineering from the University of Syracuse. Outside of work, Jin enjoys playing video games and immersing himself in the thrilling world of horror movies.
Aravind Kodandaramaiah is a Senior Prototyping full stack solution builder within the AWS Industries Prototyping and Customer Engineering (PACE) team. He focuses on helping AWS customers turn innovative ideas into solutions with measurable and delightful outcomes. He is passionate about a range of topics, including cloud security, DevOps, and AI/ML, and can be usually found tinkering with these technologies.
Arjun Shakdher is a Developer on the AWS Industries Prototyping (PACE) team who is passionate about blending technology into the fabric of life. Holding a master’s degree from Purdue University, Arjun’s current role revolves around architecting and building cutting-edge prototypes that span an array of domains, presently prominently featuring the realms of AI/ML and IoT. When not immersed in code and digital landscapes, you’ll find Arjun indulging in the world of coffee, exploring the intricate mechanics of horology, or reveling in the artistry of automobiles.
Read More