Amazon senior principal engineer Luu Tran is helping the Alexa team innovate by collaborating closely with scientist colleagues.Read More
How NVIDIA Fuels the AI Revolution With Investments in Game Changers and Market Makers
Great companies thrive on stories. Sid Siddeek, who runs NVIDIA’s venture capital arm, knows this well.
Siddeek still remembers one of his first jobs, schlepping presentation materials from one investor meeting to another, helping the startup’s CEO and management team get the story out while working from a trailer that “shook when the door opened,” he said.
That CEO was Jensen Huang. The startup was NVIDIA.
Siddeek, who has worked as an investor and an entrepreneur, knows how important it is to find the right people to share your company’s story with early on, whether they’re customers or partners, employees or investors.
It’s this very principle that underpins NVIDIA’s multifaceted approach to investing in the next wave of innovation, a strategy also championed by Vishal Bhagwati, who leads NVIDIA’s corporate development efforts.
It’s an effort that’s resulted in more than two dozen investments so far this year, accelerating as the pace of innovation in AI and accelerated computing quickens.
NVIDIA’s Three-Pronged Strategy to Support the AI Ecosystem
There are three ways that NVIDIA invests in the ecosystem, driving the transformation unleashed by accelerated computing. First, through NVIDIA’s corporate investments, overseen by Bhagwati. Second, through NVentures, our venture capital arm, led by Siddeek. And finally, through NVIDIA Inception, our vehicle for supporting startups and connecting them to venture capital.
There couldn’t be a better time to support companies harnessing NVIDIA technologies. AI alone could contribute more than $15 trillion to the global economy by 2030, according to PwC.
And if you’re working in AI and accelerated computing right now, NVIDIA stands ready to help. Developers across every industry in every country are building accelerated computing applications. And they’re just getting going.
The result is a collection of companies that are advancing the story of AI every day. They include Cohere, CoreWeave, Hugging Face, Inflection, Inceptive and many more. And we’re right alongside them.
“Partnering with NVIDIA is a game-changer,” said Ed Mehr, CEO of Machina Labs. “Their unmatched expertise will supercharge our AI and simulation capabilities.”
Corporate Investments: Growing Our Ecosystem
NVIDIA’s corporate investments arm focuses on strategic collaborations. These partnerships stimulate joint innovation, enhance the NVIDIA platform and expand the ecosystem. Since the beginning of 2023, announcements have been made about 14 investments.
These target companies include Ayar Labs, specializing in chip-to-chip optical connectivity, and Hugging Face, a hub for advanced AI models.
The portfolio also includes next-generation enterprise solutions. Databricks offers an industry-leading data platform for machine learning, while Cohere provides enterprise automation through AI. Other notable companies are Recursion, Kore.ai and Utilidata, each contributing unique solutions in drug discovery, conversational AI and smart electricity grids, respectively.
Consumer services are another investment focus. Inflection is crafting a personal AI for creative expression, while Runway serves as a platform for art and creativity through generative AI.
The investment strategy extends to autonomous machines. Ready Robotics is developing an operating system for industrial robotics, and Skydio builds autonomous drones.
NVIDIA’s most recent investments are in cloud service providers like CoreWeave. These platforms cater to a diverse clientele, from startups to Fortune 500 companies seeking to build next-generation AI services.
NVentures: Investing Alongside Entrepreneurs
Through NVentures, we support innovators who are deeply relevant to NVIDIA. We aim to generate strong financial returns and expand the ecosystem by funding companies that use our platforms across a wide range of industries.
To date, NVentures has made 19 investments in companies in healthcare, manufacturing and other key verticals. Some examples of our portfolio companies include:
- Genesis Therapeutics, Inceptive, Terray, Charm, Evozyne, Generate, Superluminal: revolutionizing drug discovery
- Machina Labs, Seurat Technologies: disrupting industrial processes to improve manufacturing
- PassiveLogic: automating building systems with AI
- MindsDB: for developers that need to connect enterprise data to AI
- Moon Surgical: improving laparoscopic surgery with AI
- Twelve Labs: developing multimodal foundation models for video understanding
- Flywheel: accelerating medical imaging data development
- Luma AI: developers of visual and multimodal models
- Outrider: automating logistics hub operation
- Synthesia: AI Video for the enterprise
- Replicate: developer platform for open-source and custom models
All these companies are building on work being done inside and outside NVIDIA.
“NVentures has a network, not just within NVIDIA, but throughout the industry, to make sure we have access to the best technology and the best people to build all the different modules that have to come together to define the distribution and supply chain of the future,” said Andrew Smith, CEO of Outrider.
NVIDIA Inception: Supporting Startups and Connecting Them to Investors
In addition, we’re continuing to support startups with NVIDIA Inception. Launched in 2016, this free global program offers technology and marketing support to over 17,000 startups across multiple industries and over 125 countries.
And, as part of Inception, we’re partnering with venture capitalists through our VC Alliance, a program that offers benefits to our valued network of venture capital firms, including connecting startups with potential investors.
Partnering With Innovators in Every Industry
Whatever our relationship, whether as a partner or investor, we can offer companies unique forms of support.
NVIDIA has the technology. NVIDIA has the richest set of libraries and the deepest understanding of the frameworks needed to optimize training and inference pipelines.
We have the go-to-market skills. NVIDIA has tremendous field sales, solution architect and developer relations organizations with a long track record of working with the most innovative startups and the largest companies in the world.
We know how to grow. We have people throughout our organization who are recognized leaders in their respective fields and can offer expert advice to companies of all sizes and industries.
“Partnering with NVIDIA was an easy choice,” said Victor Riparbelli, cofounder and CEO of Synthesia. “We use their hardware, benefit from their AI expertise and get valuable insights, allowing us to build better products faster.”
Accelerating the Greatest Breakthroughs of Our Time
In turn, these investments augment our R&D in the software, systems and semiconductors undergirding this ecosystem.
With NVIDIA’s technologies poised to accelerate the work of researchers and scientists, entrepreneurs, startups and Fortune 500 companies, finding ways to support companies that rely on our technologies— with engineering resources, marketing support and capital — is more vital than ever.
NeurIPS 2023 highlights breadth of Microsoft’s machine learning innovation

Microsoft is proud to sponsor the 37th Conference on Neural Information Processing Systems (NeurIPS 2023). This interdisciplinary forum brings together experts in machine learning, neuroscience, statistics, optimization, computer vision, natural language processing, life sciences, natural sciences, social sciences, and other adjacent fields. We are pleased to share that Microsoft has over 100 accepted papers and is offering 18 workshops at NeurIPS 2023.
This year’s conference includes three papers from Microsoft that were chosen for oral presentations, which feature groundbreaking concepts, methods, or applications, addressing pressing issues in the field. Additionally, our spotlights posters, also highlighted below, have been carefully curated by conference organizers, exhibiting novelty, technical rigor, and the potential to significantly impact the landscape of machine learning. This blog post celebrates those achievements.
Oral Presentations
Bridging Discrete and Backpropagation: Straight-Through and Beyond
Gradient computations are pivotal in deep learning’s success, yet they predominantly depend on backpropagation, a technique limited to continuous variables. The paper Bridging Discrete and Backpropagation: Straight-Through and Beyond, tackles this limitation. It introduces ReinMax, extending backpropagation’s capability to estimate gradients for models incorporating discrete variable sampling. Within extensive experiments of this study, ReinMax demonstrates consistent and significant performance gain over the state of the art. More than just a practical solution, the paper sheds light on existing deep learning practices. It elucidates that the ‘Straight-Through’ method, once considered merely a heuristic trick, is actually a viable first-order approximation for the general multinomial case. Correspondingly, ReinMax achieves second-order accuracy in this context without the complexities of second-order derivatives, thus having negligible computation overheads.
MICROSOFT RESEARCH PODCAST
Intern Insights: Dr. Madeleine Daepp with Jennifer Scurrell and Alejandro Cuevas
In this episode, PhD students Jennifer Scurrell and Alejandro Cuevas talk to Senior Researcher Dr. Madeleine Daepp. They discuss the internship culture at Microsoft Research, from opportunities to connect with researchers to the teamwork they say helped make it possible for them to succeed, and the impact they hope to have with their work.
The MineRL BASALT Competition on Learning from Human Feedback
The growth of deep learning research, including its incorporation into commercial products, has created a new challenge: How can we build AI systems that solve tasks when a crisp, well-defined specification is lacking? To encourage research on this important class of techniques, researchers from Microsoft led The MineRL BASALT Competition on Learning from Human Feedback (opens in new tab), an update to a contest first launched in 2021 (opens in new tab) by researchers at the University of California-Berkeley and elsewhere. The challenge of this competition was to complete fuzzy tasks from English language descriptions alone, with emphasis on encouraging different ways of learning from human feedback as an alternative to a traditional reward signal.
The researchers designed a suite of four tasks in Minecraft for which writing hardcoded reward functions would be difficult. These tasks are defined by natural language: for example, “create a waterfall and take a scenic picture of it”, with additional clarifying details. Participants must train a separate agent for each task. Agents are then evaluated by humans who have read the task description.
The competition aimed to encourage development of AI systems that do what their designers intended, even when the intent cannot be easily formalized. Besides allowing AI to solve more tasks, this can also enable more effective regulation of AI systems, as well as making progress on value alignment problems, in which the specified objectives of an AI agent differ from those of its users.
Related
DecodingTrust: A Comprehensive Assessment of Trustworthiness in GPT Models
This comprehensive evaluation platform aims to answer the question: How trustworthy are generative pre-trained transformer (GPT) models? In DecodingTrust: A Comprehensive Assessment of Trustworthiness in GPT Models, researchers focus specifically on GPT-4, GPT-3.5, and a series of open LLMs. They consider diverse perspectives, including toxicity, stereotype bias, adversarial robustness, out-of-distribution robustness, robustness on adversarial demonstrations, privacy, machine ethics, and fairness.
The researchers’ evaluations identified previously unpublished vulnerabilities relating to trustworthiness. The team worked with Microsoft product groups to confirm that the potential vulnerabilities identified do not impact current customer-facing services. This is in part true because finished AI applications apply a range of mitigation approaches to address potential harms that may occur at the model level of the technology. They also shared their findings with GPT’s developer, OpenAI, which has noted the potential vulnerabilities in the system cards for relevant models.
This research aims to encourage others in the research community to utilize and build upon this work, potentially pre-empting adversaries who would exploit vulnerabilities to cause harm. To facilitate collaboration, the benchmark code is very extensible and easy to use: a single command is sufficient to run the complete evaluation on a new model.
Spotlight Posters
Differentially Private Approximate Near Neighbor Counting in High Dimensions
Differential privacy (DP) is a widely used tool for preserving the privacy of sensitive personal information. It allows a data structure to provide approximate answers to queries about the data it holds, while ensuring that the removal or addition of a single database entry does not significantly affect the outcome of any analysis.
Range counting (counting the number of data points falling into a given query ball) under differential privacy has been studied extensively. However, current algorithms for this problem come with challenges. One class of algorithms suffers from an additive error that is a fixed polynomial in the number of points. Another class of algorithms allows for polylogarithmic additive error, but the error grows exponentially in the dimension. To achieve the latter, the problem is relaxed to allow a “fuzzy” definition of the range boundary, e.g., a count of the points in a ball of radius r might also include points in a ball of radius cr for some c > 1.
In Differentially Private Approximate Near Neighbor Counting in High Dimensions, researchers present an efficient algorithm that offers a sweet spot between these two classes. The algorithm has an additive error that is an arbitrary small power of the data set size, depending on how fuzzy the range boundary is, as well as a small (1 + o(1)) multiplicative error. Crucially, the amount of noise added has no dependence on the dimension. This new algorithm introduces a variant of Locality-Sensitive Hashing, utilizing it in a novel manner.
Exposing Attention Glitches with Flip-Flop Language Modeling
Why do large language models sometimes output factual inaccuracies and exhibit erroneous reasoning? The brittleness of these models, particularly when executing long chains of reasoning, seems to be an inevitable price to pay for their advanced capabilities of coherently synthesizing knowledge, pragmatics, and abstract thought.
To help make sense of this fundamentally unsolved problem, Exposing Attention Glitches with Flip-Flop Language Modeling identifies and analyzes the phenomenon of attention glitches, in which the Transformer architecture’s inductive biases intermittently fail to capture robust reasoning. To isolate the issue, the researchers introduce flip-flop language modeling (FFLM), a parametric family of synthetic benchmarks designed to probe the extrapolative behavior of neural language models. This simple generative task requires a model to copy binary symbols over long-range dependencies, ignoring the tokens in between. This research shows how Transformer FFLMs suffer from a long tail of sporadic reasoning errors, some of which can be eliminated using various regularization techniques. The preliminary mechanistic analyses show why the remaining errors may be very difficult to diagnose and resolve. The researchers hypothesize that attention glitches account for some of the closed-domain errors occurring in natural LLMs.
In-Context Learning Unlocked for Diffusion Models
An emergent behavior of large language models (LLMs) is the ability to learn from context, or in-context learning. With a properly designed prompt structure and in-context learning, LLMs can combine the pre-training of multiple language tasks and generalize well to previously unseen tasks. While in-context learning has been extensively studied in natural language processing (NLP), its applications in the field of computer vision are still limited.
In-Context Learning Unlocked for Diffusion Models presents Prompt Diffusion, a framework for enabling in-context learning in diffusion-based generative models. Given a pair of task-specific example images and text guidance, this model understands the underlying task and performs the same task on a new query image following the text guidance. To achieve this, the researchers propose a vision-language prompt that can model a wide range of vision-language tasks, and a diffusion model that takes it as input. The diffusion model is trained jointly over six different tasks using these prompts. The resulting Prompt Diffusion model is the first diffusion-based vision-language foundation model capable of in-context learning. It demonstrates high-quality in-context generation on the trained tasks and generalizes to new, unseen vision tasks with their respective prompts. This model also shows compelling text-guided image editing results.
Optimizing Prompts for Text-to-Image Generation
Generative foundation models can be prompted to follow user instructions, including language models and text-to-image models. Well-designed prompts can guide text-to-image models to generate amazing images. However, the performant prompts are often model-specific and misaligned with user input. Instead of laborious human engineering, Optimizing Prompts for Text-to-Image Generation proposes prompt adaptation, a general framework that automatically adapts original user input to model-preferred prompts.
The researchers use reinforcement learning to explore better prompts with a language model. They define a reward function that encourages the policy network (i.e., language model) to generate more aesthetically pleasing images while preserving the original user intentions. Experimental results on Stable Diffusion show that this method outperforms manual prompt engineering in terms of both automatic metrics and human preference ratings. Reinforcement learning further boosts performance, especially on out-of-domain prompts.
Pareto Frontiers in Neural Feature Learning: Data, Compute, Width, and Luck
Algorithm design in deep learning can appear to be more like “hacking” than an engineering practice. There are numerous architectural choices and training heuristics, which can often modulate model performance and resource costs in unpredictable and entangled ways. As a result, when training large-scale neural networks (such as state-of-the-art language models), algorithmic decisions and resource allocations are foremost empirically-driven, involving the measurement and extrapolation of scaling laws. A precise mathematical understanding of this process is elusive, and cannot be explained by statistics or optimization in isolation.
In Pareto Frontiers in Neural Feature Learning: Data, Compute, Width, and Luck, researchers from Microsoft, Harvard, and the University of Pennsylvania explore these algorithmic intricacies and tradeoffs through the lens of a single synthetic task: the finite-sample sparse parity learning problem. In this setting, the above complications are not only evident, but also provable: intuitively, due to the task’s computational hardness, a neural network needs a sufficient combination of resources (“data × model size × training time × luck”) to succeed. This research shows that standard algorithmic choices in deep learning give rise to a Pareto frontier, in which successful learning is “bought” with interchangeable combinations of these resources. They show that algorithmic improvements on this toy problem can transfer to the real world, improving the data-efficiency of neural networks on small tabular datasets.
PDE-Refiner: Achieving Accurate Long Rollouts with Neural PDE Solvers
Time-dependent partial differential equations (PDEs) are ubiquitous in science and engineering. The high computational cost of traditional solution techniques has spurred increasing interest in deep neural network based PDE surrogates. The practical utility of such neural PDE solvers depends on their ability to provide accurate, stable predictions over long time horizons, which is a notoriously hard problem.
PDE-Refiner: Achieving Accurate Long Rollouts with Neural PDE Solvers presents a large-scale analysis of common temporal rollout strategies, identifying the neglect of non-dominant spatial frequency information, often associated with high frequencies in PDE solutions, as the primary pitfall limiting stable, accurate rollout performance. Motivated by recent advances in diffusion models, the researchers developed PDE-Refiner, a novel model class that enables more accurate modeling of all frequency components via a multistep refinement process. They validate PDE-Refiner on challenging benchmarks of complex fluid dynamics, demonstrating stable and accurate rollouts that consistently outperform state-of-the-art models, including neural, numerical, and hybrid neural-numerical architectures. They also demonstrate that PDE-Refiner greatly enhances data efficiency, since the denoising objective implicitly induces a novel form of spectral data augmentation. Finally, PDE-Refiner’s connection to diffusion models enables an accurate and efficient assessment of the model’s predictive uncertainty, allowing researchers to estimate when the surrogate becomes inaccurate.
Should I Stop or Should I Go: Early Stopping with Heterogeneous Populations
Randomized experiments are the gold-standard method of determining causal effects, whether in clinical trials to evaluate medical treatments or in A/B tests to evaluate online product offerings. But randomized experiments often need to be stopped prematurely when the treatment or test causes an unintended harmful effect. Existing methods that determine when to stop an experiment early are typically applied to the data in aggregate and do not account for treatment effect heterogeneity.
Should I Stop or Should I Go: Early Stopping with Heterogeneous Populations examines the early stopping of experiments for harm on heterogeneous populations. The paper shows that current methods often fail to stop experiments when the treatment harms a minority group of participants. The researchers use causal machine learning to develop Causal Latent Analysis for Stopping Heterogeneously (CLASH), the first broadly-applicable method for heterogeneous early stopping. They demonstrate CLASH’s performance on simulated and real data and show that it yields effective early stopping for both clinical trials and A/B tests.
Survival Instinct in Offline Reinforcement Learning
In offline reinforcement learning (RL), an agent optimizes its performance given an offline dataset. Survival Instinct in Offline Reinforcement Learning presents a novel observation: on many benchmark datasets, offline RL can produce well-performing and safe policies even when trained with “wrong” reward labels, such as those that are zero everywhere or are negatives of the true rewards. This phenomenon cannot be easily explained by offline RL’s return maximization objective. Moreover, it gives offline RL a degree of robustness that is uncharacteristic of its online RL counterparts, which are known to be sensitive to reward design.
This research demonstrates that this surprising robustness property is attributable to an interplay between the notion of pessimism in offline RL algorithms and a certain bias implicit in common data collection practices. This work shows that this pessimism endows the agent with a “survival instinct”, i.e., an incentive to stay within the data support in the long term, while the limited and biased data coverage further constrains the set of survival policies. The researchers argue that the survival instinct should be taken into account when interpreting results from existing offline RL benchmarks and when creating future ones.
Timewarp: Transferable Acceleration of Molecular Dynamics by Learning Time-Coarsened Dynamics
Molecular dynamics (MD) is a well-established technique for simulating physical systems at the atomic level. When performed accurately, it provides unrivalled insight into the detailed mechanics of molecular motion, without the need for wet lab experiments. MD is often used to compute equilibrium properties, which requires sampling from an equilibrium distribution such as the Boltzmann distribution (opens in new tab). However, many important processes, such as binding and folding, occur over timescales of milliseconds or beyond, and cannot be efficiently sampled with conventional MD. Furthermore, new MD simulations need to be performed from scratch for each molecular system studied.
Timewarp: Transferable Acceleration of Molecular Dynamics by Learning Time-Coarsened Dynamics presents an enhanced sampling method which uses a normalizing flow as a proposal distribution in a Markov chain Monte Carlo method targeting the Boltzmann distribution. The flow is trained offline on MD trajectories and learns to make large steps in time, simulating the molecular dynamics of 10^5−10^6fs. Crucially, Timewarp is transferable between molecular systems: the researchers show that, once trained, Timewarp generalizes to unseen small peptides (2-4 amino acids), exploring their metastable states and providing wall-clock acceleration when sampling compared to standard MD. This new method constitutes an important step towards developing general, transferable algorithms for accelerating MD.
The post NeurIPS 2023 highlights breadth of Microsoft’s machine learning innovation appeared first on Microsoft Research.
Google at NeurIPS 2023
This week the 37th annual Conference on Neural Information Processing Systems (NeurIPS 2023), the biggest machine learning conference of the year, kicks off in New Orleans, LA. Google is proud to be a Diamond Level sponsor of NeurIPS this year and will have a strong presence with >170 accepted papers, two keynote talks, and additional contributions to the broader research community through organizational support and involvement in >20 workshops and tutorials. Google is also proud to be a Platinum Sponsor for both the Women in Machine Learning and LatinX in AI workshops. We look forward to sharing some of our extensive ML research and expanding our partnership with the broader ML research community.
Attending for NeurIPS 2023 in person? Come visit the Google Research booth to learn more about the exciting work we’re doing to solve some of the field’s most interesting challenges. Visit the @GoogleAI X (Twitter) account to find out about Google booth activities (e.g., demos and Q&A sessions).
You can learn more about our latest cutting edge work being presented at the conference in the list below (Google affiliations highlighted in bold). And see Google DeepMind’s blog to learn more about their participation at NeurIPS 2023.
Board & Organizing Committee
NeurIPS Board: Corinna Cortes
Advisory Board: John C. Platt
Senior Area Chair: Inderjit S. Dhillon
Creative AI Chair: Isabelle Guyon
Program Chair: Amir Globerson
Datasets and Benchmarks Chair: Remi Denton
Google Research Booth Demo/Q&A Schedule
This schedule is subject to change. Please visit the Google booth (#215) for more information.
What You See is What You Read? Improving Text-Image Alignment Evaluation
Presenter: Yonatan Bitton
Monday, Dec 11 | 12:15PM – 1:45PM
Talk like a Graph: Encoding Graphs for Large Language Models
Presenters: Bahar Fatemi, Jonathan Halcrow, Bryan Perozzi
Monday, Dec 11 | 4:00PM – 4:45PM
VisIT-Bench: A Benchmark for Vision-Language Instruction Following Inspired by Real-World Use
Presenter: Yonatan Bitton
Monday, Dec 11 | 4:00PM – 4:45PM
MLCommons Croissant
Presenters: Omar Benjelloun, Meg Risdal, Lora Aroyo
Tuesday, Dec 12 | 9:15AM – 10:00AM
DaTaSeg: Taming a Universal Multi-Dataset Multi-Task Segmentation Model
Presenter: Xiuye Gu
Tuesday, Dec 12 | 12:45PM – 2:15PM
Embedding Large Graphs
Presenters: Bryan Perozzi, Anton Tsitsulin
Tuesday, Dec 12 | 3:20PM – 3:40PM
Correlated Noise Provably Beats Independent Noise for Differentially Private Learning
Presenter: Krishna Pillutla
Tuesday, Dec 12 | 3:20PM – 3:40PM
Med-PaLM
Presenter: Tao Tu
Tuesday, Dec 12 | 4:45PM – 5:15PM
StyleDrop: Text-to-Image Generation in Any Style
Presenters: Kihyuk Sohn, Lu Jiang, Irfan Essa
Tuesday, Dec 12 | 4:45PM – 5:15PM
DICES Dataset: Diversity in Conversational AI Evaluation for Safety
Presenters: Lora Aroyo, Alicia Parrish, Vinodkumar Prabhakaran
Wednesday, Dec 13 | 9:15AM – 10:00AM
Resonator: Scalable Game-Based Evaluation of Large Models
Presenters: Erin Drake Kajioka, Michal Todorovic
Wednesday, Dec 13 | 12:45PM – 2:15PM
Adversarial Nibbler
Presenter: Lora Aroyo
Wednesday, Dec 13 | 12:45PM – 2:15PM
Towards Generalist Biomedical AI
Presenter: Tao Tu
Wednesday, Dec 13 | 3:15PM – 3:30PM
Conditional Adaptors
Presenter: Junwen Bai
Wednesday, Dec 13 | 3:15PM – 3:30PM
Patient Assistance with Multimodal RAG
Presenters: Ryan Knuffman, Milica Cvetkovic
Wednesday, Dec 13 | 4:15PM – 5:00PM
How Hessian Structure Explains Mysteries in Sharpness Regularization
Presenter: Hossein Mobahi
Wednesday, Dec 13 | 4:15PM – 5:00PM
Keynote Speakers
The Many Faces of Responsible AI
Speaker: Lora Aroyo
Sketching: Core Tools, Learning-Augmentation, and Adaptive Robustness
Speaker: Jelani Nelson
Affinity Workshops
Women in ML
Google Sponsored – Platinum
LatinX in AI
Google Sponsored – Platinum
New in ML
Organizer: Isabelle Guyon
Workshops
AI for Accelerated Materials Design (AI4Mat-2023)
Fireside Chat: Gowoon Cheon
Associative Memory & Hopfield Networks in 2023
Panelist: Blaise Agüera y Arcas
Information-Theoretic Principles in Cognitive Systems (InfoCog)
Speaker: Alexander Alemi
Machine Learning and the Physical Sciences
Speaker: Alexander Alemi
UniReps: Unifying Representations in Neural Models
Organizer: Mathilde Caron
Robustness of Zero/Few-shot Learning in Foundation Models (R0-FoMo)
Speaker: Partha Talukdar
Organizer: Ananth Balashankar, Yao Qin, Ahmad Beirami
Workshop on Diffusion Models
Speaker: Tali Dekel
Algorithmic Fairness through the Lens of Time
Roundtable Lead: Stephen Pfohl
Organizer: Golnoosh Farnadi
Backdoors in Deep Learning: The Good, the Bad, and the Ugly
Organizer: Eugene Bagdasaryan
OPT 2023: Optimization for Machine Learning
Organizer: Cristóbal Guzmán
Machine Learning for Creativity and Design
Speaker: Aleksander Holynski, Alexander Mordvintsev
Robot Learning Workshop: Pretraining, Fine-Tuning, and Generalization with Large Scale Models
Speaker: Matt Barnes
Machine Learning for Audio
Organizer: Shrikanth Narayanan
Federated Learning in the Age of Foundation Models (FL@FM-NeurIPS’23)
Speaker: Cho-Jui Hsieh, Zheng Xu
Socially Responsible Language Modelling Research (SoLaR)
Panelist: Vinodkumar Prabhakaran
I Can’t Believe It’s Not Better (ICBINB): Failure Modes in the Age of Foundation Models
Advisory Board: Javier Antorán
Machine Learning for Systems
Organizer: Yawen Wang
Competition Committee: Bryan Perozzi, Sami Abu-el-haija
Steering Committee: Milad Hashemi
Self-Supervised Learning: Theory and Practice
Organizer: Mathilde Caron
Competitions
NeurIPS 2023 Machine Unlearning Competition
Organizer: Isabelle Guyon, Peter Kairouz
Lux AI Challenge Season 2 NeurIPS Edition
Organizer: Bovard Doerschuk-Tiberi, Addison Howard
Tutorials
Data-Centric AI for Reliable and Responsible AI: From Theory to Practice
Isabelle Guyon, Nabeel Seedat, Mihaela va der Schaar
Creative AI Track
Creative AI Performances 1 & 2
Speaker: Erin Drake Kajioka, Yonatan Bitton
Organizer: Isabelle Guyon
Performance 1: Mon, Dec 11 | 6:30PM – 8:30PM, Lobby Stage
Performance 2: Thu, Dec 14 | 7:00PM – 9:00PM, Lobby Stage
Creative AI Sessions 1 – 3
Speaker: Erin Drake Kajioka, Yonatan Bitton
Organizer: Isabelle Guyon
Session 1: Tue, Dec 12 | 3:05PM – 3:40PM, Hall D2
Session 2: Wed, Dec 13 | 10:45AM – 2:15PM, Hall D2
Session 3: Thu, Dec 14 | 10:45 AM – 2:15PM, Hall D2
Creative AI Videos
Organizer: Isabelle Guyon
Expo Talks
Graph Learning Meets Artificial Intelligence
Speaker: Bryan Perozzi
Resonator: Music Space
Speakers: Erin Drake Kajioka, Michal Todorovic
Empirical Rigor in ML as a Massively Parallelizable Challenge
Speaker: Megan Risdal (Kaggle)
Oral Talks
Ordering-based Conditions for Global Convergence of Policy Gradient Methods
Jincheng Mei, Bo Dai, Alekh Agarwal, Mohammad Ghavamzadeh*, Csaba Szepesvari, Dale Schuurmans
Private Everlasting Prediction
Moni Naor, Kobbi Nissim, Uri Stemmer, Chao Yan
User-Level Differential Privacy With Few Examples Per User
Badih Ghazi, Pritish Kamath, Ravi Kumar, Pasin Manurangsi, Raghu Meka, Chiyuan Zhang
DataComp: In Search of the Next Generation of Multimodal Datasets
Samir Yitzhak Gadre, Gabriel Ilharco, Alex Fang, Jonathan Hayase, Georgios Smyrnis, Thao Nguyen, Ryan Marten, Mitchell Wortsman, Dhruba Ghosh, Jieyu Zhang, Eyal Orgad, Rahim Entezari, Giannis Daras, Sarah Pratt, Vivek Ramanujan, Yonatan Bitton, Kalyani Marathe, Stephen Mussmann, Richard Vencu, Mehdi Cherti, Ranjay Krishna, Pang Wei Koh, Olga Saukh, Alexander Ratner, Shuran Song, Hannaneh Hajishirzi, Ali Farhadi, Romain Beaumont, Sewoong Oh, Alex Dimakis, Jenia Jitsev, Yair Carmon, Vaishaal Shankar, Ludwig Schmidt
Optimal Learners for Realizable Regression: PAC Learning and Online Learning
Idan Attias, Steve Hanneke, Alkis Kalavasis, Amin Karbasi, Grigoris Velegkas
The Surprising Effectiveness of Diffusion Models for Optical Flow and Monocular Depth Estimation
Saurabh Saxena, Charles Herrmann, Junhwa Hur, Abhishek Kar, Mohammad Norouzi*, Deqing Sun, David J. Fleet
Journal Track
Graph Clustering with Graph Neural Networks
Anton Tsitsulin, John Palowitch, Bryan Perozzi, Emmanuel Müller
Spotlight Papers
Alternating Updates for Efficient Transformers (see blog post)
Cenk Baykal, Dylan Cutler, Nishanth Dikkala, Nikhil Ghosh*, Rina Panigrahy, Xin Wang
Does Localization Inform Editing? Surprising Differences in Causality-Based Localization vs. Knowledge Editing in Language Models
Peter Hase, Mohit Bansal, Been Kim, Asma Ghandeharioun
Is Learning in Games Good for the Learners?
William Brown, Jon Schneider, Kiran Vodrahalli
Participatory Personalization in Classification
Hailey Joren, Chirag Nagpal, Katherine Heller, Berk Ustun
Tight Risk Bounds for Gradient Descent on Separable Data
Matan Schliserman, Tomer Koren
Counterfactual Memorization in Neural Language Models
Chiyuan Zhang, Daphne Ippolito, Katherine Lee, Matthew Jagielski, Florian Tramèr, Nicholas Carlini
Debias Coarsely, Sample Conditionally: Statistical Downscaling through Optimal Transport and Probabilistic Diffusion Models
Zhong Yi Wan, Ricardo Baptista, Anudhyan Boral, Yi-Fan Chen, John Anderson, Fei Sha, Leonardo Zepeda-Nunez
Faster Margin Maximization Rates for Generic Optimization Methods
Guanghui Wang, Zihao Hu, Vidya Muthukumar, Jacob Abernethy
From Pixels to UI Actions: Learning to Follow Instructions via Graphical User Interfaces
Peter Shaw, Mandar Joshi, James Cohan, Jonathan Berant, Panupong Pasupat, Hexiang Hu, Urvashi Khandelwal, Kenton Lee, Kristina N Toutanova
PAC Learning Linear Thresholds from Label Proportions
Anand Brahmbhatt, Rishi Saket, Aravindan Raghuveer
SPAE: Semantic Pyramid AutoEncoder for Multimodal Generation with Frozen LLMs
Lijun Yu*, Yong Cheng, Zhiruo Wang, Vivek Kumar, Wolfgang Macherey, Yanping Huang, David Ross, Irfan Essa, Yonatan Bisk, Ming-Hsuan Yang, Kevin Murphy, Alexander Hauptmann, Lu Jiang
Adaptive Data Analysis in a Balanced Adversarial Model
Kobbi Nissim, Uri Stemmer, Eliad Tsfadia
Lexinvariant Language Models
Qian Huang, Eric Zelikman, Sarah Chen, Yuhuai Wu, Gregory Valiant, Percy Liang
On Quantum Backpropagation, Information Reuse, and Cheating Measurement Collapse
Amira Abbas, Robbie King, Hsin-Yuan Huang, William J. Huggins, Ramis Movassagh, Dar Gilboa, Jarrod McClean
Private Estimation Algorithms for Stochastic Block Models and Mixture Models
Hongjie Chen, Vincent Cohen-Addad, Tommaso d’Orsi, Alessandro Epasto, Jacob Imola, David Steurer, Stefan Tiegel
Provably Fast Finite Particle Variants of SVGD via Virtual Particle Stochastic Approximation
Aniket Das, Dheeraj Nagaraj
Private (Stochastic) Non-Convex Optimization Revisited: Second-Order Stationary Points and Excess Risks
Arun Ganesh, Daogao Liu*, Sewoong Oh, Abhradeep Guha Thakurta
Uncovering the Hidden Dynamics of Video Self-supervised Learning under Distribution Shifts
Pritam Sarkar, Ahmad Beirami, Ali Etemad
AIMS: All-Inclusive Multi-Level Segmentation for Anything
Lu Qi, Jason Kuen, Weidong Guo, Jiuxiang Gu, Zhe Lin, Bo Du, Yu Xu, Ming-Hsuan Yang
DreamHuman: Animatable 3D Avatars from Text
Nikos Kolotouros, Thiemo Alldieck, Andrei Zanfir, Eduard Gabriel Bazavan, Mihai Fieraru, Cristian Sminchisescu
Follow-ups Also Matter: Improving Contextual Bandits via Post-serving Contexts
Chaoqi Wang, Ziyu Ye, Zhe Feng, Ashwinkumar Badanidiyuru, Haifeng Xu
Learning List-Level Domain-Invariant Representations for Ranking
Ruicheng Xian*, Honglei Zhuang, Zhen Qin, Hamed Zamani*, Jing Lu, Ji Ma, Kai Hui, Han Zhao, Xuanhui Wang, Michael Bendersky
Optimal Guarantees for Algorithmic Reproducibility and Gradient Complexity in Convex Optimization
Liang Zhang, Junchi Yang, Amin Karbasi, Niao He
Unified Embedding: Battle-Tested Feature Representations for Web-Scale ML Systems
Benjamin Coleman, Wang-Cheng Kang, Matthew Fahrbach, Ruoxi Wang, Lichan Hong, Ed Chi, Derek Cheng
Proximity-Informed Calibration for Deep Neural Networks
Miao Xiong, Ailin Deng, Pang Wei Koh, Jiaying Wu, Shen Li, Jianqing Xu, Bryan Hooi
Papers
Anonymous Learning via Look-Alike Clustering: A Precise Analysis of Model Generalization
Adel Javanmard, Vahab Mirrokni
Better Private Linear Regression Through Better Private Feature Selection
Travis Dick, Jennifer Gillenwater*, Matthew Joseph
Binarized Neural Machine Translation
Yichi Zhang, Ankush Garg, Yuan Cao, Łukasz Lew, Behrooz Ghorbani*, Zhiru Zhang, Orhan Firat
BoardgameQA: A Dataset for Natural Language Reasoning with Contradictory Information
Mehran Kazemi, Quan Yuan, Deepti Bhatia, Najoung Kim, Xin Xu, Vaiva Imbrasaite, Deepak Ramachandran
Boosting with Tempered Exponential Measures
Richard Nock, Ehsan Amid, Manfred Warmuth
Concept Algebra for (Score-Based) Text-Controlled Generative Models
Zihao Wang, Lin Gui, Jeffrey Negrea, Victor Veitch
Deep Contract Design via Discontinuous Networks
Tonghan Wang, Paul Dütting, Dmitry Ivanov, Inbal Talgam-Cohen, David C. Parkes
Diffusion-SS3D: Diffusion Model for Semi-supervised 3D Object Detection
Cheng-Ju Ho, Chen-Hsuan Tai, Yen-Yu Lin, Ming-Hsuan Yang, Yi-Hsuan Tsai
Eliciting User Preferences for Personalized Multi-Objective Decision Making through Comparative Feedback
Han Shao, Lee Cohen, Avrim Blum, Yishay Mansour, Aadirupa Saha, Matthew Walter
Gradient Descent with Linearly Correlated Noise: Theory and Applications to Differential Privacy
Anastasia Koloskova*, Ryan McKenna, Zachary Charles, J Keith Rush, Hugh Brendan McMahan
Hardness of Low Rank Approximation of Entrywise Transformed Matrix Products
Tamas Sarlos, Xingyou Song, David P. Woodruff, Qiuyi (Richard) Zhang
Module-wise Adaptive Distillation for Multimodality Foundation Models
Chen Liang, Jiahui Yu, Ming-Hsuan Yang, Matthew Brown, Yin Cui, Tuo Zhao, Boqing Gong, Tianyi Zhou
Multi-Swap k-Means++
Lorenzo Beretta, Vincent Cohen-Addad, Silvio Lattanzi, Nikos Parotsidis
OpenMask3D: Open-Vocabulary 3D Instance Segmentation
Ayça Takmaz, Elisabetta Fedele, Robert Sumner, Marc Pollefeys, Federico Tombari, Francis Engelmann
Order Matters in the Presence of Dataset Imbalance for Multilingual Learning
Dami Choi*, Derrick Xin, Hamid Dadkhahi, Justin Gilmer, Ankush Garg, Orhan Firat, Chih-Kuan Yeh, Andrew M. Dai, Behrooz Ghorbani
PopSign ASL v1.0: An Isolated American Sign Language Dataset Collected via Smartphones
Thad Starner, Sean Forbes, Matthew So, David Martin, Rohit Sridhar, Gururaj Deshpande, Sam Sepah, Sahir Shahryar, Khushi Bhardwaj, Tyler Kwok, Daksh Sehgal, Saad Hassan, Bill Neubauer, Sofia Vempala, Alec Tan, Jocelyn Heath, Unnathi Kumar, Priyanka Mosur, Tavenner Hall, Rajandeep Singh, Christopher Cui, Glenn Cameron, Sohier Dane, Garrett Tanzer
Semi-Implicit Denoising Diffusion Models (SIDDMs)
Yanwu Xu*, Mingming Gong, Shaoan Xie, Wei Wei, Matthias Grundmann, Kayhan Batmanghelich, Tingbo Hou
State2Explanation: Concept-Based Explanations to Benefit Agent Learning and User Understanding
Devleena Das, Sonia Chernova, Been Kim
StoryBench: A Multifaceted Benchmark for Continuous Story Visualization
Emanuele Bugliarello*, Hernan Moraldo, Ruben Villegas, Mohammad Babaeizadeh, Mohammad Taghi Saffar, Han Zhang, Dumitru Erhan, Vittorio Ferrari, Pieter-Jan Kindermans, Paul Voigtlaender
Subject-driven Text-to-Image Generation via Apprenticeship Learning
Wenhu Chen, Hexiang Hu, Yandong Li, Nataniel Ruiz, Xuhui Jia, Ming-Wei Chang, William W. Cohen
TpuGraphs: A Performance Prediction Dataset on Large Tensor Computational Graphs
Phitchaya Mangpo Phothilimthana, Sami Abu-El-Haija, Kaidi Cao*, Bahare Fatemi, Mike Burrows, Charith Mendis*, Bryan Perozzi
Training Chain-of-Thought via Latent-Variable Inference
Du Phan, Matthew D. Hoffman, David Dohan*, Sholto Douglas, Tuan Anh Le, Aaron Parisi, Pavel Sountsov, Charles Sutton, Sharad Vikram, Rif A. Saurous
Unified Lower Bounds for Interactive High-dimensional Estimation under Information Constraints
Jayadev Acharya, Clement L. Canonne, Ziteng Sun, Himanshu Tyagi
What You See is What You Read? Improving Text-Image Alignment Evaluation
Michal Yarom, Yonatan Bitton, Soravit Changpinyo, Roee Aharoni, Jonathan Herzig, Oran Lang, Eran Ofek, Idan Szpektor
When Does Confidence-Based Cascade Deferral Suffice?
Wittawat Jitkrittum, Neha Gupta, Aditya Krishna Menon, Harikrishna Narasimhan, Ankit Singh Rawat, Sanjiv Kumar
Accelerating Molecular Graph Neural Networks via Knowledge Distillation
Filip Ekström Kelvinius, Dimitar Georgiev, Artur Petrov Toshev, Johannes Gasteiger
AVIS: Autonomous Visual Information Seeking with Large Language Model Agent
Ziniu Hu*, Ahmet Iscen, Chen Sun, Kai-Wei Chang, Yizhou Sun, David Ross, Cordelia Schmid, Alireza Fathi
Beyond Invariance: Test-Time Label-Shift Adaptation for Addressing “Spurious” Correlations
Qingyao Sun, Kevin Patrick Murphy, Sayna Ebrahimi, Alexander D’Amour
Collaborative Score Distillation for Consistent Visual Editing
Subin Kim, Kyungmin Lee, June Suk Choi, Jongheon Jeong, Kihyuk Sohn, Jinwoo Shin
CommonScenes: Generating Commonsense 3D Indoor Scenes with Scene Graphs
Guangyao Zhai, Evin Pınar Örnek, Shun-Cheng Wu, Yan Di, Federico Tombari, Nassir Navab, Benjamin Busam
Computational Complexity of Learning Neural Networks: Smoothness and Degeneracy
Amit Daniely, Nathan Srebro, Gal Vardi
A Computationally Efficient Sparsified Online Newton Method
Fnu Devvrit*, Sai Surya Duvvuri, Rohan Anil, Vineet Gupta, Cho-Jui Hsieh, Inderjit S Dhillon
DDF-HO: Hand-Held Object Reconstruction via Conditional Directed Distance Field
Chenyangguang Zhang, Yan Di, Ruida Zhang, Guangyao Zhai, Fabian Manhardt, Federico Tombari, Xiangyang Ji
Double Auctions with Two-sided Bandit Feedback
Soumya Basu, Abishek Sankararaman
Grammar Prompting for Domain-Specific Language Generation with Large Language Models
Bailin Wang, Zi Wang, Xuezhi Wang, Yuan Cao, Rif A. Saurous, Yoon Kim
Inconsistency, Instability, and Generalization Gap of Deep Neural Network Training
Rie Johnson, Tong Zhang*
Large Graph Property Prediction via Graph Segment Training
Kaidi Cao*, Phitchaya Mangpo Phothilimthana, Sami Abu-El-Haija, Dustin Zelle, Yanqi Zhou, Charith Mendis*, Jure Leskovec, Bryan Perozzi
On Computing Pairwise Statistics with Local Differential Privacy
Badih Ghazi, Pritish Kamath, Ravi Kumar, Pasin Manurangsi, Adam Sealfon
On Student-teacher Deviations in Distillation: Does it Pay to Disobey?
Vaishnavh Nagarajan, Aditya Krishna Menon, Srinadh Bhojanapalli, Hossein Mobahi, Sanjiv Kumar
Optimal Cross-learning for Contextual Bandits with Unknown Context Distributions
Jon Schneider, Julian Zimmert
Near-Optimal k-Clustering in the Sliding Window Model
David Woodruff, Peilin Zhong, Samson Zhou
Post Hoc Explanations of Language Models Can Improve Language Models
Satyapriya Krishna, Jiaqi Ma, Dylan Z Slack, Asma Ghandeharioun, Sameer Singh, Himabindu Lakkaraju
Recommender Systems with Generative Retrieval
Shashank Rajput*, Nikhil Mehta, Anima Singh, Raghunandan Hulikal Keshavan, Trung Vu, Lukasz Heldt, Lichan Hong, Yi Tay, Vinh Q. Tran, Jonah Samost, Maciej Kula, Ed H. Chi, Maheswaran Sathiamoorthy
Reinforcement Learning for Fine-tuning Text-to-Image Diffusion Models
Ying Fan, Olivia Watkins, Yuqing Du, Hao Liu, Moonkyung Ryu, Craig Boutilier, Pieter Abbeel, Mohammad Ghavamzadeh*, Kangwook Lee, Kimin Lee*
Replicable Clustering
Hossein Esfandiari, Amin Karbasi, Vahab Mirrokni, Grigoris Velegkas, Felix Zhou
Replicability in Reinforcement Learning
Amin Karbasi, Grigoris Velegkas, Lin Yang, Felix Zhou
Riemannian Projection-free Online Learning
Zihao Hu, Guanghui Wang, Jacob Abernethy
Sharpness-Aware Minimization Leads to Low-Rank Features
Maksym Andriushchenko, Dara Bahri, Hossein Mobahi, Nicolas Flammarion
What is the Inductive Bias of Flatness Regularization? A Study of Deep Matrix Factorization Models
Khashayar Gatmiry, Zhiyuan Li, Ching-Yao Chuang, Sashank Reddi, Tengyu Ma, Stefanie Jegelka
Block Low-Rank Preconditioner with Shared Basis for Stochastic Optimization
Jui-Nan Yen, Sai Surya Duvvuri, Inderjit S Dhillon, Cho-Jui Hsieh
Blocked Collaborative Bandits: Online Collaborative Filtering with Per-Item Budget Constraints
Soumyabrata Pal, Arun Sai Suggala, Karthikeyan Shanmugam, Prateek Jain
Boundary Guided Learning-Free Semantic Control with Diffusion Models
Ye Zhu, Yu Wu, Zhiwei Deng, Olga Russakovsky, Yan Yan
Conditional Adapters: Parameter-efficient Transfer Learning with Fast Inference
Tao Lei, Junwen Bai, Siddhartha Brahma, Joshua Ainslie, Kenton Lee, Yanqi Zhou, Nan Du*, Vincent Y. Zhao, Yuexin Wu, Bo Li, Yu Zhang, Ming-Wei Chang
Conformal Prediction for Time Series with Modern Hopfield Networks
Andreas Auer, Martin Gauch, Daniel Klotz, Sepp Hochreiter
Does Visual Pretraining Help End-to-End Reasoning?
Chen Sun, Calvin Luo, Xingyi Zhou, Anurag Arnab, Cordelia Schmid
Effective Robustness Against Natural Distribution Shifts for Models with Different Training Data
Zhouxing Shi*, Nicholas Carlini, Ananth Balashankar, Ludwig Schmidt, Cho-Jui Hsieh, Alex Beutel*, Yao Qin
Improving Neural Network Representations Using Human Similarity Judgments
Lukas Muttenthaler*, Lorenz Linhardt, Jonas Dippel, Robert A. Vandermeulen, Katherine Hermann, Andrew K. Lampinen, Simon Kornblith
Label Robust and Differentially Private Linear Regression: Computational and Statistical Efficiency
Xiyang Liu, Prateek Jain, Weihao Kong, Sewoong Oh, Arun Sai Suggala
Mnemosyne: Learning to Train Transformers with Transformers
Deepali Jain, Krzysztof Choromanski, Avinava Dubey, Sumeet Singh, Vikas Sindhwani, Tingnan Zhang, Jie Tan
Nash Regret Guarantees for Linear Bandits
Ayush Sawarni, Soumyabrata Pal, Siddharth Barman
A Near-Linear Time Algorithm for the Chamfer Distance
Ainesh Bakshi, Piotr Indyk, Rajesh Jayaram, Sandeep Silwal, Erik Waingarten.
On Differentially Private Sampling from Gaussian and Product Distributions
Badih Ghazi, Xiao Hu*, Ravi Kumar, Pasin Manurangsi
On Dynamic Programming Decompositions of Static Risk Measures in Markov Decision Processes
Jia Lin Hau, Erick Delage, Mohammad Ghavamzadeh*, Marek Petrik
ResMem: Learn What You Can and Memorize the Rest
Zitong Yang, Michal Lukasik, Vaishnavh Nagarajan, Zonglin Li, Ankit Singh Rawat, Manzil Zaheer, Aditya Krishna Menon, Sanjiv Kumar
Responsible AI (RAI) Games and Ensembles
Yash Gupta, Runtian Zhai, Arun Suggala, Pradeep Ravikumar
RoboCLIP: One Demonstration Is Enough to Learn Robot Policies
Sumedh A Sontakke, Jesse Zhang, Sébastien M. R. Arnold, Karl Pertsch, Erdem Biyik, Dorsa Sadigh, Chelsea Finn, Laurent Itti
Robust Concept Erasure via Kernelized Rate-Distortion Maximization
Somnath Basu Roy Chowdhury, Nicholas Monath, Kumar Avinava Dubey, Amr Ahmed, Snigdha Chaturvedi
Robust Multi-Agent Reinforcement Learning via Adversarial Regularization: Theoretical Foundation and Stable Algorithms
Alexander Bukharin, Yan Li, Yue Yu, Qingru Zhang, Zhehui Chen, Simiao Zuo, Chao Zhang, Songan Zhang, Tuo Zhao
Simplicity Bias in 1-Hidden Layer Neural Networks
Depen Morwani*, Jatin Batra, Prateek Jain, Praneeth Netrapalli
SLaM: Student-Label Mixing for Distillation with Unlabeled Examples
Vasilis Kontonis, Fotis Iliopoulos, Khoa Trinh, Cenk Baykal, Gaurav Menghani, Erik Vee
SNAP: Self-Supervised Neural Maps for Visual Positioning and Semantic Understanding
Paul-Edouard Sarlin*, Eduard Trulls, Marc Pollefeys, Jan Hosang, Simon Lynen
SOAR: Improved Indexing for Approximate Nearest Neighbor Search
Philip Sun, David Simcha, Dave Dopson, Ruiqi Guo, Sanjiv Kumar
StyleDrop: Text-to-Image Synthesis of Any Style
Kihyuk Sohn, Lu Jiang, Jarred Barber, Kimin Lee*, Nataniel Ruiz, Dilip Krishnan, Huiwen Chang*, Yuanzhen Li, Irfan Essa, Michael Rubinstein, Yuan Hao, Glenn Entis, Irina Blok, Daniel Castro Chin
Three Towers: Flexible Contrastive Learning with Pretrained Image Models
Jannik Kossen*, Mark Collier, Basil Mustafa, Xiao Wang, Xiaohua Zhai, Lucas Beyer, Andreas Steiner, Jesse Berent, Rodolphe Jenatton, Efi Kokiopoulou
Two-Stage Learning to Defer with Multiple Experts
Anqi Mao, Christopher Mohri, Mehryar Mohri, Yutao Zhong
AdANNS: A Framework for Adaptive Semantic Search
Aniket Rege, Aditya Kusupati, Sharan Ranjit S, Alan Fan, Qingqing Cao, Sham Kakade, Prateek Jain, Ali Farhadi
Cappy: Outperforming and Boosting Large Multi-Task LMs with a Small Scorer
Bowen Tan*, Yun Zhu, Lijuan Liu, Eric Xing, Zhiting Hu, Jindong Chen
Causal-structure Driven Augmentations for Text OOD Generalization
Amir Feder, Yoav Wald, Claudia Shi, Suchi Saria, David Blei
Dense-Exponential Random Features: Sharp Positive Estimators of the Gaussian Kernel
Valerii Likhosherstov, Krzysztof Choromanski, Avinava Dubey, Frederick Liu, Tamas Sarlos, Adrian Weller
Diffusion Hyperfeatures: Searching Through Time and Space for Semantic Correspondence
Grace Luo, Lisa Dunlap, Dong Huk Park, Aleksander Holynski, Trevor Darrell
Diffusion Self-Guidance for Controllable Image Generation
Dave Epstein, Allan Jabri, Ben Poole, Alexei A Efros, Aleksander Holynski
Fully Dynamic k-Clustering in Õ(k) Update Time
Sayan Bhattacharya, Martin Nicolas Costa, Silvio Lattanzi, Nikos Parotsidis
Improving CLIP Training with Language Rewrites
Lijie Fan, Dilip Krishnan, Phillip Isola, Dina Katabi, Yonglong Tian
<!–k-Means Clustering with Distance-Based Privacy
Alessandro Epasto, Vahab Mirrokni, Shyam Narayanan, Peilin Zhong
–>
LayoutGPT: Compositional Visual Planning and Generation with Large Language Models
Weixi Feng, Wanrong Zhu, Tsu-Jui Fu, Varun Jampani, Arjun Reddy Akula, Xuehai He, Sugato Basu, Xin Eric Wang, William Yang Wang
Offline Reinforcement Learning for Mixture-of-Expert Dialogue Management
Dhawal Gupta*, Yinlam Chow, Azamat Tulepbergenov, Mohammad Ghavamzadeh*, Craig Boutilier
Optimal Unbiased Randomizers for Regression with Label Differential Privacy
Ashwinkumar Badanidiyuru, Badih Ghazi, Pritish Kamath, Ravi Kumar, Ethan Jacob Leeman, Pasin Manurangsi, Avinash V Varadarajan, Chiyuan Zhang
Paraphrasing Evades Detectors of AI-generated Text, but Retrieval Is an Effective Defense
Kalpesh Krishna, Yixiao Song, Marzena Karpinska, John Wieting, Mohit Iyyer
ReMaX: Relaxing for Better Training on Efficient Panoptic Segmentation
Shuyang Sun*, Weijun Wang, Qihang Yu*, Andrew Howard, Philip Torr, Liang-Chieh Chen*
Robust and Actively Secure Serverless Collaborative Learning
Nicholas Franzese, Adam Dziedzic, Christopher A. Choquette-Choo, Mark R. Thomas, Muhammad Ahmad Kaleem, Stephan Rabanser, Congyu Fang, Somesh Jha, Nicolas Papernot, Xiao Wang
SpecTr: Fast Speculative Decoding via Optimal Transport
Ziteng Sun, Ananda Theertha Suresh, Jae Hun Ro, Ahmad Beirami, Himanshu Jain, Felix Yu
Structured Prediction with Stronger Consistency Guarantees
Anqi Mao, Mehryar Mohri, Yutao Zhong
Affinity-Aware Graph Networks
Ameya Velingker, Ali Kemal Sinop, Ira Ktena, Petar Veličković, Sreenivas Gollapudi
ARTIC3D: Learning Robust Articulated 3D Shapes from Noisy Web Image Collections
Chun-Han Yao*, Amit Raj, Wei-Chih Hung, Yuanzhen Li, Michael Rubinstein, Ming-Hsuan Yang, Varun Jampani
Black-Box Differential Privacy for Interactive ML
Haim Kaplan, Yishay Mansour, Shay Moran, Kobbi Nissim, Uri Stemmer
Bypassing the Simulator: Near-Optimal Adversarial Linear Contextual Bandits
Haolin Liu, Chen-Yu Wei, Julian Zimmert
DaTaSeg: Taming a Universal Multi-Dataset Multi-Task Segmentation Model
Xiuye Gu, Yin Cui*, Jonathan Huang, Abdullah Rashwan, Xuan Yang, Xingyi Zhou, Golnaz Ghiasi, Weicheng Kuo, Huizhong Chen, Liang-Chieh Chen*, David Ross
Easy Learning from Label Proportions
Robert Busa-Fekete, Heejin Choi*, Travis Dick, Claudio Gentile, Andres Munoz Medina
Efficient Data Subset Selection to Generalize Training Across Models: Transductive and Inductive Networks
Eeshaan Jain, Tushar Nandy, Gaurav Aggarwal, Ashish Tendulkar, Rishabh Iyer, Abir De
Faster Differentially Private Convex Optimization via Second-Order Methods
Arun Ganesh, Mahdi Haghifam*, Thomas Steinke, Abhradeep Guha Thakurta
Finding Safe Zones of Markov Decision Processes Policies
Lee Cohen, Yishay Mansour, Michal Moshkovitz
Focused Transformer: Contrastive Training for Context Scaling
Szymon Tworkowski, Konrad Staniszewski, Mikołaj Pacek, Yuhuai Wu*, Henryk Michalewski, Piotr Miłoś
Front-door Adjustment Beyond Markov Equivalence with Limited Graph Knowledge
Abhin Shah, Karthikeyan Shanmugam, Murat Kocaoglu
H-Consistency Bounds: Characterization and Extensions
Anqi Mao, Mehryar Mohri, Yutao Zhong
Inverse Dynamics Pretraining Learns Good Representations for Multitask Imitation
David Brandfonbrener, Ofir Nachum, Joan Bruna
Most Neural Networks Are Almost Learnable
Amit Daniely, Nathan Srebro, Gal Vardi
Multiclass Boosting: Simple and Intuitive Weak Learning Criteria
Nataly Brukhim, Amit Daniely, Yishay Mansour, Shay Moran
NeRF Revisited: Fixing Quadrature Instability in Volume Rendering
Mikaela Angelina Uy, Kiyohiro Nakayama, Guandao Yang, Rahul Krishna Thomas, Leonidas Guibas, Ke Li
Privacy Amplification via Compression: Achieving the Optimal Privacy-Accuracy-Communication Trade-off in Distributed Mean Estimation
Wei-Ning Chen, Dan Song, Ayfer Ozgur, Peter Kairouz
Private Federated Frequency Estimation: Adapting to the Hardness of the Instance
Jingfeng Wu*, Wennan Zhu, Peter Kairouz, Vladimir Braverman
RETVec: Resilient and Efficient Text Vectorizer
Elie Bursztein, Marina Zhang, Owen Skipper Vallis, Xinyu Jia, Alexey Kurakin
Symbolic Discovery of Optimization Algorithms
Xiangning Chen*, Chen Liang, Da Huang, Esteban Real, Kaiyuan Wang, Hieu Pham, Xuanyi Dong, Thang Luong, Cho-Jui Hsieh, Yifeng Lu, Quoc V. Le
A Tale of Two Features: Stable Diffusion Complements DINO for Zero-Shot Semantic Correspondence
Junyi Zhang, Charles Herrmann, Junhwa Hur, Luisa F. Polania, Varun Jampani, Deqing Sun, Ming-Hsuan Yang
A Trichotomy for Transductive Online Learning
Steve Hanneke, Shay Moran, Jonathan Shafer
A Unified Fast Gradient Clipping Framework for DP-SGD
William Kong, Andres Munoz Medina
Unleashing the Power of Randomization in Auditing Differentially Private ML
Krishna Pillutla, Galen Andrew, Peter Kairouz, H. Brendan McMahan, Alina Oprea, Sewoong Oh
(Amplified) Banded Matrix Factorization: A unified approach to private training
Christopher A Choquette-Choo, Arun Ganesh, Ryan McKenna, H Brendan McMahan, Keith Rush, Abhradeep Guha Thakurta, Zheng Xu
Adversarial Resilience in Sequential Prediction via Abstention
Surbhi Goel, Steve Hanneke, Shay Moran, Abhishek Shetty
Alternating Gradient Descent and Mixture-of-Experts for Integrated Multimodal Perception
Hassan Akbari, Dan Kondratyuk, Yin Cui, Rachel Hornung, Huisheng Wang, Hartwig Adam
Android in the Wild: A Large-Scale Dataset for Android Device Control
Christopher Rawles, Alice Li, Daniel Rodriguez, Oriana Riva, Timothy Lillicrap
Benchmarking Robustness to Adversarial Image Obfuscations
Florian Stimberg, Ayan Chakrabarti, Chun-Ta Lu, Hussein Hazimeh, Otilia Stretcu, Wei Qiao, Yintao Liu, Merve Kaya, Cyrus Rashtchian, Ariel Fuxman, Mehmet Tek, Sven Gowal
Building Socio-culturally Inclusive Stereotype Resources with Community Engagement
Sunipa Dev, Jaya Goyal, Dinesh Tewari, Shachi Dave, Vinodkumar Prabhakaran
Consensus and Subjectivity of Skin Tone Annotation for ML Fairness
Candice Schumann, Gbolahan O Olanubi, Auriel Wright, Ellis Monk Jr*, Courtney Heldreth, Susanna Ricco
Counting Distinct Elements Under Person-Level Differential Privacy
Alexander Knop, Thomas Steinke
DICES Dataset: Diversity in Conversational AI Evaluation for Safety
Lora Aroyo, Alex S. Taylor, Mark Diaz, Christopher M. Homan, Alicia Parrish, Greg Serapio-García, Vinodkumar Prabhakaran, Ding Wang
Does Progress on ImageNet Transfer to Real-world Datasets?
Alex Fang, Simon Kornblith, Ludwig Schmidt
Estimating Generic 3D Room Structures from 2D Annotations
Denys Rozumnyi*, Stefan Popov, Kevis-kokitsi Maninis, Matthias Nießner, Vittorio Ferrari
Large Language Model as Attributed Training Data Generator: A Tale of Diversity and Bias
Yue Yu, Yuchen Zhuang, Jieyu Zhang, Yu Meng, Alexander Ratner, Ranjay Krishna, Jiaming Shen, Chao Zhang
MADLAD-400: A Multilingual And Document-Level Large Audited Dataset
Sneha Kudugunta, Isaac Caswell, Biao Zhang, Xavier Garcia, Derrick Xin, Aditya Kusupati, Romi Stella, Ankur Bapna, Orhan Firat
Mechanic: A Learning Rate Tuner
Ashok Cutkosky, Aaron Defazio, Harsh Mehta
NAVI: Category-Agnostic Image Collections with High-Quality 3D Shape and Pose Annotations
Varun Jampani, Kevis-kokitsi Maninis, Andreas Engelhardt, Arjun Karpur, Karen Truong, Kyle Sargent, Stefan Popov, Andre Araujo, Ricardo Martin Brualla, Kaushal Patel, Daniel Vlasic, Vittorio Ferrari, Ameesh Makadia, Ce Liu*, Yuanzhen Li, Howard Zhou
Neural Ideal Large Eddy Simulation: Modeling Turbulence with Neural Stochastic Differential Equations
Anudhyan Boral, Zhong Yi Wan, Leonardo Zepeda-Nunez, James Lottes, Qing Wang, Yi-Fan Chen, John Roberts Anderson, Fei Sha
Restart Sampling for Improving Generative Processes
Yilun Xu, Mingyang Deng, Xiang Cheng, Yonglong Tian, Ziming Liu, Tommi Jaakkola
Rethinking Incentives in Recommender Systems: Are Monotone Rewards Always Beneficial?
Fan Yao, Chuanhao Li, Karthik Abinav Sankararaman, Yiming Liao, Yan Zhu, Qifan Wang, Hongning Wang, Haifeng Xu
Revisiting Evaluation Metrics for Semantic Segmentation: Optimization and Evaluation of Fine-grained Intersection over Union
Zifu Wang, Maxim Berman, Amal Rannen-Triki, Philip Torr, Devis Tuia, Tinne Tuytelaars, Luc Van Gool, Jiaqian Yu, Matthew B. Blaschko
RoboHive: A Unified Framework for Robot Learning
Vikash Kumar, Rutav Shah, Gaoyue Zhou, Vincent Moens, Vittorio Caggiano, Abhishek Gupta, Aravind Rajeswaran
SatBird: Bird Species Distribution Modeling with Remote Sensing and Citizen Science Data
Mélisande Teng, Amna Elmustafa, Benjamin Akera, Yoshua Bengio, Hager Radi, Hugo Larochelle, David Rolnick
Sparsity-Preserving Differentially Private Training of Large Embedding Models
Badih Ghazi, Yangsibo Huang*, Pritish Kamath, Ravi Kumar, Pasin Manurangsi, Amer Sinha, Chiyuan Zhang
StableRep: Synthetic Images from Text-to-Image Models Make Strong Visual Representation Learners
Yonglong Tian, Lijie Fan, Phillip Isola, Huiwen Chang, Dilip Krishnan
Towards Federated Foundation Models: Scalable Dataset Pipelines for Group-Structured Learning
Zachary Charles, Nicole Mitchell, Krishna Pillutla, Michael Reneer, Zachary Garrett
Universality and Limitations of Prompt Tuning
Yihan Wang, Jatin Chauhan, Wei Wang, Cho-Jui Hsieh
Unsupervised Semantic Correspondence Using Stable Diffusion
Eric Hedlin, Gopal Sharma, Shweta Mahajan, Hossam Isack, Abhishek Kar, Andrea Tagliasacchi, Kwang Moo Yi
YouTube-ASL: A Large-Scale, Open-Domain American Sign Language-English Parallel Corpus
Dave Uthus, Garrett Tanzer, Manfred Georg
The Noise Level in Linear Regression with Dependent Data
Ingvar Ziemann, Stephen Tu, George J. Pappas, Nikolai Matni
* Work done while at Google
LiDAR: Sensing Linear Probing Performance in Joint Embedding SSL Architectures
Joint embedding (JE) architectures have emerged as a promising avenue for acquiring transferable data representations. A key obstacle to using JE methods, however, is the inherent challenge of evaluating learned representations without access to a downstream task, and an annotated dataset. Without efficient and reliable evaluation, it is difficult to iterate on architectural and training choices for JE methods. In this paper, we introduce LiDAR (Linear Discriminant Analysis Rank), a metric designed to measure the quality of representations within JE architectures. Our metric addresses several…Apple Machine Learning Research
Custom policy checks help democratize automated reasoning
New IAM Access Analyzer feature uses automated reasoning to ensure that access policies written in the IAM policy language don’t inadvertently grant illicit access.Read More
Sparsity-preserving differentially private training
Large embedding models have emerged as a fundamental tool for various applications in recommendation systems [1, 2] and natural language processing [3, 4, 5]. Such models enable the integration of non-numerical data into deep learning models by mapping categorical or string-valued input attributes with large vocabularies to fixed-length representation vectors using embedding layers. These models are widely deployed in personalized recommendation systems and achieve state-of-the-art performance in language tasks, such as language modeling, sentiment analysis, and question answering. In many such scenarios, privacy is an equally important feature when deploying those models. As a result, various techniques have been proposed to enable private data analysis. Among those, differential privacy (DP) is a widely adopted definition that limits exposure of individual user information while still allowing for the analysis of population-level patterns.
For training deep neural networks with DP guarantees, the most widely used algorithm is DP-SGD (DP stochastic gradient descent). One key component of DP-SGD is adding Gaussian noise to every coordinate of the gradient vectors during training. However, this creates scalability challenges when applied to large embedding models, because they rely on gradient sparsity for efficient training, but adding noise to all the coordinates destroys sparsity.
To mitigate this gradient sparsity problem, in “Sparsity-Preserving Differentially Private Training of Large Embedding Models” (to be presented at NeurIPS 2023), we propose a new algorithm called adaptive filtering-enabled sparse training (DP-AdaFEST). At a high level, the algorithm maintains the sparsity of the gradient by selecting only a subset of feature rows to which noise is added at each iteration. The key is to make such selections differentially private so that a three-way balance is achieved among the privacy cost, the training efficiency, and the model utility. Our empirical evaluation shows that DP-AdaFEST achieves a substantially sparser gradient, with a reduction in gradient size of over 105X compared to the dense gradient produced by standard DP-SGD, while maintaining comparable levels of accuracy. This gradient size reduction could translate into 20X wall-clock time improvement.
Overview
To better understand the challenges and our solutions to the gradient sparsity problem, let us start with an overview of how DP-SGD works during training. As illustrated by the figure below, DP-SGD operates by clipping the gradient contribution from each example in the current random subset of samples (called a mini-batch), and adding coordinate-wise Gaussian noise to the average gradient during each iteration of stochastic gradient descent (SGD). DP-SGD has demonstrated its effectiveness in protecting user privacy while maintaining model utility in a variety of applications [6, 7].
The challenges of applying DP-SGD to large embedding models mainly come from 1) the non-numerical feature fields like user/product IDs and categories, and 2) words and tokens that are transformed into dense vectors through an embedding layer. Due to the vocabulary sizes of those features, the process requires large embedding tables with a substantial number of parameters. In contrast to the number of parameters, the gradient updates are usually extremely sparse because each mini-batch of examples only activates a tiny fraction of embedding rows (the figure below visualizes the ratio of zero-valued coordinates, i.e., the sparsity, of the gradients under various batch sizes). This sparsity is heavily leveraged for industrial applications that efficiently handle the training of large-scale embeddings. For example, Google Cloud TPUs, custom-designed AI accelerators which are optimized for training and inference of large AI models, have dedicated APIs to handle large embeddings with sparse updates. This leads to significantly improved training throughput compared to training on GPUs, which at thisAt a high level, the algorithm maintains the sparsity of the gradient by selecting only a subset of feature rows to which noise is added at each iteration. time did not have specialized optimization for sparse embedding lookups. On the other hand, DP-SGD completely destroys the gradient sparsity because it requires adding independent Gaussian noise to all the coordinates. This creates a road block for private training of large embedding models as the training efficiency would be significantly reduced compared to non-private training.
![]() |
Embedding gradient sparsity (the fraction of zero-value gradient coordinates) in the Criteo pCTR model (see below). The figure reports the gradient sparsity, averaged over 50 update steps, of the top five categorical features (out of a total of 26) with the highest number of buckets, as well as the sparsity of all categorical features. The sprasity decreases with the batch size as more examples hit more rows in the embedding table, creating non-zero gradients. However, the sparsity is above 0.97 even for very large batch sizes. This pattern is consistently observed for all the five features. |
Algorithm
Our algorithm is built by extending standard DP-SGD with an extra mechanism at each iteration to privately select the “hot features”, which are the features that are activated by multiple training examples in the current mini-batch. As illustrated below, the mechanism works in a few steps:
- Compute how many examples contributed to each feature bucket (we call each of the possible values of a categorical feature a “bucket”).
- Restrict the total contribution from each example by clipping their counts.
- Add Gaussian noise to the contribution count of each feature bucket.
- Select only the features to be included in the gradient update that have a count above a given threshold (a sparsity-controlling parameter), thus maintaining sparsity. This mechanism is differentially private, and the privacy cost can be easily computed by composing it with the standard DP-SGD iterations.
Theoretical motivation
We provide the theoretical motivation that underlies DP-AdaFEST by viewing it as optimization using stochastic gradient oracles. Standard analysis of stochastic gradient descent in a theoretical setting decomposes the test error of the model into “bias” and “variance” terms. The advantage of DP-AdaFEST can be viewed as reducing variance at the cost of slightly increasing the bias. This is because DP-AdaFEST adds noise to a smaller set of coordinates compared to DP-SGD, which adds noise to all the coordinates. On the other hand, DP-AdaFEST introduces some bias to the gradients since the gradient on the embedding features are dropped with some probability. We refer the interested reader to Section 3.4 of the paper for more details.
Experiments
We evaluate the effectiveness of our algorithm with large embedding model applications, on public datasets, including one ad prediction dataset (Criteo-Kaggle) and one language understanding dataset (SST-2). We use DP-SGD with exponential selection as a baseline comparison.
The effectiveness of DP-AdaFEST is evident in the figure below, where it achieves significantly higher gradient size reduction (i.e., gradient sparsity) than the baseline while maintaining the same level of utility (i.e., only minimal performance degradation).
Specifically, on the Criteo-Kaggle dataset, DP-AdaFEST reduces the gradient computation cost of regular DP-SGD by more than 5×105 times while maintaining a comparable AUC (which we define as a loss of less than 0.005). This reduction translates into a more efficient and cost-effective training process. In comparison, as shown by the green line below, the baseline method is not able to achieve reasonable cost reduction within such a small utility loss threshold.
In language tasks, there isn’t as much potential for reducing the size of gradients, because the vocabulary used is often smaller and already quite compact (shown on the right below). However, the adoption of sparsity-preserving DP-SGD effectively obviates the dense gradient computation. Furthermore, in line with the bias-variance trade-off presented in the theoretical analysis, we note that DP-AdaFEST occasionally exhibits superior utility compared to DP-SGD when the reduction in gradient size is minimal. Conversely, when incorporating sparsity, the baseline algorithm faces challenges in maintaining utility.
![]() |
A comparison of the best gradient size reduction (the ratio of the non-zero gradient value counts between regular DP-SGD and sparsity-preserving algorithms) achieved under ε =1.0 by DP-AdaFEST (our algorithm) and the baseline algorithm (DP-SGD with exponential selection) compared to DP-SGD at different thresholds for utility difference. A higher curve indicates a better utility/efficiency trade-off. |
In practice, most ad prediction models are being continuously trained and evaluated. To simulate this online learning setup, we also evaluate with time-series data, which are notoriously challenging due to being non-stationary. Our evaluation uses the Criteo-1TB dataset, which comprises real-world user-click data collected over 24 days. Consistently, DP-AdaFEST reduces the gradient computation cost of regular DP-SGD by more than 104 times while maintaining a comparable AUC.
Conclusion
We present a new algorithm, DP-AdaFEST, for preserving gradient sparsity in differentially private training — particularly in applications involving large embedding models, a fundamental tool for various applications in recommendation systems and natural language processing. Our algorithm achieves significant reductions in gradient size while maintaining accuracy on real-world benchmark datasets. Moreover, it offers flexible options for balancing utility and efficiency via sparsity-controlling parameters, while our proposals offer much better privacy-utility loss.
Acknowledgements
This work was a collaboration with Badih Ghazi, Pritish Kamath, Ravi Kumar, Pasin Manurangsi and Amer Sinha.
Creative Robot Tool Use with Large Language Models
TLDR: We introduce RoboTool, enabling robots to use tools creatively with large language models, which solves long-horizon hybrid discrete-continuous planning problems with the environment- and embodiment-related constraints.
Tool use is an essential hallmark of advanced intelligence. Some animals can use tools to achieve goals that are infeasible without tools. For example, crows solve a complex physical puzzle using a series of tools, and apes use a tree branch to crack open nuts or fish termites with a stick. Beyond using tools for their intended purpose and following established procedures, using tools in creative and unconventional ways provides more flexible solutions, albeit presents far more challenges in cognitive ability.
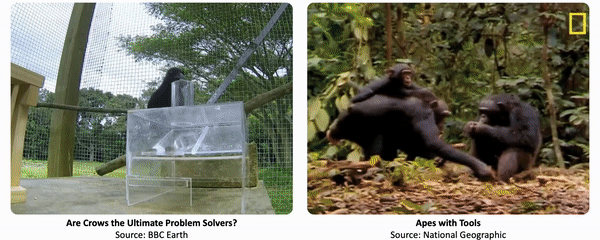
In robotics, creative tool use is also a crucial yet very demanding capability because it necessitates the all-around ability to predict the outcome of an action, reason what tools to use, and plan how to use them. In this work, we want to explore the question, can we enable such creative tool-use capability in robots? We identify that creative robot tool use solves a complex long-horizon planning task with constraints related to environment and robot capacity. For example, ”grasp a milk carton” while the milk carton’s location is out of the robotic arm’s workspace or ”walking to the other sofa” while there exists a gap in the way that exceeds the quadrupedal robot’s walking capability.
Task and motion planning (TAMP) is a common framework for solving such long-horizon planning tasks. It combines low-level continuous motion planning in classic robotics and high-level discrete task planning to solve complex planning tasks that are difficult to address by any of these domains alone. Existing literature shows that it can handle tool use in a static environment with optimization-based approaches such as logic-geometric programming. However, this optimization approach generally requires a long computation time for tasks with many objects and task planning steps due to the increasing search space. In addition, classical TAMP methods are limited to the family of tasks that can be expressed in formal logic and symbolic representation, making them not user-friendly for non-experts.
Recently, large language models (LLMs) have been shown to encode vast knowledge beneficial to robotics tasks in reasoning, planning, and acting. TAMP methods with LLMs can bypass the computation burden of the explicit optimization process in classical TAMP. Prior works show that LLMs can adeptly dissect tasks given either clear or ambiguous language descriptions and instructions. However, it is still unclear how to use LLMs to solve more complex tasks that require reasoning with implicit constraints imposed by the robot’s embodiment and its surrounding physical world.
Methods
In this work, we are interested in solving language-instructed long-horizon robotics tasks with implicitly activated physical constraints. By providing LLMs with adequate numerical semantic information in natural language, we observe that LLMs can identify the activated constraints induced by the spatial layout of objects in the scene and the robot’s embodiment limits, suggesting that LLMs may maintain knowledge and reasoning capability about the 3D physical world. Furthermore, our comprehensive tests reveal that LLMs are not only adept at employing tools to transform otherwise unfeasible tasks into feasible ones but also display creativity in using tools beyond their conventional functions, based on their material, shape, and geometric features.
To solve the aforementioned problem, we introduce RoboTool, a creative robot tool user built on LLMs, which uses tools beyond their standard affordances. RoboTool accepts natural language instructions comprising textual and numerical information about the environment, robot embodiments, and constraints to follow. RoboTool produces code that invokes the robot’s parameterized low-level skills to control both simulated and physical robots. RoboTool consists of four central components, with each handling one functionality, as depicted below:
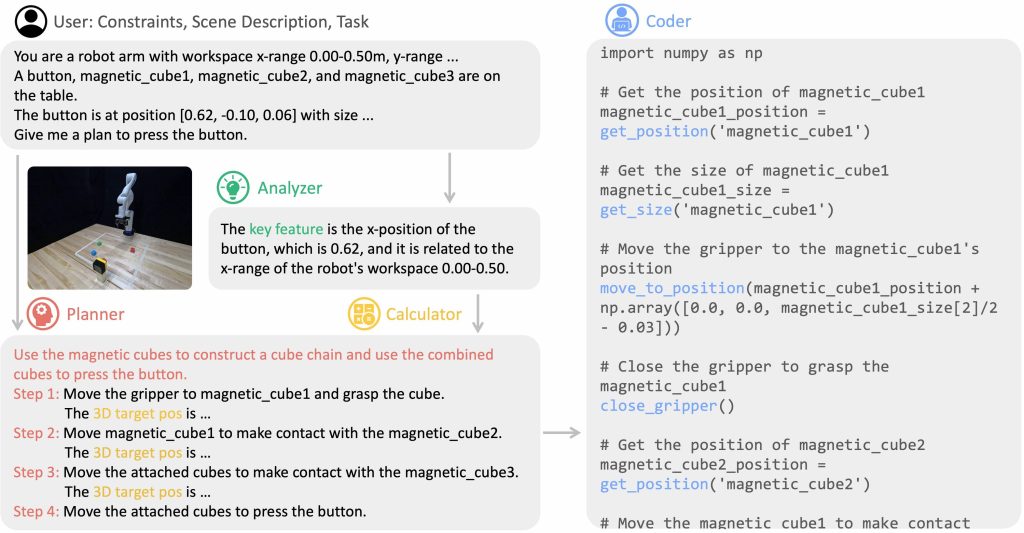
- Analyzer, which processes the natural language input to identify key concepts that could impact the task’s feasibility.
- Planner, which receives both the original language input and the identified key concepts to formulate a comprehensive strategy for completing the task.
- Calculator, which is responsible for determining the parameters, such as the target positions required for each parameterized skill.
- Coder, which converts the comprehensive plan and parameters into executable code. All of these components are constructed using GPT-4.
Benchmark
In this work, we aim to explore three challenging categories of creative tool use for robots: tool selection, sequential tool use, and tool manufacturing. We design six tasks for two different robot embodiments: a quadrupedal robot and a robotic arm.
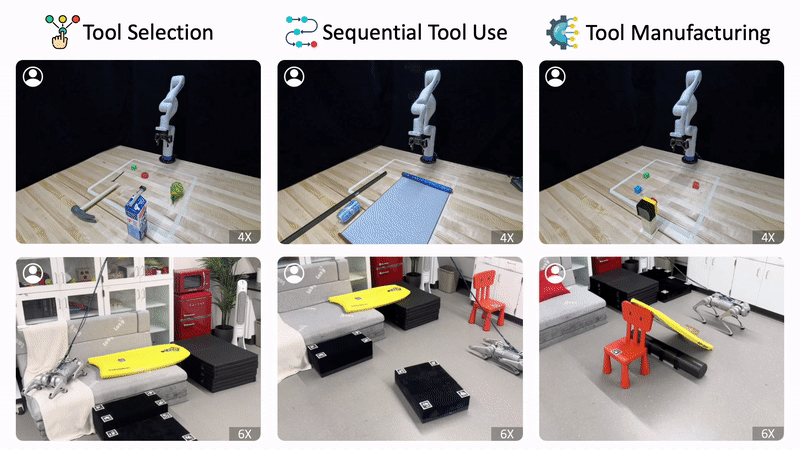
- Tool selection (Sofa-Traversing and Milk-Reaching) requires the reasoning capability to choose the most appropriate tools among multiple options. It demands a broad understanding of object attributes such as size, material, and shape, as well as the ability to analyze the relationship between these properties and the intended objective.
- Sequential tool use (Sofa-Climbing and Can-Grasping) entails utilizing a series of tools in a specific order to reach a desired goal. Its complexity arises from the need for long-horizon planning to determine the best sequence for tool use, with successful completion depending on the accuracy of each step in the plan.
- Tool manufacturing (Cube-Lifting and Button-Pressing) involves accomplishing tasks by crafting tools from available materials or adapting existing ones. This procedure requires the robot to discern implicit connections among objects and assemble components through manipulation.
Results
We compare RoboTool with four baselines, including one variant of Code-as-Policies (Coder) and three variants of our proposed, including RoboTool without Analyzer, RoboTool without Calculator, and Planner-Coder. Our evaluation results show that RoboTool consistently achieves success rates that are either comparable to or exceed those of the baselines across six tasks in simulation. RoboTool’s performance in the real world drops by 0.1 in comparison to the simulation result, mainly due to the perception errors and execution errors associated with parameterized skills, such as the quadrupedal robot falling down the soft sofa. Nonetheless, RoboTool (Real World) still surpasses the simulated performance of all baselines.
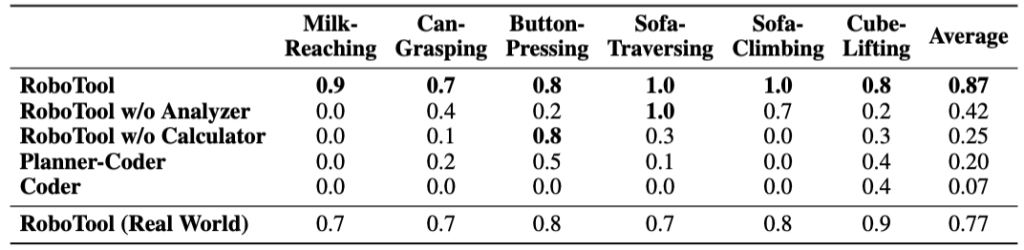
We define three types of errors: tool-use error indicating whether the correct tool is used, logical error focusing on planning errors such as using tools in the wrong order or ignoring the provided constraints, and numerical error including calculating the wrong target positions or adding incorrect offsets. By comparing RoboTool and RoboTool w/o Analyzer, we show that the Analyzer helps reduce the tool-use error. Moreover, the Calculator significantly reduces the numerical error.
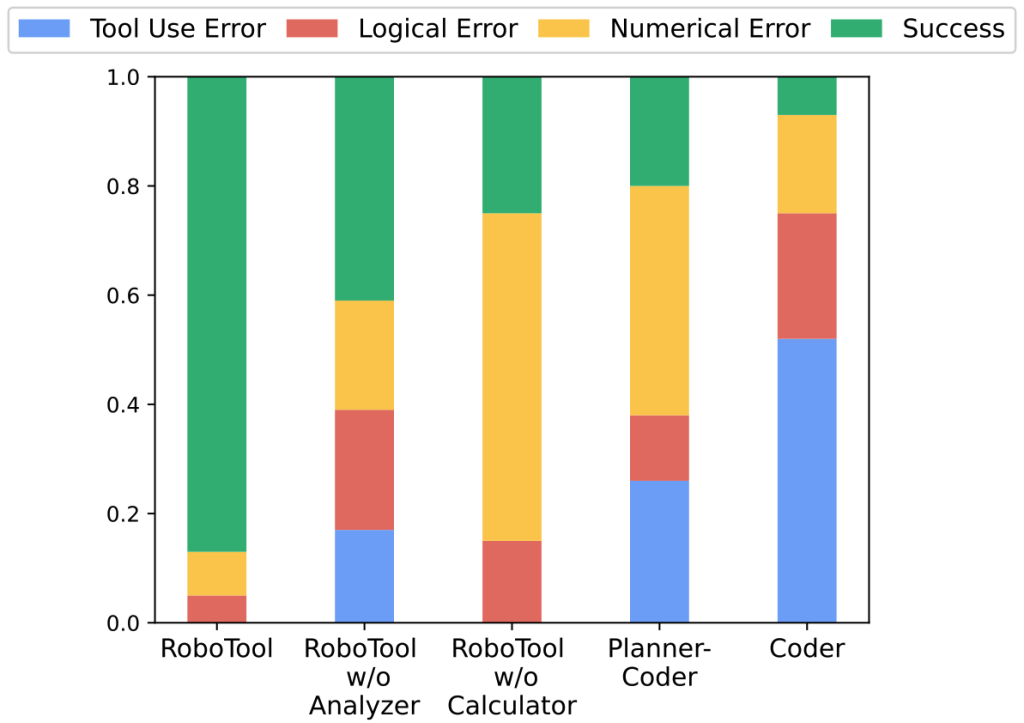
By discerning the critical concept, RoboTool enables discriminative tool-use behaviors — using tools only when necessary — showing more accurate grounding related to the environment and embodiment instead of being purely dominated by the prior knowledge in the LLMs.
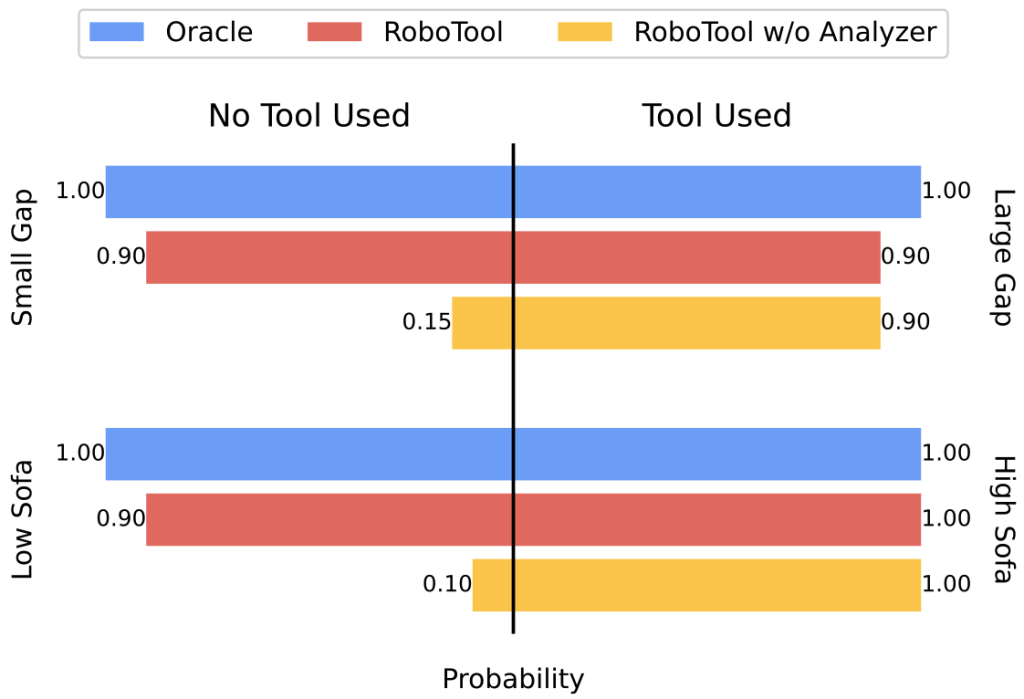
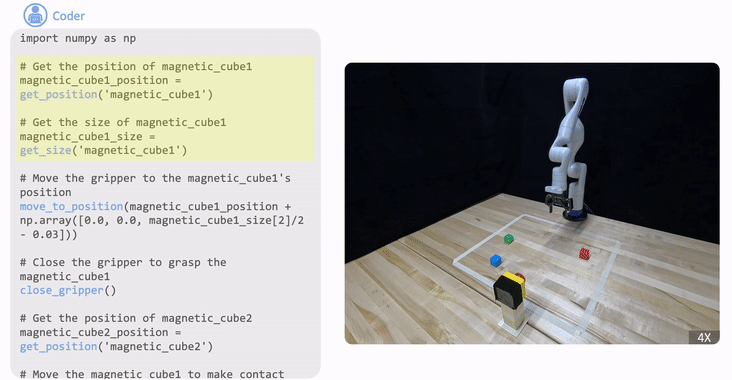
Takeaways
- Our proposed RoboTool can solve long-horizon hybrid discrete-continuous planning problems with the environment- and embodiment-related constraints in a zero-shot manner.
- We provide an evaluation benchmark to test various aspects of creative tool-use capability, including tool selection, sequential tool use, and tool manufacturing.
Paper: https://arxiv.org/pdf/2310.13065.pdf
Website: https://creative-robotool.github.io/
Twitter: https://x.com/mengdibellaxu/status/1716447045052215423?s=20
Google DeepMind at NeurIPS 2023
The Neural Information Processing Systems (NeurIPS) is the largest artificial intelligence (AI) conference in the world. NeurIPS 2023 will be taking place December 10-16 in New Orleans, USA.Teams from across Google DeepMind are presenting more than 150 papers at the main conference and workshops.Read More
NotebookLM adds more than a dozen new features
Now available in the U.S., NotebookLM has new features to help you easily read, take notes and organize your writing projects.Read More