We study differentially private stochastic convex optimization (DP-SCO) under user-level privacy where each user may hold multiple data items. Existing work for user-level DP-SCO either requires super-polynomial runtime or requires number of users that grows polynomially with the dimensionality of the problem. We develop new algorithms for user-level DP-SCO that obtain optimal rates, run in polynomial time, and require a number of users that grow logarithmically in the dimension. Moreover, our algorithms are the first to obtain optimal rates for non-smooth functions in polynomial time. These…Apple Machine Learning Research
Architect defense-in-depth security for generative AI applications using the OWASP Top 10 for LLMs
Generative artificial intelligence (AI) applications built around large language models (LLMs) have demonstrated the potential to create and accelerate economic value for businesses. Examples of applications include conversational search, customer support agent assistance, customer support analytics, self-service virtual assistants, chatbots, rich media generation, content moderation, coding companions to accelerate secure, high-performance software development, deeper insights from multimodal content sources, acceleration of your organization’s security investigations and mitigations, and much more. Many customers are looking for guidance on how to manage security, privacy, and compliance as they develop generative AI applications. Understanding and addressing LLM vulnerabilities, threats, and risks during the design and architecture phases helps teams focus on maximizing the economic and productivity benefits generative AI can bring. Being aware of risks fosters transparency and trust in generative AI applications, encourages increased observability, helps to meet compliance requirements, and facilitates informed decision-making by leaders.
The goal of this post is to empower AI and machine learning (ML) engineers, data scientists, solutions architects, security teams, and other stakeholders to have a common mental model and framework to apply security best practices, allowing AI/ML teams to move fast without trading off security for speed. Specifically, this post seeks to help AI/ML and data scientists who may not have had previous exposure to security principles gain an understanding of core security and privacy best practices in the context of developing generative AI applications using LLMs. We also discuss common security concerns that can undermine trust in AI, as identified by the Open Worldwide Application Security Project (OWASP) Top 10 for LLM Applications, and show ways you can use AWS to increase your security posture and confidence while innovating with generative AI.
This post provides three guided steps to architect risk management strategies while developing generative AI applications using LLMs. We first delve into the vulnerabilities, threats, and risks that arise from the implementation, deployment, and use of LLM solutions, and provide guidance on how to start innovating with security in mind. We then discuss how building on a secure foundation is essential for generative AI. Lastly, we connect these together with an example LLM workload to describe an approach towards architecting with defense-in-depth security across trust boundaries.
By the end of this post, AI/ML engineers, data scientists, and security-minded technologists will be able to identify strategies to architect layered defenses for their generative AI applications, understand how to map OWASP Top 10 for LLMs security concerns to some corresponding controls, and build foundational knowledge towards answering the following top AWS customer question themes for their applications:
- What are some of the common security and privacy risks with using generative AI based on LLMs in my applications that I can most impact with this guidance?
- What are some ways to implement security and privacy controls in the development lifecycle for generative AI LLM applications on AWS?
- What operational and technical best practices can I integrate into how my organization builds generative AI LLM applications to manage risk and increase confidence in generative AI applications using LLMs?
Improve security outcomes while developing generative AI
Innovation with generative AI using LLMs requires starting with security in mind to develop organizational resiliency, build on a secure foundation, and integrate security with a defense in depth security approach. Security is a shared responsibility between AWS and AWS customers. All the principles of the AWS Shared Responsibility Model are applicable to generative AI solutions. Refresh your understanding of the AWS Shared Responsibility Model as it applies to infrastructure, services, and data when you build LLM solutions.
Start with security in mind to develop organizational resiliency
Start with security in mind to develop organizational resiliency for developing generative AI applications that meet your security and compliance objectives. Organizational resiliency draws on and extends the definition of resiliency in the AWS Well-Architected Framework to include and prepare for the ability of an organization to recover from disruptions. Consider your security posture, governance, and operational excellence when assessing overall readiness to develop generative AI with LLMs and your organizational resiliency to any potential impacts. As your organization advances its use of emerging technologies such as generative AI and LLMs, overall organizational resiliency should be considered as a cornerstone of a layered defensive strategy to protect assets and lines of business from unintended consequences.
Organizational resiliency matters substantially for LLM applications
Although all risk management programs can benefit from resilience, organizational resiliency matters substantially for generative AI. Five of the OWASP-identified top 10 risks for LLM applications rely on defining architectural and operational controls and enforcing them at an organizational scale in order to manage risk. These five risks are insecure output handling, supply chain vulnerabilities, sensitive information disclosure, excessive agency, and overreliance. Begin increasing organizational resiliency by socializing your teams to consider AI, ML, and generative AI security a core business requirement and top priority throughout the whole lifecycle of the product, from inception of the idea, to research, to the application’s development, deployment, and use. In addition to awareness, your teams should take action to account for generative AI in governance, assurance, and compliance validation practices.
Build organizational resiliency around generative AI
Organizations can start adopting ways to build their capacity and capabilities for AI/ML and generative AI security within their organizations. You should begin by extending your existing security, assurance, compliance, and development programs to account for generative AI.
The following are the five key areas of interest for organizational AI, ML, and generative AI security:
- Understand the AI/ML security landscape
- Include diverse perspectives in security strategies
- Take action proactively for securing research and development activities
- Align incentives with organizational outcomes
- Prepare for realistic security scenarios in AI/ML and generative AI
Develop a threat model throughout your generative AI Lifecycle
Organizations building with generative AI should focus on risk management, not risk elimination, and include threat modeling in and business continuity planning the planning, development, and operations of generative AI workloads. Work backward from production use of generative AI by developing a threat model for each application using traditional security risks as well as generative AI-specific risks. Some risks may be acceptable to your business, and a threat modeling exercise can help your company identify what your acceptable risk appetite is. For example, your business may not require 99.999% uptime on a generative AI application, so the additional recovery time associated to recovery using AWS Backup with Amazon S3 Glacier may be an acceptable risk. Conversely, the data in your model may be extremely sensitive and highly regulated, so deviation from AWS Key Management Service (AWS KMS) customer managed key (CMK) rotation and use of AWS Network Firewall to help enforce Transport Layer Security (TLS) for ingress and egress traffic to protect against data exfiltration may be an unacceptable risk.
Evaluate the risks (inherent vs. residual) of using the generative AI application in a production setting to identify the right foundational and application-level controls. Plan for rollback and recovery from production security events and service disruptions such as prompt injection, training data poisoning, model denial of service, and model theft early on, and define the mitigations you will use as you define application requirements. Learning about the risks and controls that need to be put in place will help define the best implementation approach for building a generative AI application, and provide stakeholders and decision-makers with information to make informed business decisions about risk. If you are unfamiliar with the overall AI and ML workflow, start by reviewing 7 ways to improve security of your machine learning workloads to increase familiarity with the security controls needed for traditional AI/ML systems.
Just like building any ML application, building a generative AI application involves going through a set of research and development lifecycle stages. You may want to review the AWS Generative AI Security Scoping Matrix to help build a mental model to understand the key security disciplines that you should consider depending on which generative AI solution you select.
Generative AI applications using LLMs are typically developed and operated following ordered steps:
- Application requirements – Identify use case business objectives, requirements, and success criteria
- Model selection – Select a foundation model that aligns with use case requirements
- Model adaptation and fine-tuning – Prepare data, engineer prompts, and fine-tune the model
- Model evaluation – Evaluate foundation models with use case-specific metrics and select the best-performing model
- Deployment and integration – Deploy the selected foundation model on your optimized infrastructure and integrate with your generative AI application
- Application monitoring – Monitor application and model performance to enable root cause analysis
Ensure teams understand the critical nature of security as part of the design and architecture phases of your software development lifecycle on Day 1. This means discussing security at each layer of your stack and lifecycle, and positioning security and privacy as enablers to achieving business objectives.Architect controls for threats before you launch your LLM application, and consider whether the data and information you will use for model adaptation and fine-tuning warrants controls implementation in the research, development, and training environments. As part of quality assurance tests, introduce synthetic security threats (such as attempting to poison training data, or attempting to extract sensitive data through malicious prompt engineering) to test out your defenses and security posture on a regular basis.
Additionally, stakeholders should establish a consistent review cadence for production AI, ML, and generative AI workloads and set organizational priority on understanding trade-offs between human and machine control and error prior to launch. Validating and assuring that these trade-offs are respected in the deployed LLM applications will increase the likelihood of risk mitigation success.
Build generative AI applications on secure cloud foundations
At AWS, security is our top priority. AWS is architected to be the most secure global cloud infrastructure on which to build, migrate, and manage applications and workloads. This is backed by our deep set of over 300 cloud security tools and the trust of our millions of customers, including the most security-sensitive organizations like government, healthcare, and financial services. When building generative AI applications using LLMs on AWS, you gain security benefits from the secure, reliable, and flexible AWS Cloud computing environment.
Use an AWS global infrastructure for security, privacy, and compliance
When you develop data-intensive applications on AWS, you can benefit from an AWS global Region infrastructure, architected to provide capabilities to meet your core security and compliance requirements. This is reinforced by our AWS Digital Sovereignty Pledge, our commitment to offering you the most advanced set of sovereignty controls and features available in the cloud. We are committed to expanding our capabilities to allow you to meet your digital sovereignty needs, without compromising on the performance, innovation, security, or scale of the AWS Cloud. To simplify implementation of security and privacy best practices, consider using reference designs and infrastructure as code resources such as the AWS Security Reference Architecture (AWS SRA) and the AWS Privacy Reference Architecture (AWS PRA). Read more about architecting privacy solutions, sovereignty by design, and compliance on AWS and use services such as AWS Config, AWS Artifact, and AWS Audit Manager to support your privacy, compliance, audit, and observability needs.
Understand your security posture using AWS Well-Architected and Cloud Adoption Frameworks
AWS offers best practice guidance developed from years of experience supporting customers in architecting their cloud environments with the AWS Well-Architected Framework and in evolving to realize business value from cloud technologies with the AWS Cloud Adoption Framework (AWS CAF). Understand the security posture of your AI, ML, and generative AI workloads by performing a Well-Architected Framework review. Reviews can be performed using tools like the AWS Well-Architected Tool, or with the help of your AWS team through AWS Enterprise Support. The AWS Well-Architected Tool automatically integrates insights from AWS Trusted Advisor to evaluate what best practices are in place and what opportunities exist to improve functionality and cost-optimization. The AWS Well-Architected Tool also offers customized lenses with specific best practices such as the Machine Learning Lens for you to regularly measure your architectures against best practices and identify areas for improvement. Checkpoint your journey on the path to value realization and cloud maturity by understanding how AWS customers adopt strategies to develop organizational capabilities in the AWS Cloud Adoption Framework for Artificial Intelligence, Machine Learning, and Generative AI. You might also find benefit in understanding your overall cloud readiness by participating in an AWS Cloud Readiness Assessment. AWS offers additional opportunities for engagement—ask your AWS account team for more information on how to get started with the Generative AI Innovation Center.
Accelerate your security and AI/ML learning with best practices guidance, training, and certification
AWS also curates recommendations from Best Practices for Security, Identity, & Compliance and AWS Security Documentation to help you identify ways to secure your training, development, testing, and operational environments. If you’re just getting started, dive deeper on security training and certification, consider starting with AWS Security Fundamentals and the AWS Security Learning Plan. You can also use the AWS Security Maturity Model to help guide you finding and prioritizing the best activities at different phases of maturity on AWS, starting with quick wins, through foundational, efficient, and optimized stages. After you and your teams have a basic understanding of security on AWS, we strongly recommend reviewing How to approach threat modeling and then leading a threat modeling exercise with your teams starting with the Threat Modeling For Builders Workshop training program. There are many other AWS Security training and certification resources available.
Apply a defense-in-depth approach to secure LLM applications
Applying a defense-in-depth security approach to your generative AI workloads, data, and information can help create the best conditions to achieve your business objectives. Defense-in-depth security best practices mitigate many of the common risks that any workload faces, helping you and your teams accelerate your generative AI innovation. A defense-in-depth security strategy uses multiple redundant defenses to protect your AWS accounts, workloads, data, and assets. It helps make sure that if any one security control is compromised or fails, additional layers exist to help isolate threats and prevent, detect, respond, and recover from security events. You can use a combination of strategies, including AWS services and solutions, at each layer to improve the security and resiliency of your generative AI workloads.
Many AWS customers align to industry standard frameworks, such as the NIST Cybersecurity Framework. This framework helps ensure that your security defenses have protection across the pillars of Identify, Protect, Detect, Respond, Recover, and most recently added, Govern. This framework can then easily map to AWS Security services and those from integrated third parties as well to help you validate adequate coverage and policies for any security event your organization encounters.
Defense in depth: Secure your environment, then add enhanced AI/ML-specific security and privacy capabilities
A defense-in-depth strategy should start by protecting your accounts and organization first, and then layer on the additional built-in security and privacy enhanced features of services such as Amazon Bedrock and Amazon SageMaker. Amazon has over 30 services in the Security, Identity, and Compliance portfolio which are integrated with AWS AI/ML services, and can be used together to help secure your workloads, accounts, organization. To properly defend against the OWASP Top 10 for LLM, these should be used together with the AWS AI/ML services.
Start by implementing a policy of least privilege, using services like IAM Access Analyzer to look for overly permissive accounts, roles, and resources to restrict access using short-termed credentials. Next, make sure that all data at rest is encrypted with AWS KMS, including considering the use of CMKs, and all data and models are versioned and backed up using Amazon Simple Storage Service (Amazon S3) versioning and applying object-level immutability with Amazon S3 Object Lock. Protect all data in transit between services using AWS Certificate Manager and/or AWS Private CA, and keep it within VPCs using AWS PrivateLink. Define strict data ingress and egress rules to help protect against manipulation and exfiltration using VPCs with AWS Network Firewall policies. Consider inserting AWS Web Application Firewall (AWS WAF) in front to protect web applications and APIs from malicious bots, SQL injection attacks, cross-site scripting (XSS), and account takeovers with Fraud Control. Logging with AWS CloudTrail, Amazon Virtual Private Cloud (Amazon VPC) flow logs, and Amazon Elastic Kubernetes Service (Amazon EKS) audit logs will help provide forensic review of each transaction available to services such as Amazon Detective. You can use Amazon Inspector to automate vulnerability discovery and management for Amazon Elastic Compute Cloud (Amazon EC2) instances, containers, AWS Lambda functions, and identify the network reachability of your workloads. Protect your data and models from suspicious activity using Amazon GuardDuty’s ML-powered threat models and intelligence feeds, and enabling its additional features for EKS Protection, ECS Protection, S3 Protection, RDS Protection, Malware Protection, Lambda Protection, and more. You can use services like AWS Security Hub to centralize and automate your security checks to detect deviations from security best practices and accelerate investigation and automate remediation of security findings with playbooks. You can also consider implementing a zero trust architecture on AWS to further increase fine-grained authentication and authorization controls for what human users or machine-to-machine processes can access on a per-request basis. Also consider using Amazon Security Lake to automatically centralize security data from AWS environments, SaaS providers, on premises, and cloud sources into a purpose-built data lake stored in your account. With Security Lake, you can get a more complete understanding of your security data across your entire organization.
After your generative AI workload environment has been secured, you can layer in AI/ML-specific features, such as Amazon SageMaker Data Wrangler to identify potential bias during data preparation and Amazon SageMaker Clarify to detect bias in ML data and models. You can also use Amazon SageMaker Model Monitor to evaluate the quality of SageMaker ML models in production, and notify you when there is drift in data quality, model quality, and feature attribution. These AWS AI/ML services working together (including SageMaker working with Amazon Bedrock) with AWS Security services can help you identify potential sources of natural bias and protect against malicious data tampering. Repeat this process for each of the OWASP Top 10 for LLM vulnerabilities to ensure you’re maximizing the value of AWS services to implement defense in depth to protect your data and workloads.
As AWS Enterprise Strategist Clarke Rodgers wrote in his blog post “CISO Insight: Every AWS Service Is A Security Service”, “I would argue that virtually every service within the AWS cloud either enables a security outcome by itself, or can be used (alone or in conjunction with one or more services) by customers to achieve a security, risk, or compliance objective.” And “Customer Chief Information Security Officers (CISOs) (or their respective teams) may want to take the time to ensure that they are well versed with all AWS services because there may be a security, risk, or compliance objective that can be met, even if a service doesn’t fall into the ‘Security, Identity, and Compliance’ category.”
Layer defenses at trust boundaries in LLM applications
When developing generative AI-based systems and applications, you should consider the same concerns as with any other ML application, as mentioned in the MITRE ATLAS Machine Learning Threat Matrix, such as being mindful of software and data component origins (such as performing an open source software audit, reviewing software bill of materials (SBOMs), and analyzing data workflows and API integrations) and implementing necessary protections against LLM supply chain threats. Include insights from industry frameworks, and be aware of ways to use multiple sources of threat intelligence and risk information to adjust and extend your security defenses to account for AI, ML, and generative AI security risks that are emergent and not included in traditional frameworks. Seek out companion information on AI-specific risks from industry, defense, governmental, international, and academic sources, because new threats emerge and evolve in this space regularly and companion frameworks and guides are updated frequently. For example, when using a Retrieval Augmented Generation (RAG) model, if the model doesn’t include the data it needs, it may request it from an external data source for using during inferencing and fine-tuning. The source that it queries may be outside of your control, and can be a potential source of compromise in your supply chain. A defense-in-depth approach should be extended towards external sources to establish trust, authentication, authorization, access, security, privacy, and accuracy of the data it is accessing. To dive deeper, read “Build a secure enterprise application with Generative AI and RAG using Amazon SageMaker JumpStart”
Analyze and mitigate risk in your LLM applications
In this section, we analyze and discuss some risk mitigation techniques based on trust boundaries and interactions, or distinct areas of the workload with similar appropriate controls scope and risk profile. In this sample architecture of a chatbot application, there are five trust boundaries where controls are demonstrated, based on how AWS customers commonly build their LLM applications. Your LLM application may have more or fewer definable trust boundaries. In the following sample architecture, these trust boundaries are defined as:
- User interface interactions (request and response)
- Application interactions
- Model interactions
- Data interactions
- Organizational interactions and use
User interface interactions: Develop request and response monitoring
Detect and respond to cyber incidents related to generative AI in a timely manner by evaluating a strategy to address risk from the inputs and outputs of the generative AI application. For example, additional monitoring for behaviors and data outflow may need to be instrumented to detect sensitive information disclosure outside your domain or organization, in the case that it is used in the LLM application.
Generative AI applications should still uphold the standard security best practices when it comes to protecting data. Establish a secure data perimeter and secure sensitive data stores. Encrypt data and information used for LLM applications at rest and in transit. Protect data used to train your model from training data poisoning by understanding and controlling which users, processes, and roles are allowed to contribute to the data stores, as well as how data flows in the application, monitor for bias deviations, and using versioning and immutable storage in storage services such as Amazon S3. Establish strict data ingress and egress controls using services like AWS Network Firewall and AWS VPCs to protect against suspicious input and the potential for data exfiltration.
During the training, retraining, or fine-tuning process, you should be aware of any sensitive data that is utilized. After data is used during one of these processes, you should plan for a scenario where any user of your model suddenly becomes able to extract the data or information back out by utilizing prompt injection techniques. Understand the risks and benefits of using sensitive data in your models and inferencing. Implement robust authentication and authorization mechanisms for establishing and managing fine-grained access permissions, which don’t rely on LLM application logic to prevent disclosure. User-controlled input to a generative AI application has been demonstrated under some conditions to be able to provide a vector to extract information from the model or any non-user-controlled parts of the input. This can occur via prompt injection, where the user provides input that causes the output of the model to deviate from the expected guardrails of the LLM application, including providing clues to the datasets that the model was originally trained on.
Implement user-level access quotas for users providing input and receiving output from a model. You should consider approaches that don’t allow anonymous access under conditions where the model training data and information is sensitive, or where there is risk from an adversary training a facsimile of your model based on their input and your aligned model output. In general, if part of the input to a model consists of arbitrary user-provided text, consider the output to be susceptible to prompt injection, and accordingly ensure use of the outputs includes implemented technical and organizational countermeasures to mitigate insecure output handling, excessive agency, and overreliance. In the example earlier related to filtering for malicious input using AWS WAF, consider building a filter in front of your application for such potential misuse of prompts, and develop a policy for how to handle and evolve those as your model and data grows. Also consider a filtered review of the output before it is returned to the user to ensure it meets quality, accuracy, or content moderation standards. You may want to further customize this for your organization’s needs with an additional layer of control on inputs and outputs in front of your models to mitigate suspicious traffic patterns.
Application interactions: Application security and observability
Review your LLM application with attention to how a user could utilize your model to bypass standard authorization to a downstream tool or toolchain that they don’t have authorization to access or use. Another concern at this layer involves accessing external data stores by using a model as an attack mechanism using unmitigated technical or organizational LLM risks. For example, if your model is trained to access certain data stores that could contain sensitive data, you should ensure that you have proper authorization checks between your model and the data stores. Use immutable attributes about users that don’t come from the model when performing authorization checks. Unmitigated insecure output handling, insecure plugin design, and excessive agency can create conditions where a threat actor may use a model to trick the authorization system into escalating effective privileges, leading to a downstream component believing the user is authorized to retrieve data or take a specific action.
When implementing any generative AI plugin or tool, it is imperative to examine and comprehend the level of access being granted, as well as scrutinize the access controls that have been configured. Using unmitigated insecure generative AI plugins may render your system susceptible to supply chain vulnerabilities and threats, potentially leading to malicious actions, including running remote code.
Model interactions: Model attack prevention
You should be aware of the origin of any models, plugins, tools, or data you use, in order to evaluate and mitigate against supply chain vulnerabilities. For example, some common model formats permit the embedding of arbitrary runnable code in the models themselves. Use package mirrors, scanning, and additional inspections as relevant to your organizations security goals.
The datasets you train and fine-tune your models on must also be reviewed. If you further automatically fine-tune a model based on user feedback (or other end-user-controllable information), you must consider if a malicious threat actor could change the model arbitrarily based on manipulating their responses and achieve training data poisoning.
Data interactions: Monitor data quality and usage
Generative AI models such as LLMs generally work well because they have been trained on a large amount of data. Although this data helps LLMs complete complex tasks, it also can expose your system to risk of training data poisoning, which occurs when inappropriate data is included or omitted inside a training dataset that can alter a model’s behavior. To mitigate this risk, you should look at your supply chain and understand the data review process for your system before it’s used inside your model. Although the training pipeline is a prime source for data poisoning, you should also look at how your model gets data, such as in a RAG model or data lake, and if the source of that data is trusted and protected. Use AWS Security services such as AWS Security Hub, Amazon GuardDuty, and Amazon Inspector to help continuously monitor for suspicious activity in Amazon EC2, Amazon EKS, Amazon S3, Amazon Relational Database Service (Amazon RDS), and network access that may be indicators of emerging threats, and use Detective to visualize security investigations. Also consider using services such as Amazon Security Lake to accelerate security investigations by creating a purpose-built data lake to automatically centralize security data from AWS environments, SaaS providers, on premises, and cloud sources which contribute to your AI/ML workloads.
Organizational interactions: Implement enterprise governance guardrails for generative AI
Identify risks associated with the use of generative AI for your businesses. You should build your organization’s risk taxonomy and conduct risk assessments to make informed decisions when deploying generative AI solutions. Develop a business continuity plan (BCP) that includes AI, ML, and generative AI workloads and that can be enacted quickly to replace the lost functionality of an impacted or offline LLM application to meet your SLAs.
Identify process and resource gaps, inefficiencies, and inconsistencies, and improve awareness and ownership across your business. Threat model all generative AI workloads to identify and mitigate potential security threats that may lead to business-impacting outcomes, including unauthorized access to data, denial of service, and resource misuse. Take advantage of the new AWS Threat Composer Modeling Tool to help reduce time-to-value when performing threat modeling. Later in your development cycles, consider including introducing security chaos engineering fault injection experiments to create real-world conditions to understand how your system will react to unknowns and build confidence in the system’s resiliency and security.
Include diverse perspectives in developing security strategies and risk management mechanisms to ensure adherence and coverage for AI/ML and generative security across all job roles and functions. Bring a security mindset to the table from the inception and research of any generative AI application to align on requirements. If you need extra assistance from AWS, ask your AWS account manager to make sure that there is equal support by requesting AWS Solutions Architects from AWS Security and AI/ML to help in tandem.
Ensure that your security organization routinely takes actions to foster communication around both risk awareness and risk management understanding among generative AI stakeholders such as product managers, software developers, data scientists, and executive leadership, allowing threat intelligence and controls guidance to reach the teams that may be impacted. Security organizations can support a culture of responsible disclosure and iterative improvement by participating in discussions and bringing new ideas and information to generative AI stakeholders that relate to their business objectives. Learn more about our commitment to Responsible AI and additional responsible AI resources to help our customers.
Gain advantage in enabling better organizational posture for generative AI by unblocking time to value in the existing security processes of your organization. Proactively evaluate where your organization may require processes that are overly burdensome given the generative AI security context and refine these to provide developers and scientists a clear path to launch with the correct controls in place.
Assess where there may be opportunities to align incentives, derisk, and provide a clear line of sight on the desired outcomes. Update controls guidance and defenses to meet the evolving needs of AI/ML and generative AI application development to reduce confusion and uncertainty that can cost development time, increase risk, and increase impact.
Ensure that stakeholders who are not security experts are able to both understand how organizational governance, policies, and risk management steps apply to their workloads, as well as apply risk management mechanisms. Prepare your organization to respond to realistic events and scenarios that may occur with generative AI applications, and ensure that generative AI builder roles and response teams are aware of escalation paths and actions in case of concern for any suspicious activity.
Conclusion
To successfully commercialize innovation with any new and emerging technology requires starting with a security-first mindset, building on a secure infrastructure foundation, and thinking about how to further integrate security at each level of the technology stack early with a defense-in-depth security approach. This includes interactions at multiple layers of your technology stack, and integration points within your digital supply chain, to ensure organizational resiliency. Although generative AI introduces some new security and privacy challenges, if you follow fundamental security best practices such as using defense-in-depth with layered security services, you can help protect your organization from many common issues and evolving threats. You should implement layered AWS Security services across your generative AI workloads and larger organization, and focus on integration points in your digital supply chains to secure your cloud environments. Then you can use the enhanced security and privacy capabilities in AWS AI/ML services such as Amazon SageMaker and Amazon Bedrock to add further layers of enhanced security and privacy controls to your generative AI applications. Embedding security from the start will make it faster, easier, and more cost-effective to innovate with generative AI, while simplifying compliance. This will help you increase controls, confidence, and observability to your generative AI applications for your employees, customers, partners, regulators, and other concerned stakeholders.
Additional references
- Industry standard frameworks for AI/ML-specific risk management and security:
About the authors
Christopher Rae is a Principal Worldwide Security GTM Specialist focused on developing and executing strategic initiatives that accelerate and scale adoption of AWS security services. He is passionate about the intersection of cybersecurity and emerging technologies, with 20+ years of experience in global strategic leadership roles delivering security solutions to media, entertainment, and telecom customers. He recharges through reading, traveling, food and wine, discovering new music, and advising early-stage startups.
Elijah Winter is a Senior Security Engineer in Amazon Security, holding a BS in Cyber Security Engineering and infused with a love for Harry Potter. Elijah excels in identifying and addressing vulnerabilities in AI systems, blending technical expertise with a touch of wizardry. Elijah designs tailored security protocols for AI ecosystems, bringing a magical flair to digital defenses. Integrity driven, Elijah has a security background in both public and commercial sector organizations focused on protecting trust.
Ram Vittal is a Principal ML Solutions Architect at AWS. He has over 3 decades of experience architecting and building distributed, hybrid, and cloud applications. He is passionate about building secure and scalable AI/ML and big data solutions to help enterprise customers with their cloud adoption and optimization journey to improve their business outcomes. In his spare time, he rides his motorcycle and walks with his 3-year-old Sheepadoodle!
Navneet Tuteja is a Data Specialist at Amazon Web Services. Before joining AWS, Navneet worked as a facilitator for organizations seeking to modernize their data architectures and implement comprehensive AI/ML solutions. She holds an engineering degree from Thapar University, as well as a master’s degree in statistics from Texas A&M University.
Emily Soward is a Data Scientist with AWS Professional Services. She holds a Master of Science with Distinction in Artificial Intelligence from the University of Edinburgh in Scotland, United Kingdom with emphasis on Natural Language Processing (NLP). Emily has served in applied scientific and engineering roles focused on AI-enabled product research and development, operational excellence, and governance for AI workloads running at organizations in the public and private sector. She contributes to customer guidance as an AWS Senior Speaker and recently, as an author for AWS Well-Architected in the Machine Learning Lens.
Mixed-input matrix multiplication performance optimizations
AI-driven technologies are weaving themselves into the fabric of our daily routines, with the potential to enhance our access to knowledge and boost our overall productivity. The backbone of these applications lies in large language models (LLMs). LLMs are memory-intensive and typically require specialized hardware accelerators to efficiently deliver tens of exaflops of computing power. This blog post shows how we can start addressing the computational challenges by utilizing memory more effectively.
The bulk of an LLM’s memory and compute are consumed by weights in matrix multiplication operations. Using narrower data types reduces memory consumption. For example, storing weights in the 8-bit integer (i.e., U8 or S8) data type reduces the memory footprint by 4× relative to single-precision (F32) and 2× relative to half-precision (F16) or bfloat16 (BF16). Furthermore, previous work has shown that LLM models running matrix multiplications with weights in S8 and input in F16 (preserving higher precision of the user-input) is an effective method for increasing the efficiency with acceptable trade-offs in accuracy. This technique is known as weight-only quantization and requires efficient implementation of matrix multiplication with mixed-inputs, e.g., half-precision input multiplied with 8-bits integer. Hardware accelerators, including GPUs, support a fixed set of data types, and thus, mixed-input matrix multiplication requires software transformations to map to the hardware operations.
To that end, in this blog we focus on mapping mixed-input matrix multiplication onto the NVIDIA Ampere architecture. We present software techniques addressing data type conversion and layout conformance to map mixed-input matrix multiplication efficiently onto hardware-supported data types and layouts. Our results show that the overhead of additional work in software is minimal and enables performance close to the peak hardware capabilities. The software techniques described here are released in the open-source NVIDIA/CUTLASS repository.
![]() |
Memory footprint for an 175B parameter LLM model with various data types formats. |
The matrix-multiply-accumulate operation
Modern AI hardware accelerators such as Google’s TPU and NVIDIA’s GPU multiply matrices natively in the hardware by targeting Tensor Cores, which are specialized processing elements to accelerate matrix operations, particularly for AI workloads. In this blog, we focus on NVIDIA Ampere Tensor Cores, which provide the matrix-multiply-accumulate (mma
) operation. For the rest of the blog the reference to mma
is for Ampere Tensor Cores. The supported data types, shapes, and data layout of the two input matrices (called operands) for the mma
operation are fixed in hardware. This means that matrix multiplications with various data types and larger shapes are implemented in the software by tiling the problem onto hardware-supported data types, shapes, and layouts.
The Tensor Core mma
operation is defined by specifying two input matrices (e.g., A & B, shown below) to produce a result matrix, C. The mma
operation natively supports mixed-precision. Mixed-precision Tensor Cores allow mixing input (A and B) data type with the result (C) data type. In contrast, mixed-input matrix multiplication involves mixing the input data types, and it is not supported by the hardware, so it needs to be implemented in the software.
![]() |
Tensor Core operation of M-by-N-by-K on input matrix A of M-by-K and matrix B of K-by-N produces output matrix C of M-by-N. |
Challenges of mixed-input matrix multiplication
To simplify the discussion, we restrict to a specific example of mixed-input matrix multiplication: F16 for user input and U8 for the model weights (written as F16 * U8). The techniques described here work for various combinations of mixed-input data types.
A GPU programmer can access a hierarchy of memory, including global memory, shared memory, and registers, which are arranged in order of decreasing capacity but increasing speed. NVIDIA Ampere Tensor Core mma
operations consume input matrices from registers. Furthermore, input and output matrices are required to conform to a layout of data within a group of 32 threads known as a warp. The supported data type and layout within a warp are fixed for an mma
operation, so to implement mixed-input multiplication efficiently, it is necessary to solve the challenges of data type conversion and layout conformance in software.
Data type conversion
The mma
operation requires two input matrices with the same data type. Thus, mixed-input matrix multiplication, where one of the operands is stored in U8 in global memory and other in F16, requires a data type conversion from U8 to F16. The conversion will bring two operands to F16, mapping the mixed-input matrix multiplication to hardware-supported mixed-precision Tensor Cores. Given the large number of weights, there are a large number of such operations, and our techniques show how to reduce their latency and improve performance.
Layout conformance
The mma
operation also requires the layout of two input matrices, within the registers of a warp, to be conformat with hardware specification. The layout for the input matrix B of U8 data type in mixed-input matrix multiplication (F16 * U8) needs to conform with the converted F16 data type. This is called layout conformance and needs to be achieved in the software.
The figure below shows an mma
operation consuming matrix A and matrix B from registers to produce matrix C in registers, distributed across one warp. The thread T0 is highlighted and zoomed in to show the weight matrix B goes through data type conversion and needs a layout conformance to be able to map to the hardware-supported Tensor Core operation.
![]() |
The mapping of mixed-input (F32 = F16 * U8) operation in software to natively supported warp-level Tensor Cores in hardware (F32 = F16 * F16). (Original figure source Developing CUDA kernels to push Tensor Cores to the Absolute Limit on NVIDIA A100.) |
Software strategies addressing challenges
A typical data type conversion involves a sequence of operations on 32-bit registers, shown below. Each rectangular block represents a register and the adjoining text are the operations. The entire sequence shows the conversion from 4xU8 to 2x(2xF16). The sequence involves roughly 10 operations.
![]() |
NumericArrayConvertor from 4xU8 to 2x(2xF16) in 32-bit registers. |
There are many ways of achieving layout conformance. Two of the existing solutions are:
- Narrower bitwidth shared memory loads: In this approach, threads issue narrow bitwidth memory loads moving the U8 data from shared memory to registers. This results in two 32-bit registers, with each register containing 2xF16 values (shown above for the matrix B’s thread T0). The narrower shared memory load achieves layout conformance directly into registers without needing any shuffles; however, it does not utilize the full shared memory bandwidth.
- Pre-processing in global memory: An alternative strategy involves rearranging the data within the global memory (one level above the shared memory in memory hierarchy), allowing wider shared memory loads. This approach maximizes the shared memory bandwidth utilization and ensures that the data is loaded in a conformant layout directly in the registers. Although the rearrangement process can be executed offline prior to the LLM deployment, ensuring no impact on the application performance, it introduces an additional, non-trivial hardware-specific pre-processing step that requires an extra program to rearrange the data. NVIDIA/FasterTransformer adopts this method to effectively address layout conformance challenges.
Optimized software strategies
To further optimize and reduce the overhead of data type conversion and layout conformance, we have implemented FastNumericArrayConvertor
and FragmentShuffler
, respectively.
FastNumericArrayConvertor
operates on 4xU8 in 32-bit registers without unpacking individual 1xU8 values. Furthermore, it uses less expensive arithmetic operations which reduces the number of instructions and increases the speed of the conversion.
The conversion sequence for U8-to-F16 is shown below. The operations use packed 32b registers, avoiding explicit unpacking and packing. FastNumericArrayConvertor
uses the permute byte
to rearrange bytes of 4xU8 into two registers. Additionally, FastNumericArrayConvertor
does not use expensive integer to floating-point conversion instructions and employs vectorized operations to obtain the packed results in two 32-bit registers containing 2x(2xF16) values. The FastNumericArrayConvertor
for U8-to-F16 approximately uses six operations, a 1.6× reduction relative to the approach shown above.
![]() |
FastNumericArrayConvertor utilizes permute bytes and packed arithmetic, reducing the number of instructions in the data type conversion. |
FragmentShuffler
handles the layout conformance by shuffling data in a way that allows the use of wider bitwidth load operation, increasing shared memory bandwidth utilization and reducing the total number of operations.
NVIDIA Ampere architecture provides a load matrix instruction (ldmatrix
). The ldmatrix
is a warp-level operation, where 32 threads of a warp move the data from shared memory to registers in the shape and layout that mma
matrix A and B consume. The use of ldmatrix
reduces the number of load instructions and increases the memory bandwidth utilization. Since the ldmatrix
instruction moves U8 data to registers, the layout after the load conforms with U8*U8 mma
operation, and not with F16*F16 mma
operation. We implemented FragmentShuffler
to rearrange the data within registers using shuffle (shfl.sync)
operations to achieve the layout conformance.
The most significant contribution of this work is to achieve layout conformance through register shuffles, avoiding offline pre-processing in global memory or narrower bitwidth shared memory loads. Furthermore, we provide implementations for FastNumericArrayConvertor
covering data type conversion from U8-to-F16, S8-to-F16, U8-to-BF16, and S8-to-BF16.
Performance results
We measured the performance of eight mixed-input variants of our method (shown below in blue and red; varying the data types of matrix A and B) and two mixed-precision data types (shown in green) on an NVIDIA A100 SXM chip. The performance results are shown in FLOPS (higher is better). Notably, the first eight matrix-multipications require additional operations relative to the last two, because the mixed-precision variants directly target hardware-accelerated Tensor Core operations and do not need data type conversion and layout conformance. Even so, our approach demonstrates mixed-input matrix multiplication performance only slightly below or on par with mixed-precision.
![]() |
Mixed-input matrix multiplication performance on NVIDIA A100 40GB SMX4 chip for a compute-bound matrix problem shape m=3456, n=4096, k=2048. |
Acknowledgements
We would like to mention several folks who have contributed through technical brainstorming and improving the blog post including, Quentin Colombet, Jacques Pienaar, Allie Culp, Calin Cascaval, Ashish Gondimalla, Matt Walsh, Marek Kolodziej, and Aman Bhatia. We would like to thank our NVIDIA partners Rawn Henry, Pradeep Ramani, Vijay Thakkar, Haicheng Wu, Andrew Kerr, Matthew Nicely, and Vartika Singh.
Amazon and IIT Bombay announce inaugural award recipients
Amazon IIT–Bombay AI-ML Initiative seeks to advance artificial intelligence and machine learning research within speech, language, and multimodal-AI domains.Read More
Deploy a Microsoft Teams gateway for Amazon Q, your business expert
Amazon Q is a new generative AI-powered application that helps users get work done. Amazon Q can become your tailored business expert and let you discover content, brainstorm ideas, or create summaries using your company’s data safely and securely. You can use Amazon Q to have conversations, solve problems, generate content, gain insights, and take action by connecting to your company’s information repositories, code, data, and enterprise systems. For more information, see Introducing Amazon Q, a new generative AI-powered assistant (preview).
In this post, we show you how to bring Amazon Q, your business expert, to users in Microsoft Teams. (If you use Slack, refer to Deploy a Slack gateway for Amazon Q, your business expert.)
You’ll be able converse with Amazon Q business expert using Teams direct messages (DMs) to ask questions and get answers based on company data, get help creating new content such as email drafts, summarize attached files, and perform tasks.
You can also invite Amazon Q business expert to participate in your Teams channels. In a channel, users can ask Amazon Q business expert questions in a new message, or tag it in an existing thread at any point, to provide additional data points, resolve a debate, or summarize the conversation and capture the next steps.
Solution overview
Amazon Q business expert is amazingly powerful. Check out the following demo—seeing is believing!
In the demo, our Amazon Q business expert application is populated with some Wikipedia pages. You can populate your Amazon Q business expert application with your own company’s documents and knowledge base articles, so it will be able to answer your specific questions!
Everything you need is provided as open source in our GitHub repo.
In this post, we walk you through the process to deploy Amazon Q business expert in your AWS account and add it to Microsoft Teams. When you’re done, you’ll wonder how you ever managed without it!
The following are some of the things it can do:
- Respond to messages – In DMs, it responds to all messages. In channels, it responds only to @mentions and responds in a conversation thread.
- Render answers containing markdown – This includes headings, lists, bold, italics, tables, and more.
- Track sentiment – It provides thumbs up and thumbs down buttons to track user sentiment.
- Provide source attribution – It provides references and hyperlinks to sources used by Amazon Q business expert.
- Understand conversation context – It tracks the conversation and responds based on the context.
- Stay aware of multiple users – When it’s tagged in a thread, it knows who said what, and when, so it can contribute in context and accurately summarize the thread when asked.
- Process attached files – It can process up to five attached files for document question answering, summaries, and more.
- Start new conversations – You can reset and start new conversations in DM chats by using
/new_conversation
.
In the following sections, we show how to deploy the project to your own AWS account and Teams account, and start experimenting!
Prerequisites
You need to have an AWS account and an AWS Identity and Access Management (IAM) role and user with permissions to create and manage the necessary resources and components for this application. If you don’t have an AWS account, see How do I create and activate a new Amazon Web Services account?
You also need to have an existing, working Amazon Q business expert application. If you haven’t set one up yet, see Creating an Amazon Q application.
Lastly, you need a Microsoft account and a Microsoft Teams subscription to create and publish the app using the steps outlined in this post. If you don’t have these, see if your company can create sandboxes for you to experiment, or create a new account and trial subscription as needed to complete the steps.
Deploy the solution resources
We’ve provided pre-built AWS CloudFormation templates that deploy everything you need in your AWS account.
If you’re a developer and you want to build, deploy, or publish the solution from code, refer to the Developer README.
Complete the following steps to launch the CloudFormation stack:
- Log in to the AWS Management Console.
- Choose one of the following Launch Stack buttons for your desired AWS Region to open the AWS CloudFormation console and create a new stack.
Region | Launch Stack |
---|---|
N. Virginia (us-east-1 ) |
![]() |
Oregon (us-west-2 ) |
![]() |
- For Stack name, enter a name for your app (for example,
AMAZON-Q-TEAMS-GATEWAY
). - For AmazonQAppId, enter your existing Amazon Q business expert application ID (for example,
80xxxxx9-7xx3-4xx0-bxx4-5baxxxxx2af5
). You can copy it from the Amazon Q business expert console. - For AmazonQRegion, choose the Region where you created your Amazon Q business expert application (us-east-1 or us-west-2).
- For AmazonQUserId, enter an Amazon Q business expert user ID email address (leave blank to use a Teams user email as the user ID).
- For ContextDaysToLive, enter the length of time to keep conversation metadata cached in Amazon DynamoDB (you can leave this as the default).
When your CloudFormation stack status is CREATE_COMPLETE, choose the Outputs tab, and keep it open—you’ll need it in later steps.
Register a new app in the Microsoft Azure portal
Complete the following steps to register a new app in the Microsoft Azure portal:
- Go to the Azure Portal and log in with your Microsoft account.
- Choose New registration.
- For Name, provide the name for your app. You can keep things simple by using the stack name you used for the CloudFormation stack.
- For Who can use this application or access this API?, choose Accounts in this organizational directory only (AWS only – Single tenant).
- Choose Register.
- Note down the Application (client) ID value and the Directory (tenant) ID from the Overview page. You’ll need them later when asked for
MicrosoftAppId
andMicrosoftAppTenantId
.
- Choose Select API permissions in the navigation pane.
- Choose Add a permission.
- Choose Microsoft Graph.
- Choose Application permissions.
- Select User.Read.All.
- Select ChannelMessage.Read.All.
- Select Team.ReadBasic.All.
- Select Files.Read.All.
- Choose Add permissions. This permission allows the app to read data in your organization’s directory about the signed-in user.
- Use the options menu (three dots) on the right to choose Remove permission.
- Remove the original User.Read – Delegated permission.
- Choose Grant admin consent for Default Directory.
- Choose Certificates & secrets in the navigation pane.
- Choose New client secret.
- For Description, provide a value, such as
description of my client secret
. - Choose a value for Expires. Note that in production, you’ll need to manually rotate your secret before it expires.
- Choose Add.
- Note down the value for your new secret. You’ll need it later when asked for
MicrosoftAppPassword
.
- Optionally, choose Owners to add any additional owners for the application.
Register your new app in the Microsoft Bot Framework
Complete the following steps to register your app in the Microsoft Bot Framework:
- Go to the Microsoft Bot Framework and log in with your Microsoft account.
- Optionally, you can create and upload a custom icon for your new Amazon Q business expert bot. For example, we created the following using Amazon Bedrock image playground.
- Enter your preferred display name, bot handle, and description.
- For Messaging endpoint, copy and paste the value of
TeamsEventHandlerApiEndpoint
from your stack Outputs tab. - Do not select Enable Streaming Endpoint.
- For App type, choose Single Tenant.
- For Paste your app ID below to continue, enter the
MicrosoftAppId
value you noted earlier. - For App Tenant ID, enter the
MicrosoftAppTenantId
value you noted earlier. - Leave the other values as they are, agree to the terms, and choose Register.
- On the Channels page, under Add a featured channel, choose Microsoft Teams.
- Choose Microsoft Teams Commercial (most common), then choose Save.
- Agree to the Terms of Service and choose Agree.
Configure your secrets in AWS
Let’s configure your Teams secrets in order to verify the signature of each request and post on behalf of your Amazon Q business expert bot.
In this example, we are not enabling Teams token rotation. You can enable it for a production app by implementing rotation via AWS Secrets Manager. Create an issue (or, better yet, a pull request) in the GitHub repo if you want this feature added to a future version.
Complete the following steps to configure a secret in Secrets Manager:
- On the AWS CloudFormation console, navigate to your stack Outputs tab and choose the link for
TeamsSecretConsoleUrl
to be redirected to the Secrets Manager console. - Choose Retrieve secret value.
- Choose Edit.
- Replace the values of
MicrosoftAppId
,MicrosoftAppPassword
, andMicrosoftAppTenantId
with the values you noted in the previous steps.
Deploy your app into Microsoft Teams
Complete the following steps to deploy the app to Teams:
- Go to the Developer Portal for Teams and log in with your Microsoft Teams user account.
- Choose Apps in the navigation pane, then choose New app.
- For Name, enter your bot name.
- Enter a name for Full name and both short and full descriptions (you can use the bot name for them all if you want, just don’t leave them empty).
- Enter values for Developer information and App URLs. For testing, you can make up values, and URLs like
https://www.anycompany.com/
. Use real ones for production. - For Application (client) ID*, enter the value of
MicrosoftAppId
from earlier. - Choose Save.
- Under Branding, you can upload AI-generated icons, or different icons, or none at all, it’s up to you. The following are some examples:
- Under App features, choose Bot.
- Select Enter a bot ID, and enter the
MicrosoftAppId
value from the earlier steps. - Under What can your bot do?, select Upload and download files.
- Under Select the scopes in which people can use this command, select Personal, Team, and Group chat.
- Choose Save.
- Select Enter a bot ID, and enter the
- Choose Publish.
- Choose Download the app package to download a .zip file to your computer.
- Choose Preview in Teams to launch Microsoft Teams (work or school) app.
- In the navigation pane, choose Apps, then Manage your apps, then Upload an app.
- Choose Upload an app to your orgs app catalog, and select the .zip file you downloaded. This adds the app to Teams.
- Select the card for your new app, choose Add, and wait for it to complete (10–20 seconds).
Add your bot to one or more teams
Complete the following step to add your bot to a team:
- In the Teams app, select your team and choose Manage team.
- On the Apps tab, choose the new Amazon Q business expert app, and choose Add.
Now you can test your bot in Microsoft Teams!
Start using Amazon Q business expert
Complete the following steps to start using Amazon Q business expert in Teams:
- Open your Teams client.
- Under Apps, add your new Amazon Q business expert app to a chat.
- Optionally, add your Amazon Q business expert app to one or more Teams channels.
- In the app DM chat, enter
Hello
.
You have now deployed a powerful new AI assistant into your sandbox Teams environment.
Play with it, try all the features discussed in this post, and copy the things you saw in the demo video. Most importantly, you can ask about topics related to the documents that you have ingested into your own Amazon Q business expert application. But don’t stop there. You can find additional ways to make it useful, and when you do, let us know by posting a comment.
Once you are convinced how useful it is, talk to your Teams admins (show them this post) and work with them to deploy it in your company’s Teams organizations. Your fellow employees will thank you!
Clean up
When you’re finished experimenting with this solution, delete your app in Microsoft Teams, Bot Framework, and Azure portal. Then clean up your AWS resources by opening the AWS CloudFormation console and deleting the AMAZON-Q-TEAMS-GATEWAY
stack that you deployed. This deletes the resources that you created by deploying the solution.
Conclusions
The sample Amazon Q business expert Teams application discussed in this post is provided as open source—you can use it as a starting point for your own solution, and help us make it better by contributing back fixes and features via GitHub pull requests. Explore the code, choose Watch in the GitHub repo to be notified of new releases, and check back for the latest updates. We’d also love to hear your suggestions for improvements and features.
For more information on Amazon Q business expert, refer to the Amazon Q (For Business Use) Developer Guide.
About the Authors
Gary Benattar is a Senior Software Development Manager in AWS HR. Gary started at Amazon in 2012 as an intern, focusing on building scalable, real-time outlier detection systems. He worked in Seattle and Luxembourg and is now based in Tel Aviv, Israel, where he dedicates his time to building software to revolutionize the future of Human Resources. He co-founded a startup, Zengo, with a focus on making digital wallets secure through multi-party computation. He received his MSc in Software Engineering from Sorbonne University in Paris.
Bob Strahan is a Principal Solutions Architect in the AWS Language AI Services team.
Sharper Image: GeForce NOW Update Delivers Stunning Visuals to Android Devices
This GFN Thursday levels up PC gaming on mobile with higher-resolution support on Android devices.
This week also brings 10 new games to the GeForce NOW library, including Enshrouded.
Pixel Perfect
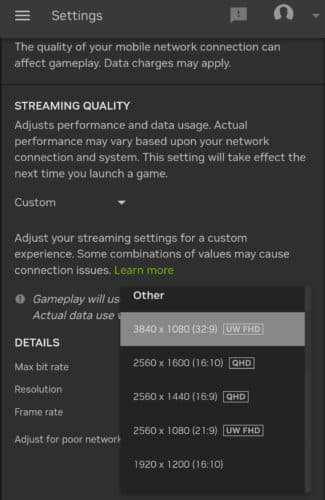
GeForce NOW transforms nearly any device into a high-powered PC gaming rig, and members streaming on Android can now access that power from the palms of their hands. The GeForce NOW Android app, rolling out now to members, unlocks a new level of visual quality for Ultimate members gaming on mobile, with improved support for streaming up to 1440p resolution at 120 frames per second.
Explore the vibrant neon landscapes of Cyberpunk 2077, stream triple-A titles like Baldur’s Gate 3 and Monster Hunter: World, and play the latest releases in the cloud, including Prince of Persia: The Lost Crown and Exoprimal — all on the go with higher resolutions for more immersive gameplay.
Ultimate members can stream these and over 1,800 titles from the GeForce NOW library on select 120Hz Android phones and tablets at pixel-perfect quality. Plus, they can take gameplay even further with eight-hour sessions and tap GeForce RTX 4080-powered servers for faster access to their gaming libraries.
Sign up for an Ultimate membership today and check out this article for more details on how to set up Android devices for PC gaming on the go.
Got Games?
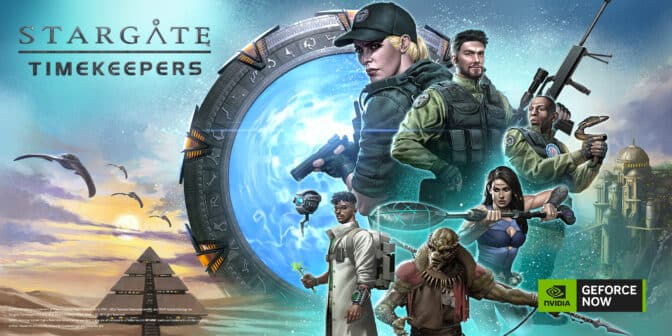
Lead a team of specialists through a story-driven campaign set in the SG-1 universe of Stargate: Timekeepers from Slitherine Ltd. Sneak characters behind enemy lines, use their unique skills, craft the perfect plan to unravel a time-loop mystery, and defeat the Goa’uld threat. It’s available to stream from the cloud this week.
More titles joining the cloud this week include:
- Stargate: Timekeepers (New release on Steam, Jan. 23)
- Enshrouded (New release on Steam, Jan. 24)
- Firefighting Simulator – The Squad (Steam)
- Metal: Hellsinger (Xbox, available on the Microsoft Store)
- Road 96: Mile 0 (Xbox, available on the Microsoft Store)
- Shadow Tactics: Blades of the Shogun (Steam)
- Shadow Tactics: Blades of the Shogun – Aiko’s Choice (Steam)
- Solasta: Crown of the Magister (Steam)
- Tails Noir (Xbox, available on the Microsoft Store)
- Wobbly Life (Steam)
Games from Spike Chunsoft will be removed from the GeForce NOW library at the request of the publisher. Fourteen titles are leaving on Friday, Feb. 2, so be sure to catch them before they go:
- 428 Shibuya Scramble
- AI: The Somnium Files
- Conception PLUS: Maidens of the Twelve Stars
- Danganronpa: Trigger Happy Havoc
- Danganronpa 2: Goodbye Despair
- Danganronpa V3: Killing Harmony
- Danganronpa Another Episode: Ultra Despair Girls
- Fire Pro Wrestling World
- Re: ZERO – Starting Life in Another World – The Prophecy of the Throne
- RESEARCH and DESTROY
- Shiren the Wanderer: The Tower of Fortune and the Dice of Fate
- STEINS;GATE
- Zanki Zero: Last Beginning
- Zero Escape: The Nonary Games
What are you planning to play this weekend? Let us know on X or in the comments below.
oh, you dropped this:
—
NVIDIA GeForce NOW (@NVIDIAGFN) January 24, 2024
Abstracts: January 25, 2024
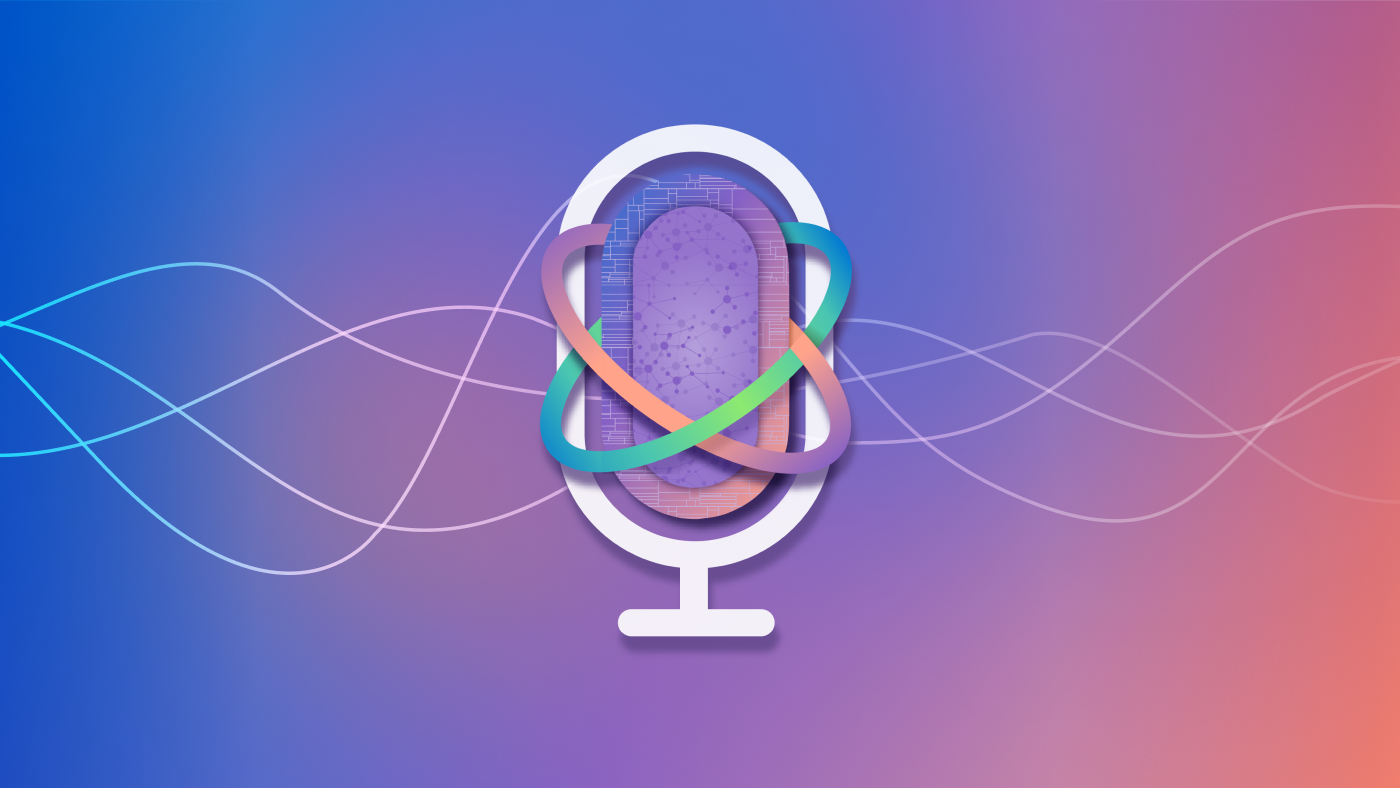
Members of the research community at Microsoft work continuously to advance their respective fields. Abstracts brings its audience to the cutting edge with them through short, compelling conversations about new and noteworthy achievements.
In this episode, Senior Researchers Jordan Ash and Dipendra Misra join host Gretchen Huizinga to discuss “The Truth is in There: Improving Reasoning in Language Models with Layer-Selective Rank Reduction,” which was accepted to the 2024 International Conference on Learning Representations (ICLR). Layer-Selective Rank reduction, or LASER, is an intervention for targeted parameter reduction in transformer-based models. The work shows that the removal of certain parameters not only maintains model performance like some existing parameter-reduction methods but can actually improve it—no additional training necessary.
To learn more about the paper and related topics, register for Microsoft Research Forum (opens in new tab), a series of panel discussions and lightning talks around science and technology research in the era of general AI.
Subscribe to the Microsoft Research Podcast:
Transcript
[MUSIC PLAYS]GRETCHEN HUIZINGA: Welcome to Abstracts, a Microsoft Research Podcast that puts the spotlight on world-class research in brief. I’m Dr. Gretchen Huizinga. In this series, members of the research community at Microsoft give us a quick snapshot—or a podcast abstract—of their new and noteworthy papers.
[MUSIC FADES]Today, I’m talking to Dr. Dipendra Misra and Dr. Jordan Ash, both senior researchers at Microsoft Research. Drs. Misra and Ash are coauthors of a paper called “The Truth is in There: Improving Reasoning in Language Models with Layer-Selective Rank Reduction,” also known as LASER. This paper has been accepted at the International Conference on Learning Representations, or ICLR, in Vienna this year, and you can read a preprint of it now on arXiv. Dipendra, Jordan, thanks for joining us on Abstracts!
JORDAN ASH: Thanks for having us.
DIPENDRA MISRA: Yeah, thanks for having us, Gretchen.
HUIZINGA: Dipendra, let’s start with a general overview of this paper. In a few sentences, describe the issue or problem your work addresses and, perhaps more importantly, why we should care about it.
MISRA: Thanks, Gretchen. So as we know, large language models, also known as LLMs, have revolutionized both business and research in artificial intelligence. They are everywhere, being used to solve a wide range of problems. So in our paper, we introduce an intervention which can be applied to any existing pretrained large language models, and our main purpose for introducing this is to see how it affects the performance of the LLMs and whether we can gain insight into how an LLM stores information in its parameters and how it uses that information to generate a response. And what our intervention does is that it performs a low-rank approximation of the parameters of the LLM. And the surprising discovery that our paper makes is that if you do this intervention correctly, then we can get significant improvement on various tasks for different LLMs.
HUIZINGA: So that’s the first part of the question. Tell me why I should care about it!
MISRA: So if you are a person who uses LLMs for solving any tasks, then you do care about performance on a given task. So, for example, you could be using LLMs to generate an email, right, from a given description. Or you could be using an LLM to do question answering. And by applying our intervention, we can gain accuracy on the task that we care about.
HUIZINGA: Well, let’s stick with you, Dipendra, for a minute and talk about the field writ large. Almost all research owes a debt to some other research that went before. So tell us a bit about the related work in this field and how your work builds on or adds to it.
MISRA: So the work that is most closely related to our LASER paper is this growing body of work on understanding how knowledge is stored and edited inside a large language model. So these works don’t apply the intervention that we do, but they were certainly inspirational for us for arriving at the intervention that we introduced. Another line of work which is very related is, like, adding a small number of parameters to improve the performance of the LLM on a given task. The most relevant work in this space is the LoRA paper, also known as the “Low-Rank Adaptation of Large Language Models,” which came from Microsoft. And what LoRA does, it adds a small number of additional parameters to an LLM and then fine-tunes it on a given task. And what our intervention, called LASER, does is that it removes parameters instead of adding it. And another line of work which is also related is the work on model compression. So there are people who focus on breaking down the size of the models as much as possible while still retaining the performance, more or less, compared to the base model. And so these people are also focused on removing parameters, but they are coming at a different angle of, like, trying to reduce the memory footprint, while what we were doing is that we are less focused on the memory footprint—that’s more like a side effect of it—and more like if I were to fiddle with this parameter of the LLM, then how does it affect the performance? And what can we learn by looking at the comparison? Like, OK, so if I remove this parameter, I see the performance drop; then it means that these parameters are storing something about this type of task on which the performance is dropping.
HUIZINGA: So I’ll ask you one more question, Dipendra, before I pull Jordan into the conversation, and that would be about your methodology. How would you describe your approach to this project, and how did you conduct the research?
MISRA: So we started by analyzing the intervention LASER on a particular LLM called GPT-J and evaluating its performance on this question-answering data CounterFact. So our idea was, like, before trying this thing on [a] bunch of things, let’s just understand this in one setting deeply and, kind of, build insights that we can then evaluate in other settings. And the reason we chose this setup was that the GPT-J large language model has its training data publicly available. It’s called the Pile dataset. And that allows us to do analysis with the training data. For example, is the performance dropping on data points which are rarer or more frequent in the training data? And this is important because training data analysis is frequently omitted in existing LLM literature, and that’s something we wanted to do. And the second reason is that the CounterFact question-answering data is both related to the prior work in this space, so there was a reason for choosing it, but also it has paraphrases of the same question. For example, it might ask, like, “Who is the president of United States of America?” But it will also have paraphrases like “The president of the United States of America is …” or “The head of the government of United States of America is …” And so it will have different variations of the same question. And then you can see if the LLM is able to get all of them right, or is it not robust to variations of the same question? And so we did analysis on this GPT-J and CounterFact dataset. And Jordan will talk more about what the results were. And so based on this rigorous analysis, we developed some insights as to what the intervention is doing. And then we evaluated these insights on other settings. So then we tried, like, two other different large language models and evaluated it on, like, multiple different datasets. And then we saw that the insights actually hold more broadly. And finally, we also evaluated this in a non-text related task, right. Because the intervention could, in principle, be applied to any neural network. So we went after this reinforcement learning model, which solves a puzzle called Sokoban. And we also saw that if you apply this intervention correctly, then you can get some performance improvement. So it’s not related to just large language models, although that was our main motivation.
HUIZINGA: Well, Jordan, let’s get your take on the last few questions here. As I’ve said before, the most interesting section of a research paper for me is the part where it says, “and what we found was …” So as a result of this research, what did you find? Were there outcomes that you expected, or were there any surprises?
ASH: I would say this paper is full of surprises. So as Dipendra was mentioning earlier, the LASER intervention removes information from a model. It doesn’t add information to a model. And up until now, there’s been a lot of work on pruning model parameters for a variety of reasons. But generally, these papers show that as parameters are removed from the model, performance just does not degrade. You can, overall, keep performance roughly the same even with a fairly drastic reduction of model parameters. And those reductions are typically done across layers of the model. What we’re showing here is surprising because we’re showing if we do a very targeted intervention, maybe at only one layer of the model, we could actually get a big boost in performance rather than just, you know, keep it the same or something like this.
HUIZINGA: Hmm. So with those results in mind, Jordan, I’m curious about practical applications. How would you say this research makes an impact in real-world situations? I know that Dipendra alluded to that earlier, but where is this most useful and who benefits most?
ASH: I think the short sales pitch for this technique is that you could potentially improve the performance of a language model with no additional training at all just by applying this intervention, which again just removes information from the model, so you don’t need to have any extra data on hand to refine the model or to add new information into it. The real-world situations we’re seeing a boost right now in LASER is for, like, question answering or reasoning-type tasks where there is, there’s, like, a concrete answer that corresponds to what you’re asking the LLM rather than just a, sort of, like, broad-purpose generative task.
HUIZINGA: So typically speaking, when you’re dealing with LLMs, part of the issue is prompt engineering. And it’s like my responsibility to be able to put the right words in it so I’ll get the best answer from the model, right? Are you saying that this helps me not have to be that good on the prompt-engineer end versus what the model can interpret and do?
ASH: I think prompt engineering still has a place in, sort of, eking out a good answer from a language model, but given a fixed prompt, this intervention seems to offer an improved accuracy over not intervening at all and applying the same prompt.
HUIZINGA: So, Jordan, I often think of an abstract as a sort of appetizer for a research paper. But let’s distill it even further. If there was one thing—sort of an amuse-bouche, if you will—that you want our listeners to take away from this work, what would it be?
ASH: For me, I like this idea of how, you know, typically if you want to get a model to perform better, you would take that model off the shelf and you would refine it on data related to the task at hand. And that might take the form of refining all of the parameters or doing some low-rank LoRA-type thing that Dipendra alluded to earlier. Here, we counterintuitively show that sometimes just carefully removing information from the model can have a positive effect, as well. And this is great news because refining a model requires a lot of new target domain data to be available, but removing information from the model doesn’t necessarily have that same constraint.
HUIZINGA: Well, finally, let’s talk a little bit about the future, Jordan, and I’ll have you close the show for us. What unanswered questions or ongoing research challenges do you see here, and what’s next maybe on your research agenda?
ASH: Yeah, I think there’s a lot of exciting future work for this project. I think for one, as a practical matter, there’s this question of just what’s the best way to find the best LASER intervention? LASER targets a specific layer of the model, and then it finds the extent by which it should be rank-reduced. That search procedure is, kind of, expensive. Right now, we’re doing it in a, sort of, exhaustive way. But also, it seems to be beneficial to apply LASER at multiple layers of the model. And that makes the search procedure, sort of, combinatorially explode. So finding out the best way to compose these interventions, I think, is an important area of future research. And then just, sort of, less on the practical side, I think there are all these questions related to just, why does this work at all? Like, why is it helpful to remove information from the model? And, you know, I think there are some rough ideas we have about this. For example, when you’re training a model on lots and lots of data, you know, it’s not all created equally. Some of it might be noisy or low quality, and some of it might be high quality. And maybe it’s better to remove those samples at training time to get a better model. So I guess there’s this question of, is pruning the model using a LASER-type intervention roughly equivalent to pruning the training data in a way to make it more favorable for eliciting a high-quality model? And again, like Dipendra alluded to earlier, this LoRA procedure, which does something that very much complements LASER and is often used to add information to a model, is it possible that LoRA is actually not just adding information but also removing information from the model? And perhaps that’s one reason why LASER seems to be so effective.
HUIZINGA: So lots of questions.
ASH: I would say so, yeah!
HUIZINGA: Well, Dipendra Misra, Jordan Ash, thanks for joining us today. And to our listeners, thanks for tuning in.
[MUSIC PLAYS]Again, you can find a link to this paper at aka.ms/abstracts (opens in new tab) or on arXiv (opens in new tab). And I’ll also add that Dipendra will be speaking about this work at the upcoming Microsoft Research Forum, and you can register for this series of events at researchforum.microsoft.com (opens in new tab). See you next time on Abstracts!
[MUSIC FADES]
The post Abstracts: January 25, 2024 appeared first on Microsoft Research.
New embedding models and API updates
We are launching a new generation of embedding models, new GPT-4 Turbo and moderation models, new API usage management tools, and soon, lower pricing on GPT-3.5 Turbo.OpenAI Blog
Build enterprise-ready generative AI solutions with Cohere foundation models in Amazon Bedrock and Weaviate vector database on AWS Marketplace
Generative AI solutions have the potential to transform businesses by boosting productivity and improving customer experiences, and using large language models (LLMs) with these solutions has become increasingly popular. Building proofs of concept is relatively straightforward because cutting-edge foundation models are available from specialized providers through a simple API call. Therefore, organizations of various sizes and across different industries have begun to reimagine their products and processes using generative AI.
Despite their wealth of general knowledge, state-of-the-art LLMs only have access to the information they were trained on. This can lead to factual inaccuracies (hallucinations) when the LLM is prompted to generate text based on information they didn’t see during their training. Therefore, it’s crucial to bridge the gap between the LLM’s general knowledge and your proprietary data to help the model generate more accurate and contextual responses while reducing the risk of hallucinations. The traditional method of fine-tuning, although effective, can be compute-intensive, expensive, and requires technical expertise. Another option to consider is called Retrieval Augmented Generation (RAG), which provides LLMs with additional information from an external knowledge source that can be updated easily.
Additionally, enterprises must ensure data security when handling proprietary and sensitive data, such as personal data or intellectual property. This is particularly important for organizations operating in heavily regulated industries, such as financial services and healthcare and life sciences. Therefore, it’s important to understand and control the flow of your data through the generative AI application: Where is the model located? Where is the data processed? Who has access to the data? Will the data be used to train models, eventually risking the leak of sensitive data to public LLMs?
This post discusses how enterprises can build accurate, transparent, and secure generative AI applications while keeping full control over proprietary data. The proposed solution is a RAG pipeline using an AI-native technology stack, whose components are designed from the ground up with AI at their core, rather than having AI capabilities added as an afterthought. We demonstrate how to build an end-to-end RAG application using Cohere’s language models through Amazon Bedrock and a Weaviate vector database on AWS Marketplace. The accompanying source code is available in the related GitHub repository hosted by Weaviate. Although AWS will not be responsible for maintaining or updating the code in the partner’s repository, we encourage customers to connect with Weaviate directly regarding any desired updates.
Solution overview
The following high-level architecture diagram illustrates the proposed RAG pipeline with an AI-native technology stack for building accurate, transparent, and secure generative AI solutions.
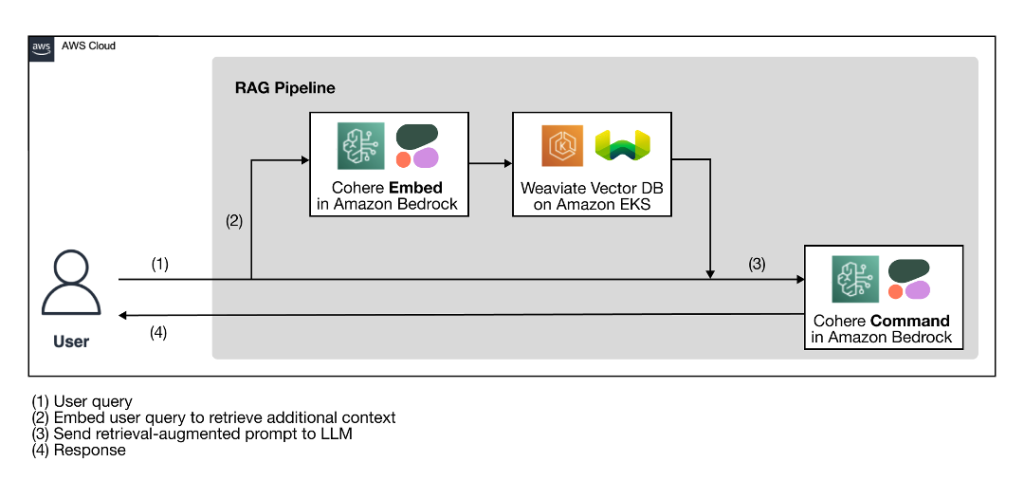
Figure 1: RAG workflow using Cohere’s language models through Amazon Bedrock and a Weaviate vector database on AWS Marketplace
As a preparation step for the RAG workflow, a vector database, which serves as the external knowledge source, is ingested with the additional context from the proprietary data. The actual RAG workflow follows the four steps illustrated in the diagram:
- The user enters their query.
- The user query is used to retrieve relevant additional context from the vector database. This is done by generating the vector embeddings of the user query with an embedding model to perform a vector search to retrieve the most relevant context from the database.
- The retrieved context and the user query are used to augment a prompt template. The retrieval-augmented prompt helps the LLM generate a more relevant and accurate completion, minimizing hallucinations.
- The user receives a more accurate response based on their query.
The AI-native technology stack illustrated in the architecture diagram has two key components: Cohere language models and a Weaviate vector database.
Cohere language models in Amazon Bedrock
The Cohere Platform brings language models with state-of-the-art performance to enterprises and developers through a simple API call. There are two key types of language processing capabilities that the Cohere Platform provides—generative and embedding—and each is served by a different type of model:
- Text generation with Command – Developers can access endpoints that power generative AI capabilities, enabling applications such as conversational, question answering, copywriting, summarization, information extraction, and more.
- Text representation with Embed – Developers can access endpoints that capture the semantic meaning of text, enabling applications such as vector search engines, text classification and clustering, and more. Cohere Embed comes in two forms, an English language model and a multilingual model, both of which are now available on Amazon Bedrock.
The Cohere Platform empowers enterprises to customize their generative AI solution privately and securely through the Amazon Bedrock deployment. Amazon Bedrock is a fully managed cloud service that enables development teams to build and scale generative AI applications quickly while helping keep your data and applications secure and private. Your data is not used for service improvements, is never shared with third-party model providers, and remains in the Region where the API call is processed. The data is always encrypted in transit and at rest, and you can encrypt the data using your own keys. Amazon Bedrock supports security requirements, including U.S. Health Insurance Portability and Accountability Act (HIPAA) eligibility and General Data Protection Regulation (GDPR) compliance. Additionally, you can securely integrate and easily deploy your generative AI applications using the AWS tools you are already familiar with.
Weaviate vector database on AWS Marketplace
Weaviate is an AI-native vector database that makes it straightforward for development teams to build secure and transparent generative AI applications. Weaviate is used to store and search both vector data and source objects, which simplifies development by eliminating the need to host and integrate separate databases. Weaviate delivers subsecond semantic search performance and can scale to handle billions of vectors and millions of tenants. With a uniquely extensible architecture, Weaviate integrates natively with Cohere foundation models deployed in Amazon Bedrock to facilitate the convenient vectorization of data and use its generative capabilities from within the database.
The Weaviate AI-native vector database gives customers the flexibility to deploy it as a bring-your-own-cloud (BYOC) solution or as a managed service. This showcase uses the Weaviate Kubernetes Cluster on AWS Marketplace, part of Weaviate’s BYOC offering, which allows container-based scalable deployment inside your AWS tenant and VPC with just a few clicks using an AWS CloudFormation template. This approach ensures that your vector database is deployed in your specific Region close to the foundation models and proprietary data to minimize latency, support data locality, and protect sensitive data while addressing potential regulatory requirements, such as GDPR.
Use case overview
In the following sections, we demonstrate how to build a RAG solution using the AI-native technology stack with Cohere, AWS, and Weaviate, as illustrated in the solution overview.
The example use case generates targeted advertisements for vacation stay listings based on a target audience. The goal is to use the user query for the target audience (for example, “family with small children”) to retrieve the most relevant vacation stay listing (for example, a listing with playgrounds close by) and then to generate an advertisement for the retrieved listing tailored to the target audience.

Figure 2: First few rows of vacation stay listings available from Inside Airbnb.
The dataset is available from Inside Airbnb and is licensed under a Creative Commons Attribution 4.0 International License. You can find the accompanying code in the GitHub repository.
Prerequisites
To follow along and use any AWS services in the following tutorial, make sure you have an AWS account.
Enable components of the AI-native technology stack
First, you need to enable the relevant components discussed in the solution overview in your AWS account. Complete the following steps:
- In the left Amazon Bedrock console, choose Model access in the navigation pane.
- Choose Manage model access on the top right.
- Select the foundation models of your choice and request access.
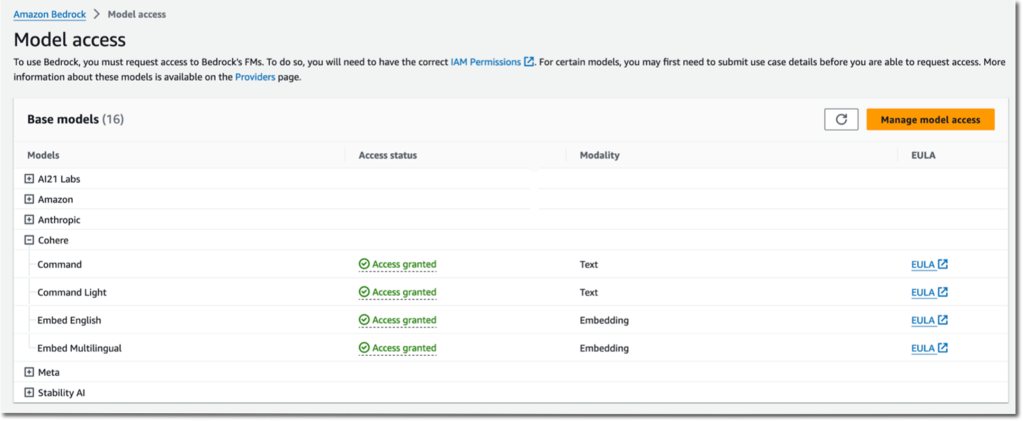
Figure 3: Manage model access in Amazon Bedrock console.
Next, you set up a Weaviate cluster.
- Subscribe to the Weaviate Kubernetes Cluster on AWS Marketplace.
- Launch the software using a CloudFormation template according to your preferred Availability Zone.
The CloudFormation template is pre-populated with default values.
- For Stack name, enter a stack name.
- For helmauthenticationtype, it is recommended to enable authentication by setting
helmauthenticationtype
toapikey
and defining a helmauthenticationapikey. - For helmauthenticationapikey, enter your Weaviate API key.
- For helmchartversion, enter your version number. It must be at least v.16.8.0. Refer to the GitHub repo for the latest version.
- For helmenabledmodules, make sure
tex2vec-aws
andgenerative-aws
are present in the list of enabled modules within Weaviate.
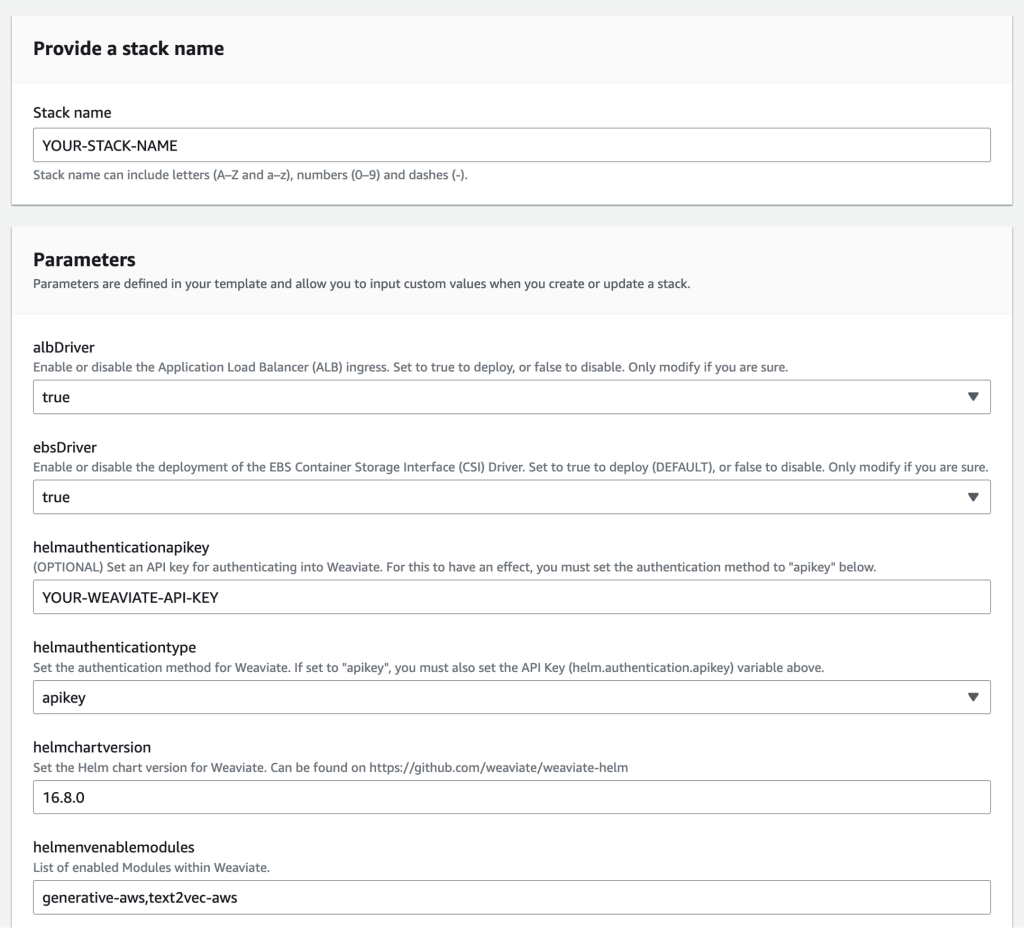
Figure 4: CloudFormation template.
This template takes about 30 minutes to complete.
Connect to Weaviate
Complete the following steps to connect to Weaviate:
- In the Amazon SageMaker console, navigate to Notebook instances in the navigation pane via Notebook > Notebook instances on the left.
- Create a new notebook instance.
- Install the Weaviate client package with the required dependencies:
- Connect to your Weaviate instance with the following code:
- Weaviate URL – Access Weaviate via the load balancer URL. In the Amazon Elastic Compute Cloud (Amazon EC2) console, choose Load balancers in the navigation pane and find the load balancer. Look for the DNS name column and add
http://
in front of it. - Weaviate API key – This is the key you set earlier in the CloudFormation template (
helmauthenticationapikey
). - AWS access key and secret access key – You can retrieve the access key and secret access key for your user in the AWS Identity and Access Management (IAM) console.
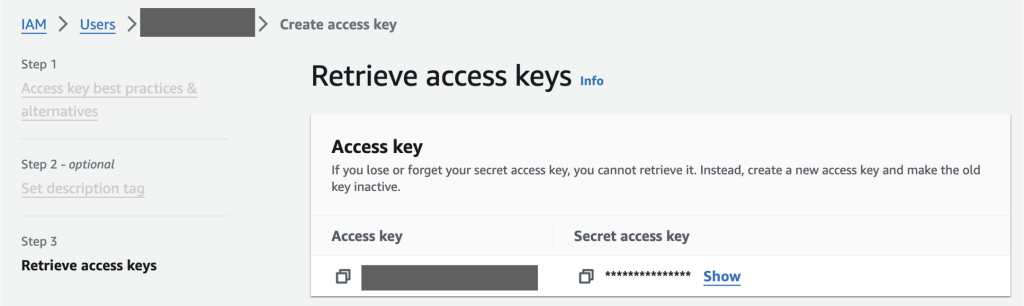
Figure 5: AWS Identity and Access Management (IAM) console to retrieve AWS access key and secret access key.
Configure the Amazon Bedrock module to enable Cohere models
Next, you define a data collection (class
) called Listings
to store the listings’ data objects, which is analogous to creating a table in a relational database. In this step, you configure the relevant modules to enable the usage of Cohere language models hosted on Amazon Bedrock natively from within the Weaviate vector database. The vectorizer (“text2vec-aws
“) and generative module (“generative-aws
“) are specified in the data collection definition. Both of these modules take three parameters:
- “service” – Use “
bedrock
” for Amazon Bedrock (alternatively, use “sagemaker
” for Amazon SageMaker JumpStart) - “Region” – Enter the Region where your model is deployed
- “model” – Provide the foundation model’s name
See the following code:
Ingest data into the Weaviate vector database
In this step, you define the structure of the data collection by configuring its properties. Aside from the property’s name and data type, you can also configure if only the data object will be stored or if it will be stored together with its vector embeddings. In this example, host_name
and property_type
are not vectorized:
Run the following code to create the collection in your Weaviate instance:
You can now add objects to Weaviate. You use a batch import process for maximum efficiency. Run the following code to import data. During the import, Weaviate will use the defined vectorizer to create a vector embedding for each object. The following code loads objects, initializes a batch process, and adds objects to the target collection one by one:
Retrieval Augmented Generation
You can build a RAG pipeline by implementing a generative search query on your Weaviate instance. For this, you first define a prompt template in the form of an f-string that can take in the user query ({target_audience}
) directly and the additional context ({{host_name}}
, {{property_type}}
, {{description}}
, and {{neighborhood_overview}}
) from the vector database at runtime:
Next, you run a generative search query. This prompts the defined generative model with a prompt that is comprised of the user query as well as the retrieved data. The following query retrieves one listing object (.with_limit(1)
) from the Listings
collection that is most similar to the user query (.with_near_text({"concepts": target_audience})
). Then the user query (target_audience
) and the retrieved listings properties (["description", "neighborhood", "host_name", "property_type"]
) are fed into the prompt template. See the following code:
In the following example, you can see that the preceding piece of code for target_audience = “Family with small children”
retrieves a listing from the host Marre. The prompt template is augmented with Marre’s listing details and the target audience:
Based on the retrieval-augmented prompt, Cohere’s Command model generates the following targeted advertisement:
Alternative customizations
You can make alternative customizations to different components in the proposed solution, such as the following:
- Cohere’s language models are also available through Amazon SageMaker JumpStart, which provides access to cutting-edge foundation models and enables developers to deploy LLMs to Amazon SageMaker, a fully managed service that brings together a broad set of tools to enable high-performance, low-cost machine learning for any use case. Weaviate is integrated with SageMaker as well.
- A powerful addition to this solution is the Cohere Rerank endpoint, available through SageMaker JumpStart. Rerank can improve the relevance of search results from lexical or semantic search. Rerank works by computing semantic relevance scores for documents that are retrieved by a search system and ranking the documents based on these scores. Adding Rerank to an application requires only a single line of code change.
- To cater to different deployment requirements of different production environments, Weaviate can be deployed in various additional ways. For example, it is available as a direct download from Weaviate website, which runs on Amazon Elastic Kubernetes Service (Amazon EKS) or locally via Docker or Kubernetes. It’s also available as a managed service that can run securely within a VPC or as a public cloud service hosted on AWS with a 14-day free trial.
- You can serve your solution in a VPC using Amazon Virtual Private Cloud (Amazon VPC), which enables organizations to launch AWS services in a logically isolated virtual network, resembling a traditional network but with the benefits of AWS’s scalable infrastructure. Depending on the classified level of sensitivity of the data, organizations can also disable internet access in these VPCs.
Clean up
To prevent unexpected charges, delete all the resources you deployed as part of this post. If you launched the CloudFormation stack, you can delete it via the AWS CloudFormation console. Note that there may be some AWS resources, such as Amazon Elastic Block Store (Amazon EBS) volumes and AWS Key Management Service (AWS KMS) keys, that may not be deleted automatically when the CloudFormation stack is deleted.

Figure 6: Delete all resources via the AWS CloudFormation console.
Conclusion
This post discussed how enterprises can build accurate, transparent, and secure generative AI applications while still having full control over their data. The proposed solution is a RAG pipeline using an AI-native technology stack as a combination of Cohere foundation models in Amazon Bedrock and a Weaviate vector database on AWS Marketplace. The RAG approach enables enterprises to bridge the gap between the LLM’s general knowledge and the proprietary data while minimizing hallucinations. An AI-native technology stack enables fast development and scalable performance.
You can start experimenting with RAG proofs of concept for your enterprise-ready generative AI applications using the steps outlined in this post. The accompanying source code is available in the related GitHub repository. Thank you for reading. Feel free to provide comments or feedback in the comments section.
About the authors
James Yi is a Senior AI/ML Partner Solutions Architect in the Technology Partners COE Tech team at Amazon Web Services. He is passionate about working with enterprise customers and partners to design, deploy, and scale AI/ML applications to derive business value. Outside of work, he enjoys playing soccer, traveling, and spending time with his family.
Leonie Monigatti is a Developer Advocate at Weaviate. Her focus area is AI/ML, and she helps developers learn about generative AI. Outside of work, she also shares her learnings in data science and ML on her blog and on Kaggle.
Meor Amer is a Developer Advocate at Cohere, a provider of cutting-edge natural language processing (NLP) technology. He helps developers build cutting-edge applications with Cohere’s Large Language Models (LLMs).
Shun Mao is a Senior AI/ML Partner Solutions Architect in the Emerging Technologies team at Amazon Web Services. He is passionate about working with enterprise customers and partners to design, deploy and scale AI/ML applications to derive their business values. Outside of work, he enjoys fishing, traveling and playing Ping-Pong.
Research Focus: Week of January 22, 2024
Welcome to Research Focus, a series of blog posts that highlights notable publications, events, code/datasets, new hires and other milestones from across the research community at Microsoft.

EVENT SERIES
Register for Microsoft Research Forum
Join Microsoft Research Forum (opens in new tab) for a continuous exchange of ideas about science and technology research in the era of general AI. This series, which begins on January 30, will explore recent research advances, bold new ideas, and important discussions with the global research community. Register now to receive access to all episodes in this quarterly series and be part of the conversation.
NEW RESEARCH
Improving Text Embeddings with Large Language Models
Text embeddings are vector representations of natural language that encode semantic information. They are widely used in various natural language processing tasks, such as information retrieval, question answering, semantic textual similarity, bitext mining, item recommendation, etc.
In a recent paper: Improving Text Embeddings with Large Language Models (opens in new tab), researchers from Microsoft introduce a novel and simple method for obtaining high-quality text embeddings using only synthetic data and less than 1k training steps. Unlike existing methods, this new method does not require building complex training pipelines or manually collected datasets that are often constrained by task diversity and language coverage. The researchers leverage proprietary large language models (LLMs) to generate diverse synthetic data for hundreds of thousands of text embedding tasks across nearly 100 languages. They then fine-tune open-source decoder-only LLMs on the synthetic data using standard contrastive loss. Experiments demonstrate that this method achieves strong performance on highly competitive text embedding benchmarks without using any labeled data. Furthermore, when fine-tuned with a mixture of synthetic and labeled data, the model sets new state-of-the-art results on the BEIR (opens in new tab) and MTEB (opens in new tab) benchmarks.
Spotlight: On-demand video
AI Explainer: Foundation models and the next era of AI
Explore how the transformer architecture, larger models and more data, and in-context learning have helped advance AI from perception to creation.
NEW RESEARCH
DevEx in Action: A study of its tangible impacts
For many professional software developers, the development lifecycle is riddled with friction and red tape, and successful delivery of code to production is a frustratingly infrequent event. Even worse, the problems are often compounded by a lack of management engagement, delaying and frustrating top engineers.
Developer experience (DevEx) is garnering increased attention at many organizations as leaders seek to optimize software delivery against a backdrop of fiscal tightening and transformational technologies such as AI. Developers and technical leaders generally understand that good DevEx leads to better products, more effective software delivery, and developer happiness. Yet, at many organizations, proposed initiatives and investments to improve DevEx struggle to get buy-in, as business stakeholders question the value proposition of improvements.
In a recent paper: DevEx in Action: A study of its tangible impacts (opens in new tab), researchers from Microsoft, GitHub, and DX (opens in new tab) examine this problem and present empirical evidence of how improvements in DevEx influence outcomes like productivity, code quality, and innovation.
The post Research Focus: Week of January 22, 2024 appeared first on Microsoft Research.