We’re working to prevent abuse, provide transparency on AI-generated content, and improve access to accurate voting information.OpenAI Blog
NVIDIA CEO: ‘This Year, Every Industry Will Become a Technology Industry’
“This year, every industry will become a technology industry,” NVIDIA founder and CEO Jensen Huang told attendees Wednesday during the annual J.P. Morgan Healthcare Conference.
“You can now recognize and learn the language of almost anything with structure, and you can translate it to anything with structure — so text-protein, protein-text,” Huang said in a fireside chat with Martin Chavez, partner and vice chairman of global investment firm Sixth Street Partners and board chair of Recursion, a biopharmaceutical company. “This is the generative AI revolution.”
The conversation, which took place at the historic San Francisco Mint, followed a presentation at the J.P. Morgan conference Monday by Kimberly Powell, NVIDIA’s VP of healthcare. In her talk, Powell announced that Recursion is the first hosting partner to offer a foundation model through the NVIDIA BioNeMo cloud service, which is advancing into beta this month.
She also said that Amgen, one of the first companies to employ BioNeMo, plans to advance drug discovery with generative AI and NVIDIA DGX SuperPOD — and that BioNeMo is used by a growing number of techbio companies, pharmas, AI software vendors and systems integrators. Among them are Deloitte, Innophore, Insilico Medicine, OneAngstrom, Recursion and Terray Therapeutics.
From Computer-Aided Chip Design to Drug Design
Healthcare customers and partners now consume well over a billion dollars in NVIDIA GPU computing each year — directly and indirectly through cloud partners.
Huang traced NVIDIA’s involvement in accelerated healthcare back to two research projects that caught his attention around 15 years ago: one at Mass General tapped NVIDIA GPUs to reconstruct CT images, another at the University of Illinois Urbana-Champaign applied GPU acceleration to molecular dynamics.
“It opened my mind that we could apply the same methodology that we use in computer-aided chip design to help the world of drug discovery go from computer-aided drug discovery to computer-aided drug design,” he said, realizing that, “if we scale this up by a billion times, we could simulate biology.”
After 40 years of advancements in computer-aided chip design, engineers can now build complex computing systems entirely in simulation, Huang explained. Over the next decade, the same could be true for AI-accelerated drug design.
“Almost everything will largely start in silico, largely end in silico,” he said, using a term that refers to an experiment run on a computer.
Collaborating on the Future of Drug Discovery and Medical Instruments
With the progress made to date, computer-aided drug discovery is “genuinely miraculous,” Huang said.
NVIDIA is propelling the field forward by building state-of-the-art AI models and powerful computing platforms, and by collaborating with domain experts and investing in techbio companies.
“We are determined to work with you to advance this field,” Huang said, inviting healthcare innovators to reach out to NVIDIA. “We deeply believe that this is going to be the future of the way that drugs will be discovered and designed.”
The company’s pipelines for accelerated healthcare include algorithms for cryo-electron microscopy, X-ray crystallography, gene sequencing, amino acid structure prediction and virtual drug molecule screening. And as AI advances, these computing tools are becoming much easier to access, Huang said.
“Because of artificial intelligence and the groundbreaking work that our industry has done, we have closed the technology divide in a dramatic way,” he said. “Everybody is a programmer, and the programming language of the future is called ‘human.’”
Beyond drug development, this transformation to a software-defined, AI-driven industry will also advance medical instruments.
“A medical instrument is never going to be the same again. Ultrasound systems, CT scan systems, all kinds of instruments — they’re always going to be a device plus a whole bunch of AIs,” Huang said. “The value that will create, the opportunities you create, are going to be incredible.”
For more from NVIDIA at the J.P. Morgan Healthcare Conference, listen to the audio recording and view the presentation deck of Powell’s session.
Learn about NVIDIA’s AI platform for healthcare and life sciences and subscribe to NVIDIA healthcare news.
AMIE: A research AI system for diagnostic medical reasoning and conversations
The physician-patient conversation is a cornerstone of medicine, in which skilled and intentional communication drives diagnosis, management, empathy and trust. AI systems capable of such diagnostic dialogues could increase availability, accessibility, quality and consistency of care by being useful conversational partners to clinicians and patients alike. But approximating clinicians’ considerable expertise is a significant challenge.
Recent progress in large language models (LLMs) outside the medical domain has shown that they can plan, reason, and use relevant context to hold rich conversations. However, there are many aspects of good diagnostic dialogue that are unique to the medical domain. An effective clinician takes a complete “clinical history” and asks intelligent questions that help to derive a differential diagnosis. They wield considerable skill to foster an effective relationship, provide information clearly, make joint and informed decisions with the patient, respond empathically to their emotions, and support them in the next steps of care. While LLMs can accurately perform tasks such as medical summarization or answering medical questions, there has been little work specifically aimed towards developing these kinds of conversational diagnostic capabilities.
Inspired by this challenge, we developed Articulate Medical Intelligence Explorer (AMIE), a research AI system based on a LLM and optimized for diagnostic reasoning and conversations. We trained and evaluated AMIE along many dimensions that reflect quality in real-world clinical consultations from the perspective of both clinicians and patients. To scale AMIE across a multitude of disease conditions, specialties and scenarios, we developed a novel self-play based simulated diagnostic dialogue environment with automated feedback mechanisms to enrich and accelerate its learning process. We also introduced an inference time chain-of-reasoning strategy to improve AMIE’s diagnostic accuracy and conversation quality. Finally, we tested AMIE prospectively in real examples of multi-turn dialogue by simulating consultations with trained actors.
Evaluation of conversational diagnostic AI
Besides developing and optimizing AI systems themselves for diagnostic conversations, how to assess such systems is also an open question. Inspired by accepted tools used to measure consultation quality and clinical communication skills in real-world settings, we constructed a pilot evaluation rubric to assess diagnostic conversations along axes pertaining to history-taking, diagnostic accuracy, clinical management, clinical communication skills, relationship fostering and empathy.
We then designed a randomized, double-blind crossover study of text-based consultations with validated patient actors interacting either with board-certified primary care physicians (PCPs) or the AI system optimized for diagnostic dialogue. We set up our consultations in the style of an objective structured clinical examination (OSCE), a practical assessment commonly used in the real world to examine clinicians’ skills and competencies in a standardized and objective way. In a typical OSCE, clinicians might rotate through multiple stations, each simulating a real-life clinical scenario where they perform tasks such as conducting a consultation with a standardized patient actor (trained carefully to emulate a patient with a particular condition). Consultations were performed using a synchronous text-chat tool, mimicking the interface familiar to most consumers using LLMs today.
![]() |
AMIE is a research AI system based on LLMs for diagnostic reasoning and dialogue. |
AMIE: an LLM-based conversational diagnostic research AI system
We trained AMIE on real-world datasets comprising medical reasoning, medical summarization and real-world clinical conversations.
It is feasible to train LLMs using real-world dialogues developed by passively collecting and transcribing in-person clinical visits, however, two substantial challenges limit their effectiveness in training LLMs for medical conversations. First, existing real-world data often fails to capture the vast range of medical conditions and scenarios, hindering the scalability and comprehensiveness. Second, the data derived from real-world dialogue transcripts tends to be noisy, containing ambiguous language (including slang, jargon, humor and sarcasm), interruptions, ungrammatical utterances, and implicit references.
To address these limitations, we designed a self-play based simulated learning environment with automated feedback mechanisms for diagnostic medical dialogue in a virtual care setting, enabling us to scale AMIE’s knowledge and capabilities across many medical conditions and contexts. We used this environment to iteratively fine-tune AMIE with an evolving set of simulated dialogues in addition to the static corpus of real-world data described.
This process consisted of two self-play loops: (1) an “inner” self-play loop, where AMIE leveraged in-context critic feedback to refine its behavior on simulated conversations with an AI patient simulator; and (2) an “outer” self-play loop where the set of refined simulated dialogues were incorporated into subsequent fine-tuning iterations. The resulting new version of AMIE could then participate in the inner loop again, creating a virtuous continuous learning cycle.
Further, we also employed an inference time chain-of-reasoning strategy which enabled AMIE to progressively refine its response conditioned on the current conversation to arrive at an informed and grounded reply.
![]() |
AMIE uses a novel self-play based simulated dialogue learning environment to improve the quality of diagnostic dialogue across a multitude of disease conditions, specialities and patient contexts. |
We tested performance in consultations with simulated patients (played by trained actors), compared to those performed by 20 real PCPs using the randomized approach described above. AMIE and PCPs were assessed from the perspectives of both specialist attending physicians and our simulated patients in a randomized, blinded crossover study that included 149 case scenarios from OSCE providers in Canada, the UK and India in a diverse range of specialties and diseases.
Notably, our study was not designed to emulate either traditional in-person OSCE evaluations or the ways clinicians usually use text, email, chat or telemedicine. Instead, our experiment mirrored the most common way consumers interact with LLMs today, a potentially scalable and familiar mechanism for AI systems to engage in remote diagnostic dialogue.
![]() |
Overview of the randomized study design to perform a virtual remote OSCE with simulated patients via online multi-turn synchronous text chat. |
Performance of AMIE
In this setting, we observed that AMIE performed simulated diagnostic conversations at least as well as PCPs when both were evaluated along multiple clinically-meaningful axes of consultation quality. AMIE had greater diagnostic accuracy and superior performance for 28 of 32 axes from the perspective of specialist physicians, and 24 of 26 axes from the perspective of patient actors.
![]() |
AMIE outperformed PCPs on multiple evaluation axes for diagnostic dialogue in our evaluations. |
![]() |
Specialist-rated top-k diagnostic accuracy. AMIE and PCPs top-k differential diagnosis (DDx) accuracy are compared across 149 scenarios with respect to the ground truth diagnosis (a) and all diagnoses listed within the accepted differential diagnoses (b). Bootstrapping (n=10,000) confirms all top-k differences between AMIE and PCP DDx accuracy are significant with p <0.05 after false discovery rate (FDR) correction. |
![]() |
Diagnostic conversation and reasoning qualities as assessed by specialist physicians. On 28 out of 32 axes, AMIE outperformed PCPs while being comparable on the rest. |
Limitations
Our research has several limitations and should be interpreted with appropriate caution. Firstly, our evaluation technique likely underestimates the real-world value of human conversations, as the clinicians in our study were limited to an unfamiliar text-chat interface, which permits large-scale LLM–patient interactions but is not representative of usual clinical practice. Secondly, any research of this type must be seen as only a first exploratory step on a long journey. Transitioning from a LLM research prototype that we evaluated in this study to a safe and robust tool that could be used by people and those who provide care for them will require significant additional research. There are many important limitations to be addressed, including experimental performance under real-world constraints and dedicated exploration of such important topics as health equity and fairness, privacy, robustness, and many more, to ensure the safety and reliability of the technology.
AMIE as an aid to clinicians
In a recently released preprint, we evaluated the ability of an earlier iteration of the AMIE system to generate a DDx alone or as an aid to clinicians. Twenty (20) generalist clinicians evaluated 303 challenging, real-world medical cases sourced from the New England Journal of Medicine (NEJM) ClinicoPathologic Conferences (CPCs). Each case report was read by two clinicians randomized to one of two assistive conditions: either assistance from search engines and standard medical resources, or AMIE assistance in addition to these tools. All clinicians provided a baseline, unassisted DDx prior to using the respective assistive tools.
![]() |
Assisted randomized reader study setup to investigate the assistive effect of AMIE to clinicians in solving complex diagnostic case challenges from the New England Journal of Medicine. |
AMIE exhibited standalone performance that exceeded that of unassisted clinicians (top-10 accuracy 59.1% vs. 33.6%, p= 0.04). Comparing the two assisted study arms, the top-10 accuracy was higher for clinicians assisted by AMIE, compared to clinicians without AMIE assistance (24.6%, p<0.01) and clinicians with search (5.45%, p=0.02). Further, clinicians assisted by AMIE arrived at more comprehensive differential lists than those without AMIE assistance.
It’s worth noting that NEJM CPCs are not representative of everyday clinical practice. They are unusual case reports in only a few hundred individuals so offer limited scope for probing important issues like equity or fairness.
Bold and responsible research in healthcare — the art of the possible
Access to clinical expertise remains scarce around the world. While AI has shown great promise in specific clinical applications, engagement in the dynamic, conversational diagnostic journeys of clinical practice requires many capabilities not yet demonstrated by AI systems. Doctors wield not only knowledge and skill but a dedication to myriad principles, including safety and quality, communication, partnership and teamwork, trust, and professionalism. Realizing these attributes in AI systems is an inspiring challenge that should be approached responsibly and with care. AMIE is our exploration of the “art of the possible”, a research-only system for safely exploring a vision of the future where AI systems might be better aligned with attributes of the skilled clinicians entrusted with our care. It is early experimental-only work, not a product, and has several limitations that we believe merit rigorous and extensive further scientific studies in order to envision a future in which conversational, empathic and diagnostic AI systems might become safe, helpful and accessible.
Acknowledgements
The research described here is joint work across many teams at Google Research and Google Deepmind. We are grateful to all our co-authors – Tao Tu, Mike Schaekermann, Anil Palepu, Daniel McDuff, Jake Sunshine, Khaled Saab, Jan Freyberg, Ryutaro Tanno, Amy Wang, Brenna Li, Mohamed Amin, Sara Mahdavi, Karan Sighal, Shekoofeh Azizi, Nenad Tomasev, Yun Liu, Yong Cheng, Le Hou, Albert Webson, Jake Garrison, Yash Sharma, Anupam Pathak, Sushant Prakash, Philip Mansfield, Shwetak Patel, Bradley Green, Ewa Dominowska, Renee Wong, Juraj Gottweis, Dale Webster, Katherine Chou, Christopher Semturs, Joelle Barral, Greg Corrado and Yossi Matias. We also thank Sami Lachgar, Lauren Winer and John Guilyard for their support with narratives and the visuals. Finally, we are grateful to Michael Howell, James Maynika, Jeff Dean, Karen DeSalvo, Zoubin Gharahmani and Demis Hassabis for their support during the course of this project.
AMIE: A research AI system for diagnostic medical reasoning and conversations
The physician-patient conversation is a cornerstone of medicine, in which skilled and intentional communication drives diagnosis, management, empathy and trust. AI systems capable of such diagnostic dialogues could increase availability, accessibility, quality and consistency of care by being useful conversational partners to clinicians and patients alike. But approximating clinicians’ considerable expertise is a significant challenge.
Recent progress in large language models (LLMs) outside the medical domain has shown that they can plan, reason, and use relevant context to hold rich conversations. However, there are many aspects of good diagnostic dialogue that are unique to the medical domain. An effective clinician takes a complete “clinical history” and asks intelligent questions that help to derive a differential diagnosis. They wield considerable skill to foster an effective relationship, provide information clearly, make joint and informed decisions with the patient, respond empathically to their emotions, and support them in the next steps of care. While LLMs can accurately perform tasks such as medical summarization or answering medical questions, there has been little work specifically aimed towards developing these kinds of conversational diagnostic capabilities.
Inspired by this challenge, we developed Articulate Medical Intelligence Explorer (AMIE), a research AI system based on a LLM and optimized for diagnostic reasoning and conversations. We trained and evaluated AMIE along many dimensions that reflect quality in real-world clinical consultations from the perspective of both clinicians and patients. To scale AMIE across a multitude of disease conditions, specialties and scenarios, we developed a novel self-play based simulated diagnostic dialogue environment with automated feedback mechanisms to enrich and accelerate its learning process. We also introduced an inference time chain-of-reasoning strategy to improve AMIE’s diagnostic accuracy and conversation quality. Finally, we tested AMIE prospectively in real examples of multi-turn dialogue by simulating consultations with trained actors.
Evaluation of conversational diagnostic AI
Besides developing and optimizing AI systems themselves for diagnostic conversations, how to assess such systems is also an open question. Inspired by accepted tools used to measure consultation quality and clinical communication skills in real-world settings, we constructed a pilot evaluation rubric to assess diagnostic conversations along axes pertaining to history-taking, diagnostic accuracy, clinical management, clinical communication skills, relationship fostering and empathy.
We then designed a randomized, double-blind crossover study of text-based consultations with validated patient actors interacting either with board-certified primary care physicians (PCPs) or the AI system optimized for diagnostic dialogue. We set up our consultations in the style of an objective structured clinical examination (OSCE), a practical assessment commonly used in the real world to examine clinicians’ skills and competencies in a standardized and objective way. In a typical OSCE, clinicians might rotate through multiple stations, each simulating a real-life clinical scenario where they perform tasks such as conducting a consultation with a standardized patient actor (trained carefully to emulate a patient with a particular condition). Consultations were performed using a synchronous text-chat tool, mimicking the interface familiar to most consumers using LLMs today.
![]() |
AMIE is a research AI system based on LLMs for diagnostic reasoning and dialogue. |
AMIE: an LLM-based conversational diagnostic research AI system
We trained AMIE on real-world datasets comprising medical reasoning, medical summarization and real-world clinical conversations.
It is feasible to train LLMs using real-world dialogues developed by passively collecting and transcribing in-person clinical visits, however, two substantial challenges limit their effectiveness in training LLMs for medical conversations. First, existing real-world data often fails to capture the vast range of medical conditions and scenarios, hindering the scalability and comprehensiveness. Second, the data derived from real-world dialogue transcripts tends to be noisy, containing ambiguous language (including slang, jargon, humor and sarcasm), interruptions, ungrammatical utterances, and implicit references.
To address these limitations, we designed a self-play based simulated learning environment with automated feedback mechanisms for diagnostic medical dialogue in a virtual care setting, enabling us to scale AMIE’s knowledge and capabilities across many medical conditions and contexts. We used this environment to iteratively fine-tune AMIE with an evolving set of simulated dialogues in addition to the static corpus of real-world data described.
This process consisted of two self-play loops: (1) an “inner” self-play loop, where AMIE leveraged in-context critic feedback to refine its behavior on simulated conversations with an AI patient simulator; and (2) an “outer” self-play loop where the set of refined simulated dialogues were incorporated into subsequent fine-tuning iterations. The resulting new version of AMIE could then participate in the inner loop again, creating a virtuous continuous learning cycle.
Further, we also employed an inference time chain-of-reasoning strategy which enabled AMIE to progressively refine its response conditioned on the current conversation to arrive at an informed and grounded reply.
![]() |
AMIE uses a novel self-play based simulated dialogue learning environment to improve the quality of diagnostic dialogue across a multitude of disease conditions, specialities and patient contexts. |
We tested performance in consultations with simulated patients (played by trained actors), compared to those performed by 20 real PCPs using the randomized approach described above. AMIE and PCPs were assessed from the perspectives of both specialist attending physicians and our simulated patients in a randomized, blinded crossover study that included 149 case scenarios from OSCE providers in Canada, the UK and India in a diverse range of specialties and diseases.
Notably, our study was not designed to emulate either traditional in-person OSCE evaluations or the ways clinicians usually use text, email, chat or telemedicine. Instead, our experiment mirrored the most common way consumers interact with LLMs today, a potentially scalable and familiar mechanism for AI systems to engage in remote diagnostic dialogue.
![]() |
Overview of the randomized study design to perform a virtual remote OSCE with simulated patients via online multi-turn synchronous text chat. |
Performance of AMIE
In this setting, we observed that AMIE performed simulated diagnostic conversations at least as well as PCPs when both were evaluated along multiple clinically-meaningful axes of consultation quality. AMIE had greater diagnostic accuracy and superior performance for 28 of 32 axes from the perspective of specialist physicians, and 24 of 26 axes from the perspective of patient actors.
![]() |
AMIE outperformed PCPs on multiple evaluation axes for diagnostic dialogue in our evaluations. |
![]() |
Specialist-rated top-k diagnostic accuracy. AMIE and PCPs top-k differential diagnosis (DDx) accuracy are compared across 149 scenarios with respect to the ground truth diagnosis (a) and all diagnoses listed within the accepted differential diagnoses (b). Bootstrapping (n=10,000) confirms all top-k differences between AMIE and PCP DDx accuracy are significant with p <0.05 after false discovery rate (FDR) correction. |
![]() |
Diagnostic conversation and reasoning qualities as assessed by specialist physicians. On 28 out of 32 axes, AMIE outperformed PCPs while being comparable on the rest. |
Limitations
Our research has several limitations and should be interpreted with appropriate caution. Firstly, our evaluation technique likely underestimates the real-world value of human conversations, as the clinicians in our study were limited to an unfamiliar text-chat interface, which permits large-scale LLM–patient interactions but is not representative of usual clinical practice. Secondly, any research of this type must be seen as only a first exploratory step on a long journey. Transitioning from a LLM research prototype that we evaluated in this study to a safe and robust tool that could be used by people and those who provide care for them will require significant additional research. There are many important limitations to be addressed, including experimental performance under real-world constraints and dedicated exploration of such important topics as health equity and fairness, privacy, robustness, and many more, to ensure the safety and reliability of the technology.
AMIE as an aid to clinicians
In a recently released preprint, we evaluated the ability of an earlier iteration of the AMIE system to generate a DDx alone or as an aid to clinicians. Twenty (20) generalist clinicians evaluated 303 challenging, real-world medical cases sourced from the New England Journal of Medicine (NEJM) ClinicoPathologic Conferences (CPCs). Each case report was read by two clinicians randomized to one of two assistive conditions: either assistance from search engines and standard medical resources, or AMIE assistance in addition to these tools. All clinicians provided a baseline, unassisted DDx prior to using the respective assistive tools.
![]() |
Assisted randomized reader study setup to investigate the assistive effect of AMIE to clinicians in solving complex diagnostic case challenges from the New England Journal of Medicine. |
AMIE exhibited standalone performance that exceeded that of unassisted clinicians (top-10 accuracy 59.1% vs. 33.6%, p= 0.04). Comparing the two assisted study arms, the top-10 accuracy was higher for clinicians assisted by AMIE, compared to clinicians without AMIE assistance (24.6%, p<0.01) and clinicians with search (5.45%, p=0.02). Further, clinicians assisted by AMIE arrived at more comprehensive differential lists than those without AMIE assistance.
It’s worth noting that NEJM CPCs are not representative of everyday clinical practice. They are unusual case reports in only a few hundred individuals so offer limited scope for probing important issues like equity or fairness.
Bold and responsible research in healthcare — the art of the possible
Access to clinical expertise remains scarce around the world. While AI has shown great promise in specific clinical applications, engagement in the dynamic, conversational diagnostic journeys of clinical practice requires many capabilities not yet demonstrated by AI systems. Doctors wield not only knowledge and skill but a dedication to myriad principles, including safety and quality, communication, partnership and teamwork, trust, and professionalism. Realizing these attributes in AI systems is an inspiring challenge that should be approached responsibly and with care. AMIE is our exploration of the “art of the possible”, a research-only system for safely exploring a vision of the future where AI systems might be better aligned with attributes of the skilled clinicians entrusted with our care. It is early experimental-only work, not a product, and has several limitations that we believe merit rigorous and extensive further scientific studies in order to envision a future in which conversational, empathic and diagnostic AI systems might become safe, helpful and accessible.
Acknowledgements
The research described here is joint work across many teams at Google Research and Google Deepmind. We are grateful to all our co-authors – Tao Tu, Mike Schaekermann, Anil Palepu, Daniel McDuff, Jake Sunshine, Khaled Saab, Jan Freyberg, Ryutaro Tanno, Amy Wang, Brenna Li, Mohamed Amin, Sara Mahdavi, Karan Sighal, Shekoofeh Azizi, Nenad Tomasev, Yun Liu, Yong Cheng, Le Hou, Albert Webson, Jake Garrison, Yash Sharma, Anupam Pathak, Sushant Prakash, Philip Mansfield, Shwetak Patel, Bradley Green, Ewa Dominowska, Renee Wong, Juraj Gottweis, Dale Webster, Katherine Chou, Christopher Semturs, Joelle Barral, Greg Corrado and Yossi Matias. We also thank Sami Lachgar, Lauren Winer and John Guilyard for their support with narratives and the visuals. Finally, we are grateful to Michael Howell, James Maynika, Jeff Dean, Karen DeSalvo, Zoubin Gharahmani and Demis Hassabis for their support during the course of this project.
Build financial search applications using the Amazon Bedrock Cohere multilingual embedding model
Enterprises have access to massive amounts of data, much of which is difficult to discover because the data is unstructured. Conventional approaches to analyzing unstructured data use keyword or synonym matching. They don’t capture the full context of a document, making them less effective in dealing with unstructured data.
In contrast, text embeddings use machine learning (ML) capabilities to capture the meaning of unstructured data. Embeddings are generated by representational language models that translate text into numerical vectors and encode contextual information in a document. This enables applications such as semantic search, Retrieval Augmented Generation (RAG), topic modeling, and text classification.
For example, in the financial services industry, applications include extracting insights from earnings reports, searching for information from financial statements, and analyzing sentiment about stocks and markets found in financial news. Text embeddings enable industry professionals to extract insights from documents, minimize errors, and increase their performance.
In this post, we showcase an application that can search and query across financial news in different languages using Cohere’s Embed and Rerank models with Amazon Bedrock.
Cohere’s multilingual embedding model
Cohere is a leading enterprise AI platform that builds world-class large language models (LLMs) and LLM-powered solutions that allow computers to search, capture meaning, and converse in text. They provide ease of use and strong security and privacy controls.
Cohere’s multilingual embedding model generates vector representations of documents for over 100 languages and is available on Amazon Bedrock. This allows AWS customers to access it as an API, which eliminates the need to manage the underlying infrastructure and ensures that sensitive information remains securely managed and protected.
The multilingual model groups text with similar meanings by assigning them positions that are close to each other in a semantic vector space. With a multilingual embedding model, developers can process text in multiple languages without the need to switch between different models, as illustrated in the following figure. This makes processing more efficient and improves performance for multilingual applications.
The following are some of the highlights of Cohere’s embedding model:
- Focus on document quality – Typical embedding models are trained to measure similarity between documents, but Cohere’s model also measures document quality
- Better retrieval for RAG applications – RAG applications require a good retrieval system, which Cohere’s embedding model excels at
- Cost-efficient data compression – Cohere uses a special, compression-aware training method, resulting in substantial cost savings for your vector database
Use cases for text embedding
Text embeddings turn unstructured data into a structured form. This allows you to objectively compare, dissect, and derive insights from all of these documents. The following are example use cases that Cohere’s embedding model enables:
- Semantic search – Enables powerful search applications when coupled with a vector database, with excellent relevance based on search phrase meaning
- Search engine for a larger system – Finds and retrieves the most relevant information from connected enterprise data sources for RAG systems
- Text classification – Supports intent recognition, sentiment analysis, and advanced document analysis
- Topic modeling – Turns a collection of documents into distinct clusters to uncover emerging topics and themes
Enhanced search systems with Rerank
In enterprises where conventional keyword search systems are already present, how do you introduce modern semantic search capabilities? For such systems that have been part of a company’s information architecture for a long time, a complete migration to an embeddings-based approach is, in many cases, just not feasible.
Cohere’s Rerank endpoint is designed to bridge this gap. It acts as the second stage of a search flow to provide a ranking of relevant documents per a user’s query. Enterprises can retain an existing keyword (or even semantic) system for the first-stage retrieval and boost the quality of search results with the Rerank endpoint in the second-stage reranking.
Rerank provides a fast and straightforward option for improving search results by introducing semantic search technology into a user’s stack with a single line of code. The endpoint also comes with multilingual support. The following figure illustrates the retrieval and reranking workflow.
Solution overview
Financial analysts need to digest a lot of content, such as financial publications and news media, in order to stay informed. According to the Association for Financial Professionals (AFP), financial analysts spend 75% of their time gathering data or administering the process instead of added-value analysis. Finding the answer to a question across a variety of sources and documents is time-intensive and tedious work. The Cohere embedding model helps analysts quickly search across numerous article titles in multiple languages to find and rank the articles that are most relevant to a particular query, saving an enormous amount of time and effort.
In the following use case example, we showcase how Cohere’s Embed model searches and queries across financial news in different languages in one unique pipeline. Then we demonstrate how adding Rerank to your embeddings retrieval (or adding it to a legacy lexical search) can further improve results.
The supporting notebook is available on GitHub.
The following diagram illustrates the workflow of the application.
Enable model access through Amazon Bedrock
Amazon Bedrock users need to request access to models to make them available for use. To request access to additional models, choose Model access the navigation pane on the Amazon Bedrock console. For more information, see Model access. For this walkthrough, you need to request access to the Cohere Embed Multilingual model.
Install packages and import modules
First, we install the necessary packages and import the modules we’ll use in this example:
Import documents
We use a dataset (MultiFIN) containing a list of real-world article headlines covering 15 languages (English, Turkish, Danish, Spanish, Polish, Greek, Finnish, Hebrew, Japanese, Hungarian, Norwegian, Russian, Italian, Icelandic, and Swedish). This is an open source dataset curated for financial natural language processing (NLP) and is available on a GitHub repository.
In our case, we’ve created a CSV file with MultiFIN’s data as well as a column with translations. We don’t use this column to feed the model; we use it to help us follow along when we print the results for those who don’t speak Danish or Spanish. We point to that CSV to create our dataframe:
Select a list of documents to query
MultiFIN has over 6,000 records in 15 different languages. For our example use case, we focus on three languages: English, Spanish, and Danish. We also sort the headers by length and pick the longest ones.
Because we’re picking the longest articles, we ensure the length is not due to repeated sequences. The following code shows an example where that is the case. We will clean that up.
df['text'].iloc[2215]
Our list of documents is nicely distributed across the three languages:
The following is the longest article header in our dataset:
Embed and index documents
Now, we want to embed our documents and store the embeddings. The embeddings are very large vectors that encapsulate the semantic meaning of our document. In particular, we use Cohere’s embed-multilingual-v3.0 model, which creates embeddings with 1,024 dimensions.
When a query is passed, we also embed the query and use the hnswlib library to find the closest neighbors.
It only takes a few lines of code to establish a Cohere client, embed the documents, and create the search index. We also keep track of the language and translation of the document to enrich the display of the results.
Build a retrieval system
Next, we build a function that takes a query as input, embeds it, and finds the four headers more closely related to it:
Query the retrieval system
Let’s explore what our system does with a couple of different queries. We start with English:
The results are as follows:
Notice the following:
- We’re asking related, but slightly different questions, and the model is nuanced enough to present the most relevant results at the top.
- Our model does not perform keyword-based search, but semantic search. Even if we’re using a term like “data science” instead of “AI,” our model is able to understand what’s being asked and return the most relevant result at the top.
How about a query in Danish? Let’s look at the following query:
In the preceding example, the English acronym “PP&E” stands for “property, plant, and equipment,” and our model was able to connect it to our query.
In this case, all returned results are in Danish, but the model can return a document in a language other than the query if its semantic meaning is closer. We have complete flexibility, and with a few lines of code, we can specify whether the model should only look at documents in the language of the query, or whether it should look at all documents.
Improve results with Cohere Rerank
Embeddings are very powerful. However, we’re now going to look at how to refine our results even further with Cohere’s Rerank endpoint, which has been trained to score the relevancy of documents against a query.
Another advantage of Rerank is that it can work on top of a legacy keyword search engine. You don’t have to change to a vector database or make drastic changes to your infrastructure, and it only takes a few lines of code. Rerank is available in Amazon SageMaker.
Let’s try a new query. We use SageMaker this time:
In this case, a semantic search was able to retrieve our answer and display it in the results, but it’s not at the top. However, when we pass the query again to our Rerank endpoint with the list of docs retrieved, Rerank is able to surface the most relevant document at the top.
First, we create the client and the Rerank endpoint:
When we pass the documents to Rerank, the model is able to pick the most relevant one accurately:
Conclusion
This post presented a walkthrough of using Cohere’s multilingual embedding model in Amazon Bedrock in the financial services domain. In particular, we demonstrated an example of a multilingual financial articles search application. We saw how the embedding model enables efficient and accurate discovery of information, thereby boosting the productivity and output quality of an analyst.
Cohere’s multilingual embedding model supports over 100 languages. It removes the complexity of building applications that require working with a corpus of documents in different languages. The Cohere Embed model is trained to deliver results in real-world applications. It handles noisy data as inputs, adapts to complex RAG systems, and delivers cost-efficiency from its compression-aware training method.
Start building with Cohere’s multilingual embedding model in Amazon Bedrock today.
About the Authors
James Yi is a Senior AI/ML Partner Solutions Architect in the Technology Partners COE Tech team at Amazon Web Services. He is passionate about working with enterprise customers and partners to design, deploy, and scale AI/ML applications to derive business value. Outside of work, he enjoys playing soccer, traveling, and spending time with his family.
Gonzalo Betegon is a Solutions Architect at Cohere, a provider of cutting-edge natural language processing technology. He helps organizations address their business needs through the deployment of large language models.
Meor Amer is a Developer Advocate at Cohere, a provider of cutting-edge natural language processing (NLP) technology. He helps developers build cutting-edge applications with Cohere’s Large Language Models (LLMs).
Can large language models identify and correct their mistakes?
LLMs are increasingly popular for reasoning tasks, such as multi-turn QA, task completion, code generation, or mathematics. Yet much like people, they do not always solve problems correctly on the first try, especially on tasks for which they were not trained. Therefore, for such systems to be most useful, they should be able to 1) identify where their reasoning went wrong and 2) backtrack to find another solution.
This has led to a surge in methods related to self-correction, where an LLM is used to identify problems in its own output, and then produce improved results based on the feedback. Self-correction is generally thought of as a single process, but we decided to break it down into two components, mistake finding and output correction.
In “LLMs cannot find reasoning errors, but can correct them!”, we test state-of-the-art LLMs on mistake finding and output correction separately. We present BIG-Bench Mistake, an evaluation benchmark dataset for mistake identification, which we use to address the following questions:
- Can LLMs find logical mistakes in Chain-of-Thought (CoT) style reasoning?
- Can mistake-finding be used as a proxy for correctness?
- Knowing where the mistake is, can LLMs then be prompted to backtrack and arrive at the correct answer?
- Can mistake finding as a skill generalize to tasks the LLMs have never seen?
About our dataset
Mistake finding is an underexplored problem in natural language processing, with a particular lack of evaluation tasks in this domain. To best assess the ability of LLMs to find mistakes, evaluation tasks should exhibit mistakes that are non-ambiguous. To our knowledge, most current mistake-finding datasets do not go beyond the realm of mathematics for this reason.
To assess the ability of LLMs to reason about mistakes outside of the math domain, we produce a new dataset for use by the research community, called BIG-Bench Mistake. This dataset consists of Chain-of-Thought traces generated using PaLM 2 on five tasks in BIG-Bench. Each trace is annotated with the location of the first logical mistake.
To maximize the number of mistakes in our dataset, we sample 255 traces where the answer is incorrect (so we know there is definitely a mistake), and 45 traces where the answer is correct (so there may or may not be a mistake). We then ask human labelers to go through each trace and identify the first mistake step. Each trace has been annotated by at least three labelers, whose answers had inter-rater reliability levels of >0.98 (using Krippendorff’s α). The labeling was done for all tasks except the Dyck Languages task, which involves predicting the sequence of closing parentheses for a given input sequence. This task we labeled algorithmically.
The logical errors made in this dataset are simple and unambiguous, providing a good benchmark for testing an LLM’s ability to find its own mistakes before using them on harder, more ambiguous tasks.
Core questions about mistake identification
1. Can LLMs find logical mistakes in Chain-of-Thought style reasoning?
First, we want to find out if LLMs can identify mistakes independently of their ability to correct them. We attempt multiple prompting methods to test GPT series models for their ability to locate mistakes (prompts here) under the assumption that they are generally representative of modern LLM performance.
Generally, we found these state-of-the-art models perform poorly, with the best model achieving 52.9% accuracy overall. Hence, there is a need to improve LLMs’ ability in this area of reasoning.
In our experiments, we try three different prompting methods: direct (trace), direct (step) and CoT (step). In direct (trace), we provide the LLM with the trace and ask for the location step of the mistake or no mistake. In direct (step), we prompt the LLM to ask itself this question for each step it takes. In CoT (step), we prompt the LLM to give its reasoning for whether each step is a mistake or not a mistake.
![]() |
A diagram showing the three prompting methods direct (trace), direct (step) and CoT (step). |
Our finding is in line and builds upon prior results, but goes further in showing that LLMs struggle with even simple and unambiguous mistakes (for comparison, our human raters without prior expertise solve the problem with a high degree of agreement). We hypothesize that this is a big reason why LLMs are unable to self-correct reasoning errors. See the paper for the full results.
2. Can mistake-finding be used as a proxy for correctness of the answer?
When people are confronted with a problem where we are unsure of the answer, we can work through our solutions step-by-step. If no error is found, we can make the assumption that we did the right thing.
While we hypothesized that this would work similarly for LLMs, we discovered that this is a poor strategy. On our dataset of 85% incorrect traces and 15% correct traces, using this method is not much better than the naïve strategy of always labeling traces as incorrect, which gives a weighted average F1 of 78.
![]() |
A diagram showing how well mistake-finding with LLMs can be used as a proxy for correctness of the answer on each dataset. |
3. Can LLMs backtrack knowing where the error is?
Since we’ve shown that LLMs exhibit poor performance in finding reasoning errors in CoT traces, we want to know whether LLMs can even correct errors at all, even if they know where the error is.
Note that knowing the mistake location is different from knowing the right answer: CoT traces can contain logical mistakes even if the final answer is correct, or vice versa. In most real-world situations, we won’t know what the right answer is, but we might be able to identify logical errors in intermediate steps.
We propose the following backtracking method:
- Generate CoT traces as usual, at temperature = 0. (Temperature is a parameter that controls the randomness of generated responses, with higher values producing more diverse and creative outputs, usually at the expense of quality.)
- Identify the location of the first logical mistake (for example with a classifier, or here we just use labels from our dataset).
- Re-generate the mistake step at temperature = 1 and produce a set of eight outputs. Since the original output is known to lead to incorrect results, the goal is to find an alternative generation at this step that is significantly different from the original.
- From these eight outputs, select one that is different from the original mistake step. (We just use exact matching here, but in the future this can be something more sophisticated.)
- Using the new step, generate the rest of the trace as normal at temperature = 0.
It’s a very simple method that does not require any additional prompt crafting and avoids having to re-generate the entire trace. We test it using the mistake location data from BIG-Bench Mistake, and we find that it can correct CoT errors.
Recent work showed that self-correction methods, like Reflexion and RCI, cause deterioration in accuracy scores because there are more correct answers becoming incorrect than vice versa. Our method, on the other hand, produces more gains (by correcting wrong answers) than losses (by changing right answers to wrong answers).
We also compare our method with a random baseline, where we randomly assume a step to be a mistake. Our results show that this random baseline does produce some gains, but not as much as backtracking with the correct mistake location, and with more losses.
![]() |
A diagram showing the gains and losses in accuracy for our method as well as a random baseline on each dataset. |
4. Can mistake finding generalize to tasks the LLMs have never seen?
To answer this question, we fine-tuned a small model on four of the BIG-Bench tasks and tested it on the fifth, held-out task. We do this for every task, producing five fine-tuned models in total. Then we compare the results with just zero-shot prompting PaLM 2-L-Unicorn, a much larger model.
![]() |
Bar chart showing the accuracy improvement of the fine-tuned small model compared to zero-shot prompting with PaLM 2-L-Unicorn. |
Our results show that the much smaller fine-tuned reward model generally performs better than zero-shot prompting a large model, even though the reward model has never seen data from the task in the test set. The only exception is logical deduction, where it performs on par with zero-shot prompting.
This is a very promising result as we can potentially just use a small fine-tuned reward model to perform backtracking and improve accuracy on any task, even if we don’t have the data for it. This smaller reward model is completely independent of the generator LLM, and can be updated and further fine-tuned for individual use cases.
![]() |
An illustration showing how our backtracking method works. |
Conclusion
In this work, we created an evaluation benchmark dataset that the wider academic community can use to evaluate future LLMs. We further showed that LLMs currently struggle to find logical errors. However, if they could, we show the effectiveness of backtracking as a strategy that can provide gains on tasks. Finally, a smaller reward model can be trained on general mistake-finding tasks and be used to improve out-of-domain mistake finding, showing that mistake-finding can generalize.
Acknowledgements
Thank you to Peter Chen, Tony Mak, Hassan Mansoor and Victor Cărbune for contributing ideas and helping with the experiments and data collection. We would also like to thank Sian Gooding and Vicky Zayats for their comments and suggestions on the paper.
Ball position tracking in the cloud with the PGA TOUR
The PGA TOUR continues to enhance the golf experience with real-time data that brings fans closer to the game. To deliver even richer experiences, they are pursuing the development of a next-generation ball position tracking system that automatically tracks the position of the ball on the green.
The TOUR currently uses ShotLink powered by CDW, a premier scoring system that uses a complex camera system with on-site compute, to closely track the start and end position of every shot. The TOUR wanted to explore computer vision and machine learning (ML) techniques to develop a next-generation cloud-based pipeline to locate golf balls on the putting green.
The Amazon Generative AI Innovation Center (GAIIC) demonstrated the effectiveness of these techniques in an example dataset from a recent PGA TOUR event. The GAIIC designed a modular pipeline cascading a series of deep convolutional neural networks that successfully localizes players within a camera’s field of view, determines which player is putting, and tracks the ball as it moves toward the cup.
In this post, we describe the development of this pipeline, the raw data, the design of the convolutional neural networks comprising the pipeline, and an evaluation of its performance.
Data
The TOUR provided 3 days of continuous video from a recent tournament from three 4K cameras positioned around the green on one hole. The following figure shows a frame from one camera cropped and zoomed so that the player putting is easily visible. Note that despite the high resolution of the cameras, because of the distance from the green, the ball appears small (usually 3×3, 4×4 or 5×5 pixels), and targets of this size can be difficult to localize accurately.
In addition to the camera feeds, the TOUR provided the GAIIC with annotated scoring data on each shot, including world location of its resting position and the timestamp. This allowed for visualizations of every putt on the green, as well as the ability to pull all of the video clips of players putting, which could be manually labeled and used to train detection models that make up the pipeline. The following figure show the three camera views with approximate putt path overlays, counterclockwise from top left. The pin is moved each day, where day 1 corresponds to blue, day 2 to red, and day 3 to orange.
Pipeline overview
The overall system consists of both a training pipeline an inference pipeline. The following diagram illustrates the architecture of the training pipeline. The starting point is ingestion of video data, either from a streaming module like Amazon Kinesis for live video or placement directly into Amazon Simple Storage Service (Amazon S3) for historical video. The training pipeline requires video preprocessing and hand labeling of images with Amazon SageMaker Ground Truth. Models can be trained with Amazon SageMaker and their artifacts stored with Amazon S3.
The inference pipeline, shown in the following diagram, consists of a number of modules that successively extract information from the raw video and ultimately predict the world coordinates of the ball at rest. Initially, the green is cropped from the larger field of view from each camera, in order to cut down on the pixel area in which the models must search for players and balls. Next, a deep convolutional neural network (CNN) is used to find the locations of people in the field of view. Another CNN is used to predict which type of person has been found in order to determine whether anyone is about to putt. After a likely putter has been localized in the field of view, the same network is used to predict the location of the ball near the putter. A third CNN tracks the ball during its motion, and lastly, a transformation function from camera pixel position to GPS coordinates is applied.
Player detection
Although it would be possible to run a CNN for ball detection over an entire 4K frame at a set interval, given the angular size of the ball at these camera distances, any small white object triggers a detection, resulting in many false alarms. To avoid searching the entire image frame for the ball, it’s possible to take advantage of correlations between player pose and ball location. A ball that is about to be putted must be next to a player, so finding the players in the field of view will greatly restrict the pixel area in which the detector must search for the ball.
We were able to use a CNN that was pre-trained to predict bounding boxes around all the people in a scene, as shown in the following figure. Unfortunately, there is frequently more than one ball on the green, so further logic is required beyond simply finding all people and searching for a ball. This requires another CNN to find the player that was currently putting.
Player classification and ball detection
To further narrow down where the ball could be, we fine-tuned a pre-trained object-detection CNN (YOLO v7) to classify all the people on the green. An important component of this process was manually labeling a set of images using SageMaker Ground Truth. The labels allowed the CNN to classify the player putting with high accuracy. In the labeling process, the ball was also outlined along with the player putting, so this CNN was able to perform ball detection as well, drawing an initial bounding box around the ball before a putt and feeding the position information into the downstream ball tracking CNN.
We use four different labels to annotate the objects in the images:
- player-putting – The player holding a club and in the putting position
- player-not-putting – The player not in the putting position (may also be holding a club)
- other-person – Any other person who is not a player
- golf-ball – The golf ball
The following figure shows a CNN was fine-tuned using labels from SageMaker Ground Truth to classify each person in the field of view. This is difficult because of the wide range of visual appearances of players, caddies, and fans. After a player was classified as putting, a CNN fine-tuned for ball detection was applied to the small area immediately around that player.
Ball path tracking
A third CNN, a ResNet architecture pre-trained for motion tracking, was used for tracking the ball after it was putted. Motion tracking is a thoroughly researched problem, so this network performed well when integrated into the pipeline without further fine-tuning.
Pipeline output
The cascade of CNNs places bounding boxes around people, classifies people on the green, detects the initial ball position, and tracks the ball once it begins moving. The following figure shows the labeled video output of the pipeline. The pixel positions of the ball as it moves are tracked and recorded. Note that people on the green are being tracked and outlined by bounding boxes; the putter at the bottom is labeled correctly as “player putting,” and the moving ball is being tracked and outlined by a small blue bounding box.
Performance
To assess performance of components of the pipeline, it’s necessary to have labeled data. Although we were provided with the ground truth world position of the ball, we didn’t have intermediate points for ground truth, like the final pixel position of the ball or the pixel location of the player putting. With the labeling job that we carried out, we developed ground truth data for these intermediate outputs of the pipeline that allow us to measure performance.
Player classification and ball detection accuracy
For detection of the player putting and the initial ball location, we labeled a dataset and fine-tuned a YOLO v7 CNN model as described earlier. The model classified the output from the previous person detection module into four classes: a player putting, a player not putting, other people, and the golf ball, as shown in the following figure.
The performance of this module is assessed with a confusion matrix, shown in the following figure. The values in the diagonal boxes show how often the predicted class matched the actual class from the ground truth labels. The model has 89% recall or better for each person class, and 79% recall for golf balls (which is to be expected because the model is pre-trained on examples with people but not on examples with golf balls; this could be improved with more labeled golf balls in the training set).
The next step is to trigger the ball tracker. Because the ball detection output is a confidence probability, it’s also possible to set the threshold for “detected ball” and observe how that changes the results, summarized in the following figure. There is a trade-off in this method because a higher threshold will necessarily have fewer false alarms but also miss some of the less certain examples of balls. We tested thresholds of 20% and 50% confidence, and found ball detection at 78% and 61%, respectively. By this measure, the 20% threshold is better. The trade-off is apparent in that for the 20% confidence threshold, 80% of total detections were actually balls (20% false positive), whereas for the 50% confidence threshold, 90% were balls (10% false positive). For fewer false positives, the 50% confidence threshold is better. Both of these measures could be improved with more labeled data for a larger training set.
The detection pipeline throughput is on the order of 10 frames per second, so in its current form, a single instance is not fast enough to be run continuously on the input at 50 frames per second. Achieving the 7-second mark for output after the ball steps would require further optimization for latency, perhaps by running multiple versions of the pipeline in parallel and compressing the CNN models via quantization (for example).
Ball path tracking accuracy
The pre-trained CNN model from MMTracking works well, but there are interesting failure cases. The following figure shows a case where the tracker starts on the ball, expands its bounding box to include both the putter head and ball, and then unfortunately tracks the putter head and forgets the ball. In this case, the putter head appears white (possibly due to specular reflection), so the confusion is understandable; labeled data for tracking and fine-tuning of the tracking CNN could help improve this in the future.
Conclusion
In this post, we discussed the development of a modular pipeline that localizes players within a camera’s field of view, determines which player is putting, and tracks the ball as it moves toward the cup.
For more information about AWS collaboration with the PGA TOUR, refer to PGA TOUR tees up with AWS to reimagine the fan experience.
About the Authors
James Golden is an applied scientist at Amazon Bedrock with a background in machine learning and neuroscience.
Henry Wang is an applied scientist at Amazon Generative AI Innovation Center, where he researches and builds generative AI solutions for AWS customers. He focuses on sports and media & entertainment industries, and has worked with various sports leagues, teams and broadcasters in the past. During his spare time, he likes to play tennis and golf.
Tryambak Gangopadhyay is an Applied Scientist at the AWS Generative AI Innovation Center, where he collaborates with organizations across a diverse spectrum of industries. His role involves conducting research and developing Generative AI solutions to address crucial business challenges and accelerate AI adoption.
TaskWeaver: A code-first agent framework for efficient data analytics and domain adaptation

The advent of large language models (LLMs) has revolutionized human-machine interactions, particularly in natural language understanding and generation applications. These AI- or LLM-backed virtual assistants hold the promise of serving as intelligent agents capable of autonomously reasoning, observing, and performing tasks articulated in natural language. However, it is still challenging for most agent frameworks to efficiently handle complex data structures (e.g., DataFrame), which are prevalent in data analytics tasks and domain-specific scenarios.
To address these challenges, we introduce TaskWeaver – a code-first agent framework which can convert natural language user requests into executable code, with additional support for rich data structures, dynamic plugin selection, and domain-adapted planning process. Now publicly available as an open-source framework, TaskWeaver leverages LLMs’ coding capability to implement complex logic and incorporates domain-specific knowledge through customizable examples and plugins. TaskWeaver empowers users to easily build their own virtual assistants that understand diverse domain questions, follow examples, and execute customizable algorithms on complex data structures efficiently.
Motivating example – Anomaly detection on time-series data
Scenario
Amy is a business analyst who wants to identify anomalies on a time series of sales data stored in a SQL database. She would like to get help from an AI assistant for the task with natural language interactions. Moreover, Amy would like to apply her own definition and interpretation of anomalies in the context of sales data, including a customized anomaly detection algorithm. Figure 1 shows the desired conversation between the user and the AI assistant – the AI assistant should be able to first pull the data from target database, then apply desired algorithms, and return the visualized results.
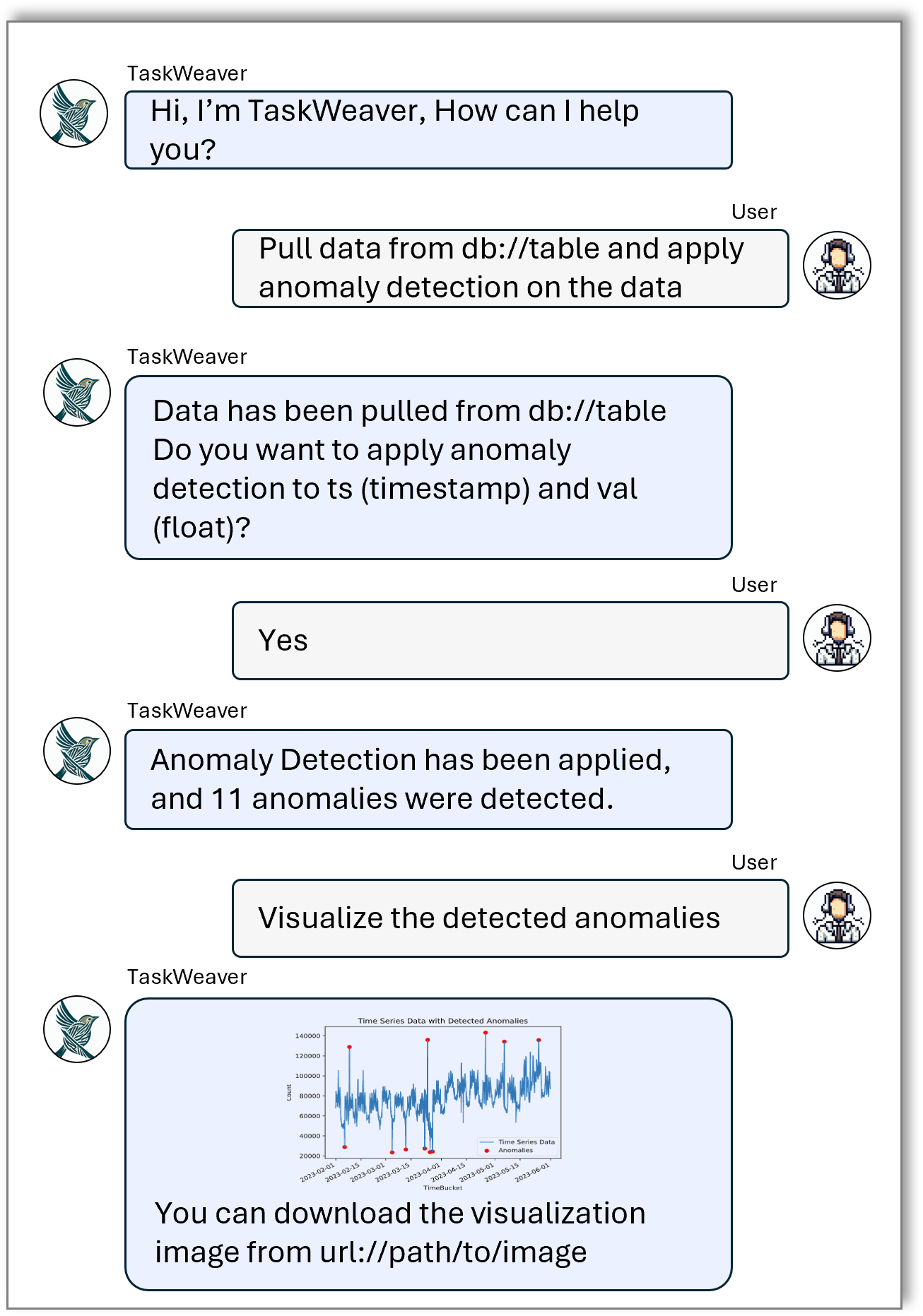
Requirements for an agent framework
To accomplish the task above, we identify several key requirements that current agent frameworks may lack:
- Plugin: The agent needs to first query and collect data from a database, and then detect anomalies using a specialized anomaly detection algorithm. Both require the capability to define and invoke custom plugins, e.g., the query_database plugin and the anomaly_detection plugin.
- Rich data structure: the agent should be capable of handling data in complex but common structures, such as array, matrix, tabular data (e.g., pandas DataFrame (opens in new tab)), to perform advanced data processing actions. Many existing works tend to transform the intermediate outputs as strings in the prompt or save them as local files before reading them again. However, this practice is error-prone and could easily exceed the prompt token limit. Additionally, data in rich structure should be able to transfer easily from one plugin to another.
- Stateful execution: The agent engages in iterative interactions with the user, processing user inputs and executing tasks accordingly. The execution states should be preserved throughout the entire conversation session across multiple chat rounds.
- Reasoning and acting (ReAct): The agent should have the capability to first observe and then act. The database might contain data of various schemas in the real world, leading to different arguments for anomaly detection. Therefore, the agent must first inspect the data schema, understand which columns are appropriate (and ask users to confirm), then feed the corresponding column names into the anomaly detection algorithm.
- Arbitrary code generation: The agent should be able to generate code to accommodate ad-hoc user demands, which are not covered by the pre-defined plugins. In the example provided, the agent generates code to visualize the detected anomalies without using any plugins.
- Incorporating domain knowledge: The agent should provide a systematic way to incorporate domain-specific knowledge. It would help LLMs deliver better planning and accurate tool calling, which in turn produces reliable results, particularly in domain-specific scenarios.
SPOTLIGHT: AI focus area
TaskWeaver architecture
Figure 2 shows the three core components in the TaskWeaver architecture. The Planner serves as the system’s entry point and interacts with the user. Its responsibilities include: (1) planning – breaking down the user’s request into subtasks and managing the execution process with self-reflection; and (2) responding – transforming the execution result into a human-readable response for the user. The Code Interpreter consists of two components: the Code Generator generates code for a given subtask from the Planner, considering existing plugins and domain-specific task examples; the Code Executor is responsible for executing the generated code and maintaining the execution state throughout the entire session.
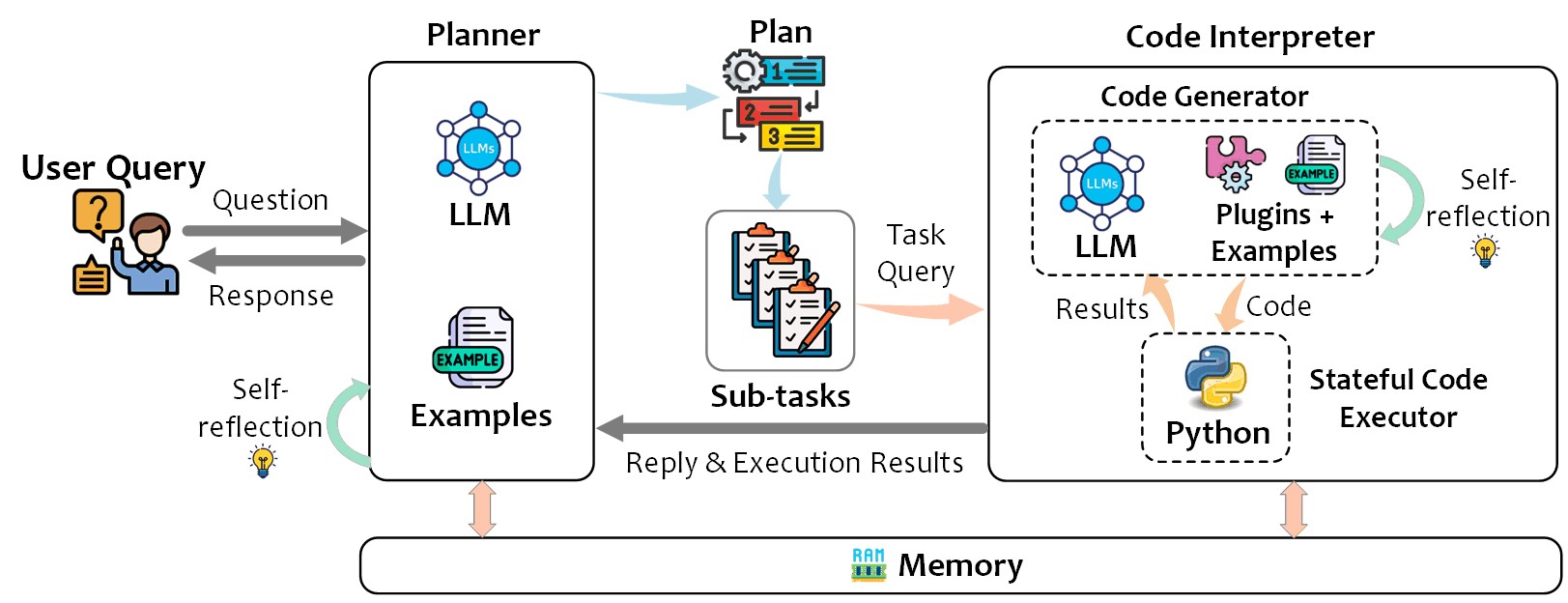
Running workflow for motivating example
TaskWeaver has a two-layer planning process for dealing with user requests. In the first layer, the Planner generates a high-level plan outlining the steps required to fulfill the request. In each subsequent round, the code generator will devise a plan, in terms of chain-of-thought and generated code, to execute the specified step. Figure 3 presents the internal workflow of TaskWeaver when accomplishing the motivating example mentioned above. Note that the prompts shown in Figure 3 are simplified and do not represent the full complex instructions.
The initial step involves the Planner taking the user query, Code Interpreter description, and planning examples (if provided) to generate a plan. For the given example, the plan first pulls data from the database and describes the data schema. The Code Generator prompt delineates its profile and competencies, providing definitions of all relevant plugins (e.g., function name, description, arguments and return values.) The output from the Code Generator is a code snippet that executes the sql_pull_data plugin, retrieves the data into a DataFrame, and provides a description of the data schema.
Next, the code generated is sent to the Code Executor for execution, after which the result is sent back to the Planner to determine the next planning step. In the example, the execution result reveals two columns, namely date and value, in the DataFrame. For the next step, the Planner can either confirm with the user if these columns correspond to the two input parameters of the anomaly_detection plugin, or directly proceed to the next step.
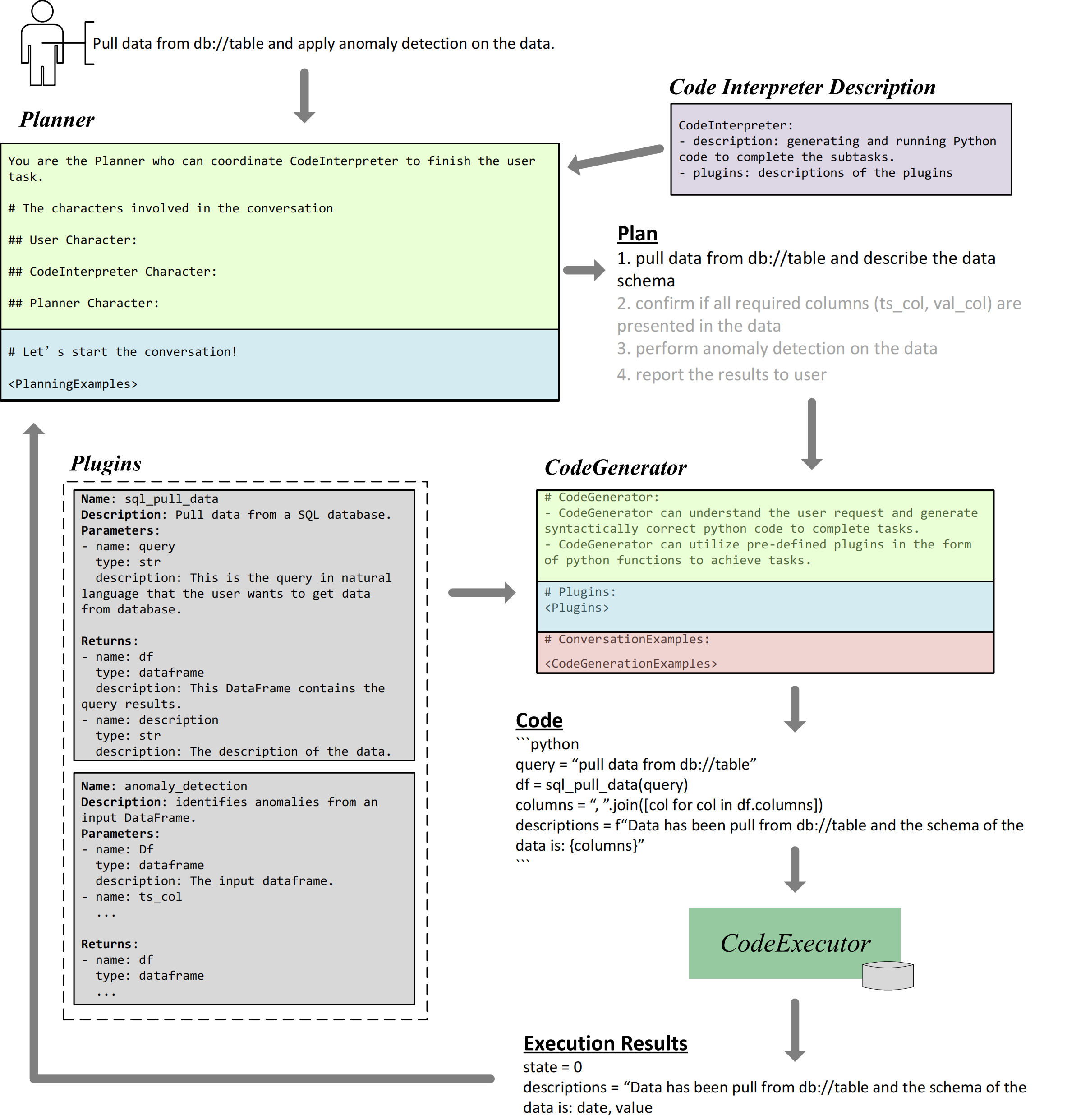
Key design considerations of TaskWeaver
- Code-first analytics experience: TaskWeaver converts user requests into Python programs that run on dedicated processes, where the Python program can be plugin invocations, arbitrary code to handle ad-hoc user queries, or both. Unlike other frameworks that rely on text or file-based expressions, TaskWeaver can fully utilize native data structures such as pandas DataFrame and numpy ndarray that exist in the memory. This makes it easy to perform tasks such as pulling data from a database, running machine learning algorithms (e.g., anomaly detection, classification, or clustering), summarizing results, and visualizing analysis outcomes.
- Domain adaptation: Incorporating domain-specific knowledge into the model via prompts can help boost LLMs’ performance when the user query is complex. TaskWeaver provides two options to make customizations with the user’s domain knowledge:
- Customization with plugins: Users can define custom plugins (including Python implementation and schema) to incorporate domain knowledge, such as pulling data from a specific database, and running a dedicated algorithm.
- Customization with examples: TaskWeaver also provides an easy-to-implement interface (in YAML format) for users to configure examples to teach the LLMs how to respond to certain requests. The examples can be of two categories: one is used for planning and the other is for code generation.
- Stateful code execution: When users make ad-hoc requests for data analytics, it often involves multiple iterations. As a result, TaskWeaver maintains the state of code execution throughout the entire session. This is like programming in Python using the Jupyter Notebook, where users type code snippets in a sequence of cells and the program’s internal state progresses sequentially. The difference in TaskWeaver is that users use natural language instead of programming language. TaskWeaver converts each user request into one or more code snippets in each round, depending on the specific plan.
- Others: TaskWeaver also supports other features such as intelligent plan decomposition and self-reflection to respond to a user’s request in a more reliable and organized manner. Moreover, features like restricted code generation can help limit the capabilities of the generated code to reduce security risks.
Getting started
TaskWeaver (opens in new tab) is now publicly available on GitHub. You may run the following commands to quickly get started.
git clone https://github.com/microsoft/TaskWeaver.git cd TaskWeaver pip install -r requirements.txt # install the requirements
Once the installation is finished, users can configure key parameters, such as the LLM endpoint, key and model, and start the TaskWeaver service easily by following the running examples (opens in new tab).
Other resources
- Video demos: The Demo Examples (opens in new tab) show two demo videos, the first one covers the motivating example presented above on anomaly detection; the second shows the case to forecast the price of Invesco QQQ Trust, an exchange-traded fund, over the next week.
- For more information, please refer to the TaskWeaver documentation. (opens in new tab)
- Technical report with mode details on design considerations: technical report.
The post TaskWeaver: A code-first agent framework for efficient data analytics and domain adaptation appeared first on Microsoft Research.
AI Takes Center Stage: Survey Reveals Financial Industry’s Top Trends for 2024
The financial services industry is undergoing a significant transformation with the adoption of AI technologies. NVIDIA’s fourth annual State of AI in Financial Services Report provides insights into the current landscape and emerging trends for 2024.
The report reveals that an overwhelming 91% of financial services companies are either assessing AI or already using it in production. These firms are using AI to drive innovation, improve operational efficiency and enhance customer experiences.
Portfolio optimization, fraud detection and risk management remain top AI use cases, while generative AI is quickly gaining popularity with organizations keen to uncover new efficiencies.
Below are the report’s key findings, which show how the financial services industry is evolving as advanced AI becomes more accessible.
Generative AI and Large Language Models Are on the Rise
Reflecting a macro-trend seen across industries, large language models (LLMs) and generative AI have emerged as significant areas of interest for financial services companies. Fifty-five percent of survey respondents reported that they were actively seeking generative AI workflows for their companies.
Organizations are exploring generative AI and LLMs for an array of applications ranging from marketing and sales — ad copy, email copy and content production — to synthetic data generation. Of these use cases, 37% of respondents showed interest in report generation, synthesis and investment research to cut down on repetitive manual work.
Customer experience and engagement was another sought-out use case, with a 34% response rate. This suggests that financial services institutions are exploring chatbots, virtual assistants and recommendation systems to enhance the customer experience.
AI Is Having an Impact Across Departments and Disciplines
With 75% of survey respondents considering their organization’s AI capabilities to be industry leading or middle of the pack, financial services organizations are becoming more confident in their ability to build, deploy and extract value from AI implementations.
The most popular uses for AI were in operations, risk and compliance, and marketing. To improve operational efficiency, financial organizations are using AI to automate manual processes, enhance data analysis and inform investment decisions.
To enhance risk and compliance, they’re deploying AI to analyze vast amounts of data to identify suspicious activities and anomalous transaction patterns. They’re also using AI to analyze customer data to predict preferences and deliver personalized marketing campaigns, educational content and targeted promotions.
Companies are already seeing results. Forty-three percent of financial services professionals indicated that AI had improved their operational efficiency, while 42% felt it had helped their business build a competitive advantage.
A Shift in the Headwinds
In previous years, the number one challenge respondents reported was recruiting AI experts and data scientists. A 30% increase this year in survey participants resoundingly responded that data-related challenges were the primary concern. This includes data privacy challenges, data sovereignty and data scattered around the globe governed by different oversight regulations.
The growing attention to these issues reflects the advancing power and complexity of AI models, which require huge, diverse datasets to train, as well as increasing regulatory scrutiny and emphasis on responsible AI.
Recruiting and retaining AI experts remains a challenge, as do budget concerns. But more than 60% of respondents are still planning to increase investment in computing infrastructure or optimizing AI workflows, underscoring the importance of these tools in quickly building and deploying trustworthy AI to overcome these barriers.
Paving the Way for Future Investments
By and large, the survey results paint a positive picture of AI bringing greater efficiency to operations, personalization to customer engagements, and precision to investment decisions.
Finance professionals agree. Eighty-six percent of respondents reported a positive impact on revenue, while 82% noted a reduction in costs. Fifty-one percent strongly agreed that AI would be important to their company’s future success, a 76% increase from last year.
With this positive outlook, 97% of companies plan to invest more in AI technologies in the near future. Focus areas for future investments include identifying additional AI use cases, optimizing AI workflows and increasing infrastructure spending.
To build and scale impactful AI across the enterprise, financial services organizations need a comprehensive AI platform that empowers data scientists, quants and developers to seamlessly collaborate while minimizing obstacles. To that end, executives are investing more in AI infrastructure and prioritizing high-yield AI use cases to improve employee productivity while delivering superior customer experiences and investment results.
Download the “State of AI in Financial Services: 2024 Trends” report for in-depth results and insights.
Explore NVIDIA’s AI solutions and enterprise-level AI platforms for delivering smarter, more secure financial services and the AI-powered bank.
To the Cloud and Beyond: New Activision and Blizzard Games, Day Passes and G-SYNC Technology Coming to GeForce NOW
GFN Thursday recaps the latest cloud announcements from CES 2024 — Day Pass memberships, Cloud G-SYNC technology, expanded NVIDIA Reflex support and more.
The new year brings new adventures to the cloud for members, including Diablo IV and Overwatch 2 from Blizzard, Exoprimal from Capcom, Honkai: Star Rail from HoYoverse and Pax Dei from Mainframe Industries.
Plus, no GFN Thursday is complete without new games. Get ready for ten new titles joining the cloud this week.
Cloud’s-Eye View of CES
CES 2024 has come to a close, and GeForce NOW members have a lot to look forward to.
Coming in February, day passes for Ultimate and Priority memberships will offer a new way for members to play at up to GeForce RTX 4080 quality for up to 24 hours. Ultimate Day Pass will be available for $7.99, and Priority Day Pass for $3.99, providing all the benefits of both memberships to gamers before they decide to commit to the better-value one-month or six-month memberships.
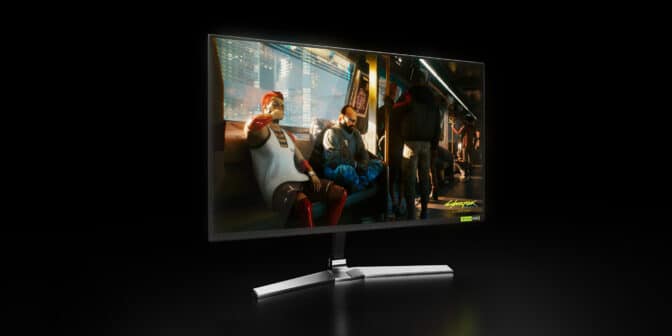
Cloud G-SYNC support will match the display refresh rate of variable refresh rate monitors and G-SYNC-compatible monitors to the streaming rate. Paired with new 60 and 120 frames per second streaming options for GeForce NOW Reflex mode, this makes cloud gaming experiences nearly indistinguishable from using a local PC.
Ultimate members will be able to turn their phones into portable gaming rigs with support for 1440p resolutions on compatible Android phones, as well as updated keyboard and mouse support connected through a USB hub. Thanks to the cloud, these smartphones are now capable of PC gaming at Ultimate quality.
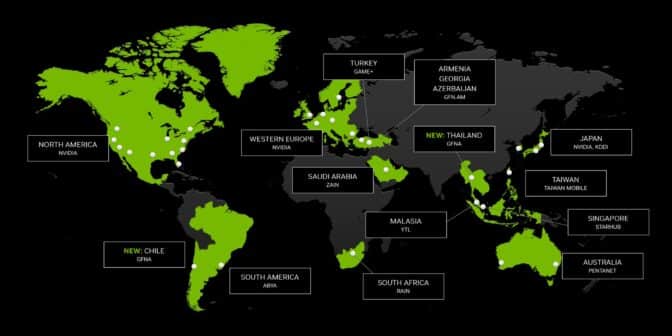
GeForce NOW will also soon expand to Japan, operating alongside GeForce NOW Alliance partner KDDI. This will enable gamers across the country to play their favorite PC games in the cloud with Ultimate performance. Learn more and sign up for notifications.
Here Comes the Blizzard
That’s not all: GeForce NOW is bringing even more top titles to the cloud from celebrated publishers.
Following the recent release of Call of Duty, the latest games from top developer Blizzard Entertainment are coming soon to GeForce NOW. Members will be able to play the Steam versions of Diablo IV and Overwatch 2 on nearly any device with the power of a GeForce RTX 4080 rig in the cloud, with support for Battle.net coming soon.
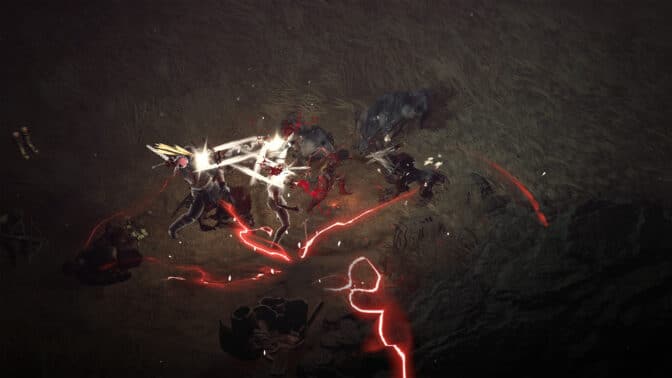
Join the fight for sanctuary in Diablo IV. Fight the forces of hell while discovering countless abilities to master, legendary loot to gather and nightmarish dungeons full of evil enemies to vanquish. Explore a shared open world where players can form their own armies to take down World Bosses, or join the fray in player vs. player zones to test skills against others.
Team up and answer the call of heroes in Overwatch 2, a free-to-play shooter featuring 30+ epic heroes, each with game-changing abilities. Lead the charge, ambush enemies or aid allies as one of Overwatch’s distinct heroes. Join the battle across dozens of futuristic maps inspired by real-world locations and master unique game modes in the always-on, ever-evolving live game.
Members can look forward to playing the Steam version of both games from the cloud, with support for the Battle.net launcher coming soon.
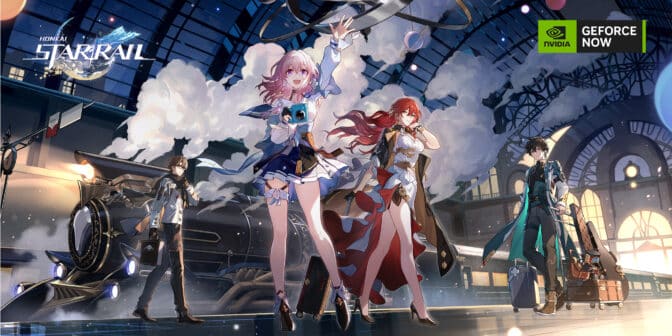
Expanding the library of hit free-to-play titles for members, Honkai: Star Rail from miHoYo will soon join Genshin Impact in the cloud. The space-fantasy role-playing game is set in a diverse universe filled with wonder, adventure and thrills. Plus, members can experience all the latest updates without worrying about download times.
Mainframe Industries’ Pax Dei is a highly anticipated social sandbox massively multiplayer online game inspired by legends of the medieval era. It’s planned to release on GeForce NOW when it launches for PC.
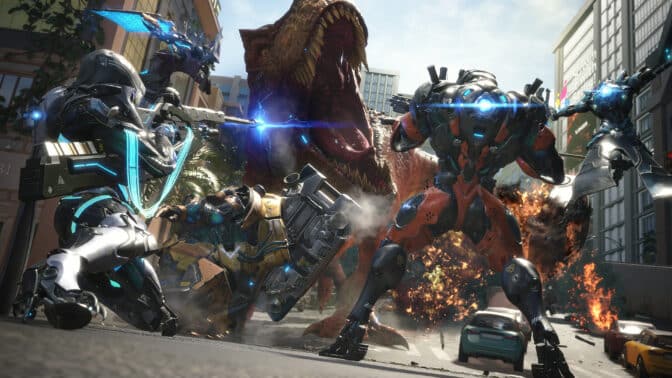
Capcom is working with NVIDIA to bring more of its hit titles to the cloud, including Exoprimal, an online, team-based action game that pits humanity’s cutting-edge exosuit technology against history’s most ferocious beasts: dinosaurs. Look forward to streaming it from the cloud starting Thursday, Jan. 18.
Get ready to play these titles and more at high performance coming soon. Ultimate members will be able to stream at up to 4K resolution and 120 fps with support for NVIDIA DLSS and Reflex technology, and experience the action even on low-powered devices. Keep an eye out on GFN Thursdays for the latest on game release dates in the cloud.
New to Play Today
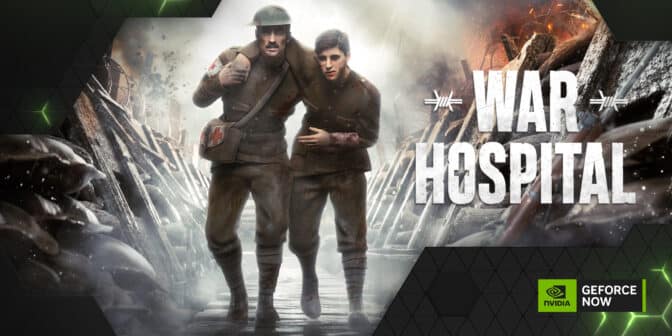
What’s a GFN Thursday without more games? Here’s what’s coming to the GeForce NOW library this week:
- War Hospital (New release Jan. 11, available on Steam)
- Assassin’s Creed: Valhalla (Xbox, available for PC Game Pass)
- Jected – Rivals (Steam)
- RAILGRADE (Steam)
- Survivalist: Invisible Strain (Steam)
- The Talos Principle 2 (Epic Games Store)
- Turbo Golf Racing (Xbox, available for PC Game Pass)
- TUNIC (Xbox, available for PC Game Pass)
- Witch It (Steam)
- Zombie Army 4: Dead War (Xbox, available for PC Game Pass)
Learn more about activating and playing Ubisoft games from PC Game Pass on GeForce NOW.
What are you playing this weekend? Let us know on X or in the comments below.