The model delivers dramatically enhanced performance, with a breakthrough in long-context understanding across modalities.Read More
Our next-generation model: Gemini 1.5
Gemini 1.5 delivers dramatically enhanced performance, with a breakthrough in long-context understanding across modalities.Read More
Artistry With Adobe: Creator Esteban Toro Delivers Inspirational Master Class Powered by AI and RTX
Adobe is putting generative AI into the hands of creators with Adobe Firefly — powered by NVIDIA in the cloud — and adding to its impressive app lineup with exciting new features.
The AI-powered Enhance Speech tool, available soon in Adobe Premiere Pro, is accelerated by NVIDIA RTX. This new feature removes unwanted noise and improves the quality of dialogue clips so they sound professionally recorded.
Esteban Toro, senior community relationship manager at Adobe and this week’s featured In the NVIDIA Studio artist, expertly wields AI-powered features in Adobe Photoshop and Lightroom to create his emotionally moving Cinematic Portraits series.
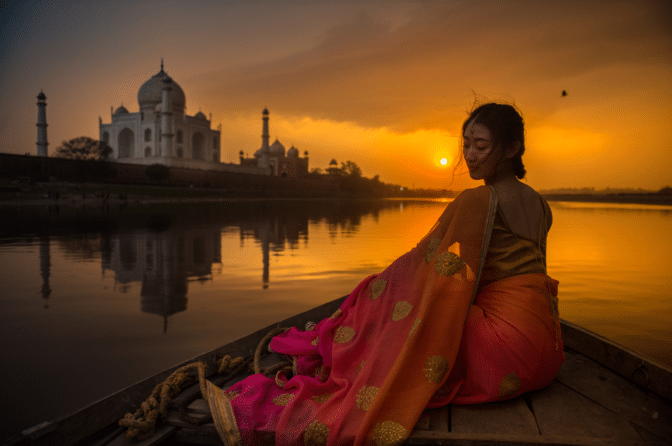
Have a Chat with RTX — the tech demo app that lets GeForce RTX owners personalize a generative pretrained transformer large language model connected to their own content, whether in documents, notes, videos or other data formats. Since it runs locally on a Windows RTX PC or workstation, results are fast and secure. Download Chat with RTX today.
Don’t forget GTC registration is open for virtual or in-person attendance. Running March 18-21 in San Jose, Calif., the event delivers something for every technical level and interest area, including sessions on how to power content creation using OpenUSD and generative AI.
And Omniverse OpenUSD month rolls on, spotlighting the open and extensible ecosystem for describing, composing, simulating and collaborating within 3D worlds. Follow NVIDIA Studio on Instagram, X and Facebook to learn more.
Storytelling With Adobe AI and RTX
The talented Toro is driven by stories.
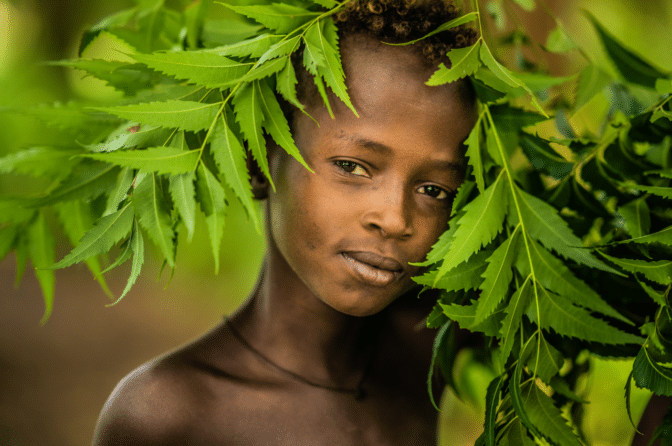
“Understanding how every person has a different upbringing and how the decisions they made took them to different places is absolutely inspiring,” said Toro. “When I discover a story worth telling, I just feel a necessity to tell it — and tell it right.”
It’s those stories that gave rise to Cinematic Portraits, a photo and video collection of people Toro’s befriended, such as Korean painter Kim Nam Soon, age 81, who impressively learned how to paint at 65.
Toro’s planning process is long and thorough — he can only retell stories by first having a conversation with each subject, making sure that they understand what the project is about and building a relationship with them so they feel comfortable enough to authentically share.
He captures video and photos of his subjects using Hasselblad and Sony camera gear. Then, he uses Adobe apps, accelerated by GeForce RTX and NVIDIA RTX technology, in post-production.
Toro deployed the Enhance Speech tool to boost the clarity and quality of voice recordings and adjusted enhancement levels with the Mix Amount setting — all powered by AI. The feature is 75% faster on a GeForce RTX 4090 laptop GPU compared with an RTX 3080 Ti.
“Without AI, the footage, filmed in challenging, noisy conditions, would be unusable,” he said.
The Text-Based Editing tool in Premiere Pro allowed Toro to use speech-to-text AI capabilities to automatically create captions, supported in 18 languages, for video footage — speeding the editing process.
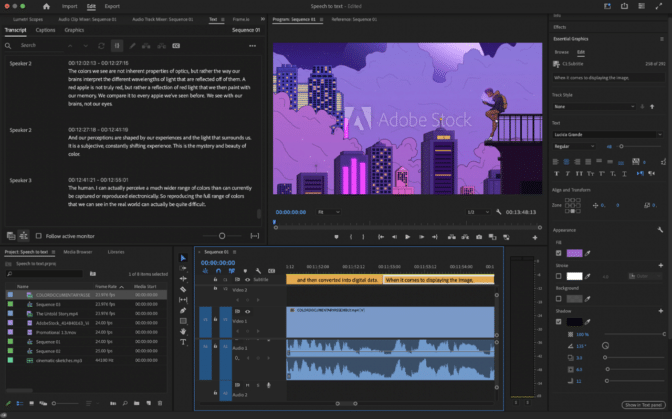
Toro also used the Filler Word Detection feature, which detects and deletes filler words and pauses, to achieve cleaner, more accurate transcripts. Filler words are language agnostic, so the feature works in all 18 languages supported in Text-Based Editing.
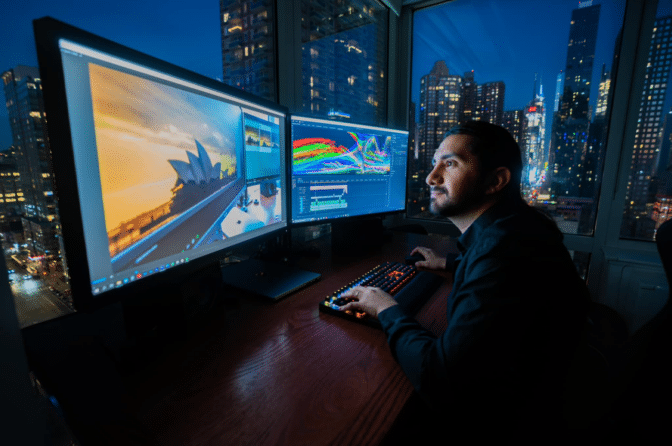
Adobe offers a wide variety of time-saving features, such as the AI-powered Auto Reframe tool for automated editing in multiple size formats for social media with project templates. Toro’s NVIDIA Studio laptop with the GeForce RTX 4070 graphics card accelerates all of these powerful tools.
Final file exports were achieved 4x faster than with a CPU alone thanks to the GPU-accelerated NVIDIA video encoder (NVENC). Toro quickly and easily added finishing touches in Photoshop Lightroom, using the RTX-accelerated, AI-powered Raw Details feature to refine the color detail of his high-resolution RAW images, and the Super Resolution feature to upscale images with higher quality than traditional methods.
“Having a dedicated GPU for video projects when filming high-quality video is almost mandatory,” said Toro. “Using NVIDIA GPUs allows me to render and process my projects faster, so the post-processing tools are serving my creative ideas, and I’m not limited by what the computer can do, but exactly what I want to create.”
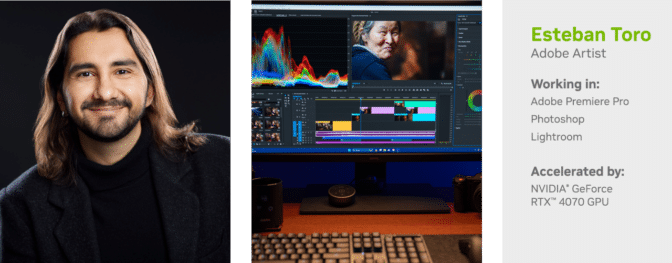
Follow Esteban Toro on Instagram.
NVIDIA Eos Revealed: Peek Into Operations of a Top 10 Supercomputer
Providing a peek at the architecture powering advanced AI factories, NVIDIA Thursday released a video that offers the first public look at Eos, its latest data-center-scale supercomputer.
An extremely large-scale NVIDIA DGX SuperPOD, Eos is where NVIDIA developers create their AI breakthroughs using accelerated computing infrastructure and fully optimized software.
Eos is built with 576 NVIDIA DGX H100 systems, NVIDIA Quantum-2 InfiniBand networking and software, providing a total of 18.4 exaflops of FP8 AI performance.
Revealed in November at the Supercomputing 2023 trade show, Eos — named for the Greek goddess said to open the gates of dawn each day — reflects NVIDIA’s commitment to advancing AI technology.
Eos Supercomputer Fuels Innovation
Each DGX H100 system is equipped with eight NVIDIA H100 Tensor Core GPUs. Eos features a total of 4,608 H100 GPUs.
As a result, Eos can handle the largest AI workloads to train large language models, recommender systems, quantum simulations and more.
It’s a showcase of what NVIDIA’s technologies can do, when working at scale.
Eos is arriving at the perfect time. People are changing the world with generative AI, from drug discovery to chatbots to autonomous machines and beyond.
To achieve these breakthroughs, they need more than AI expertise and development skills. They need an AI factory — a purpose-built AI engine that’s always available and can help ramp their capacity to build AI models at scale
Eos delivers. Ranked No. 9 in the TOP500 list of the world’s fastest supercomputers, Eos pushes the boundaries of AI technology and infrastructure.
It includes NVIDIA’s advanced accelerated computing and networking alongside sophisticated software offerings such as NVIDIA Base Command and NVIDIA AI Enterprise.
Eos’s architecture is optimized for AI workloads demanding ultra-low-latency and high-throughput interconnectivity across a large cluster of accelerated computing nodes, making it an ideal solution for enterprises looking to scale their AI capabilities.
Based on NVIDIA Quantum-2 InfiniBand with In-Network Computing technology, its network architecture supports data transfer speeds of up to 400Gb/s, facilitating the rapid movement of large datasets essential for training complex AI models.
At the heart of Eos lies the groundbreaking DGX SuperPOD architecture powered by NVIDIA’s DGX H100 systems.
The architecture is built to provide the AI and computing fields with tightly integrated full-stack systems capable of computing at an enormous scale.
As enterprises and developers worldwide seek to harness the power of AI, Eos stands as a pivotal resource, promising to accelerate the journey towards AI-infused applications that fuel every organization.
The Easiest Upgrade: Play at Ultimate Quality With GeForce NOW
GFN Thursday keeps its fourth anniversary celebrations rolling by bringing Ubisoft’s Skull and Bones and Microsoft’s Halo Infinite to the cloud this week.
They’re part of five newly supported games, and thanks to the power of the cloud, members can play them at unrivaled quality across nearly any device.
The Ultimate Upgrade, Instantly
When GeForce NOW launched in 2020, members flocked to take advantage of NVIDIA GeForce RTX 20 Series GPU-powered servers and experience real-time ray tracing on low-powered devices. For the first time, high-performance PC gaming was available to all.
Later, members gained access to the Ultimate upgrade, as NVIDIA cloud gaming servers brought GeForce RTX 3080-class power to users across the globe.
Now, with the NVIDIA Ada Lovelace GPU architecture, cloud gaming has taken another leap forward, powered by the GeForce RTX 4080 SuperPOD.
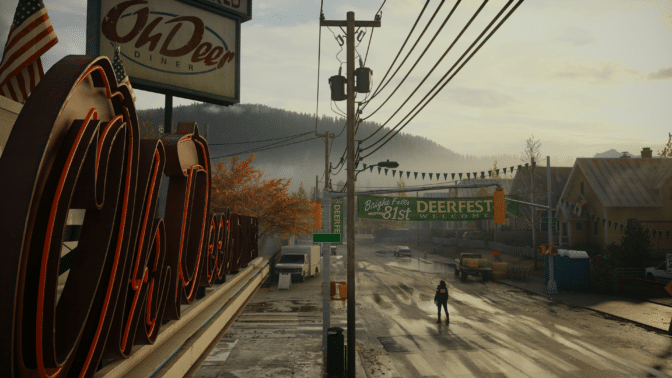
That means nearly anyone can experience groundbreaking PC gaming technologies like NVIDIA DLSS 3.5, with its AI-powered Frame Generation and upscaling features. Members can explore their favorite game worlds rendered with cinematic lighting and reflections thanks to RTX ON, with full ray tracing supported in titles like Cyberpunk 2077 and Alan Wake 2. Experience immersive gaming, even on old laptops or smartphones.
Enjoy the greatest PC games available at up to 4K resolution with an Ultimate membership, and explore a whole new world with support for 21:9 ultrawide resolutions.
Members also have the competitive edge in the cloud, thanks to support for NVIDIA Reflex technology. Ultimate members can take aim and make every shot count with ultra-low latency and support for up to 240 frames per second performance — a first for cloud gaming — all made possible by GeForce NOW. Upgrade today to feel the difference.
Shiver Me Timbers
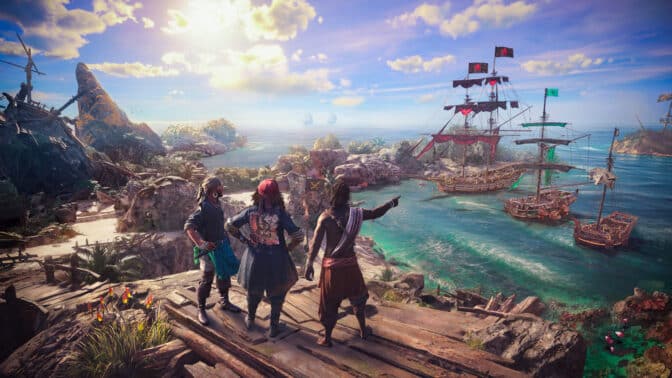
Enter the perilous world of Skull and Bones, Ubisoft’s nautical action-packed adventure streaming now on GeForce NOW.
Sail the seas as a fearsome pirate kingpin, gaining infamy and gathering resources while building a smuggling empire. Engage in thrilling naval battles and risk it all for the biggest loot. Equip powerful weapons to outgun other ships and rain terror on enemy forts. Craft and sail up to 10 ships, each with unique perks, and become a force of destruction on the water.
Upgrade to a GeForce NOW Ultimate membership to loot and plunder at full quality, with support for ultrawide resolutions and gameplay at up to 4K resolution and 120 fps on PCs and Macs.
Infinite Action
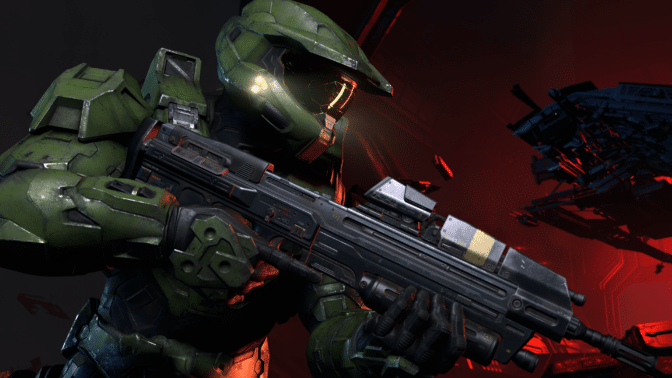
Step inside the armor of humanity’s greatest hero. Halo Infinite joins GeForce NOW this week, delivering the most expansive Master Chief campaign yet and a groundbreaking, free-to-play multiplayer experience. Plus, read this article and search for Halo Infinite for more details on how to launch the game.
It’s part of five new games this week:
- Banishers: Ghosts of New Eden (New release on Steam, Feb. 12)
- Deep Rock Galactic: Survivor (New release on Steam, Feb. 14)
- Goat Simulator 3 (New release on Steam, Feb 15)
- Skull and Bones (New release on Ubisoft, Feb. 16)
- Halo Infinite (Steam and Xbox, available on PC Game Pass)
What are you planning to play this weekend? Let us know on X or in the comments below.
Happy Valentine’s Day!
Raise your hand if you’re in a cirrus relationship.
pic.twitter.com/g9bVP4BmPX
—
NVIDIA GeForce NOW (@NVIDIAGFN) February 14, 2024
What’s Your Story: Nicole Forsgren

In the Microsoft Research Podcast series What’s Your Story, Johannes Gehrke explores the who behind the technical and scientific advancements helping to reshape the world. A systems expert whose 10 years with Microsoft spans research and product, Gehrke talks to members of the company’s research community about what motivates their work and how they got where they are today.
Partner Research Manager and leading developer experience expert Nicole Forsgren oversees Microsoft Research efforts to enhance software engineering effectiveness through the study of developer productivity, community, and well-being. In this episode, she discusses AI’s potential impact on software engineering, what she loves about tech, and how thoughtful decision making—combined with listening to her gut—has led to opportunities as a developer, accounting professor, and founder and CEO of a startup that was eventually acquired by Google.
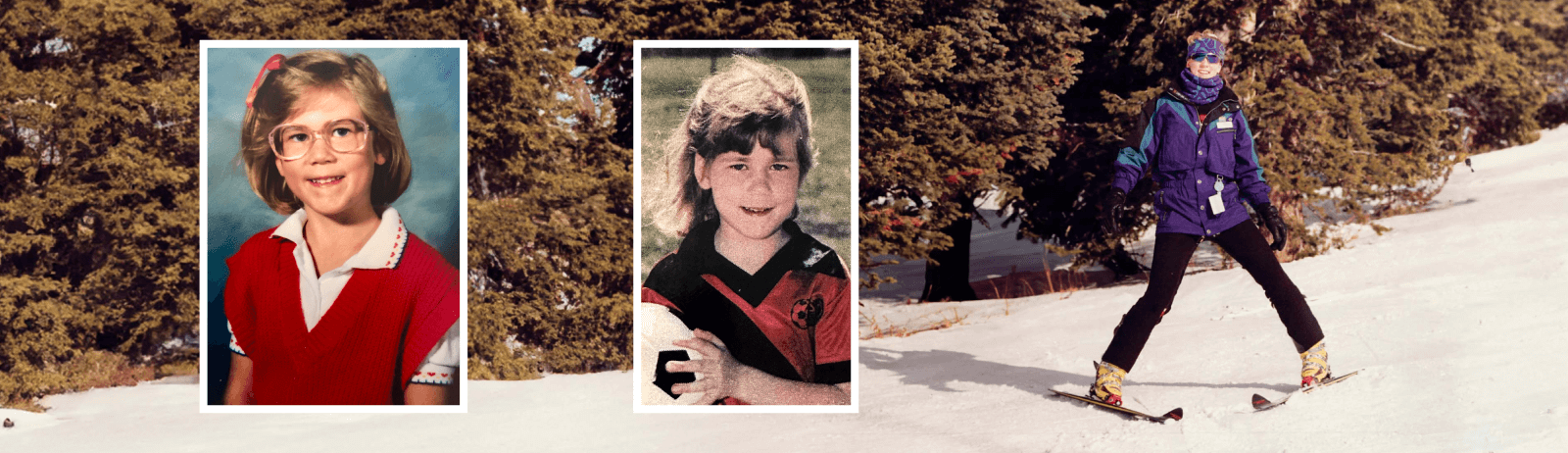
Learn more:
- Nicole Forsgren at Microsoft Research
- (opens in new tab)Nicole Forsgren website (opens in new tab)
- (opens in new tab)Quantifying the impact of developer experience (opens in new tab)
Microsoft Azure Blog, January 2024 - Yes, good DevEx increases productivity. Here is the data. (opens in new tab)
GitHub blog, January 2024 - Accelerate: The Science of Lean Software and DevOps: Building and Scaling High Performing Technology Organizations (opens in new tab)
Book, 2018
Subscribe to the Microsoft Research Podcast:
Transcript
[TEASER] [MUSIC PLAYS UNDER DIALOGUE]NICOLE FORSGREN: Assume that something can be figured out and that it’s not hard. I didn’t find out until the end of college that computers were hard. And so if it’s hard, that’s OK. It might mean that you should just spin it on its head and try to take another look.
[TEASER ENDS]JOHANNES GEHRKE: Microsoft Research works at the cutting edge. But how much do we know about the people behind the science and technology that we create? This is What’s Your Story, and I’m Johannes Gehrke. In my 10 years with Microsoft, across product and research, I’ve been continuously excited and inspired by the people I work with, and I’m curious about how they became the talented and passionate people they are today. So I sat down with some of them. Now, I’m sharing their stories with you. In this podcast series, you’ll hear from them about how they grew up, the critical choices that shaped their lives, and their advice to others looking to carve a similar path.
[MUSIC FADES]In this episode, I’m talking with Partner Research Manager Nicole Forsgren. A leading expert in the field of DevOps and the lead of Developer Experience Lab, Nicole oversees Microsoft Research efforts to better understand and enhance the developer experience through the study of their productivity, community, and well-being. Prior to joining Microsoft, Nicole was a successful software engineer at IBM, a college professor, and a co-founder and CEO of a startup that was later acquired by Google. Here’s my conversation with Nicole, beginning with her childhood in Idaho.
GEHRKE: We’ve talked a little bit beforehand, but you’ve had this amazing career in tech. How did you actually … tell us a bit about how you grew up, and how did you end up in tech?
NICOLE FORSGREN: Yeah, it’s, you know, it’s, kind of, this ridiculous story. I grew up in a little farm town and ended up going to college and thought I would just be there a year or two because …
GEHRKE: Little farm town in?
FORSGREN: In Idaho.
GEHRKE: In Idaho?
FORSGREN: Yeah. So across the street from a potato field. My grandpa was a potato farmer. And where I’m from, girls—a lot of girls—go to college but not usually for very long. You kind of go to school to get married. And so I was majoring in psych and family science.
GEHRKE: So in high school, you didn’t know anything about or you weren’t excited about tech yet or anything?
FORSGREN: I don’t think I knew much about it, right.
GEHRKE: OK.
FORSGREN: So, I mean, we had a computer at home. My dad was a civil engineer. But I had only ever used it to write papers. I knew WordPerfect because back then WordPerfect was, kind of, the thing.
GEHRKE: Yeah, right. So it was a PC?
FORSGREN: It was a PC. It had, you know, reveal codes, which …
GEHRKE: It was DOS, just not Windows yet, right, or was it Windows?
FORSGREN: I think it was DOS back then. Yeah. And that was …
GEHRKE: And so connector interfaces and everything?
FORSGREN: Yeah,we had all the interfaces. And we had a typing class in high school, but that, that was it. And then I went on to college, and a couple of months into my freshman year, my dad, who I was really close with … so I grew up as a tomboy. I was always mountain biking up in the mountains. I was always just, like, dirty and, kind of, just playing around. I was very much a tomboy. Two days after Thanksgiving, he was in a snowmobile accident and in a coma, and suddenly, I sat back and I realized that, you know, all the things that I thought I was going to be able to rely on, like, you know, if I ever needed to fall back on my family or my dad or pay for things, for some reason, like, that was what stood out to me for some reason. You know, I was in college on a volleyball scholarship, and, like, I was paying for things and it was OK. But for some reason, that was what stood out at me—because I was the oldest of four children. The youngest was 11 years younger than me. So I was like, if anything happens, I think I just felt that responsibility, was that I was going to need to have money. And one of the girls on the practice team, I think in just a totally random comment, said that she could make money in her degree.
GEHRKE: Because you hadn’t chosen that as the …
FORSGREN: Yeah, it just, sort of, came … ooh, because when we went to college, it was, I was going to get married and so then I wouldn’t need money, right. Because I didn’t choose a degree for that. I want to say it was within a couple of days of my dad’s accident—so he was still in a coma; he was still hurt—and it was a very side comment she had made. And I remember hearing it, kind of, in the back, and I, kind of, perked up, and I was like, “What do you mean you can make money with your degree?” She said, “Oh, yeah, when I graduate”—with a two-year degree—”I can make $40,000 a year.”
GEHRKE: So she wasn’t at … was she at your university, or she was at a different …?
FORSGREN: So I went to a JC first because we don’t really go to college. And I was like, oh, $40,000. And I remember thinking, well, if I stay in psych and family science, to be able to make that kind of money, I would need to be a high school teacher, which requires a master’s degree. And so I talked to her just a little bit, and I said, “Well, what’s your degree?” She said computer information systems. I said, “Well, do you like it?” She said, “Yeah, it’s really cool. It’s computers.” I said, “Oh, and what can you make again?” She said about $40,000. I was like, “Cool.” And I just remember looking up that degree and going into finding, you know, I just found the counselors in that degree and telling them I wanted to change my major.
GEHRKE: Oh, wow, that’s a huge decision. I mean, first of all, did your father recover?
FORSGREN: So he ended up coming out of his coma within a couple of months, but he was brain damaged for the next few years. And he passed just after I graduated from college.
GEHRKE: I’m sorry to hear that. But then … so you went ahead and changed your major and went into …?
FORSGREN: I changed my major—without ever having a computer class …
GEHRKE: Right.
FORSGREN: … to CIS, which is, now I know, computers and business. That summer, I applied for an internship and got it. [LAUGHS]
GEHRKE: After your freshman year?
FORSGREN: After my freshman year.
GEHRKE: Wow. What kind of internship? Where was it?
FORSGREN: It was in mainframes for computer hospital systems. And so I showed up, and they thought I was full time, which I was not. I just interviewed well apparently. And so they threw me the manual and the documentation.
GEHRKE: What kind of mainframe was it? Do you remember what kind of machine it was?
FORSGREN: Yeah, it was AS/400s, and it was programing RPG and CLI, and it was for …
GEHRKE: Beautiful languages. [LAUGHS]
FORSGREN: They were. I loved it. And it was Siemens, um—SMS MedSeries 4 was the company, later acquired by Siemens. And it was a wonderful team, and I spent the first week reading the documentation and proofreading the documentation [LAUGHS], and then I just, kind of, dove in.
GEHRKE: So proofreading? You mean you discovered a bunch of bugs?
FORSGREN: There were a lot of typos, a couple of bugs, right. Because I just, kind of, had to figure it out as I went.
GEHRKE: Wow. So it’s not like somebody did preparing with you at the beginning? You were just handed the manual, and then you went with it?
FORSGREN: I was just handed the manual, and then I went with it. I had to …
GEHRKE: Wow, it’s an amazing self-starter.
FORSGREN: … figure out one or two things, and then I just dove in, and then I went back that next year and had my first RPG class. [LAUGHS]
GEHRKE: Wow. They were teaching RPG in college?
FORSGREN: Yeah. So this was … my freshman year was ’97-’98, and then my sophomore year was ’98-’99, so it was a JC, and they were, kind of, gearing up one or two classes in anticipation of the Y2K bug.
GEHRKE: Right.
FORSGREN: And so mainframes …
GEHRKE: Oh, I remember that. Yes …
FORSGREN: …and financial and hospital systems …
GEHRKE: Everybody thought the world was ending or at least some people were thinking the world would end.
FORSGREN: They were so worried about it. And so they had brought back one or two mainframe languages to help with the Y2K crisis. And so that summer, I did hospital systems. The next summer, I did, um, it was a company called Assist Cayenta, and it was, like, it was financial systems and ordering systems. That’s how I started my career, in, like, it was a version of mainframes.
GEHRKE: And then did you get that high-paying job afterwards?
FORSGREN: Yeah. So I had a full-time offer, but by then, I decided to go for my four-year degree. Transferred to Utah State, which was within a few hours from home. I still had to, kind of, stay closer to home because the family, and I wanted to, you know, make sure I was close for my dad. But then I did a six-month internship at IBM. I was a software engineer, and then they hired me full time when, again, I was a software engineer, so …
GEHRKE: But you basically finished the two-year college, then went to Utah State. Finished a four-year degree.
FORSGREN: Finished a four-year degree. Again, there I was … they called it business information systems—now it’s management information systems—at Utah State. It was a very technical degree, so I was doing network engineering. I was doing databases. I was doing, you know, C++. All my programing classes were all in the computer science department. When I got hired at IBM, they hired me as a software engineer. I was working on large-scale enterprise storage systems.
GEHRKE: Which language did you program in then?
FORSGREN: There, I was doing some C++. I was doing a little bit of C. I was doing some Java, and I was doing a little bit of their firmware.
GEHRKE: Wow.
FORSGREN: And then I eventually ended up managing some of their systems, so I did some Bash, and then eventually, I even got a hardware patent, so I—it was kind of everything!
GEHRKE: Wow. So what is the patent about?
FORSGREN: It’s a way to, kind of, further obfuscate for cold boot attacks. This really, kind of, fantastic article came out showing that if you take compressed air and turn the can upside down, you can freeze some of the bits in chips.
GEHRKE: Right.
FORSGREN: And then if you rip the chip out, you can read what’s on the chip.
GEHRKE: Oh, wow.
FORSGREN: Because it freezes all the elect—
GEHRKE: Super interesting.
FORSGREN: … all the electrons on the chip. And we were like, well, this is ridiculous because it’s only frozen for 2 to 5 seconds. But then if you rip it out and you drop it in, like, liquid nitrogen, then you can read it for 2 to 5 minutes.
GEHRKE: Right.
FORSGREN: Like, well, again, this is ridiculous. But if you’ve entered in a password, then it’s stored in plaintext. Well, it’s not ridiculous if you get your way into a lab—and at the time, I was working in a large lab—because you’ve entered in the password for one computer, one of the servers, one of the stored servers, and you’ve destroyed the, you know, you’ve broken the disk for that one server, but the password is going to be the same for every single server in the lab.
GEHRKE: OK.
FORSGREN: And so we realized that this is a serious, you know, problem that’s a threat vector for a lab. And it’s pretty easy to get into labs, right. Like, you can just, you can follow anybody in. And so we wrote a patent that kind of further obfuscates and it hides where passwords are stored through malloc() calls.
GEHRKE: I see, so … I see. So the location of the password was then somewhat obfuscated and not so easy to find.
FORSGREN: Well, so the location of passwords but also additional plaintext strings and other strings are obfuscated through, kind of, throughout the pieces of, like, different areas of chips.
GEHRKE: This was motivated by the hardware but then implemented in software?
FORSGREN: Yeah.
GEHRKE: OK, wow, that’s super interesting.
FORSGREN: So part of hardware and part of software.
GEHRKE: I mean, just imagine this career, right, in … I guess in high school you’re playing volleyball?
FORSGREN: In high school and college, I was playing volleyball. Ended up getting hurt, so I didn’t play volleyball longer.
GEHRKE: OK. But then switched to, you know …
FORSGREN: Switched to tech.
GEHRKE: MIS, and …
FORSGREN: Yeah, switched to MIS.
GEHRKE: Patent.
FORSGREN: Patent—and shoutout to, you know, my coauthors on the patent, Ben Donie, Andreas Koster. They were great because we all just, kind of, ended up brainstorming one day. And it was this, kind of, windy path, but it was, I think, it was interesting because at the time, you know, I originally went into tech because I thought I would really need money but ended up falling in love with it, right. I’ve had a lot of fun along the way.
GEHRKE: So what did you fall in love with with tech? What really gets you going on tech?
FORSGREN: I love the fact that there are hard, interesting problems that you can solve lots of different ways. And if I can’t solve it initially and if I can’t solve it the first time, I can just kind of spin it around or pivot it in different ways and then just solve it again.
GEHRKE: So it’s this notion that you have this not only hard problems—because in math, you have lots of hard problems, as well—but here there’s the experimentation with it and the trial and error or …?
FORSGREN: The experimentation and the fact that it’s applied and the fact that I can build something and watch it work. Math I liked. And math, up to a point, I was pretty good at math, and I could kind of see how the equations were supposed to work. Computers and programs helped because I could really see how it was working.
GEHRKE: Yeah, I think that, I mean, I can so much relate to this because that’s how I fell in love with computing, because you have this machine here and it basically can do everything, right. And I mean, we will get later to AI. What we see with the current AI systems, right, you can see that, you know, it can be nearly intelligent or it is intelligent, right. And so it’s just amazing to have that kind of machine below you or with you to help you and to be able to train it and program it. And so, you were at IBM …
FORSGREN: So I was at IBM.
GEHRKE: … and now you’re at MSR (Microsoft Research).
FORSGREN: And now I’m at MSR.
GEHRKE: So what’s the bridge in between?
FORSGREN: Oh, so also, kind of, a windy path, but it’s interesting because as I look back, I guess it makes sense to me. So then I had an opportunity to go get a PhD, and I actually started doing, kind of, large data, like, NL, you know, natural language. You know, how do we want to think about, you know, analyzing sentiment analysis, analyzing, you know, those types of, you know, big questions like when are people lying, when are people not lying, machine learning problems. But I was also working at IBM at the time. Because I, like, continued to like, you know, working on systems and working on large problems. So I was doing both at the same time, and I ended up doing usability study … completely randomly. And …
GEHRKE: What was the study on? What did you study?
FORSGREN: So we did a usability study for sysadmins. And it was interesting because at the time, IBM was trying to build, like, a GUI for large-scale sysadmins. And so Todd Eischeid and I did the usability study. We wrote up the findings for IBM; we shared them. And, you know, we found that in some cases, they would use this frontend user interface and it was fine. And in some cases, they were like, “I don’t know,” right, “like I could click this button, but it really makes me nervous.” And we’re like, “It’s OK. It’s a sandbox. This is fake. You can click the button. I’m curious to see what the next step is.” And they were like, “It’s just so risky.” And it struck me as being, sort of, interesting because, like, there were just cases where risk and complexity really interfered with our ability to trust a system or to trust a GUI. And so we wrote this up and we shared it, and IBM was like, eh, you know, we used user-centered guidelines; we used, you know, user-centered design guidelines. We were like, but the same guidelines can’t work for complex systems and complex distributed systems that can work for laptops. And …
GEHRKE: Because in one way, I affect this one machine here, but in the other way, I affect this row of machines, and I know how many this actually is.
FORSGREN: Right. And they really wanted to see the command line interface and the backend data, and they really wanted to verify. And so you really had this difference between not just risk and complexity but also expertise. You know, there were some cases where you could hide complexity and other cases where it just wasn’t appropriate, right. And, you know, simultaneously, I’m working on these really, really large projects with IBM, and people are just, kind of, burning out. And I thought, you know, there has to be something to this, right. And there were kind of rumors as I was going to tech conferences still of, you know, this new-fangled way to make software and, kind of, reduce burden. And so I just pivoted. I changed my research project to start studying what now we know of as DevOps.
GEHRKE: Maybe we will get to this in a little bit, but I’m so impressed by, you know, you go to college, do A. Something happens. You do B, and don’t look back. Same thing here, right? You’re a successful developer at IBM. You have this event which says, wow, I should study this intensively, and you go and get a PhD, right. I mean, it’s just super impressive. How do you do that?
FORSGREN: It’s, I will say, it’s not always super straightforward, [LAUGHTER] but there are times when I, kind of, sit and I’m like, I’m getting one or two signals. I really want to take a half-step back and say, here’s an opportunity. Should I take the opportunity, or should I not? There have been one or two times where I’m not sure, you know, but there have been times where I’m like, I need to jump, and I can reevaluate in six months or a year, but I’m going to take this now. And I will say about 90 percent of the time I have been absolutely on.
GEHRKE: I find this so fascinating because we all are faced with different opportunities and chances in our lives. How do you evaluate this? Do you have, like, a checklist? Do you go back and ruminate and meditate on the mountain? Or what’s your method?
FORSGREN: I actually have a spreadsheet. [LAUGHS]
GEHRKE: OK.
FORSGREN: But I don’t always follow it. So what I like to do is identify, like, what are my criteria, which is, you know, what things are important to me.
GEHRKE: Yup.
FORSGREN: You know, some of my big moves—what’s important to me in the city? And then for each of those criteria … or when I am considering a new job—what things are important, and then how important are they?
GEHRKE: And you put, like, a risk score behind it or, like, a score?
FORSGREN: Either a risk score or an importance score. And then I’ll just, kind of, multiply it out. And, now, then I will go back and I’ll like …
GEHRKE: That sounds so amazingly systematic.
FORSGREN: … and then I’ll nudge all the numbers, and then sometimes, I’ll still change my mind because there’s something about your gut that says, I don’t know. But by identifying all those things first, it helps me think through it. Now, the spreadsheet, like, I’ve shared with folks, and it’s really interesting because just the exercise of saying what things are important to me for this decision and how important are they sometimes … not even doing like the math, right. Because it’s not real math. Let’s be real. Or it’s, like, very simplified math. Just identifying those things, sometimes just that exercise, people are like, “Oh, I know what my decision is now.”
GEHRKE: I think often just even thinking about this with that clarity, right, creates the resulting clarity then and think about all the factors.
FORSGREN: Right. And there have been times where I’ve completely changed what I thought I would do and, like, I can give an example. After I left academia, I was at a startup, and then following that, I started, like, my cute little baby startup company. I thought for sure I was going to go to a large consulting firm. I mean, I had them ranked. I thought I knew my choices, and I was like, no, let’s, let me think. Let me identify what order I should go in. And, like, it didn’t matter how I, kind of, rearranged all the numbers, starting my own company was at the top.
GEHRKE: Well, let’s go to that in your career.
FORSGREN: Yeah, so let’s, let’s catch up.
GEHRKE: Exactly, so you decide to go do your PhD?
FORSGREN: So I finished the PhD. I stay in academia for a while because I really like the research that I’m doing. It’s really interesting. I think I’m, kind of, on to something, and academia is a good place to do this, right. So I was a professor at Pepperdine for a few years. I go to Utah State for a few years. Again, like, what’s this opportunity? Pepperdine was a lovely place. The faculty were incredible. Malibu is gorgeous. Utah State, my alma mater, comes along, and they’re like, we would like to hire you, and we’ll create an opportunity for a new position. So I took that pivot. And now it’s a joint appointment to create an analytics program in the MIS department. I was also an accounting professor, because I have a master’s in accounting, so it was, kind of, this, like, perfect situation. I was there for, you know, two, three years, and I’m doing really, really well. A really strong path to tenure. Letter from the provost saying that things are looking really well. And I’m like, this is good, but it’s not great.
GEHRKE: You had a spreadsheet that said that basically or [LAUGHS] …
FORSGREN: This was in my gut.
GEHRKE: This is just “I had a feeling.”
FORSGREN: In my gut, I’m like, I’m doing really well. My research is going really well. We just hit the Wall Street Journal because we find early signal that DevOps—like now it’s being called DevOps—shows organizational impact. And so a few folks in industry were coming to me and they said, you know, “This is super relevant. You’re really changing how we’re doing things.” This was still, kind of, earlyish in this research program, and they said, “What if we create an opportunity for you where you could spend half of your time doing research and half of your time helping our company improve our engineering practices,” and I was like, I think I might do this. You know, at that point, I just decided to go for it, and it was a little company here in Seattle called Chef Software.
GEHRKE: It was actually here in Seattle?
FORSGREN: I was here in Seattle.
GEHRKE: Chef Software?
FORSGREN: Yeah,they did configuration management software. And also, that was a really interesting tie because—or, kind of, like pull, tug and pull because I was doing this research with their competitor called Puppet, and I went to Chef, and so I’m, kind of, like, managing these relationships really well or as well as I could. But that was also one of my first exposures to managing conflict at work and in professional relationships because this report, it was a research report, but it was done, kind of, through industry. So the main sponsor was Puppet, but I was working at Chef. And so how do I manage that? So I was at Chef for a year and a half, and then at the end of that year and a half, we, kind of, looked at each other and we were like, I think we’ve reached the end of this road because I had done about as much as I could do for this little startup. They had about 200, 250 people. They really didn’t need a researcher. They were doing me a solid. And then at the same time, we had, kind of, spun out a separate entity called DORA—DevOps Research and Assessment—and we said, so what should happen here? We had been doing research, the State of DevOps Report, but then so many companies were coming to us and they were saying, what if I want to have our own customized assessment? How could we do this? And, you know, I looked at a couple of my co-founders, you know, Jez Humble and Gene Kim, and I said, I know how to do this. I know the algorithm I would use. I know how I would build this out. I think we could do it, a SaaS company. I have a very low risk tolerance. I have never wanted to start a company. And I think, you know, to your prior question, like, how have I thought about doing this before? I actually looked at them, and I said, you know, who wants to be CEO? And they said, we think you should. And I said, eh, I’ll give you one year, and then I want you to tell me if you want me to continue doing this. And so we started this company. I mean, our first prototype, I drew pen and paper in the back of a notebook, and I showed it to Capital One, and we said, would you buy it if it looked like this? And then we just, kind of, iteratively built out pieces and pieces. And I was like copy-pasting pieces of it into reports as we went. And then at our offsite after one year, we read our results. I shared ARR and sales projections, and I said, OK, well, you have a first right of refusal, and do you want to renew? And they looked at me like what? And I’m like, no, it’s been 12 months. Do you want me to return again? And we just, kind of, decided if we wanted to. After that, things were going really well, but we were also growing and scaling really, really rapidly. Too fast for us to keep up as a bootstrapped company. And so, you know, I needed to figure out how to gain and acquire infrastructure. And so that would either have been external funding and hiring really rapidly or getting acquired, and so we … I approached a handful of companies, and Google acquired us.
GEHRKE: Wow. It’s an amazing story and must have also been a time of craziness and also fear of uncertainty. How, you know, what were some of your emotions during that time?
FORSGREN: It was. It was a lot. It was really interesting because it was, kind of, balancing also a few things, right. Because I had … I think some of it is also balancing identity, right. Am I a researcher, and how do I think about maintaining that identity and that credibility that I care so much about when the research and “publication path”—you know, kind of, in finger quotes—that I care so much about has shifted, right. Because now I’m doing a lot of applied research, and how do I think about that? Some of it was, am I an entrepreneur, and what does that look like, and what does, what does that credibility look like? How do I put out a product fast enough? How do I manage and maintain this company? How do I manage and maintain relationships with my employees, with my partners, you know, with this partner ecosystem that we’ve developed? And then when we get acquired by Google, what does that look like? How do you manage that growth path?
GEHRKE: And these are all very, very different skills than you had as a software developer or even as a professor.
FORSGREN: Especially as a professor. Right. And it was also a really, really wonderful time, I think, to grow and learn and iterate. And I’m really grateful for the partners that I had along the way, right. There were times that were bumpy, right. But I appreciate that we were really honest with each other, right. There were times when we disagreed, and there were times when I also said, like, I know this is the right thing. Please, you have to let me do this. And there were times when, you know, they had their expertise.
GEHRKE: And then, you know, you were at the company. The company gets sold to Google.
FORSGREN: Yep, company gets sold to Google.
GEHRKE: Now you’re at MSR.
FORSGREN: And then I was at Google for a little bit. And then I had this amazing opportunity to join GitHub. And I was really excited about that because that framing in that invitation, we were talking about, you know, what could it look like? You know, what would a perfect world be where I could return to something that looked like research and strategy? Because I realized there were pieces about research that I really, really loved. And there were also pieces about, I don’t want to say products—it’s not quite product—but it’s about strategy. What is it that I love doing in terms of, kind of, execution and identifying holes, and how does that feed back into the research projects that I want to do? And so when Microsoft first approached me, they said, you know, what would your ideal job look like? And I, kind of, laid that out, and they said, you know, well, let’s think this through. And so we started with GitHub because, you know, if you want to study developers, that’s an amazing place to start. So we did a couple of iterations with the Octoverse report that were really rewarding and then we said, you know, another good place, you know, another wonderful opportunity would be to think about developers and a Developer Experience Lab. And if there’s a research lab, where does that live? And we talked about it some more. You and I did, too.
GEHRKE: That’s how we started talking … exactly.
FORSGREN: Yeah, and we thought, you know, MSR really is the perfect place for that to live.
GEHRKE: And you’ve been at MSR now for a few years, and now we’re going through yet another change. I mean, you’ve been through many of these changes before. We talk about this current change. It seems like, again, coming back to this, you’ve been amazing of, like, when the environment shifts, finding out where to go. You know, you have your spreadsheets, you know, as one mechanism. Now we’re at another sea change with AI, right. And AI is clearly changing the way we write code, which is, sort of, the innermost loop right now with GitHub, with Copilot, but it’s probably going to make it much more into the inner and outer loop and the whole way we write code and the way we develop with low code. So, I mean, first of all, how do you think about that sea change, and how do you deal with your research group and, you know, yourself as an identity, again, as the world around you is having this massive change towards AI?
FORSGREN: You know, sometimes, I just laugh that the world is a circle, right. I’m really excited because, you know, we’ve come back around to getting to rethink what it means to do what we love, right. So I’m personally getting an opportunity to come back to be a developer again, right. What does it mean to dive back in and learn brand-new things again? Because AI is new for almost all of us. Even people who have been studying AI for 10 years are saying, like, so much of this is new, so much of this is something that we couldn’t have predicted. I’m getting to, you know, dive back in and play with new tools and new technologies, and that I really love. I also love that you mentioned that, you know, there’s the inner loop and there’s the outer loop. And so in terms of my team, I’m really, really excited about the team that I get to work with because …
GEHRKE: Do you want to explain maybe a little bit, just for the audience, inner and outer loop?
FORSGREN: Yeah, so inner and outer loop. So inner loop is, you know, the coding that we do, kind of, locally, so it can be writing the actual code, you know, local build, if you do local build. It can be debugging; it can be everything that’s like just right there on your screen as you’re writing your code. Now outer loop is everything that you do to get that code running in production, right. So it can be additional tests. It can be integration build. It can be, you know, everything out through release and deployment until we’re operating that code and then, like, continuing to operate that on our systems at scale.
GEHRKE: I mean, the way I saw this was so impressive when I joined Microsoft, of course, right. What it means to actually … that first of all, software development is a team sport, right.
FORSGREN: Yes.
GEHRKE: And also it’s not a team of like 11 people like soccer, where I grew up with, but it’s a team sport of, you know, potentially thousands of people, right, and that there’s an, actually, there’s a lot engineering systems around it. And it’s called software engineering for a good reason.
FORSGREN: Yeah.
GEHRKE: Because there’s systems around it that help us to scale to that many people contributing to a single outcome. And so the inner loop is, basically, my notion is that when I do my own little exercises with the ball and then the outer loop is when I actually, you know, do strategy with the whole team and see that I integrate well. Is that a reasonable analogy?
FORSGREN: Exactly. And so sometimes the joke is, you know, well it worked on my machine, right. That’s kind of inner loop. Yeah. And then outer loop is all of the orchestration, right. All of the architecture. Everything else that we need to make things, especially really large things and complex distributed systems, work at scale. Yeah.
GEHRKE: And so if you think about … how do you think AI would influence both the inner and outer loop? I mean, we see what’s coming out in terms of, you know, GitHub Copilot and even more capabilities. I mean, in the “[Sparks of Artificial General Intelligence]” paper, we describe that GPT-4 can actually write an application with close to a thousand lines of code, right. So how do you think AI’s actually going to influence developer productivity and software engineering as a whole?
FORSGREN: I think there are so many exciting ways to think through this, right. I love your point that there’s inner and there’s outer loop, right. So, yes, we absolutely have opportunities to think about how it influences the way we write code, but I think it also has so many opportunities downstream, right. How can it, how could we use it to improve our code bases, right? Can it identify technical debt and clean up some of our technical debt for us? Can it help us think about downstream incident management? Can it help us manage our servers and our systems? Can we use it to take a look at our code for us? Can we ask it, can you please help me improve the security posture of my code? Can you help me improve the performance of my code? Can you help me improve anything else in my code, right? Even that explicitly: can you say, is there anything I haven’t thought of in my code, and what should I do? We can ask it, you know, to proactively watch and monitor our systems’ performance for us and then proactively manage that for us, you know. As we look, you know, even further into the future, there may be opportunities for brand-new abstraction layers. What happens if we let LLMs, or invite LLMs, to execute for us and then reason about that code for us so that all we need to do is guide and direct it?
GEHRKE: We’ve been talking a little bit about code, but maybe in the future, code would be, sort of, this low-level abstraction like what we have right now with assembly. There are very few people who still optimize, let’s say, locks and database systems with the assembly code, but most people write at a much higher level of abstraction. So what do you see as this next level of abstractions that are coming?
FORSGREN: You know, I think …
GEHRKE: Is it language, basically, language interaction?
FORSGREN: I do think some will be language. You know, I really like that idea for at least a few things, for a couple of things I just mentioned, right. Like asking it to do things like please check for security; please improve the performance. Can you help me generate workloads? Can you help me run these type of canary tests, right?
GEHRKE: Like semantic linters with a very bigger … with much bigger capabilities.
FORSGREN: Exactly. I also think there’s an opportunity for graphical interfaces, and by that, I mean what if we create a diagram or a UML and then ask it to implement that in the best way possible. Or reverse it, right? When I think about when I was working in code bases, the best way I could get a feel of the code base was to code it, and then I could create this mental model. If we’re doing less coding, how can we create that mental model? I think there could be wonderful opportunities to ask or invite these LLMs to diagram some of these code bases for us.
GEHRKE: So interesting …
FORSGREN: You know, don’t just ask it to create documentation or explain it to us, because language can be somewhat limited, but we know that diagrams and pictures can be incredibly powerful.
GEHRKE: I see. Create the actual architecture diagram for us.
FORSGREN: Create an architecture diagram—and create it in two or three different ways to help us understand how different components interact, which can also help us understand where there may be redundancies in code. There’s a huge amount of technical debt out there, right. So I think that, kind of, opens up some really interesting ideas for what could be there. You know, there are also some wonderful horizons that we’re already approaching in terms of testing and exploratory testing and what that can mean for really improving the way our code works.
GEHRKE: It’s super exciting. I mean, I would love to be able to talk more about that with you. But let me ask one last question. I mean, you’ve had this absolutely stunning career. I mean, if you think about where you started out, you know, then developer, you know, professor, startup founder, you know, working in a big company, being in a restructuring of the company, for someone who’s starting out, what’s the career advice that you give for anybody who’s right now starting and going into tech, people who are at university or just graduating?
FORSGREN: I think there would be two, and I think they’re related. One would be assume that something can be figured out and that it’s not hard. I think that would probably be one of my best tricks. I didn’t find out until the end of college that girls were bad at math, which I am not, or that computers were hard. It really helped that for most of my life, my dad just, sort of, helped me rethink through things or repivot or retry lots of things. And so if it’s hard, that’s OK. It just might mean that you should just spin it on its head and try to take another look. So that would be the first one. And I think the second one is consider new opportunities and go ahead and take them. And if after six or nine or 12 months, it’s not the right opportunity, go ahead and change your mind. I would not be where I am today if I hadn’t taken one or two really incredible opportunities that seemed a little bananas at the time, and it just worked out.
GEHRKE: That’s amazing advice, especially also in something that tells us to A/B test even our own life. The only problem is that we have only a limited number of tests that we can do, but I mean, it clearly is an amazing story. Thank you so much for the conversation.
FORSGREN: Yeah, thank you.
GEHRKE: Thank you.
To learn more about Nicole’s work or to see photos of Nicole as a child in Idaho, visit aka.ms/ResearcherStories (opens in new tab).
The post What’s Your Story: Nicole Forsgren appeared first on Microsoft Research.
Do large language models understand the world?
In addition to its practical implications, recent work on “meaning representations” could shed light on some old philosophical questions.Read More
Video generation models as world simulators
We explore large-scale training of generative models on video data. Specifically, we train text-conditional diffusion models jointly on videos and images of variable durations, resolutions and aspect ratios. We leverage a transformer architecture that operates on spacetime patches of video and image latent codes. Our largest model, Sora, is capable of generating a minute of high fidelity video. Our results suggest that scaling video generation models is a promising path towards building general purpose simulators of the physical world.OpenAI Blog
Digitalization: A Game Changer for the Auto Industry
The fusion of the physical and digital worlds is reshaping the automotive industry. NVIDIA’s automotive partners are using digitalization to transform every phase of the product lifecycle — evolving primarily physical, manual processes into software-driven, AI-enhanced digital systems.
Watch the video to learn more.
Digitalization: A Game Changer From End to End
Kaivan Karimi, global partner strategy lead at Microsoft, observes that companies are achieving “huge” results from “digitizing the physical entity, running simulations and rendering in 3D, whether it’s factory automation or modernizing the design and development of the car.”
Brian Ullem, vice president of engineering at Capgemini, explains that, with “the 30,000 parts that go into a car, it takes approximately five years to develop a vehicle end to end. Instead of building 50 or 100 cars, we can use digitalization to simulate without having to build prototypes. That saves a lot of time and money in the process.”
Thomas Mueller, chief technology officer of engineering at Wipro, adds that with digitalization, “we are now able to run simulations at a low cost…and improve the user experience.”
Simulation: Critical for Autonomous Driving
“Simulation is crucial to the development of autonomous systems,” says Ziv Binyamini, CEO of Foretellix. “On one hand, you need the real world, but this is highly costly. So you have to complement it with the ability to simulate a virtual world where everything is possible. And then you can, in a very cost-effective way, iterate quickly and ensure the system operates under all of these conditions.”
Simulation “gives our customers the power to validate their ADAS or autonomous systems virtually — with highly accurate sensors in the camera, lidar and radar domains — without having to rely on actual physical drives,” adds Tony Karam, global sales director at Ansys.
Austin Russell, founder and CEO of Luminar, agrees that “simulation is absolutely critical for autonomous driving. It’s great to see the work that NVIDIA has been doing in that domain, with not just the hardware but also the software.”
NVIDIA Omniverse: The Digital-Physical Convergence
“Software is a new component in the value proposition,” notes Walid Negm, chief technology officer of product engineering at Deloitte. The companies that will “survive and thrive are going to have to become much more efficient using the digital-physical convergence. The Omniverse experience is going to be important for the automotive sector.”
Shiv Tasker, global vice president of engineering at Capgemini, adds that the “visualization and production of digital twins relies on an efficient, high-performance infrastructure as well as the platforms that make it easy for customers to adopt the technology.”
Omniverse “will allow your worldwide team to simultaneously collaborate,” says Karimi of Microsoft. “Design engineers, migration engineers, test engineers — everybody collaborates simultaneously. That’s the power of NVIDIA Omniverse.”
Learn more about the NVIDIA DRIVE platform and how it’s helping industry leaders redefine transportation.
Join NVIDIA at GTC from March 18-21 in San Jose, Calif., to learn more about digitalization in the automotive industry.
How we’re partnering with the industry, governments and civil society to advance AI
Google shares more on how it’s partnering with industry, governments and civil society to advance AI.Read More