With the 2018 launch of RTX technologies and the first consumer GPU built for AI — GeForce RTX — NVIDIA accelerated the shift to AI computing. Since then, AI on RTX PCs and workstations has grown into a thriving ecosystem with more than 100 million users and 500 AI applications.
Generative AI is now ushering in a new wave of capabilities from PC to cloud. And NVIDIA’s rich history and expertise in AI is helping ensure all users have the performance to handle a wide range of AI features.
Users at home and in the office are already taking advantage of AI on RTX with productivity- and entertainment-enhancing software. Gamers feel the benefits of AI on GeForce RTX GPUs with higher frame rates at stunning resolutions in their favorite titles. Creators can focus on creativity, instead of watching spinning wheels or repeating mundane tasks. And developers can streamline workflows using generative AI for prototyping and to automate debugging.
The field of AI is moving fast. As research advances, AI will tackle more complex tasks. And the demanding performance needs will be handled by RTX.
What Is AI?
In its most fundamental form, artificial intelligence is a smarter type of computing. It’s the capability of a computer program or a machine to think, learn and take actions without being explicitly coded with commands to do so, or a user having to control each command.
AI can be thought of as the ability for a device to perform tasks autonomously, by ingesting and analyzing enormous amounts of data, then recognizing patterns in that data — often referred to as being “trained.”
AI development is always oriented around developing systems that perform tasks that would otherwise require human intelligence, and often significant levels of input, to complete — only at speeds beyond any individual’s or group’s capabilities. For this reason, AI is broadly seen as both disruptive and highly transformational.
A key benefit of AI systems is the ability to learn from experiences or patterns inside data, adjusting conclusions on their own when fed new inputs or data. This self-learning allows AI systems to accomplish a stunning variety of tasks, including image recognition, speech recognition, language translation, medical diagnostics, car navigation, image and video enhancement, and hundreds of other use cases.
The next step in the evolution of AI is content generation — referred to as generative AI. It enables users to quickly create new content, and iterate on it, based on a variety of inputs, which can include text, images, sounds, animation, 3D models or other types of data. It then generates new content in the same or a new form.
Popular language applications, like the cloud-based ChatGPT, allow users to generate long-form copy based on a short text request. Image generators like Stable Diffusion turn descriptive text inputs into the desired image. New applications are turning text into video and 2D images into 3D renderings.
GeForce RTX AI PCs and NVIDIA RTX Workstations
AI PCs are computers with dedicated hardware designed to help AI run faster. It’s the difference between sitting around waiting for a 3D image to load, and seeing it update instantaneously with an AI denoiser.
On RTX GPUs, these specialized AI accelerators are called Tensor Cores. And they dramatically speed up AI performance across the most demanding applications for work and play.
One way that AI performance is measured is in teraops, or trillion operations per second (TOPS). Similar to an engine’s horsepower rating, TOPS can give users a sense of a PC’s AI performance with a single metric. The current generation of GeForce RTX GPUs offers performance options that range from roughly 200 AI TOPS all the way to over 1,300 TOPS, with many options across laptops and desktops in between. Professionals get even higher AI performance with the NVIDIA RTX 6000 Ada Generation GPU.
To put this in perspective, the current generation of AI PCs without GPUs range from 10 to 45 TOPS.
More and more types of AI applications will require the benefits of having a PC capable of performing certain AI tasks locally — meaning on the device rather than running in the cloud. Benefits of running on an AI PC include that computing is always available, even without an internet connection; systems offer low latency for high responsiveness; and increased privacy so that users don’t have to upload sensitive materials to an online database before it becomes usable by an AI.
AI for Everyone
RTX GPUs bring more than just performance. They introduce capabilities only possible with RTX technology. Many of these AI features are accessible — and impactful — to millions, regardless of the individual’s skill level.
From AI upscaling to improved video conferencing to intelligent, personalizable chatbots, there are tools to benefit all types of users.
RTX Video uses AI to upscale streaming video and display it in HDR. Bringing lower-resolution video in standard dynamic range to vivid, up to 4K high-resolution high dynamic range. RTX users can enjoy the feature with one-time, one-click enablement on nearly any video streamed in a Chrome or Edge browser.
NVIDIA Broadcast, a free app for RTX users with a straightforward user interface, has a host of AI features that improve video conferencing and livestreaming. It removes unwanted background sounds like clicky keyboards, vacuum cleaners and screaming children with Noise and Echo Removal. It can replace or blur backgrounds with better edge detection using Virtual Background. It smooths low-quality camera images with Video Noise Removal. And it can stay centered on the screen with eyes looking at the camera no matter where the user moves, using Auto Frame and Eye Contact.
Chat with RTX is a local, personalized AI chatbot demo that’s easy to use and free to download.
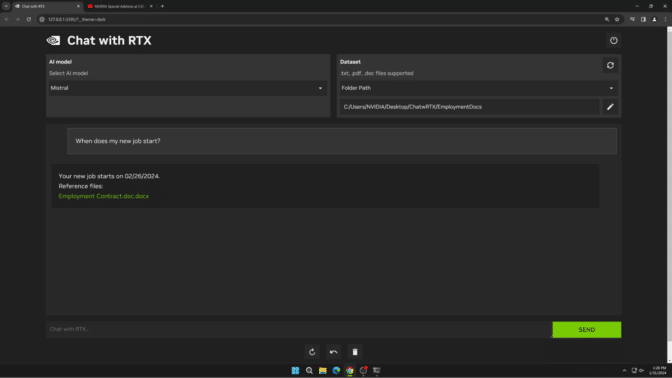
Users can easily connect local files on a PC to a supported large language model simply by dropping files into a single folder and pointing the demo to the location. It enables queries for quick, contextually relevant answers.
Since Chat with RTX runs locally on Windows with GeForce RTX PCs and NVIDIA RTX workstations, results are fast — and the user’s data stays on the device. Rather than relying on cloud-based services, Chat with RTX lets users process sensitive data on a local PC without the need to share it with a third party or have an internet connection.
AI for Gamers
Over the past six years, game performance has seen the greatest leaps with AI acceleration. Gamers have been turning NVIDIA DLSS on since 2019, boosting frame rates and improving image quality. It’s a technique that uses AI to generate pixels in video games automatically. With ongoing improvements, it now increases frame rates by up to 4x.
And with the introduction of Ray Reconstruction in the latest version, DLSS 3.5, visual quality is further enhanced in some of the world’s top titles, setting a new standard for visually richer and more immersive gameplay.
There are now over 500 games and applications that have revolutionized the ways people play and create with ray tracing, DLSS and AI-powered technologies.
Beyond frames, AI is set to improve the way gamers interact with characters and remaster classic games.
NVIDIA ACE microservices — including generative AI-powered speech and animation models — are enabling developers to add intelligent, dynamic digital avatars to games. Demonstrated at CES, ACE won multiple awards for its ability to bring game characters to life as a glimpse into the future of PC gaming.
NVIDIA RTX Remix, a platform for modders to create stunning RTX remasters of classic games, delivers generative AI tools that can transform basic textures from classic games into modern, 4K-resolution, physically based rendering materials. Several projects have already been released or are in the works, including Half-Life 2 RTX and Portal with RTX.
AI for Creators
AI is unlocking creative potential by reducing or automating tedious tasks, freeing up time for pure creativity. These features run fastest or solely on PCs with NVIDIA RTX or GeForce RTX GPUs.
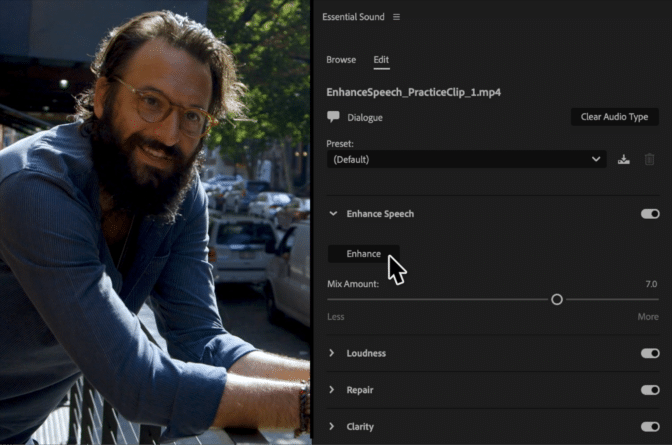
Adobe Premiere Pro’s Enhance Speech tool is accelerated by RTX, using AI to remove unwanted noise and improve the quality of dialogue clips so they sound professionally recorded. It’s up to 4.5x faster on RTX vs. Mac. Another Premiere feature, Auto Reframe, uses GPU acceleration to identify and track the most relevant elements in a video and intelligently reframes video content for different aspect ratios.
Another time-saving AI feature for video editors is DaVinci Resolve’s Magic Mask. Previously, if editors needed to adjust the color/brightness of a subject in one shot or remove an unwanted object, they’d have to use a combination of rotoscoping techniques or basic power windows and masks to isolate the subject from the background.
Magic Mask has completely changed that workflow. With it, simply draw a line over the subject and the AI will process for a moment before revealing the selection. And GeForce RTX laptops can run the feature 2.5x faster than the fastest non-RTX laptops.
This is just a sample of the ways that AI is increasing the speed of creativity. There are now more than 125 AI applications accelerated by RTX.
AI for Developers
AI is enhancing the way developers build software applications through scalable environments, hardware and software optimizations, and new APIs.
NVIDIA AI Workbench helps developers quickly create, test and customize pretrained generative AI models and LLMs using PC-class performance and memory footprint. It’s a unified, easy-to-use toolkit that can scale from running locally on RTX PCs to virtually any data center, public cloud or NVIDIA DGX Cloud.
After building AI models for PC use cases, developers can optimize them using NVIDIA TensorRT — the software that helps developers take full advantage of the Tensor Cores in RTX GPUs.
TensorRT acceleration is now available in text-based applications with TensorRT-LLM for Windows. The open-source library increases LLM performance and includes pre-optimized checkpoints for popular models, including Google’s Gemma, Meta Llama 2, Mistral and Microsoft Phi-2.
Developers also have access to a TensorRT-LLM wrapper for the OpenAI Chat API. With just one line of code change, continue.dev — an open-source autopilot for VS Code and JetBrains that taps into an LLM — can use TensorRT-LLM locally on an RTX PC for fast, local LLM inference using this popular tool.
Every week, we’ll demystify AI by making the technology more accessible, and we’ll showcase new hardware, software, tools and accelerations for RTX AI PC users.
The iPhone moment of AI is here, and it’s just the beginning. Welcome to AI Decoded.
Get weekly updates directly in your inbox by subscribing to the AI Decoded newsletter.