Today, we are excited to announce the capability to fine-tune Code Llama models by Meta using Amazon SageMaker JumpStart. The Code Llama family of large language models (LLMs) is a collection of pre-trained and fine-tuned code generation models ranging in scale from 7 billion to 70 billion parameters. Fine-tuned Code Llama models provide better accuracy and explainability over the base Code Llama models, as evident on its testing against HumanEval and MBPP datasets. You can fine-tune and deploy Code Llama models with SageMaker JumpStart using the Amazon SageMaker Studio UI with a few clicks or using the SageMaker Python SDK. Fine-tuning of Llama models is based on the scripts provided in the llama-recipes GitHub repo from Meta using PyTorch FSDP, PEFT/LoRA, and Int8 quantization techniques.
In this post, we walk through how to fine-tune Code Llama pre-trained models via SageMaker JumpStart through a one-click UI and SDK experience available in the following GitHub repository.
What is SageMaker JumpStart
With SageMaker JumpStart, machine learning (ML) practitioners can choose from a broad selection of publicly available foundation models. ML practitioners can deploy foundation models to dedicated Amazon SageMaker instances from a network isolated environment and customize models using SageMaker for model training and deployment.
What is Code Llama
Code Llama is a code-specialized version of Llama 2 that was created by further training Llama 2 on its code-specific datasets and sampling more data from that same dataset for longer. Code Llama features enhanced coding capabilities. It can generate code and natural language about code, from both code and natural language prompts (for example, “Write me a function that outputs the Fibonacci sequence”). You can also use it for code completion and debugging. It supports many of the most popular programming languages used today, including Python, C++, Java, PHP, Typescript (JavaScript), C#, Bash, and more.
Why fine-tune Code Llama models
Meta published Code Llama performance benchmarks on HumanEval and MBPP for common coding languages such as Python, Java, and JavaScript. The performance of Code Llama Python models on HumanEval demonstrated varying performance across different coding languages and tasks ranging from 38% on 7B Python model to 57% on 70B Python models. In addition, fine-tuned Code Llama models on SQL programming language have shown better results, as evident in SQL evaluation benchmarks. These published benchmarks highlight the potential benefits of fine-tuning Code Llama models, enabling better performance, customization, and adaptation to specific coding domains and tasks.
No-code fine-tuning via the SageMaker Studio UI
To start fine-tuning your Llama models using SageMaker Studio, complete the following steps:
- On the SageMaker Studio console, choose JumpStart in the navigation pane.
You will find listings of over 350 models ranging from open source and proprietary models.
- Search for Code Llama models.
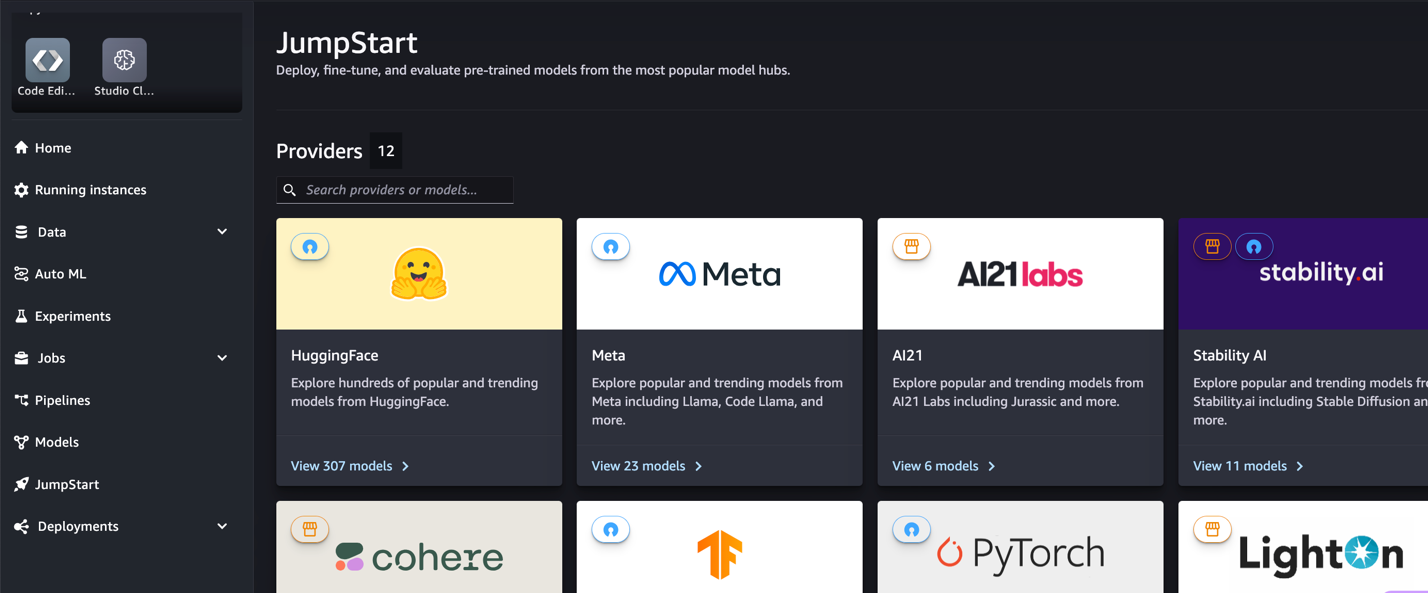
If you don’t see Code Llama models, you can update your SageMaker Studio version by shutting down and restarting. For more information about version updates, refer to Shut down and Update Studio Apps. You can also find other model variants by choosing Explore all Code Generation Models or searching for Code Llama in the search box.
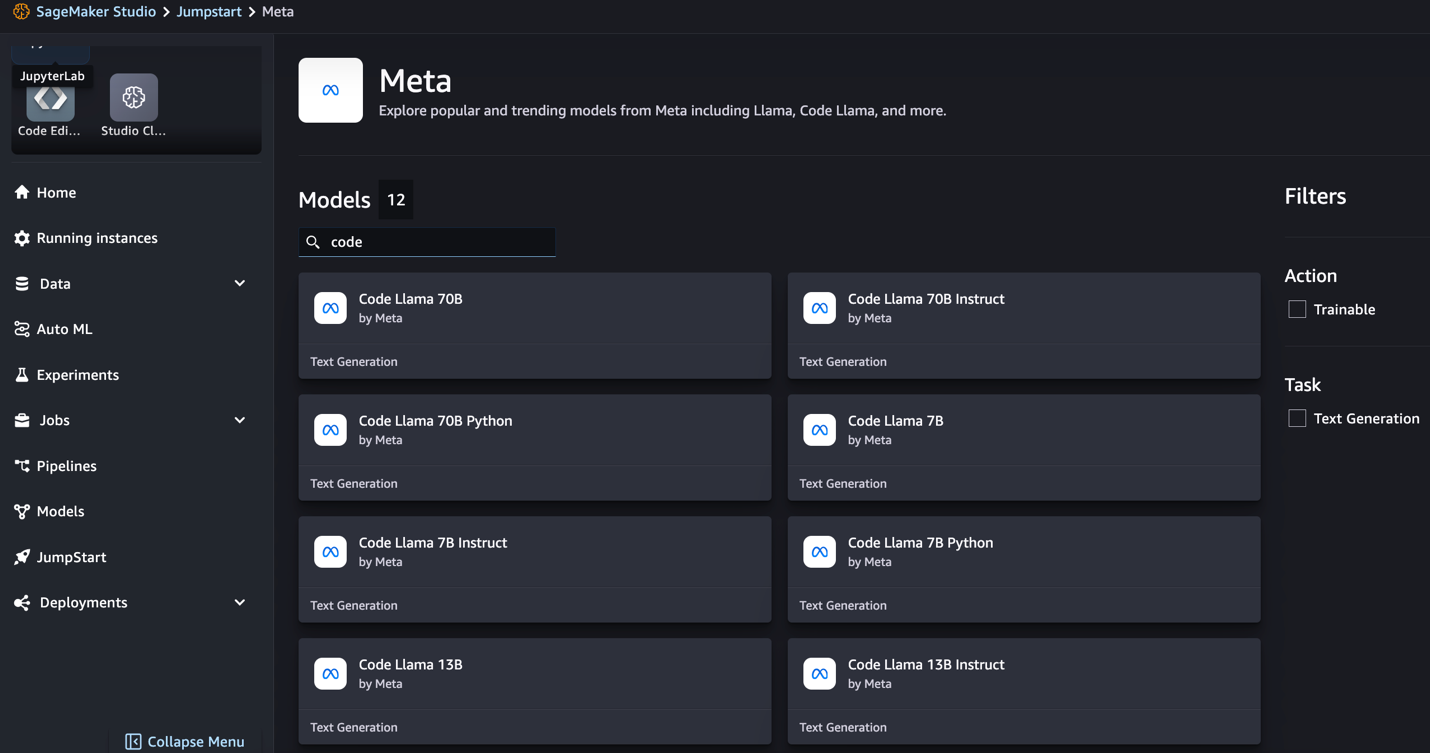
SageMaker JumpStart currently supports instruction fine-tuning for Code Llama models. The following screenshot shows the fine-tuning page for the Code Llama 2 70B model.
- For Training dataset location, you can point to the Amazon Simple Storage Service (Amazon S3) bucket containing the training and validation datasets for fine-tuning.
- Set your deployment configuration, hyperparameters, and security settings for fine-tuning.
- Choose Train to start the fine-tuning job on a SageMaker ML instance.
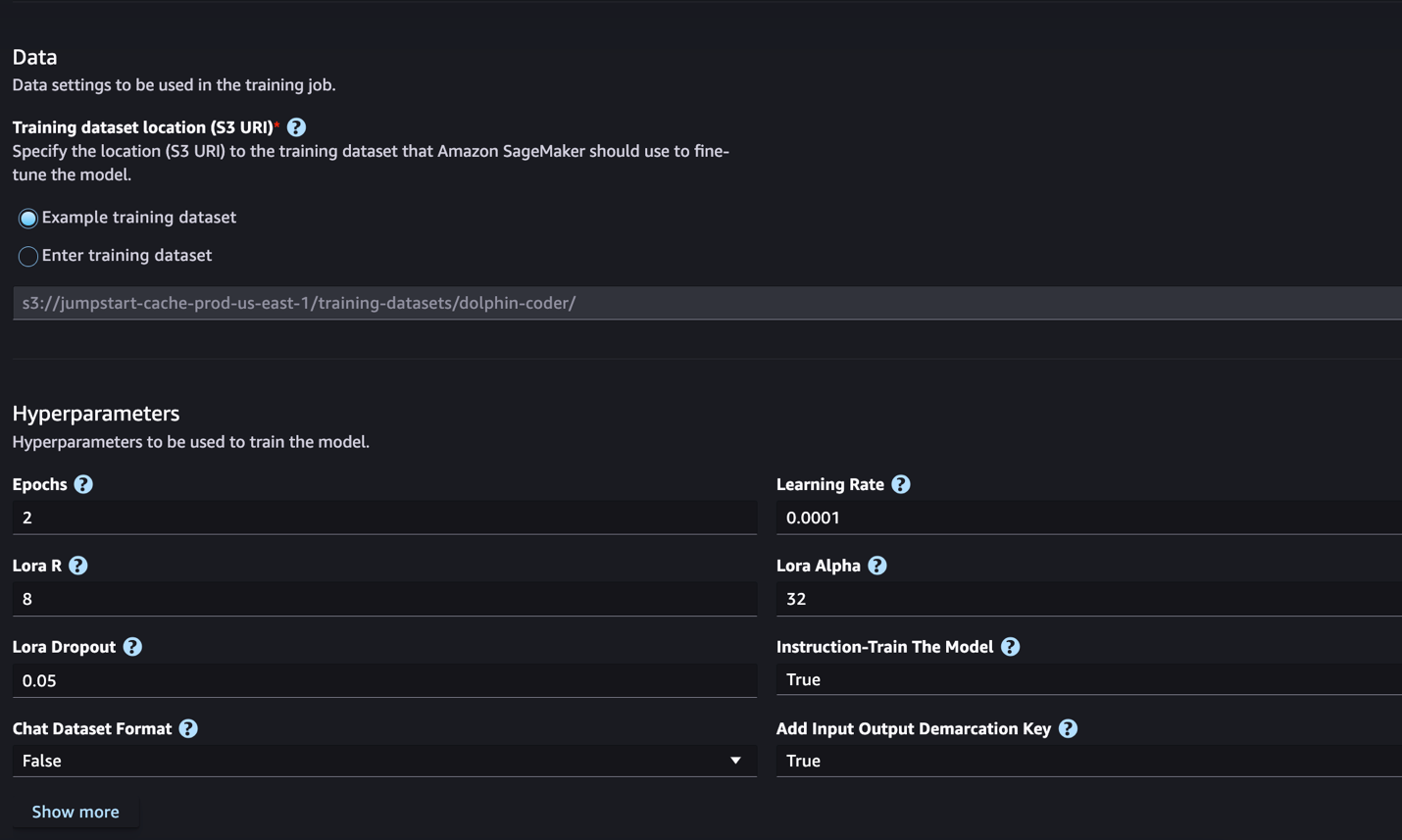
We discuss the dataset format you need prepare for instruction fine-tuning in the next section.
- After the model is fine-tuned, you can deploy it using the model page on SageMaker JumpStart.
The option to deploy the fine-tuned model will appear when fine-tuning is finished, as shown in the following screenshot.
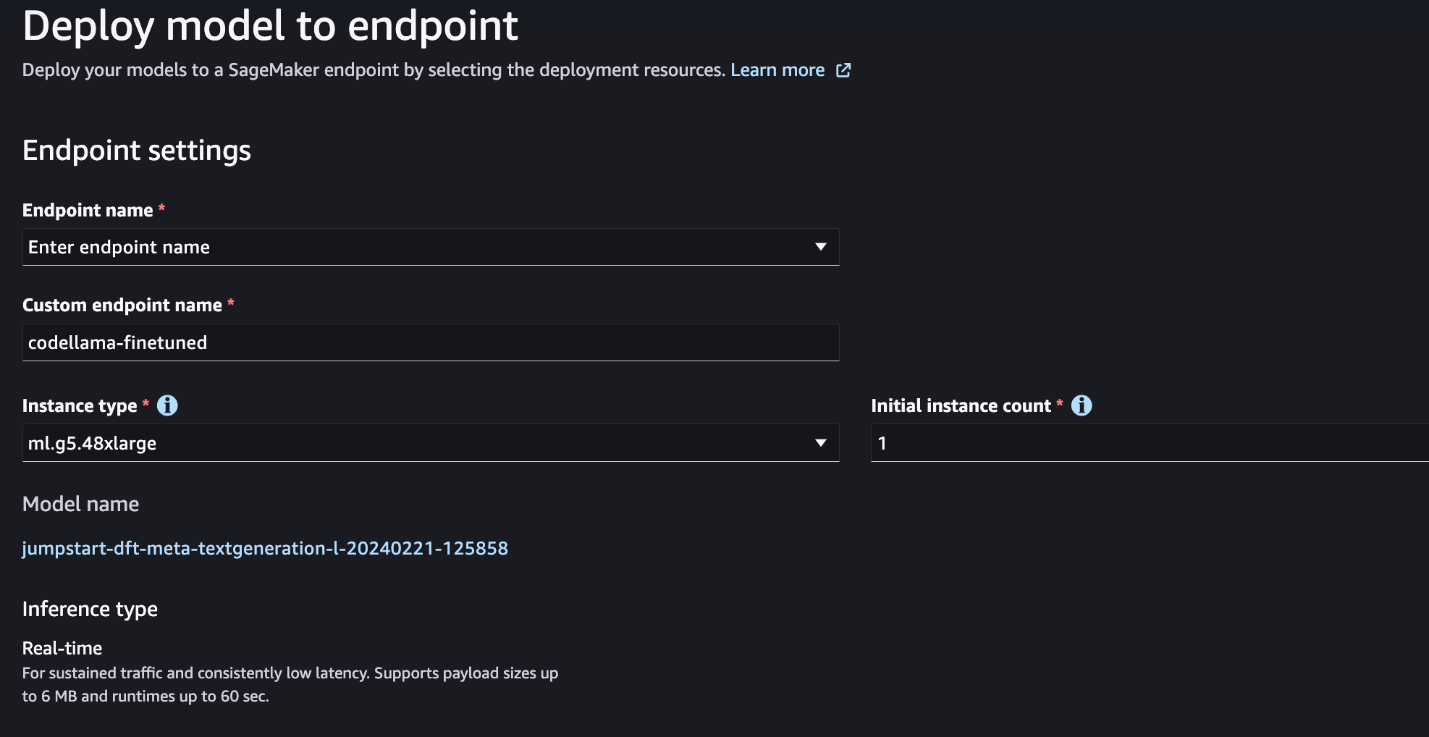
Fine-tune via the SageMaker Python SDK
In this section, we demonstrate how to fine-tune Code LIama models using the SageMaker Python SDK on an instruction-formatted dataset. Specifically, the model is fine-tuned for a set of natural language processing (NLP) tasks described using instructions. This helps improve the model’s performance for unseen tasks with zero-shot prompts.
Complete the following steps to complete your fine-tuning job. You can get the entire fine-tuning code from the GitHub repository.
First, let’s look at the dataset format required for the instruction fine-tuning. The training data should be formatted in a JSON lines (.jsonl) format, where each line is a dictionary representing a data sample. All training data must be in a single folder. However, it can be saved in multiple .jsonl files. The following is a sample in JSON lines format:
{
'system_prompt': 'a chat',
'question': 'Please focus on the efficiency of this problem and provide code in python:nYou are given two strings `s` and `t` consisting of only lowercase English letters.nnReturn _the minimum number of characters that need to be appended to the end of_ `s` _so that_ `t` _becomes a **subsequence** of_ `s`.nnA **subsequence** is a string that can be derived from another string by deleting some or no characters without changing the order of the remaining characters.nn**Example 1:**nn**Input:** s = "coaching ", t = "coding "n**Output:** 4n**Explanation:** Append the characters "ding " to the end of s so that s = "coachingding ".nNow, t is a subsequence of s ( "**co**aching**ding** ").nIt can be shown that appending any 3 characters to the end of s will never make t a subsequence.nn**Example 2:**nn**Input:** s = "abcde ", t = "a "n**Output:** 0n**Explanation:** t is already a subsequence of s ( "**a**bcde ").nn**Example 3:**nn**Input:** s = "z ", t = "abcde "n**Output:** 5n**Explanation:** Append the characters "abcde " to the end of s so that s = "zabcde ".nNow, t is a subsequence of s ( "z**abcde** ").nIt can be shown that appending any 4 characters to the end of s will never make t a subsequence.nn**Constraints:**nn* `1 <= s.length, t.length <= 105`n* `s` and `t` consist only of lowercase English letters.',
'response': '### Explanationn The algorithm maintains two pointers, `i` and `j`, to iterate through the given strings `s` and `t`. The pointer `i` iterates through the string `s` and the pointer `j` iterates through the string `t`. While iterating through `s`, if we find a character that matches the current character in `t`, we increment the `j` pointer. This is because the matched character can be a part of the subsequence we are looking for. The iteration stops when either `i` reaches the end of `s` or `j` reaches the end of `t`.nnAt the end, we subtract the index `j` from the length of `t`. This gives us the minimum number of characters that need to be appended to the end of `s` so that `t` becomes a subsequence of `s`.nn### Pseudocode:n```nMIN-ADDITION(s, t)n s_len = LENGTH(s)n t_len = LENGTH(t)n i = 1n j = 1n WHILE i <= s_len AND j <= t_lenn IF s[i] == t[j]n j = j + 1n ENDIFn i = i + 1n ENDWHILEn RETURN t_len - j + 1nENDMIN-ADDITIONn```nn### Coden```pythonndef min_addition(s: str, t: str) -> int:n s_len = len(s)n t_len = len(t)n i, j = 0, 0n while i < s_len and j < t_len:n if s[i] == t[j]:n j += 1n i += 1n return t_len - jn```n'
}
The training folder can contain a template.json
file describing the input and output formats. The following is an example template:
{
"prompt": "{system_prompt} ### Input: {question}",
"completion": "{response}"
}
To match the template, each sample in the JSON lines files must include system_prompt
, question
, and response
fields. In this demonstration, we use the Dolphin Coder dataset from Hugging Face.
After you prepare the dataset and upload it to the S3 bucket, you can start fine-tuning using the following code:
from sagemaker.jumpstart.estimator import JumpStartEstimator
model_id = "meta-textgeneration-llama-codellama-7b"
model_version = "*"
train_data_location = f"s3://{your_own_bucket_hosting_training_data}/" # training data in s3 bucket
estimator = JumpStartEstimator(
model_id=model_id,
model_version=model_version,
hyperparameters= hyperparameters,
environment={
"accept_eula": "false"
}, # please change `accept_eula` to be `true` to accept EULA.
)
estimator.fit({"training": train_data_location})
You can deploy the fine-tuned model directly from the estimator, as shown in the following code. For details, see the notebook in the GitHub repository.
finetuned_predictor = estimator.deploy()
Fine-tuning techniques
Language models such as Llama are more than 10 GB or even 100 GB in size. Fine-tuning such large models requires instances with significantly high CUDA memory. Furthermore, training these models can be very slow due to the size of the model. Therefore, for efficient fine-tuning, we use the following optimizations:
- Low-Rank Adaptation (LoRA) – This is a type of parameter efficient fine-tuning (PEFT) for efficient fine-tuning of large models. With this method, you freeze the whole model and only add a small set of adjustable parameters or layers into the model. For instance, instead of training all 7 billion parameters for Llama 2 7B, you can fine-tune less than 1% of the parameters. This helps in significant reduction of the memory requirement because you only need to store gradients, optimizer states, and other training-related information for only 1% of the parameters. Furthermore, this helps in reduction of training time as well as the cost. For more details on this method, refer to LoRA: Low-Rank Adaptation of Large Language Models.
- Int8 quantization – Even with optimizations such as LoRA, models such as Llama 70B are still too big to train. To decrease the memory footprint during training, you can use Int8 quantization during training. Quantization typically reduces the precision of floating point data types. Although this decreases the memory required to store model weights, it degrades the performance due to loss of information. Int8 quantization uses only a quarter precision but doesn’t incur degradation of performance because it doesn’t simply drop the bits. It rounds the data from one type to the another. To learn about Int8 quantization, refer to LLM.int8(): 8-bit Matrix Multiplication for Transformers at Scale.
- Fully Sharded Data Parallel (FSDP) – This is a type of data-parallel training algorithm that shards the model’s parameters across data parallel workers and can optionally offload part of the training computation to the CPUs. Although the parameters are sharded across different GPUs, computation of each microbatch is local to the GPU worker. It shards parameters more uniformly and achieves optimized performance via communication and computation overlapping during training.
The following table summarizes the details of each model with different settings.
Model |
Default Setting |
LORA + FSDP |
LORA + No FSDP |
Int8 Quantization + LORA + No FSDP |
Code Llama 2 7B |
LORA + FSDP |
Yes |
Yes |
Yes |
Code Llama 2 13B |
LORA + FSDP |
Yes |
Yes |
Yes |
Code Llama 2 34B |
INT8 + LORA + NO FSDP |
No |
No |
Yes |
Code Llama 2 70B |
INT8 + LORA + NO FSDP |
No |
No |
Yes |
Fine-tuning of Llama models is based on scripts provided by the following GitHub repo.
Supported hyperparameters for training
Code Llama 2 fine-tuning supports a number of hyperparameters, each of which can impact the memory requirement, training speed, and performance of the fine-tuned model:
- epoch – The number of passes that the fine-tuning algorithm takes through the training dataset. Must be an integer greater than 1. Default is 5.
- learning_rate – The rate at which the model weights are updated after working through each batch of training examples. Must be a positive float greater than 0. Default is 1e-4.
- instruction_tuned – Whether to instruction-train the model or not. Must be
True
or False
. Default is False
.
- per_device_train_batch_size – The batch size per GPU core/CPU for training. Must be a positive integer. Default is 4.
- per_device_eval_batch_size – The batch size per GPU core/CPU for evaluation. Must be a positive integer. Default is 1.
- max_train_samples – For debugging purposes or quicker training, truncate the number of training examples to this value. Value -1 means using all of the training samples. Must be a positive integer or -1. Default is -1.
- max_val_samples – For debugging purposes or quicker training, truncate the number of validation examples to this value. Value -1 means using all of the validation samples. Must be a positive integer or -1. Default is -1.
- max_input_length – Maximum total input sequence length after tokenization. Sequences longer than this will be truncated. If -1,
max_input_length
is set to the minimum of 1024 and the maximum model length defined by the tokenizer. If set to a positive value, max_input_length
is set to the minimum of the provided value and the model_max_length
defined by the tokenizer. Must be a positive integer or -1. Default is -1.
- validation_split_ratio – If validation channel is
none
, the ratio of the train-validation split from the train data must be between 0–1. Default is 0.2.
- train_data_split_seed – If validation data is not present, this fixes the random splitting of the input training data to training and validation data used by the algorithm. Must be an integer. Default is 0.
- preprocessing_num_workers – The number of processes to use for preprocessing. If
None
, the main process is used for preprocessing. Default is None
.
- lora_r – Lora R. Must be a positive integer. Default is 8.
- lora_alpha – Lora Alpha. Must be a positive integer. Default is 32
- lora_dropout – Lora Dropout. must be a positive float between 0 and 1. Default is 0.05.
- int8_quantization – If
True
, the model is loaded with 8-bit precision for training. Default for 7B and 13B is False
. Default for 70B is True
.
- enable_fsdp – If True, training uses FSDP. Default for 7B and 13B is True. Default for 70B is False. Note that
int8_quantization
is not supported with FSDP.
When choosing the hyperparameters, consider the following:
- Setting
int8_quantization=True
decreases the memory requirement and leads to faster training.
- Decreasing
per_device_train_batch_size
and max_input_length
reduces the memory requirement and therefore can be run on smaller instances. However, setting very low values may increase the training time.
- If you’re not using Int8 quantization (
int8_quantization=False
), use FSDP (enable_fsdp=True
) for faster and efficient training.
Supported instance types for training
The following table summarizes the supported instance types for training different models.
Model |
Default Instance Type |
Supported Instance Types |
Code Llama 2 7B |
ml.g5.12xlarge |
ml.g5.12xlarge,
ml.g5.24xlarge,
ml.g5.48xlarge,
ml.p3dn.24xlarge,
ml.g4dn.12xlarge
|
Code Llama 2 13B |
ml.g5.12xlarge |
ml.g5.24xlarge,
ml.g5.48xlarge,
ml.p3dn.24xlarge,
ml.g4dn.12xlarge
|
Code Llama 2 70B |
ml.g5.48xlarge |
ml.g5.48xlarge
ml.p4d.24xlarge
|
When choosing the instance type, consider the following:
- G5 instances provide the most efficient training among the instance types supported. Therefore, if you have G5 instances available, you should use them.
- Training time largely depends on the amount of the number of GPUs and the CUDA memory available. Therefore, training on instances with the same number of GPUs (for example, ml.g5.2xlarge and ml.g5.4xlarge) is roughly the same. Therefore, you can use the cheaper instance for training (ml.g5.2xlarge).
- When using p3 instances, training will be done with 32-bit precision because bfloat16 is not supported on these instances. Therefore, the training job will consume double the amount of CUDA memory when training on p3 instances compared to g5 instances.
To learn about the cost of training per instance, refer to Amazon EC2 G5 Instances.
Evaluation
Evaluation is an important step to assess the performance of fine-tuned models. We present both qualitative and quantitative evaluations to show improvement of fine-tuned models over non-fine-tuned ones. In qualitative evaluation, we show an example response from both fine-tuned and non-fine-tuned models. In quantitative evaluation, we use HumanEval, a test suite developed by OpenAI to generate Python code to test the abilities of producing correct and accurate results. The HumanEval repository is under MIT license. We fine-tuned Python variants of all Code LIama models over different sizes (Code LIama Python 7B, 13B, 34B, and 70B on the Dolphin Coder dataset), and present the evaluation results in the following sections.
Qualitatively evaluation
With your fine-tuned model deployed, you can start using the endpoint to generate code. In the following example, we present responses from both base and fine-tuned Code LIama 34B Python variants on a test sample in the Dolphin Coder dataset:
alpaca_format = """{system_prompt}
### Input:
{question}
### Response:n"""
system="You are a coding AI"
prompt = """Please explain the reasoning behind the following solution and
provide code in python: Given a string `s`, return the longest palindromic
substring in `s`. **Example 1:** **Input:** s = "babad " **Output:** "bab "
**Explanation:** "aba " is also a valid answer. **Example 2:**
**Input:** s = "cbbd " **Output:** "bb "
**Constraints:** * `1 <= s.length <= 1000` * `s` consist of only digits
and English letters."""
payload = json.dumps({
"inputText": alpaca_format.format(system_prompt=system, question=prompt),
"textGenerationConfig": {
"maxTokenCount": 2048,
"temperature":0.2
}})
finetuned_response = finetuned_predictor.predict(payload)
finetuned_response["generated_text"]
The fine-tuned Code Llama model, in addition to providing the code for the preceding query, generates a detailed explanation of the approach and a pseudo code.
Code Llama 34b Python Non-Fine-Tuned Response:
### Code
def longestPalindrome(s):
# Fill this in.
longest = ""
for i in range(len(s)):
for j in range(len(s), i, -1):
if len(longest) >= j-i:
break
elif s[i:j] == s[i:j][::-1]:
longest = s[i:j]
break
return longest
print(longestPalindrome("abaxyzzyxf"))
# axyzzyx
###Reference:
- [https://www.geeksforgeeks.org/longest-palindrome-substring-set-1/]
(https://www.geeksforgeeks.org/longest-palindrome-substring-set-1/)
Code Llama 34B Python Fine-Tuned Response
### Explanation
The algorithm uses dynamic programming to find the longest palindromic substring.
It initializes a 2D boolean table `dp` with the same dimensions as the input string `s`.
The `dp[i][j]` value is set to `true` if the substring from index `i` to index `j` is a palindrome.
The algorithm then iterates through the string in a nested loop, starting from the first character to the last.
It checks if the substring from index `i` to index `j` is a palindrome.
If it is, it sets the `dp[i][j]` value to `true`.
If the substring is of length 2 or 3, it sets the `dp[i][j]` value to `true` as well.
After the nested loop, the algorithm iterates through the `dp` table to find the longest palindromic substring.
It keeps track of the maximum length and the starting and ending indices of the substring.
Finally, the algorithm returns the substring from the starting index to the ending index.
### Code
```python
def longestPalindrome(s: str) -> str:
n = len(s)
dp = [[False] * n for _ in range(n)]
for i in range(n):
dp[i][i] = True
if i + 1 < n:
dp[i][i + 1] = s[i] == s[i + 1]
max_len = 1
start = 0
end = 0
for i in range(n - 1):
for j in range(i + 1, n):
if j - i == 1 or dp[i + 1][j - 1]:
dp[i][j] = s[i] == s[j]
if j - i + 1 > max_len:
max_len = j - i + 1
start = i
end = j
return s[start:end + 1]
```
Ground Truth
### Explanation
1. Initialize `start` and `maxLength` for result substring.
2. Iterate through the given string `s` using the index `i`.
3. For each index `i`, create two pointers `l` and `r` starting at `i`.
4. Check if there's a consecutive sequence of identical characters, increment the right pointer `r` until the end of the sequence is reached.
5. Update the index `i` to the current value of `r`.
6. Expand the pointers `l` and `r` outwards to find the longest palindromic substring, checking that characters on both sides are equal.
7. If the current length of the substring is greater than `maxLength`, update `start` and `maxLength`.
8. Return the longest palindromic substring using the `start` and `maxLength`.
### Code
```python
def longest_palindromic_substring(s: str) → str:
n = len(s)
if n == 0:
return ""
start, max_length = 0, 1
for i in range(n):
l, r = i, i
while r < n - 1 and s[r] == s[r + 1]:
r += 1
i = r
while l > 0 and r < n - 1 and s[l - 1] == s[r + 1]:
l -= 1
r += 1
length = r - l + 1
if length > max_length:
start, max_length = l, length
return s[start:start + max_length]
```
Interestingly, our fine-tuned version of Code Llama 34B Python provides a dynamic programming-based solution to the longest palindromic substring, which is different from the solution provided in the ground truth from the selected test example. Our fine-tuned model reasons and explains the dynamic programming-based solution in detail. On the other hand, the non-fine-tuned model hallucinates potential outputs right after the print
statement (shown in the left cell) because the output axyzzyx
is not the longest palindrome in the given string. In terms of time complexity, the dynamic programming solution is generally better than the initial approach. The dynamic programming solution has a time complexity of O(n^2), where n is the length of the input string. This is more efficient than the initial solution from the non-fine-tuned model, which also had a quadratic time complexity of O(n^2) but with a less optimized approach.
This looks promising! Remember, we only fine-tuned the Code LIama Python variant with 10% of the Dolphin Coder dataset. There is a lot more to explore!
Despite of thorough instructions in the response, we still need examine the correctness of the Python code provided in the solution. Next, we use an evaluation framework called Human Eval to run integration tests on the generated response from Code LIama to systematically examine its quality.
Quantitative evaluation with HumanEval
HumanEval is an evaluation harness for evaluating an LLM’s problem-solving capabilities on Python-based coding problems, as described in the paper Evaluating Large Language Models Trained on Code. Specifically, it consists of 164 original Python-based programming problems that assess a language model’s ability to generate code based on provided information like function signature, docstring, body, and unit tests.
For each Python-based programming question, we send it to a Code LIama model deployed on a SageMaker endpoint to get k responses. Next, we run each of the k responses on the integration tests in the HumanEval repository. If any response of the k responses passes the integration tests, we count that test case succeed; otherwise, failed. Then we repeat the process to calculate the ratio of successful cases as the final evaluation score named pass@k
. Following standard practice, we set k as 1 in our evaluation, to only generate one response per question and test whether it passes the integration test.
The following is a sample code to use HumanEval repository. You can access the dataset and generate a single response using a SageMaker endpoint. For details, see the notebook in the GitHub repository.
%pip3 install human_eval
import json
from human_eval.evaluation import evaluate_functional_correctness
from human_eval.data import write_jsonl, read_problems
from tqdm import tqdm
problems = read_problems()
num_samples_per_task = 1 # value k: number of responses for each question
samples = [
dict(task_id=task_id, completion=generate_one_completion(problems[task_id]["prompt"]))
for task_id in tqdm(problems)
for _ in range(num_samples_per_task)
]
write_jsonl("samples.jsonl", samples)
evaluate_functional_correctness('./samples.jsonl')
The following table shows the improvements of the fine-tuned Code LIama Python models over the non-fine-tuned models across different model sizes. To ensure correctness, we also deploy the non-fine-tuned Code LIama models in SageMaker endpoints and run through Human Eval evaluations. The pass@1 numbers (the first row in the following table) match the reported numbers in the Code Llama research paper. The inference parameters are consistently set as "parameters": {"max_new_tokens": 384, "temperature": 0.2}
.
As we can see from the results, all the fine-tuned Code LIama Python variants show significant improvement over the non-fine-tuned models. In particular, Code LIama Python 70B outperforms the non-fine-tuned model by approximately 12%.
. |
7B Python |
13B Python |
34B |
34B Python |
70B Python |
Pre-trained model performance (pass@1) |
38.4 |
43.3 |
48.8 |
53.7 |
57.3 |
Fine-tuned model performance (pass@1) |
45.12 |
45.12 |
59.1 |
61.5 |
69.5 |
Now you can try fine-tuning Code LIama models on your own dataset.
Clean up
If you decide that you no longer want to keep the SageMaker endpoint running, you can delete it using AWS SDK for Python (Boto3), AWS Command Line Interface (AWS CLI), or SageMaker console. For more information, see Delete Endpoints and Resources. Additionally, you can shut down the SageMaker Studio resources that are no longer required.
Conclusion
In this post, we discussed fine-tuning Meta’s Code Llama 2 models using SageMaker JumpStart. We showed that you can use the SageMaker JumpStart console in SageMaker Studio or the SageMaker Python SDK to fine-tune and deploy these models. We also discussed the fine-tuning technique, instance types, and supported hyperparameters. In addition, we outlined recommendations for optimized training based on various tests we carried out. As we can see from these results of fine-tuning three models over two datasets, fine-tuning improves summarization compared to non-fine-tuned models. As a next step, you can try fine-tuning these models on your own dataset using the code provided in the GitHub repository to test and benchmark the results for your use cases.
About the Authors
Dr. Xin Huang is a Senior Applied Scientist for Amazon SageMaker JumpStart and Amazon SageMaker built-in algorithms. He focuses on developing scalable machine learning algorithms. His research interests are in the area of natural language processing, explainable deep learning on tabular data, and robust analysis of non-parametric space-time clustering. He has published many papers in ACL, ICDM, KDD conferences, and Royal Statistical Society: Series A.
Vishaal Yalamanchali is a Startup Solutions Architect working with early-stage generative AI, robotics, and autonomous vehicle companies. Vishaal works with his customers to deliver cutting-edge ML solutions and is personally interested in reinforcement learning, LLM evaluation, and code generation. Prior to AWS, Vishaal was an undergraduate at UCI, focused on bioinformatics and intelligent systems.
Meenakshisundaram Thandavarayan works for AWS as an AI/ ML Specialist. He has a passion to design, create, and promote human-centered data and analytics experiences. Meena focuses on developing sustainable systems that deliver measurable, competitive advantages for strategic customers of AWS. Meena is a connector and design thinker, and strives to drive businesses to new ways of working through innovation, incubation, and democratization.
Dr. Ashish Khetan is a Senior Applied Scientist with Amazon SageMaker built-in algorithms and helps develop machine learning algorithms. He got his PhD from University of Illinois Urbana-Champaign. He is an active researcher in machine learning and statistical inference, and has published many papers in NeurIPS, ICML, ICLR, JMLR, ACL, and EMNLP conferences.
Read More