An animation that projects traffic fluctuations onto the U.S. map offers an example of how the Supply Chain Optimization Technologies team uses data visualization to glean insights.Read More
Bethesda’s ‘Fallout’ Titles Join GeForce NOW
Welcome to the wasteland, Vault Dwellers. Bethesda’s Fallout 4 and Fallout 76 are bringing post-nuclear adventures to the cloud.
These highly acclaimed action role-playing games lead 10 new titles joining GeForce NOW this week.
Announced as coming to GeForce NOW at CES, Honkai: Star Rail is targeting a release this quarter. Stay tuned for future updates.
Vault Into the Cloud
Adventurers needed, whether for mapping the irradiated wasteland or shaping the fate of humanity.
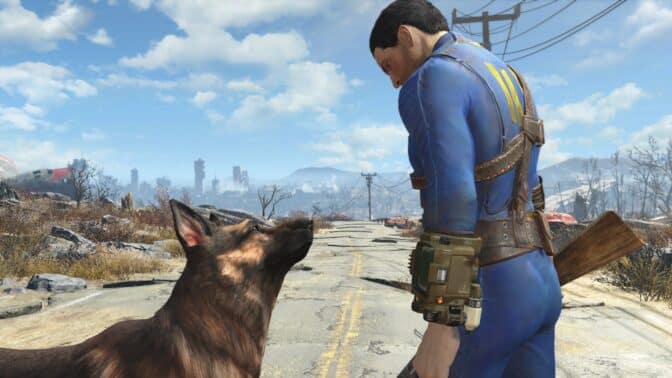
Embark on a journey through ruins of the post-apocalyptic Commonwealth in Fallout 4. As the sole survivor of Vault 111, navigate a world destroyed by nuclear war, make choices to reshape the wasteland and rebuild society one settlement at a time. With a vast, open world, dynamic crafting systems and a gripping storyline, the game offers an immersive single-player experience that challenges dwellers to emerge as beacons of hope for humanity’s remnants.
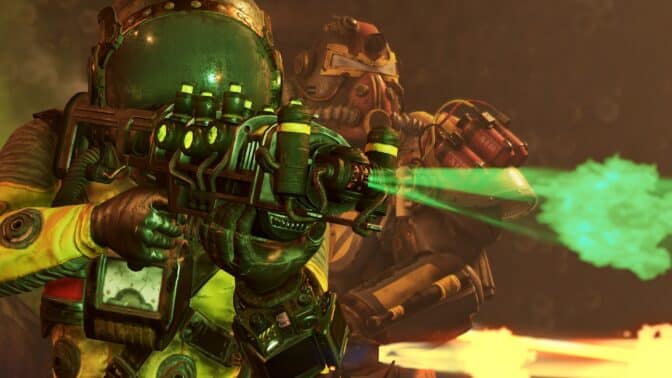
Plus, in Fallout 76, head back to the early days of post-nuclear Appalachia and experience the Fallout universe’s largest, most dynamic world. Encounter unique challenges, build portable player homes called C.A.M.P.s, and cooperate or compete with other survivors in the mountainous lands in West Virginia.
Join the proud ranks of Vault survivors in the cloud today and stream these titles, including Creation Club content for Fallout 4, across devices. With longer gaming sessions and faster access to servers, GeForce NOW members can play anywhere, anytime, and at up to 4K resolution, streaming with an Ultimate membership. The games come just in time for those tuning into the Fallout series TV adaptation, released today, for a Fallout-filled week.
Go Big or Go Home
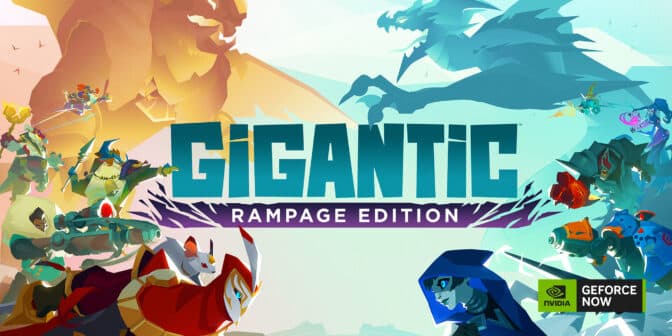
Gigantic: Rampage Edition promises big fun with epic 5v5 matches, crossplay support, an exciting roster of heroes and more. Rush to the cloud to jump into the latest game from Arc Games and team with four other players to control objectives and take down the opposing team’s mighty Guardian. Think fast, be bold and go gigantic!
Look forward to these new games this week:
- Gigantic: Rampage Edition (New release on Steam, April 9)
- Inkbound 1.0 (New release, on Steam, April 9)
- Broken Roads (New release on Steam, April 10)
- Infection Free Zone (New release on Steam, April 11)
- Shadow of the Tomb Raider: Definitive Edition (New release on Xbox and available on PC Game Pass, April 11)
- Backpack Battles (Steam)
- Fallout 4 (Steam)
- Fallout 76 (Steam and Xbox, available on PC Game Pass)
- Ghostrunner (Epic Games Store, free April 11-18)
- Terra Invicta (Xbox, available on PC Game Pass)
What are you planning to play this weekend? Let us know on X or in the comments below.
can’t help 𝙛𝙖𝙡𝙡ing into the cloud
—
NVIDIA GeForce NOW (@NVIDIAGFN) April 10, 2024
Ideas: Language technologies for everyone with Kalika Bali
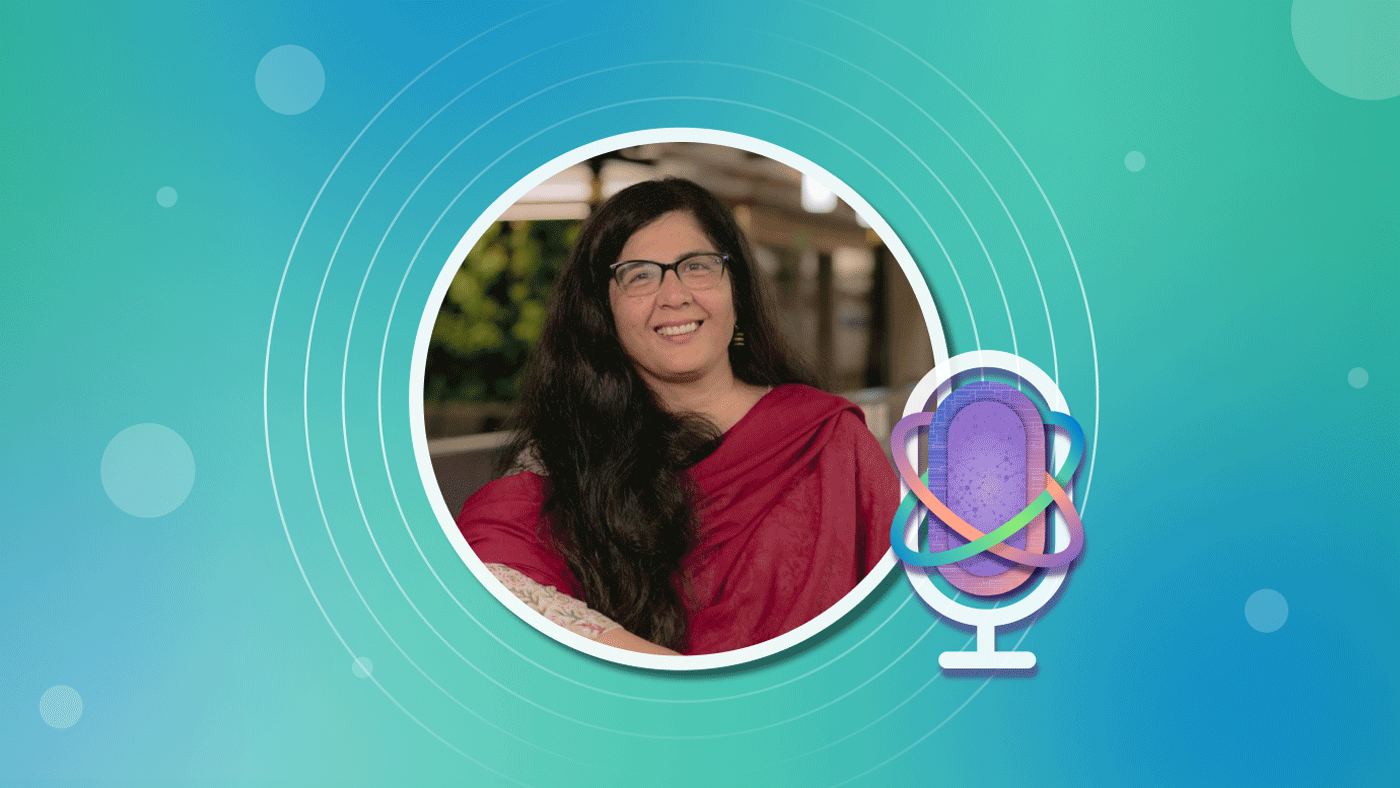
Behind every emerging technology is a great idea propelling it forward. In the new Microsoft Research Podcast series, Ideas, members of the research community at Microsoft discuss the beliefs that animate their research, the experiences and thinkers that inform it, and the positive human impact it targets.
In this episode, host Gretchen Huizinga talks with Principal Researcher Kalika Bali. Inspired by an early vision of “talking computers” and a subsequent career in linguistics, Bali has spent the last two decades bringing the two together. Aided by recent advances in large language models and motivated by her belief that everyone should have access to AI in their own language, Bali and her teams are building language technology applications that they hope will bring the benefits of generative AI to under-resourced and underserved language communities around the world.
Learn more:
- The State and Fate of Linguistic Diversity and Inclusion in the NLP World
Publication, July 2020 - Project VeLLM
Project page - Kahani: Visual Storytelling
Project page - Kahani: Visual Storytelling through Culturally Nuanced Images
Microsoft Research Forum | Episode 1, January 2024 - Teachers in India help Microsoft Research design AI tool for creating great classroom content
Microsoft Research blog, October 2023 - Digital Labor: Project Karya
Project page - Village by village, creating the building blocks for AI tools with work that also educates (opens in new tab)
Microsoft Source Asia blog, February 2024
Subscribe to the Microsoft Research Podcast:
Transcript
[TEASER] [MUSIC PLAYS UNDER DIALOGUE]KALIKA BALI: I do think, in some sense, the pushback that I got for my idea makes me think it was outrageous. I didn’t think it was outrageous at all at that time! I thought it was a very reasonable idea! But there was a very solid pushback and not just from your colleagues. You know, for researchers, publishing papers is important! No one would publish a paper which focused only on, say, Indian languages or low-resource languages. We’ve come a very long way even in the research community on that, right. We kept pushing, pushing, pushing! And now there are tracks, there are workshops, there are conferences which are devoted to multilingual and low-resource languages.
[TEASER ENDS]GRETCHEN HUIZINGA: You’re listening to Ideas, a Microsoft Research Podcast that dives deep into the world of technology research and the profound questions behind the code. I’m Dr. Gretchen Huizinga. In this series, we’ll explore the technologies that are shaping our future and the big ideas that propel them forward.
[MUSIC FADES]
I’m excited to be live in the booth today with Kalika Bali, a principal researcher at Microsoft Research India. Kalika is working on language technologies that she hopes will bring the benefits of generative AI to under-resourced and underserved language communities around the world. Kalika, it’s a pleasure to speak with you today. Welcome to Ideas!
KALIKA BALI: Thank you. Thank you, Gretchen. Thank you for having me.
HUIZINGA: So before we dive in on the big ideas behind Kalika Bali’s research, let’s talk about you for a second. Tell us about your “origin story,” as it were, and if there is one, what “big idea” or animating “what if?” captured your imagination and inspired you to do what you’re doing today?
BALI: So, you know, I’m a great reader. I started reading well before I was taught in school how to read, and I loved science fiction. I come from a family where reading was very much a part of our everyday lives. My dad was a journalist, and I had read a lot of science fiction growing up, and I also saw a lot of science fiction, you know, movies … Star Trek … everything that I could get hold of in India. And I remember watching 2001: Space Odyssey. And there was this HAL that spoke. He actually communicated that he was a computer. And I was just so struck by it. I was like, this is so cool! You know, here are computers that can talk! Now, how cool would that be if it would happen in real life? I was not at all aware of what was happening in speech technology, whether it was possible or not possible, but that’s something that really got me into it. I’ve always, like, kind of, been very curious about languages and how they work and, you know, how people use different things in languages to express not just meaning, not just communicating, but you know expressing themselves, really. And so I think it’s a combination of HAL and this curiosity I had about the various ways in which people use languages that got me into what I’m doing now.
HUIZINGA: OK. So that’s an interesting path, and I want to go into that just a little bit, but let me anchor this: how old were you when you saw this talking computer?
BALI: Oh, I was in my early teens.
HUIZINGA: OK. And so at that time, did you have any conception that … ?
BALI: No. You know, there weren’t computers around me when I was growing up. We saw, you know, some at school, you know, people coded in BASIC …
HUIZINGA: Right?
BALI: And we heard about them a lot, but I hadn’t seen one since I was in high school.
HUIZINGA: OK. So there’s this inception moment, an aha moment, of that little spark and then you kind of drifted away from the computer side of it, and what … tell us about how you went from there to that!
BALI: So that, that’s actually a very funny story because I actually wanted to study chemistry. I was really fascinated by how these, you know, molecular parts rotate around each other and, you know, we can’t even tell where an electron is, etc. It sounded, like, really fun and cool. So I actually studied chemistry, but then I was actually going to pick up the admission form for my sister, who wanted to study in this university, and … or, no, she wanted to take an exam for her master’s. And I went there. I picked up the form, and I said, this is a cool place. I would love to study here! And then I started looking at everything like, you know, what can I apply for here? And something called linguistics came up, and I had no idea what linguistics was. So I went to the British Library, got like a thin book on introduction to linguistics, and it sounded fun! And I took the exam. And then, as they say, that was history. Then I just got into it.
HUIZINGA: OK. I mean, so much has happened in between then and now, and I think we’ll kind of get there in … but I do want you to connect the larger dot from how you got from linguistics to Microsoft Research [LAUGHTER] as a computer scientist.
BALI: So I actually started teaching at the University of South Pacific as a linguistics faculty in Fiji. And I was very interested in acoustics of speech sounds, etc., etc. That’s what I was teaching. And then there was a speech company in Belgium that was looking to start some work in Indian languages, and they contacted me, and at that time, you needed people who knew about languages to build language technology, especially people who knew about phonetics, acoustics, for speech technology. And that’s how I got into it. And then, you know, I just went from startups to companies and then Microsoft Research, 18 years ago, almost 18 years ago.
HUIZINGA: Wow. OK. I would love to actually talk to you about all that time. But we don’t have time because I have a lot more things to talk to you about, technology-wise. But I do want to know, you know, how would you describe the ideas behind your overarching research philosophy, and who are your influences, as they say in the rock-and-roll world? [LAUGHTER] Who inspired you? Real-life person, scientist or not, besides, HAL 9000, who’s fictional, and any seminal papers that, sort of, got you interested in that along the way?
BALI: So since I was really into speech, Ken Stevens—who was a professor, who sadly is no longer with us anymore, at MIT—was a big influence. He, kind of, had this whole idea of how speech is produced. And, you know, the first time I was exposed to the whole idea of the mathematics behind the speech, and I think he influenced me a lot on the speech side of things. For the language side of things, you know, my professor in India Professor Anvita Abbi—you know, she’s a Padma Shri, like, she’s been awarded by the Indian government for her work in, you know, very obscure, endangered languages—you know, she kind of gave me a feel for what languages are, and why they are important, and why it’s important to save them and not let them die away.
HUIZINGA: Right.
BALI: So I think I would say both of them. But what really got me into wanting to work with Indian language technology in a big way was I was working in Belgium, I was working in London, and I saw the beginning of how technology is, kind of, you know, making things easier, exciting; there’s cool technology available for English, for French, for German … But in a country like India, it was more about giving access to people who have no access, right? It actually mattered, because here are people who may not be very literate and therefore not be able to use technology in the way we know it, but they can talk.
HUIZINGA: Right.
BALI: And they can speak, and they should be able to access technology by doing that.
HUIZINGA: Right. OK. So just real quickly, that was then. What have you seen change in that time, and how profoundly have the ideas evolved?
BALI: So just from pure methodology and what’s possible, you know, I have seen it all. When I started working in language technology, mainly for Indian languages, but even for other languages, it was all a rule-based system. So everybody had to create all these rules that then were, you know, responsible for building or like making that technology work. But then, just at that time, you know, all the statistical systems and methodologies came into being. So we had hidden Markov models, you know, doing their thing in speech, and it was all about a lot of data. But that data still had to be procured in a certain way, labeled, annotated. It was still a very long and resource-intensive process. Now, with generative AI, the thing that I am excited about is, we have a very powerful tool, right?
HUIZINGA: Mm-hmm.
BALI: And, yes, it requires a lot of data, but it can learn also; you know, we can fine-tune stuff on smaller datasets …
HUIZINGA: Yeah …
BALI: … to work for, you know, relevant things. So it’s not going to take me years and years and years to first procure the data, then have it tagged for part of speech … then, you know, have it tagged for sentiment, have it tagged for this, have it tagged for that, and then, only can I think of building anything.
HUIZINGA: Right.
BALI: So it just shortens that timeline so much, and it’s very exciting.
HUIZINGA: Right. As an ex-English teacher—which I don’t think there is such a thing as an ex-English teacher; you’re always silently correcting someone’s grammar! [LAUGHTER]—just what you said about tagging parts of speech as what they are, right? And that, I used to teach that. And then you start to think, how would you translate that for a machine? So fascinating. So, Kalika, you have said that your choice of career was accidental—and you’ve alluded to the, sort of, the fortuitous things that happened along the way—but that linguistics is one subject that goes from absolute science to absolute philosophy. Can you unpack that a little bit more and how this idea impacted your work in language technology?
BALI: Yeah. So, so if you think about it, you know, language has a physical aspect, right. We move our various speech organs in a certain way. Our ears are constructed in a certain way. There is a physics of it where, when I speak, there are sound waves, right, which are going into your ear, and that’s being interpreted. So, you know, if you think about that, that’s like an absolute science behind it, right? But then, when you come to the structure of language, you know, the syntax, like you’re an English teacher, so you know this really well, that you know, there’s semantics; there’s, you know, morphology, how our words form, how our sentences form. And that’s like a very abstract kind of method that allows us to put, you know, meaningful sentences out there, right?
HUIZINGA: Right …
BALI: But then there’s this other part of how language works in society, right. The way I talk to my mother would be probably very different to the way I’m talking to you, would be very different from the way I talk to my friends, at a very basic level, right? The way, in India, I would greet someone older to me would be very different from the way I would greet somebody here, because here it’s like much less formal and that, you know, age hierarchy is probably less? If I did the same thing in India, I would be considered the rudest creature ever. [LAUGHS] So … and then, you know, you go into the whole philosophy—psycholinguistics part. What happens in our brains, you know, when we are speaking? Because language is controlled by various parts of our brain, right. And then, you go to the pure philosophy part, like why? How does language even occur? Why do we name things the way we name things? You know, why do we have a language of thought? You know, what language are we thinking in? [LAUGHTER]
HUIZINGA: Right.
BALI: So, so it really does cover the entire gamut of language …
HUIZINGA: Yeah, yeah, yeah …
BALI: … like from science to philosophy.
HUIZINGA: Yeah, as I said before, when we were talking out there, my mother-in-law was from Holland, and every time she did math or adding, she would do it in Dutch, which—she’d be speaking in English and then she’d go over here and count in Dutch out loud. And it’s like, yeah, your brain switches back and forth. This is so exciting to me. I had no idea how much I would love this podcast! So, much of your research is centered on this big idea called “design thinking,” and it’s got a whole discipline in universities around the world. And you’ve talked about using something you call the 4D process for your work. Could you explain that process, and how it plays out in the research you do with the communities you serve?
BALI: Yeah, so we’ve kind of adapted this. My ex-colleague Monojit Choudhury and I, kind of, came up with this whole thing about 4D thinking, which is essentially discover, design, develop and deploy, right. And when we are working with, especially with, marginalized or low-resource-language communities, the very basic thing we have to do is discover, because we cannot go with, you know, our own ideas and perceptions about what is required. And I can give you a very good example of this, right. You know, most of us, as researchers and technologists, when we think of language technology, we are thinking about machine translation; we’re thinking about speech recognition; we are thinking about state-of-the-art technology. And here we were talking to a community that spoke the language Idu Mishmi, which is a very small community in northeast of India. And we were talking about, you know, we can do this, we can do that. And they just turned to us and said, what we really want is a mobile digital dictionary! [LAUGHS]
HUIZINGA: Wow. Yeah …
BALI: Right? And, you know, if you don’t talk, if you don’t observe, if you are not open to what the community’s needs might be, then you’ll miss that, right. You’ll miss the real thing that will make a difference to that community. So that’s the discover part. The design part, again, you have to design with the community. You cannot go and design a system that they are unable to use properly, right. And again, another very good example, one of the people I know, you know, he gave me this very good example of why you have to think, even at the architecture level when you’re designing such things, is like a lot of applications in India and around the world require your telephone number for verification. Now, for women, it might be a safety issue. They might not want to give their telephone number. Or in India, many women might not even have a telephone, like a mobile number, right. So how do you think of other ways in which they can verify, right? And so that’s the design part. The develop and the deploy part, kind of, go hand in hand, because I think it’s a very iterative process. You develop quickly, you put it out there, allow it to fail and, you know …
HUIZINGA: Mm-hmm. Iterate …
BALI: Iterate. So that’s like the, kind of, design thinking that we have.
HUIZINGA: Yeah, I see that happening in accessibility technology areas, too, as well as language …
BALI: Yeah, and, you know, working with the communities, very quickly, you become really humble.
HUIZINGA: Sure.
BALI: There’s a lot of humility in me now. Though I have progressed in my career and, you know, supposedly become wiser, I am much more humble about what I know and what I can do than I was when I started off, you know.
HUIZINGA: I love that. Well, one thing I want to talk to you about that has intrigued me, there’s a thing that happens in India where you mix languages …
BALI: Yes!
HUIZINGA: You speak both Hindi and English at the same time, and you think, oh, you speak English, but it’s like, no, there’s words I don’t understand in that. What do you call that, and how did that drive your interest? I mean, that was kind of an early-on kind of thing in your work, right? Talk about that.
BALI: So that’s called code-mixing or code-switching. The only linguistic difference is code-mixing happens within a sentence, and code-switching means one sentence in one language and another.
HUIZINGA: Oh, really?
BALI: Yeah. So … but this is, like, not just India. This is a very, very common feature of multilingual societies all over the world. So it’s not multilingual individuals, but at the societal level, when you have multilingualism, then, you know, this is a marker of multilingualism. But code-mixing particularly means that you have to be fluent in both languages to actually code-mix, right. You have to have a certain amount of fluency in both languages. And there are various reasons why people do this. You know, it’s been studied by psychologists and linguists for a long time. And for most people like me, multilingual people, that’s the language we dream in, we think about. [LAUGHTER] That’s the language we talk to our siblings and friends in, right. And for us, it’s, like, just natural. We just keep …
HUIZINGA: Mixing …
BALI: … flipping between the two languages for a variety of reasons. We might do it for emphasis; we might do it for humor. We might just decide, OK, I’m going to pick this from this … the brain decides I’m going to pick this from this language …
HUIZINGA: Sure.
BALI: … and this … So the reason we got interested in, like, looking into code-mixing was that when we are saying that we want humans to be able to interact with machines in their most natural language, then by some estimates, half the world speaks like this!
HUIZINGA: Right.
BALI: So we have to be able to understand exactly how they speak and, you know, be able to process and understand their language, which is code-mixed …
HUIZINGA: Sure. Well, it seems like the human brain can pick this up and process it fairly quickly and easily, especially if it knows many languages. For a machine, it would be much more difficult?
BALI: It is. So initially, it was really difficult because, you know, the way we created systems was one language at a time …
HUIZINGA: Right!
BALI: … right. And it’s not about having an English engine and a Hindi engine available. It doesn’t work that way.
HUIZINGA: No!
BALI: So you’d really need something that, you know, is able to tackle the languages together. And in some theories, this is almost considered a language of its own because it’s not like you’re randomly mixing. There is a structure to …
HUIZINGA: Oh, is there?
BALI: Yeah. Where you can, where you can’t …
HUIZINGA: Gotcha.
BALI: You know, so there is a structure or grammar, you can say, of code-mixing. So we went after that. We, kind of, created tools which could generate grammatically viable code-mixed sentences given parallel data, etc.
HUIZINGA: That’s awesome. Amazing.
BALI: So, yeah, it takes effort to do it. But again, right now, because the generative AI models have at their disposal, you know, so many languages and at least, like, theoretically can work in many, many, many languages, you know, code-mixing might be an easier problem to solve right now.
HUIZINGA: Right. OK. So we’re talking mostly about widely used languages, and you’re very concerned right now on this idea of low-resource languages. So unpack what you mean by low-resource, and what’s missing from the communities that speak those languages?
BALI: Yeah. So when we say low-resource languages, we typically mean that languages do not have, say, digital resources, linguistic resources, language resources, that would enable technology building. It doesn’t mean that the communities themselves are impoverished in culture or linguistic richness, etc., right. But the reason why these communities do not have a lot of language resources, linguistic resources, digital resources, most of the time, it is because they are also marginalized in other ways … social and economic marginalization.
HUIZINGA: Right.
BALI: And these are … if you look at them, they’re not ti—I mean, of course, some of them are tiny, but when we say low-resource communities, we are talking about really big numbers.
HUIZINGA: Oh, really?
BALI: Yeah. So one of the languages that I have worked with—language communities that I’ve worked with—speak a language called Gondi, which is like a Dravidian language that is spoken in … like a South Indian language that is spoken in north, central-north area. It’s a tribal language, and it’s got around three million speakers.
HUIZINGA: Oh, wow!
BALI: Yeah. That’s like more than Welsh, …
HUIZINGA: Yeah! [LAUGHS]
BALI: … right? But because socio-politically, they have been—or economically, they have been marginalized, they do not have the resources to build technologies. And, you know, when we say empower everyone and we only empower the top tier, I don’t think we fulfill our ambition to empower everyone. And like I said earlier, for these communities, all the technology that we have, digital tools that we have access to, they really matter for them. So, for example, you know, a lot of government schemes or the forest reserve laws are provided, say, in Hindi. If they are provided in Gondi, these people have a real idea of what they can do.
HUIZINGA: Yeah. … Sure.
BALI: Similarly, for education, you know, there are books and books and books in Hindi. There’s no book available for Gondi. So how is the next generation even going to learn the language?
HUIZINGA: Right.
BALI: And there are many, many languages which are low resource. In fact, you know, we did a study sometime in 2020, I think, we published this paper on linguistic diversity, and there we saw that, you know, we divided languages in five categories, and the top most which have all the resources to build every possible technology have only five languages, right. And more than half of the world’s languages are at the bottom. So it is a big problem.
HUIZINGA: Yeah. Let’s talk about some of the specific technologies you’re working on. And I want to go from platform to project because you’ve got a big idea in a platform you call VeLLM. Talk about that.
BALI: So VeLLM, which actually means jaggery—the sweet, sugary jaggery—in Tamil, one of the languages in India …
HUIZINGA: Let me, let me interject that it’s not vellum like the paper, or what you’re talking about. It’s capital V, little e, and then LLM, which stands for large language model?
BALI: So universal, the “V” comes from there. Empowerment, “e” comes from there. Through large language models …
HUIZINGA: Got it. OK. But you shortened it to VeLLM.
BALI: Yeah.
HUIZINGA: OK.
BALI: So, so the thing with VeLLM is that a bunch of us got together just when this whole GPT was released, etc. We have a very strong group that works on technologies for empowerment in the India lab, Microsoft Research India. And we got together to see what it is that we can do now that we have access to such a strong and powerful tool. And we started thinking of the work that we’ve been doing, which is to, you know, build these technologies for specific areas and specific languages, specific demographies. So we, kind of, put all that knowledge and all that experience we had and thought of like, how can we scale that, really, across everything that we do? So VeLLM, at its base, you know, takes a GPT-like LLM, you know, as a horizontal across everything. On top of it, we have again, horizontals of machine learning, of multilingual tools and processes, which allow us to take the outputs from, say, GPT-like things and adapt it to different languages or, you know, some different kind of domain, etc. And then we have verticals on top of it, which allow people to build specific applications.
HUIZINGA: Let me just go back and say GPT … I think most of our audience will know that that stands for generative pretrained transformer models. But just so we have that for anyone who doesn’t know, let’s anchor that. So VeLLM basically was an enabling platform …
BALI: Yes.
HUIZINGA: … on which to build specific technologies that would solve problems in a vertical application.
BALI: Yes. Yes. And because it’s a platform, we’re also working on tools that are needed across domains …
HUIZINGA: Oh, interesting.
BALI: … as well as tools that are needed for specific domains.
HUIZINGA: OK, so let’s talk about some of the specifics because we could get into the weeds on the tools that everybody needs, but I like the ideas that you’re working on and the specific needs that you’re meeting, the felt-need thing that gets an idea going. So talk about this project that you’ve worked on called Kahani. Could you explain what that is, and how it works? It’s really interesting to me.
BALI: So Kahani, actually, is about storytelling, culturally appropriate storytelling, with spectacular images, as well as like textual story.
HUIZINGA: So visual storytelling?
BALI: Visual storytelling with the text. So this actually started when my colleague Sameer Segal, he was trying to use generative AI to create stories for his daughter, and he discovered that, you know, things are not very culturally appropriate! So I’ll give an example that, you know, if you want to take Frozen and take it to, like, the south Indian state of Kerala, you’ll have the beaches of Kerala, you’ll have even have the coconut trees, but then you will have this blond princess in a princess gown …
HUIZINGA: Sure …
BALI: … who’s there, right? So that’s where we started discussing this, and we, kind of, started talking about, how can we create visuals that are anchored on text of a story that’s culturally appropriate? So when we’re talking about, say, Little Red Riding Hood, if we ask the generative AI model, OK, that I want the story of Little Red Riding Hood but in an Indian context, it does a fantastic job. It actually gives you a very nice story, which, you know, just reflects the Red Riding Hood story into an Indian context. But the images don’t really …
HUIZINGA: Match … [LAUGHTER]
BALI: … Match at all. So that’s where the whole Kahani thing started. And we did a hackathon project on it. And then a lot of people got interested. It’s an ongoing project, so I won’t say that it’s out there yet, but we are very excited about it, but because think of it, we can actually create stories for children, you know, which is what we started with, but we can create so much more media, so much more culturally appropriate storytelling, which is not necessarily targeted at children.
HUIZINGA: Yeah, yeah.
BALI: So that’s what Kahani is about.
HUIZINGA: OK. And I saw a demo of it that your colleague did for Research Forum here, and there was an image of a girl—it was beautiful—and then there was a mask of some kind or a … what was that?
BALI: So the mask is called Nazar Battu, which is actually, you have these masks which are supposed to drive away the evil eye. So that’s what the mask was about. It’s a very Indian thing. You know, when you build a nice house, you put one on top of it so that the envious glances are, like, kept at bay. So, yeah, so that’s what it was.
HUIZINGA: And was there some issue of the generative AI not really understanding what that was?
BALI: No, it didn’t understand what it was.
HUIZINGA: So then can you fix that and make it more culturally aware?
BALI: So that’s what we are trying to do for the image thing. So we have another project on culture awareness where we are looking at understanding how much generative AI knows about other cultures.
HUIZINGA: Interesting.
BALI: So that’s a simultaneous project that’s happening. But in Kahani, a lot of it is, like, trying to get reference images, you know …
HUIZINGA: Yeah. … Into the system?
BALI: Into the system …
HUIZINGA: Gotcha …
BALI: … and trying to anchor on that.
HUIZINGA: Mmmm. So—and we’re not going to talk about that project, I don’t think—but … how do you assess whether an AI knows? By just asking it? By prompting and seeing what happens?
BALI: Yeah, yeah, yeah. So in another project, what we did was, we asked humans to play a game to get cultural artifacts from them. The problem with asking humans what cultural artifacts are important to them is we don’t think of like things as culture, right. [LAUGHS] This is food!
HUIZINGA: It’s just who we are!
BALI: This is my food. Like, you know, it’s not a culturally important artifact. This is how I greet my parents. It’s not like culturally …
HUIZINGA: So it’s just like fish swimming in water. You don’t see the water.
BALI: Exactly. So we gamified this thing, and we were able to get certain cultural artifacts, and we tried to get generative AI models to tell us about the same artifacts. And it didn’t do too well … [LAUGHS]
HUIZINGA: But that’s why it’s research!
BALI: Yes!
HUIZINGA: You try, you iterate, you try again … cool. As I mentioned earlier, I was a high school English teacher and an English major. I’m not correcting your grammar because it’s fantastic.
BALI: Thank you.
HUIZINGA: But as a former educator, one of the projects I felt was really compelling that you’re working on is called Shiksha. It’s a copilot in education. Tell our audience about this.
BALI: So this is actually our proof of concept for the VeLLM platform. Since almost all of us were interested in education, we decided to go for education as the first use case that we’re going to work on. And actually, it was a considered decision to go target teachers instead of students. I mean, you must have seen a lot of work being done on taking generative AI to students, right. But we feel that, you know, teachers are necessary to teach because they’re not just giving you information about the subject. They’re giving you skills to learn, which hopefully will stay with you for a lifetime, right. And if we enable teachers, they will enable so many hundreds of students. One teacher can enable thousands of students, right, over her career. So instead of, like, going and targeting students, if we make it possible for teachers to do their jobs more effectively or, like, you know, help them get over the problems they have, then we are actually creating an ecosystem where things will scale really fast, really quickly. And in India, you know, this is especially true because the government has actually come up with some digital resources for teachers to use, but there’s a lot more that can be done. So we interviewed about a hundred-plus teachers across different parts of the country. And this is the, you know, discover part.
HUIZINGA: Yeah!
BALI: And we found out that lesson plans are a big headache! [LAUGHS]
HUIZINGA: Yes, they are! Can confirm!
BALI: Yeah. And they spend a lot of time doing lesson plans because they’re required to create a lesson plan for every class they teach …
HUIZINGA: Sure. With learning outcomes …
BALI: Exactly.
HUIZINGA: All of it.
BALI: All of it. So that’s where we, you know, zeroed in on—how to make it easier for teachers to create lesson plans. And that’s what the Shiksha project is about. You know, there is an enrollment process where the teachers say what subject they’re teaching, what classes they’re teaching, what boards, because there are different boards of education …
HUIZINGA: Right …
BALI: … which have different syllabus. So all that. But after that, it takes less than seven minutes for a teacher to create an entire lesson plan for a particular topic. You know, class assignments, class activities, home assignments, homework—everything! Like the whole thing in seven minutes! And these teachers have the ability to go and correct it. Like, it’s an interactive thing. So, you know, they might say, I think this activity is too difficult for my students.
HUIZINGA: Yeah …
BALI: Can I have, like, an easier one? Or, can I change this to this? So it allows them to interactively personalize, modify the plan that’s put out. And I find that really exciting. And we’ve tested this with the Sikshana Foundation, which works with teachers in India. We’ve tested this with them. The teachers are very excited and now Sikshana wants to scale it to other schools.
HUIZINGA: Right … well, my first question is, where were you when I was teaching, Kalika?
BALI: There was no generative AI!
HUIZINGA: No. In fact, we just discovered the fax machine when I started teaching. Oh, that dates me! You know, back to what you said about teachers being instrumental in the lives of their students. You know, we can remember our favorite teachers, our best teachers. We don’t remember a machine.
BALI: No.
HUIZINGA: And what you’ve done with this is to embody the absolute sort of pinnacle of what AI can do, which is to be the collaborator, the assistant, the augmenter, and the helper so that the teacher can do that inspirational, connective-tissue job with the students without having to, like, sacrifice the rest of their life making lesson plans and grading papers. Oh, my gosh. OK. On the positive side, we’ve just talked about what this work proposes and how it’s good, but I always like to dig a little bit into the potential unintended consequences and what could possibly go wrong if, in fact, you got everything right. So I’ll anchor this in another example. When GPT models first came out, the first reaction came from educators. It feels like we’re in a bit of a paradigm shift like we were when the calculator and the internet even came out. [It’s] like, how do we process this? So I want to go philosophically here and talk about how you foresee us adopting and moving forward with generative AI in education, writ large.
BALI: Yeah, I think this is a question that troubles a lot of us and not just in education, but in all spheres that generative AI is …
HUIZINGA: Art …
BALI: … art …
HUIZINGA: … writing …
BALI: … writing …
HUIZINGA: … journalism …
BALI: Absolutely. And I think the way I, kind of, think about it in my head is it’s a tool. At the end of it, it is a tool. It’s a very powerful tool, but it is a tool, and humans must always have the agency over it. And we need to come up, as a society, you know, we need to come up with the norms of using the tool. And if you think about it, you know, internet, taking internet as an example, there is a lot of harm that internet has propagated, right. The darknet and all the other stuff that happens, right. But on the whole, there are regulations, but there are also an actual consensus around what constitutes the positive use of internet, right.
HUIZINGA: Sure, yeah.
BALI: Nobody says that, for example, deepfakes are …
HUIZINGA: Mm-hmm. Good …
BALI: … good, right. So we have to come from there and think about what kind of regulations we need to have in place, what kind of consensus we need to have in place, what’s missing.
HUIZINGA: Right. Another project that has been around, and it isn’t necessarily on top of VeLLM, but it’s called Karya, and you call it a social impact organization that serves not just one purpose, but three. Talk about that.
BALI: Oh, Karya is my favorite! [LAUGHS] So Karya started as a research project within Microsoft Research India, and this was the brainchild again of my colleague—I have like some of the most amazing colleagues, too, that I work with!—called Vivek Seshadri. And Vivek wanted to create, you know, digital work for people who do not have access to such work. So he wanted to go to the rural communities, to people who belong to slightly lower socioeconomic demographies, and provide work, like microtasks kind of work, gig work, to them. And he was doing this, and then we started talking, and I said, you know, we need so much data for all these languages and all these different tasks, and that could be, like, a really cool thing to try on Karya, and that’s where it all started, my involvement with Karya, which is still pretty strong. And Karya then became such a stable project that Microsoft Research India spun it out. So it’s now its own standalone startup right now like a social enterprise, and they work on providing digital work. They work on providing skills, like upskilling. They work on awareness, like, you know, making people aware of certain social, financial, other such trainings. So what’s been most amazing is that Karya has been able to essentially collect data for AI in the most ethical way possible. They pay their workers a little over the minimal wage. They also have something called data ownership practice, where the data that is created by, say, me, I have some sort of ownership on it. So what that means is that every time Karya sells a dataset, a royalty comes back …
HUIZINGA: No … !
BALI: Yeah! To the workers.
HUIZINGA: OK, we need to scale this out! [LAUGHS] OK. So to give a concrete example, the three purposes would be educational, financial—on their end—and data collection, which would ultimately support a low-resource language by having digital assets.
BALI: Absolutely!
HUIZINGA: So you could give somebody something to read in their language …
BALI: Yeah.
HUIZINGA: … that would educate them in the process. They would get paid to do it, and then you would have this data.
BALI: Yes!
HUIZINGA: OK. So cool. So simple.
BALI: Like I said, it’s my favorite project.
HUIZINGA: I get that. I totally get that.
BALI: And they … they’ve been, you know, they have been winning awards and things all over for the work that they’re doing right now. And I am very involved in one project with them, which is to do with gender-intentional AI, or gender-intentional datasets for AI, for Indian languages. And that’s really crucial because, you know, we talk about gender bias in datasets, etc., but all that understanding comes from a very Western perspective and for languages like English, etc. They do not translate very well to Indian languages.
HUIZINGA: Right.
BALI: And in this particular project, we’re looking at first, how to define gender bias. How do we even get data around gender bias? What does it even mean to say that technology is gender intentional?
HUIZINGA: Right. All right, well, let’s talk a little bit about what I like to call outrageous ideas. And these are the ones that, you know, on the research spectrum from sort of really practical applied research to blue sky get dismissed or viewed as unrealistic or unattainable. So years ago—here’s a little story about you—when you told your tech colleagues that you wanted to work with the world’s most marginalized languages, they told you you’d only marginalize yourself.
BALI: Yes!
HUIZINGA: But you didn’t say no. You didn’t say no. Um, two questions. Did you feel like your own idea was outrageous back then? And do you still have anything outrageous yet to accomplish in this plan?
BALI: Oh, yeah! I hope so! Yeah. No, I do think, in some sense, the pushback that I got for my idea makes me think it was outrageous. I didn’t think it was outrageous at all at that time! [LAUGHS] I thought it was a very reasonable idea! But there was a very solid pushback and not just from your colleagues. You know, for researchers, publishing papers is important! No one would publish a paper which focused only on, say, Indian languages or low-resource languages. We’ve come a very long way even in the research community on that, right. We kept pushing, pushing, pushing! And now, there are tracks, there are workshops, there are conferences which are devoted to multilingual and low-resource languages. When I said I wanted to work on Hindi, and Hindi is the biggest language in India, right. And even for that, I was told, why don’t you work on German instead? And I’m like, there are lots of people working on German who will solve the problems with German! Nobody is looking at Hindi! I mean, people should work on all the languages. People should work on German, but I don’t want to work on German! So there was a lot of pushback back then, and I see a little bit of that with the very low-resource languages even now. And I think some people think it’s a “feel-good” thing, whereas I think it’s not. I think it’s a very economically viable, necessary thing to build technology for these communities, for these languages. No one thought Hindi was economically viable 15 years ago, for whatever reason …
HUIZINGA: That … that floors me …
BALI: Yeah, but, you know, we’re not talking about tens of thousands of people in some of these languages; we’re talking about millions.
HUIZINGA: Yeah.
BALI: I still think that is a job that I need to continue, you know, pushing back on.
HUIZINGA: Do you think that any of that sort of outrageous reaction was due to the fact that the technology wasn’t as advanced as it is now and that it might have changed in terms of what we can do?
BALI: There was definitely the aspect of technology there that it was just quite difficult and very, very resource-intensive to build it for languages which did not have resources. You know, there was a time when we were talking about how to go about doing this, and because people in various big tech companies, people did not really remember a time when, for English, they had to start data collection from scratch because everyone who was working on, say, English at that time was building on what people had done years and years ago. So they could not even conceptualize that you had to start from scratch for anything, right. But now with the technology as well, I’m quite optimistic and trying to think of how cool it would be to do, you know, smaller data collections and fine-tuned models specifically and things like that, so I think that the technology is definitely one big thing, but economics is a big factor, too.
HUIZINGA: Mmm-hmm. Well, I’m glad that you said it isn’t just the feel good, but it actually would make economic sense because that’s some of the driver behind what technologies get “greenlit,” as it were. Is there anything outrageous now that you could think of that, even to you, sounds like, oh, we could never do that …
BALI: Well … I didn’t think HAL was outrageous, so I’m not … [LAUGHS]
HUIZINGA: Back to HAL 9000! [LAUGHS]
BALI: Yeah, so I don’t think of things as outrageous or not. I just think of things as things that need to get done, if that makes any sense?
HUIZINGA: Totally. Maybe it’s, how do we override “Open the pod bay door, HAL”—“No, I’m sorry, Dave. I can’t do that”? [LAUGHS]
BALI: Yes. [LAUGHS] Yeah…
HUIZINGA: Well, as we close—and I’m sad to close because you are so much fun—I want to do a little vision casting, but in reverse. So let’s fast-forward 20 years and look back. How have the big ideas behind your life’s work impacted the world, and how are people better off or different now because of you and the teams that you’ve worked with?
BALI: So the way I see it is that people across the board, irrespective of the language they speak, the communities they belong to, the demographies they represent, can use technology to make their lives, their work, better. I know it sounds like really a very big and almost too good to be true, but that’s what I’m aiming for.
HUIZINGA: Well, Kalika Bali, I’m so grateful I got to talk to you in person. And thanks for taking time out from your busy trip from India to sit down with me and our audience and share your amazing ideas.
[MUSIC PLAYS]BALI: Thank you so much, Gretchen.
[MUSIC FADES]
The post Ideas: Language technologies for everyone with Kalika Bali appeared first on Microsoft Research.
Build an active learning pipeline for automatic annotation of images with AWS services
This blog post is co-written with Caroline Chung from Veoneer.
Veoneer is a global automotive electronics company and a world leader in automotive electronic safety systems. They offer best-in-class restraint control systems and have delivered over 1 billion electronic control units and crash sensors to car manufacturers globally. The company continues to build on a 70-year history of automotive safety development, specializing in cutting-edge hardware and systems that prevent traffic incidents and mitigate accidents.
Automotive in-cabin sensing (ICS) is an emerging space that uses a combination of several types of sensors such as cameras and radar, and artificial intelligence (AI) and machine learning (ML) based algorithms for enhancing safety and improving riding experience. Building such a system can be a complex task. Developers have to manually annotate large volumes of images for training and testing purposes. This is very time consuming and resource intensive. The turnaround time for such a task is several weeks. Furthermore, companies have to deal with issues such as inconsistent labels due to human errors.
AWS is focused on helping you increase your development speed and lower your costs for building such systems through advanced analytics like ML. Our vision is to use ML for automated annotation, enabling retraining of safety models, and ensuring consistent and reliable performance metrics. In this post, we share how, by collaborating with Amazon’s Worldwide Specialist Organization and the Generative AI Innovation Center, we developed an active learning pipeline for in-cabin image head bounding boxes and key points annotation. The solution reduces cost by over 90%, accelerates the annotation process from weeks to hours in terms of the turnaround time, and enables reusability for similar ML data labeling tasks.
Solution overview
Active learning is an ML approach that involves an iterative process of selecting and annotating the most informative data to train a model. Given a small set of labeled data and a large set of unlabeled data, active learning improves model performance, reduces labeling effort, and integrates human expertise for robust results. In this post, we build an active learning pipeline for image annotations with AWS services.
The following diagram demonstrates the overall framework for our active learning pipeline. The labeling pipeline takes images from an Amazon Simple Storage Service (Amazon S3) bucket and outputs annotated images with the cooperation of ML models and human expertise. The training pipeline preprocesses data and uses them to train ML models. The initial model is set up and trained on a small set of manually labeled data, and will be used in the labeling pipeline. The labeling pipeline and training pipeline can be iterated gradually with more labeled data to enhance the model’s performance.
In the labeling pipeline, an Amazon S3 Event Notification is invoked when a new batch of images comes into the Unlabeled Datastore S3 bucket, activating the labeling pipeline. The model produces the inference results on the new images. A customized judgement function selects parts of the data based on the inference confidence score or other user-defined functions. This data, with its inference results, is sent for a human labeling job on Amazon SageMaker Ground Truth created by the pipeline. The human labeling process helps annotate the data, and the modified results are combined with the remaining auto annotated data, which can be used later by the training pipeline.
Model retraining happens in the training pipeline, where we use the dataset containing the human-labeled data to retrain the model. A manifest file is produced to describe where the files are stored, and the same initial model is retrained on the new data. After retraining, the new model replaces the initial model, and the next iteration of the active learning pipeline starts.
Model deployment
Both the labeling pipeline and training pipeline are deployed on AWS CodePipeline. AWS CodeBuild instances are used for implementation, which is flexible and fast for a small amount of data. When speed is needed, we use Amazon SageMaker endpoints based on the GPU instance to allocate more resources to support and accelerate the process.
The model retraining pipeline can be invoked when there is new dataset or when the model’s performance needs improvement. One critical task in the retraining pipeline is to have the version control system for both the training data and the model. Although AWS services such as Amazon Rekognition have the integrated version control feature, which makes the pipeline straightforward to implement, customized models require metadata logging or additional version control tools.
The entire workflow is implemented using the AWS Cloud Development Kit (AWS CDK) to create necessary AWS components, including the following:
- Two roles for CodePipeline and SageMaker jobs
- Two CodePipeline jobs, which orchestrate the workflow
- Two S3 buckets for the code artifacts of the pipelines
- One S3 bucket for labeling the job manifest, datasets, and models
- Preprocessing and postprocessing AWS Lambda functions for the SageMaker Ground Truth labeling jobs
The AWS CDK stacks are highly modularized and reusable across different tasks. The training, inference code, and SageMaker Ground Truth template can be replaced for any similar active learning scenarios.
Model training
Model training includes two tasks: head bounding box annotation and human key points annotation. We introduce them both in this section.
Head bounding box annotation
Head bounding box annotation is a task to predict the location of a bounding box of the human head in an image. We use an Amazon Rekognition Custom Labels model for head bounding box annotations. The following sample notebook provides a step-by-step tutorial on how to train a Rekognition Custom Labels model via SageMaker.
We first need to prepare the data to start the training. We generate a manifest file for the training and a manifest file for the test dataset. A manifest file contains multiple items, each of which is for an image. The following is an example of the manifest file, which includes the image path, size, and annotation information:
Using the manifest files, we can load datasets to a Rekognition Custom Labels model for training and testing. We iterated the model with different amounts of training data and tested it on the same 239 unseen images. In this test, the mAP_50
score increased from 0.33 with 114 training images to 0.95 with 957 training images. The following screenshot shows the performance metrics of the final Rekognition Custom Labels model, which yields great performance in terms of F1 score, precision, and recall.
We further tested the model on a withheld dataset that has 1,128 images. The model consistently predicts accurate bounding box predictions on the unseen data, yielding a high mAP_50
of 94.9%. The following example shows an auto-annotated image with a head bounding box.
Key points annotation
Key points annotation produces locations of key points, including eyes, ears, nose, mouth, neck, shoulders, elbows, wrists, hips, and ankles. In addition to the location prediction, visibility of each point is needed to predict in this specific task, for which we design a novel method.
For key points annotation, we use a Yolo 8 Pose model on SageMaker as the initial model. We first prepare the data for training, including generating label files and a configuration .yaml file following Yolo’s requirements. After preparing the data, we train the model and save artifacts, including the model weights file. With the trained model weights file, we can annotate the new images.
In the training stage, all the labeled points with locations, including visible points and occluded points, are used for training. Therefore, this model by default provides the location and confidence of the prediction. In the following figure, a large confidence threshold (main threshold) near 0.6 is capable of dividing the points that are visible or occluded versus outside of camera’s viewpoints. However, occluded points and visible points are not separated by the confidence, which means the predicted confidence is not useful for predicting the visibility.
To get the prediction of visibility, we introduce an additional model trained on the dataset containing only visible points, excluding both occluded points and outside of camera’s viewpoints. The following figure shows the distribution of points with different visibility. Visible points and other points can be separated in the additional model. We can use a threshold (additional threshold) near 0.6 to get the visible points. By combining these two models, we design a method to predict the location and visibility.
A key point is first predicted by the main model with location and main confidence, then we get the additional confidence prediction from the additional model. Its visibility is then classified as follows:
- Visible, if its main confidence is greater than its main threshold, and its additional confidence is greater than the additional threshold
- Occluded, if its main confidence is greater than its main threshold, and its additional confidence is less than or equal to the additional threshold
- Outside of camera’s review, if otherwise
An example of key points annotation is demonstrated in the following image, where solid marks are visible points and hollow marks are occluded points. Outside of the camera’s review points are not shown.
Based on the standard OKS definition on the MS-COCO dataset, our method is able to achieve mAP_50 of 98.4% on the unseen test dataset. In terms of visibility, the method yields a 79.2% classification accuracy on the same dataset.
Human labeling and retraining
Although the models achieve great performance on test data, there are still possibilities for making mistakes on new real-world data. Human labeling is the process to correct these mistakes for enhancing model performance using retraining. We designed a judgement function that combined the confidence value that output from the ML models for the output of all head bounding box or key points. We use the final score to identify these mistakes and the resultant bad labeled images, which need to be sent to the human labeling process.
In addition to bad labeled images, a small portion of images are randomly chosen for human labeling. These human-labeled images are added into the current version of the training set for retraining, enhancing model performance and overall annotation accuracy.
In the implementation, we use SageMaker Ground Truth for the human labeling process. SageMaker Ground Truth provides a user-friendly and intuitive UI for data labeling. The following screenshot demonstrates a SageMaker Ground Truth labeling job for head bounding box annotation.
The following screenshot demonstrates a SageMaker Ground Truth labeling job for key points annotation.
Cost, speed, and reusability
Cost and speed are the key advantages of using our solution compared to human labeling, as shown in the following tables. We use these tables to represent the cost savings and speed accelerations. Using the accelerated GPU SageMaker instance ml.g4dn.xlarge, the whole life training and inference cost on 100,000 images is 99% less than the cost of human labeling, while the speed is 10–10,000 times faster than the human labeling, depending on the task.
The first table summarizes the cost performance metrics.
Model | mAP_50 based on 1,128 test images | Training cost based on 100,000 images | Inference cost based on 100,000 images | Cost reduction compared to human annotation | Inference time based on 100,000 images | Time acceleration compared to human annotation |
Rekognition head bounding box | 0.949 | $4 | $22 | 99% less | 5.5 h | Days |
Yolo Key points | 0.984 | $27.20 | * $10 | 99.9% less | minutes | Weeks |
The following table summarizes performance metrics.
Annotation Task | mAP_50 (%) | Training Cost ($) | Inference Cost ($) | Inference Time |
Head Bounding Box | 94.9 | 4 | 22 | 5.5 hours |
Key Points | 98.4 | 27 | 10 | 5 minutes |
Moreover, our solution provides reusability for similar tasks. Camera perception developments for other systems like advanced driver assist system (ADAS) and in-cabin systems can also adopt our solution.
Summary
In this post, we showed how to build an active learning pipeline for automatic annotation of in-cabin images utilizing AWS services. We demonstrate the power of ML, which enables you to automate and expedite the annotation process, and the flexibility of the framework that uses models either supported by AWS services or customized on SageMaker. With Amazon S3, SageMaker, Lambda, and SageMaker Ground Truth, you can streamline data storage, annotation, training, and deployment, and achieve reusability while reducing costs significantly. By implementing this solution, automotive companies can become more agile and cost-efficient by using ML-based advanced analytics such as automated image annotation.
Get started today and unlock the power of AWS services and machine learning for your automotive in-cabin sensing use cases!
About the Authors
Yanxiang Yu is an Applied Scientist at at the Amazon Generative AI Innovation Center. With over 9 years of experience building AI and machine learning solutions for industrial applications, he specializes in generative AI, computer vision, and time series modeling.
Tianyi Mao is an Applied Scientist at AWS based out of Chicago area. He has 5+ years of experience in building machine learning and deep learning solutions and focuses on computer vision and reinforcement learning with human feedbacks. He enjoys working with customers to understand their challenges and solve them by creating innovative solutions using AWS services.
Yanru Xiao is an Applied Scientist at the Amazon Generative AI Innovation Center, where he builds AI/ML solutions for customers’ real-world business problems. He has worked in several fields, including manufacturing, energy, and agriculture. Yanru obtained his Ph.D. in Computer Science from Old Dominion University.
Paul George is an accomplished product leader with over 15 years of experience in automotive technologies. He is adept at leading product management, strategy, Go-to-Market and systems engineering teams. He has incubated and launched several new sensing and perception products globally. At AWS, he is leading strategy and go-to-market for autonomous vehicle workloads.
Caroline Chung is an engineering manager at Veoneer (acquired by Magna International), she has over 14 years of experience developing sensing and perception systems. She currently leads interior sensing pre-development programs at Magna International managing a team of compute vision engineers and data scientists.
Combating Corruption With Data: Cleanlab and Berkeley Research Group on Using AI-Powered Investigative Analytics
Talk about scrubbing data. Curtis Northcutt, cofounder and CEO of Cleanlab, and Steven Gawthorpe, senior data scientist at Berkeley Research Group, speak about Cleanlab’s groundbreaking approach to data curation with Noah Kravitz, host of NVIDIA’s AI Podcast, in an episode recorded live at the NVIDIA GTC global AI conference. The startup’s tools enhance data reliability and trustworthiness through sophisticated error identification and correction algorithms. Northcutt and Gawthorpe provide insights into how AI-powered data analytics can help combat economic crimes and corruption and discuss the intersection of AI, data science and ethical governance in fostering a more just society.
Cleanlab is a member of the NVIDIA Inception program for cutting-edge startups.
Stay tuned for more episodes recorded live from GTC.
Time Stamps
1:05: Northcutt on Cleanlab’s inception and mission
2:41: What Cleanlab offers its customers
4:24: The human element in Cleanlab’s data verification
8:57: Gawthorpe on the core functions, aims of the Berkeley Research Group
10:42: Gawthorpe’s approach to data collection and analysis in fraud investigations
16:38: Cleanlab’s one-click solution for generating machine learning models
18:30: The evolution of machine learning and its impact on data analytics
20:07: Future directions in data-driven crimefighting
You Might Also Like…
The Case for Generative AI in the Legal Field – Ep. 210
Thomson Reuters, the global content and technology company, is transforming the legal industry with generative AI. In the latest episode of NVIDIA’s AI Podcast, host Noah Kravitz spoke with Thomson Reuters’ Chief Product Officer David Wong about its potential — and implications.
Making Machines Mindful: NYU Professor Talks Responsible AI – Ep. 205
Artificial intelligence is now a household term. Responsible AI is hot on its heels. Julia Stoyanovich, associate professor of computer science and engineering at NYU and director of the university’s Center for Responsible AI, wants to make the terms “AI” and “responsible AI” synonymous.
MLCommons’ David Kanter, NVIDIA’s Daniel Galvez on Publicly Accessible Datasets – Ep. 167
On this episode of NVIDIA’s AI Podcast, host Noah Kravitz spoke with David Kanter, founder and executive director of MLCommons, and NVIDIA senior AI developer technology engineer Daniel Galvez, about the democratization of access to speech technology and how ML Commons is helping advance the research and development of machine learning for everyone.
Metaspectral’s Migel Tissera on AI-Based Data Management – Ep. 155
Cofounder and CTO of Metaspectral speaks with NVIDIA AI Podcast host Noah Kravitz about how Metaspectral’s technologies help space explorers make quicker and better use of the massive amounts of image data they collect out in the cosmos.
Subscribe to the AI Podcast
Get the AI Podcast through iTunes, Google Podcasts, Google Play, Amazon Music, Castbox, DoggCatcher, Overcast, PlayerFM, Pocket Casts, Podbay, PodBean, PodCruncher, PodKicker, Soundcloud, Spotify, Stitcher and TuneIn.
Make the AI Podcast better: Have a few minutes to spare? Fill out this listener survey.
The Building Blocks of AI: Decoding the Role and Significance of Foundation Models
Editor’s note: This post is part of the AI Decoded series, which demystifies AI by making the technology more accessible, and which showcases new hardware, software, tools and accelerations for RTX PC users.
Skyscrapers start with strong foundations. The same goes for apps powered by AI.
A foundation model is an AI neural network trained on immense amounts of raw data, generally with unsupervised learning.
It’s a type of artificial intelligence model trained to understand and generate human-like language. Imagine giving a computer a huge library of books to read and learn from, so it can understand the context and meaning behind words and sentences, just like a human does.
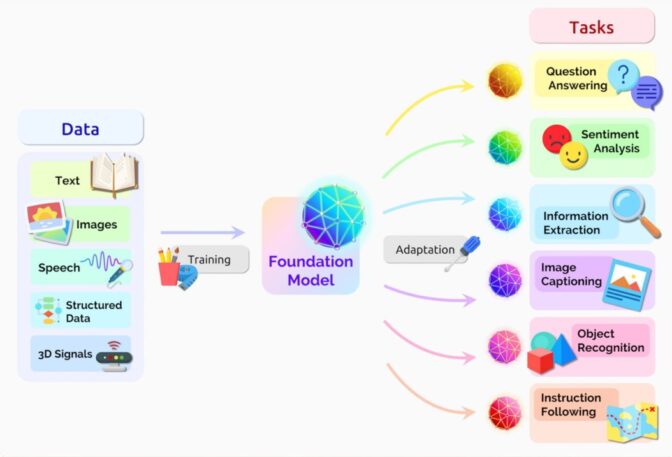
A foundation model’s deep knowledge base and ability to communicate in natural language make it useful for a broad range of applications, including text generation and summarization, copilot production and computer code analysis, image and video creation, and audio transcription and speech synthesis.
ChatGPT, one of the most notable generative AI applications, is a chatbot built with OpenAI’s GPT foundation model. Now in its fourth version, GPT-4 is a large multimodal model that can ingest text or images and generate text or image responses.
Online apps built on foundation models typically access the models from a data center. But many of these models, and the applications they power, can now run locally on PCs and workstations with NVIDIA GeForce and NVIDIA RTX GPUs.
Foundation Model Uses
Foundation models can perform a variety of functions, including:
- Language processing: understanding and generating text
- Code generation: analyzing and debugging computer code in many programming languages
- Visual processing: analyzing and generating images
- Speech: generating text to speech and transcribing speech to text
They can be used as is or with further refinement. Rather than training an entirely new AI model for each generative AI application — a costly and time-consuming endeavor — users commonly fine-tune foundation models for specialized use cases.
Pretrained foundation models are remarkably capable, thanks to prompts and data-retrieval techniques like retrieval-augmented generation, or RAG. Foundation models also excel at transfer learning, which means they can be trained to perform a second task related to their original purpose.
For example, a general-purpose large language model (LLM) designed to converse with humans can be further trained to act as a customer service chatbot capable of answering inquiries using a corporate knowledge base.
Enterprises across industries are fine-tuning foundation models to get the best performance from their AI applications.
Types of Foundation Models
More than 100 foundation models are in use — a number that continues to grow. LLMs and image generators are the two most popular types of foundation models. And many of them are free for anyone to try — on any hardware — in the NVIDIA API Catalog.
LLMs are models that understand natural language and can respond to queries. Google’s Gemma is one example; it excels at text comprehension, transformation and code generation. When asked about the astronomer Cornelius Gemma, it shared that his “contributions to celestial navigation and astronomy significantly impacted scientific progress.” It also provided information on his key achievements, legacy and other facts.
Extending the collaboration of the Gemma models, accelerated with the NVIDIA TensorRT-LLM on RTX GPUs, Google’s CodeGemma brings powerful yet lightweight coding capabilities to the community. CodeGemma models are available as 7B and 2B pretrained variants that specialize in code completion and code generation tasks.
MistralAI’s Mistral LLM can follow instructions, complete requests and generate creative text. In fact, it helped brainstorm the headline for this blog, including the requirement that it use a variation of the series’ name “AI Decoded,” and it assisted in writing the definition of a foundation model.
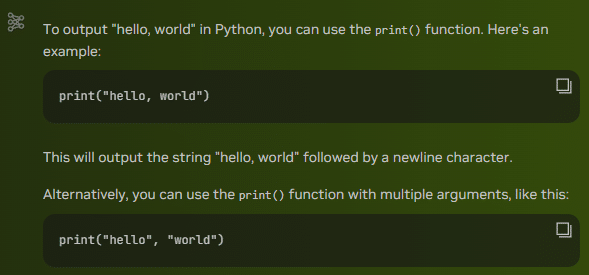
Meta’s Llama 2 is a cutting-edge LLM that generates text and code in response to prompts.
Mistral and Llama 2 are available in the NVIDIA ChatRTX tech demo, running on RTX PCs and workstations. ChatRTX lets users personalize these foundation models by connecting them to personal content — such as documents, doctors’ notes and other data — through RAG. It’s accelerated by TensorRT-LLM for quick, contextually relevant answers. And because it runs locally, results are fast and secure.
Image generators like StabilityAI’s Stable Diffusion XL and SDXL Turbo let users generate images and stunning, realistic visuals. StabilityAI’s video generator, Stable Video Diffusion, uses a generative diffusion model to synthesize video sequences with a single image as a conditioning frame.
Multimodal foundation models can simultaneously process more than one type of data — such as text and images — to generate more sophisticated outputs.
A multimodal model that works with both text and images could let users upload an image and ask questions about it. These types of models are quickly working their way into real-world applications like customer service, where they can serve as faster, more user-friendly versions of traditional manuals.
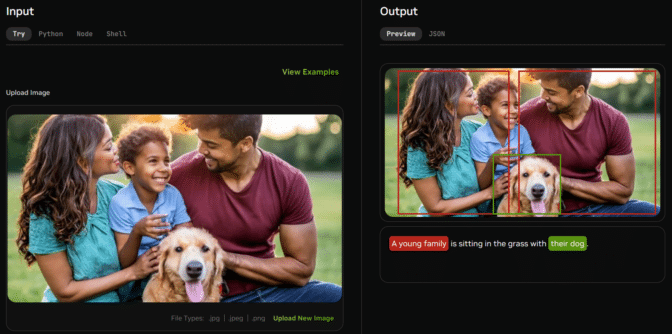
Kosmos 2 is Microsoft’s groundbreaking multimodal model designed to understand and reason about visual elements in images.
Think Globally, Run AI Models Locally
GeForce RTX and NVIDIA RTX GPUs can run foundation models locally.
The results are fast and secure. Rather than relying on cloud-based services, users can harness apps like ChatRTX to process sensitive data on their local PC without sharing the data with a third party or needing an internet connection.
Users can choose from a rapidly growing catalog of open foundation models to download and run on their own hardware. This lowers costs compared with using cloud-based apps and APIs, and it eliminates latency and network connectivity issues. Generative AI is transforming gaming, videoconferencing and interactive experiences of all kinds. Make sense of what’s new and what’s next by subscribing to the AI Decoded newsletter.
NVIDIA Joins $110 Million Partnership to Help Universities Teach AI Skills
The Biden Administration has announced a new $110 million AI partnership between Japan and the United States that includes an initiative to fund research through a collaboration between the University of Washington and the University of Tsukuba.
NVIDIA is committing $25 million in a collaboration with Amazon that aims to bring the latest technologies to the University of Washington, in Seattle, and the University of Tsukuba, which is northeast of Tokyo.
Universities around the world are preparing students for crucial AI skills by providing access to the high performance computing capabilities of supercomputing.
“This collaboration between the University of Washington, University of Tsukuba, Amazon, and NVIDIA will help provide the research and workforce training for our regions’ tech sectors to keep up with the profound impacts AI is having across every sector of our economy,” said Jay Inslee, governor of Washington State.
Creating AI Opportunities for Students
NVIDIA has been investing in universities for decades computing resources, advanced training curriculums, donations, and other support to provide students and professors with access to high performance computing (HPC) for groundbreaking research results.
NVIDIA founder and CEO Jensen Huang and his wife, Lori Huang, donated $50 million to their alma mater Oregon State University — where they met and earned engineering degrees — to help build one of the world’s fastest supercomputers in a facility bearing their names. This computing center will help students research, develop and apply AI across Oregon State’s top-ranked programs in agriculture, computer sciences, climate science, forestry, oceanography, robotics, water resources, materials sciences and more.
The University of Florida recently unveiled Malachowsky Hall, which was made possible with a $50 million donation from NVIDIA co-founder Chris Malachowsky. This new building along with a previous donation of an AI supercomputer is enabling the University of Florida to offer world class AI training and research opportunities.
Strengthening US-Japan AI Research Collaboration
The U.S.-Japan HPC alliance will advance AI research and development and support the two nations’ global leadership in cutting-edge technology.
The University of Washington and Tsukuba University initiative will support research in critical areas where AI can drive impactful change, such as robotics, healthcare, climate change and atmospheric science, among others.
In addition to the university partnership, NVIDIA recently announced a collaboration with Japan’s National Institute of Advanced Industrial Science and Technology (AIST) on AI and quantum technology.
Addressing Worldwide AI Talent Shortage
Demand for key AI skills is creating a talent shortage worldwide. Some experts calculate there has been a fivefold increase in demand for these skills as a percentage of total U.S. jobs. Universities around the world are looking for ways to prepare students with new skills for the workforce, and corporate-university partnerships are a key tool to help bridge the gap.
NVIDIA unveiled at GTC 2024 new professional certifications in generative AI to help enable the next generation of developers to obtain technical credibility in this important domain.
Learn more about NVIDIA generative AI courses here and here.
Knowledge Bases for Amazon Bedrock now supports custom prompts for the RetrieveAndGenerate API and configuration of the maximum number of retrieved results
With Knowledge Bases for Amazon Bedrock, you can securely connect foundation models (FMs) in Amazon Bedrock to your company data for Retrieval Augmented Generation (RAG). Access to additional data helps the model generate more relevant, context-specific, and accurate responses without retraining the FMs.
In this post, we discuss two new features of Knowledge Bases for Amazon Bedrock specific to the RetrieveAndGenerate
API: configuring the maximum number of results and creating custom prompts with a knowledge base prompt template. You can now choose these as query options alongside the search type.
Overview and benefits of new features
The maximum number of results option gives you control over the number of search results to be retrieved from the vector store and passed to the FM for generating the answer. This allows you to customize the amount of background information provided for generation, thereby giving more context for complex questions or less for simpler questions. It allows you to fetch up to 100 results. This option helps improve the likelihood of relevant context, thereby improving the accuracy and reducing the hallucination of the generated response.
The custom knowledge base prompt template allows you to replace the default prompt template with your own to customize the prompt that’s sent to the model for response generation. This allows you to customize the tone, output format, and behavior of the FM when it responds to a user’s question. With this option, you can fine-tune terminology to better match your industry or domain (such as healthcare or legal). Additionally, you can add custom instructions and examples tailored to your specific workflows.
In the following sections, we explain how you can use these features with either the AWS Management Console or SDK.
Prerequisites
To follow along with these examples, you need to have an existing knowledge base. For instructions to create one, see Create a knowledge base.
Configure the maximum number of results using the console
To use the maximum number of results option using the console, complete the following steps:
- On the Amazon Bedrock console, choose Knowledge bases in the left navigation pane.
- Select the knowledge base you created.
- Choose Test knowledge base.
- Choose the configuration icon.
- Choose Sync data source before you start testing your knowledge base.
- Under Configurations, for Search Type, select a search type based on your use case.
For this post, we use hybrid search because it combines semantic and text search to provider greater accuracy. To learn more about hybrid search, see Knowledge Bases for Amazon Bedrock now supports hybrid search.
- Expand Maximum number of source chunks and set your maximum number of results.
To demonstrate the value of the new feature, we show examples of how you can increase the accuracy of the generated response. We used Amazon 10K document for 2023 as the source data for creating the knowledge base. We use the following query for experimentation: “In what year did Amazon’s annual revenue increase from $245B to $434B?”
The correct response for this query is “Amazon’s annual revenue increased from $245B in 2019 to $434B in 2022,” based on the documents in the knowledge base. We used Claude v2 as the FM to generate the final response based on the contextual information retrieved from the knowledge base. Claude 3 Sonnet and Claude 3 Haiku are also supported as the generation FMs.
We ran another query to demonstrate the comparison of retrieval with different configurations. We used the same input query (“In what year did Amazon’s annual revenue increase from $245B to $434B?”) and set the maximum number of results to 5.
As shown in the following screenshot, the generated response was “Sorry, I am unable to assist you with this request.”
Next, we set the maximum results to 12 and ask the same question. The generated response is “Amazon’s annual revenue increase from $245B in 2019 to $434B in 2022.”
As shown in this example, we are able to retrieve the correct answer based on the number of retrieved results. If you want to learn more about the source attribution that constitutes the final output, choose Show source details to validate the generated answer based on the knowledge base.
Customize a knowledge base prompt template using the console
You can also customize the default prompt with your own prompt based on the use case. To do so on the console, complete the following steps:
- Repeat the steps in the previous section to start testing your knowledge base.
- Enable Generate responses.
- Select the model of your choice for response generation.
We use the Claude v2 model as an example in this post. The Claude 3 Sonnet and Haiku model is also available for generation.
- Choose Apply to proceed.
After you choose the model, a new section called Knowledge base prompt template appears under Configurations.
- Choose Edit to start customizing the prompt.
- Adjust the prompt template to customize how you want to use the retrieved results and generate content.
For this post, we gave a few examples for creating a “Financial Advisor AI system” using Amazon financial reports with custom prompts. For best practices on prompt engineering, refer to Prompt engineering guidelines.
We now customize the default prompt template in several different ways, and observe the responses.
Let’s first try a query with the default prompt. We ask “What was the Amazon’s revenue in 2019 and 2021?” The following shows our results.
From the output, we find that it’s generating the free-form response based on the retrieved knowledge. The citations are also listed for reference.
Let’s say we want to give extra instructions on how to format the generated response, like standardizing it as JSON. We can add these instructions as a separate step after retrieving the information, as part of the prompt template:
The final response has the required structure.
By customizing the prompt, you can also change the language of the generated response. In the following example, we instruct the model to provide an answer in Spanish.
After removing $output_format_instructions$
from the default prompt, the citation from the generated response is removed.
In the following sections, we explain how you can use these features with the SDK.
Configure the maximum number of results using the SDK
To change the maximum number of results with the SDK, use the following syntax. For this example, the query is “In what year did Amazon’s annual revenue increase from $245B to $434B?” The correct response is “Amazon’s annual revenue increase from $245B in 2019 to $434B in 2022.”
The ‘numberOfResults
’ option under ‘retrievalConfiguration
’ allows you to select the number of results you want to retrieve. The output of the RetrieveAndGenerate
API includes the generated response, source attribution, and the retrieved text chunks.
The following are the results for different values of ‘numberOfResults
’ parameters. First, we set numberOfResults = 5
.
Then we set numberOfResults = 12
.
Customize the knowledge base prompt template using the SDK
To customize the prompt using the SDK, we use the following query with different prompt templates. For this example, the query is “What was the Amazon’s revenue in 2019 and 2021?”
The following is the default prompt template:
The following is the customized prompt template:
With the default prompt template, we get the following response:
If you want to provide additional instructions around the output format of the response generation, like standardizing the response in a specific format (like JSON), you can customize the existing prompt by providing more guidance. With our custom prompt template, we get the following response.
The ‘promptTemplate
‘ option in ‘generationConfiguration
‘ allows you to customize the prompt for better control over answer generation.
Conclusion
In this post, we introduced two new features in Knowledge Bases for Amazon Bedrock: adjusting the maximum number of search results and customizing the default prompt template for the RetrieveAndGenerate
API. We demonstrated how to configure these features on the console and via SDK to improve performance and accuracy of the generated response. Increasing the maximum results provides more comprehensive information, whereas customizing the prompt template allows you to fine-tune instructions for the foundation model to better align with specific use cases. These enhancements offer greater flexibility and control, enabling you to deliver tailored experiences for RAG-based applications.
For additional resources to start implementing in your AWS environment, refer to the following:
- User guide: Knowledge bases for Amazon Bedrock
- YouTube video: Use RAG to improve responses in generative AI application
- GitHub repo code samples: Amazon Bedrock Knowledge Base – Samples for building RAG workflows
About the authors
Sandeep Singh is a Senior Generative AI Data Scientist at Amazon Web Services, helping businesses innovate with generative AI. He specializes in Generative AI, Artificial Intelligence, Machine Learning, and System Design. He is passionate about developing state-of-the-art AI/ML-powered solutions to solve complex business problems for diverse industries, optimizing efficiency and scalability.
Suyin Wang is an AI/ML Specialist Solutions Architect at AWS. She has an interdisciplinary education background in Machine Learning, Financial Information Service and Economics, along with years of experience in building Data Science and Machine Learning applications that solved real-world business problems. She enjoys helping customers identify the right business questions and building the right AI/ML solutions. In her spare time, she loves singing and cooking.
Sherry Ding is a senior artificial intelligence (AI) and machine learning (ML) specialist solutions architect at Amazon Web Services (AWS). She has extensive experience in machine learning with a PhD degree in computer science. She mainly works with public sector customers on various AI/ML related business challenges, helping them accelerate their machine learning journey on the AWS Cloud. When not helping customers, she enjoys outdoor activities.
The science behind Echo Frames
How the team behind Echo Frames delivered longer battery life and improved sound quality inside the slim form factor of a pair of eyeglasses.Read More
Faster Dynamically Quantized Inference with XNNPack
Posted by Alan Kelly, Software Engineer
We are excited to announce that XNNPack’s Fully Connected and Convolution 2D operators now support dynamic range quantization. XNNPack is TensorFlow Lite’s CPU backend and CPUs deliver the widest reach for ML inference and remain the default target for TensorFlow Lite. Consequently, improving CPU inference performance is a top priority. We quadrupled inference performance in TensorFlow Lite’s XNNPack backend compared to the single precision baseline by adding support for dynamic range quantization to the Fully Connected and Convolution operators. This means that more AI powered features may be deployed to older and lower tier devices.
Previously, XNNPack offered users the choice between either full integer quantization, where the weights and activations are stored as signed 8-bit integers, or half-precision (fp16) or single-precision (fp32) floating-point inference. In this article we demonstrate the benefits of dynamic range quantization.
Dynamic Range Quantization
Dynamically quantized models are similar to fully-quantized models in that the weights for the Fully Connected and Convolution operators are quantized to 8-bit integers during model conversion. All other tensors are not quantized, they remain as float32 tensors. During model inference, the floating-point layer activations are converted to 8-bit integers before being passed to the Fully Connected and Convolution operators. The quantization parameters (the zero point and scale) for each row of the activation tensor are calculated dynamically based on the observed range of activations. This maximizes the accuracy of the quantization process as the activations make full use of the 8 quantized bits. In fully-quantized models, these parameters are fixed during model conversion, based on the range of the activation values observed using a representative dataset. The second difference between full quantization and dynamic range quantization is that the output of the Fully Connected and Convolution operators is in 32-bit floating-point format, as opposed to 8-bit integer for fully-quantized operators. With dynamic range quantization, we get most of the performance gains of full quantization, yet with higher overall accuracy.
Traditionally the inference of such models was done using TensorFlow Lite’s native operators. Now dynamically quantized models can benefit from XNNPack’s highly-optimized per-architecture implementations of the Fully Connected and Convolution2D operators. These operators are optimized for all architectures supported by XNNPack (ARM, ARM64, x86 SSE/AVX/AVX512 and WebAssembly), including the latest ArmV9 processors such as the Pixel 8’s Tensor G3 CPU or the One Plus 11’s SnapDragon 8 Gen 2 CPU.
How can you use it?
Two steps are required to use dynamic range quantization. You must first convert your model from TensorFlow with support for dynamic range quantization. Existing models already converted using dynamic range quantization do not need to be reconverted. Dynamic range quantization can be enabled during model conversion by enabling the
converter.optimizations = [tf.lite.Optimize.DEFAULT]
converter flag. Unlike full integer quantization, no representative dataset is required and unsupported operators do not prevent conversion from succeeding. Dynamic range quantization is therefore far more accessible to non-expert users than full integer quantization.
From TensorFlow 2.17, dynamically quantized XNNPack inference will be enabled by default in prebuilt binaries. If you want to use it sooner, the nightly TensorFlow builds may be used.
Mixed Precision Inference
In our previous article we presented the impressive performance gains from using half precision inference. Half-precision and dynamic range quantization may now be combined within XNNPack to get the best possible on-device CPU inference performance on devices which have hardware fp16 support (most phones on sale today do). The Fully Connected and Convolution 2D operators can output fp16 data instead of fp32. The Pixel 3, released in 2018, was the first Pixel model with fp16 support. fp16 uses half as many bits to store a floating-point value compared to fp32, meaning that the relative accuracy of each value is reduced due to the significantly shorter mantissa (10 vs 23 bits). Not all models support fp16 inference, but if a model supports it, the computational cost of vectorized floating-point operators can be reduced by half as the CPU can process twice as much data per instruction. Dynamically quantized models with compute-intensive floating point operators, such as Batch Matrix Multiply and Softmax, can benefit from fp16 inference as well.
Performance Improvements
Below, we present benchmarks on four public models covering common computer vision tasks:
- EfficientNetV2 – image classification and feature extraction
- Inception-v3 – image classification
- Deeplab-v3 – semantic segmentation
- Stable Diffusion – image generation (diffusion model)
Each model was converted three times where possible: full float, full 8 bit signed integer quantization and dynamic range quantization. Stable Diffusion’s diffusion model could not be converted using full integer quantization due to unsupported operators. The speed-up versus the original float32 model using TFLite’s kernels is shown below.
- FP32 refers to the baseline float32 model.
- QS8 refers to full signed 8 bit integer quantization using XNNPack.
- QD8-F32 refers to dynamically quantized 8 bit integers with fp32 activations using XNNPack.
- QD8-F16 refers to dynamically quantized 8 bit integers with fp16 activations using XNNPack.
![]() |
The speed-up versus TFLite’s dynamically quantized Fully Connected and Convolution operators are shown below. By simply using the latest version of TensorFlow Lite, you can benefit from these speed-ups.
![]() |
We can clearly see that dynamic range quantization is competitive with, and in some cases can exceed, the performance of full integer quantization. Stable Diffusion’s diffusion model runs up to 6.2 times faster than the original float32 model! This is a game changer for on-device performance.
We would expect that full integer quantization would be faster than dynamic range quantization as all operations are calculated using integer arithmetic. Furthermore, dynamic range quantization has the additional overhead of converting the floating point activations to quantized 8 bit integers. Surprisingly, in two of the three models tested, this is not true. Profiling the models using TFLite’s profiler solves the mystery. These models are slower due to a combination of quantization artifacts — quantized arithmetic is more efficient when the ratio of the input and output scales fall within a certain range and missing op support in XNNPack. These quantization parameters are determined during model conversion based on a provided representative dataset. Since the ratio of the scales falls outside the optimal range, a less optimal path must be taken and performance suffers.
Finally, we demonstrate that model precision is mostly preserved when using mixed precision inference, we compare the image generated by the dynamically quantized Stable Diffusion model using fp32 activations with that generated using fp16 activations with the random number generator seeded with the same number to verify that using fp16 activations for the diffusion model does not impact the quality of the generated image.
![]() |
Image generated using fp16 inference (left) and fp32 inference (right) |
Both generated images are indeed lovely cats, corresponding to the given prompt. The images are indistinguishable which is a very strong indication that the diffusion model is suited to fp16 inference. Of course, as for all neural networks, any quantization strategy should be validated using a large validation dataset, and not just a single test.
Conclusions
Full integer quantization is hard, converting models is difficult, error prone and accuracy is not guaranteed. The representative dataset must be truly representative to minimize quantization errors. Dynamic range quantization offers a compromise between full integer quantization and fp32 inference: the models are of similar size to the fully-quantized model and performance gains are often similar and sometimes exceeds that of fully-quantized models. Using Stable Diffusion, we showed that dynamic range quantization and fp16 inference can be combined giving game changing performance improvements. XNNPack’s dynamic range quantization is now powering Gemini, Google Meet, and Chrome OS audio denoising and will launch in many other products this year. This same technology is now available to our open source users so try it for yourself using the models linked above and following the instructions in the How can I use it section!
Acknowledgements
We would like to thank Frank Barchard and Quentin Khan for contributions towards dynamic range quantization inference in TensorFlow Lite and XNNPack.