A novel loss function and a way to aggregate multimodal input data are key to dramatic improvements on some test data.Read More
Introducing the Frontier Safety Framework
Our approach to analyzing and mitigating future risks posed by advanced AI modelsRead More
New support for AI advancement in Central and Eastern Europe
Google invests $2 million in INSAIT, the Institute for Computer Science, Artificial Intelligence and Technology in Sofia, Bulgaria.Read More
How LotteON built a personalized recommendation system using Amazon SageMaker and MLOps
This post is co-written with HyeKyung Yang, Jieun Lim, and SeungBum Shim from LotteON.
LotteON aims to be a platform that not only sells products, but also provides a personalized recommendation experience tailored to your preferred lifestyle. LotteON operates various specialty stores, including fashion, beauty, luxury, and kids, and strives to provide a personalized shopping experience across all aspects of customers’ lifestyles.
To enhance the shopping experience of LotteON’s customers, the recommendation service development team is continuously improving the recommendation service to provide customers with the products they are looking for or may be interested in at the right time.
In this post, we share how LotteON improved their recommendation service using Amazon SageMaker and machine learning operations (MLOps).
Problem definition
Traditionally, the recommendation service was mainly provided by identifying the relationship between products and providing products that were highly relevant to the product selected by the customer. However, it was necessary to upgrade the recommendation service to analyze each customer’s taste and meet their needs. Therefore, we decided to introduce a deep learning-based recommendation algorithm that can identify not only linear relationships in the data, but also more complex relationships. For this reason, we built the MLOps architecture to manage the created models and provide real-time services.
Another requirement was to build a continuous integration and continuous delivery (CI/CD) pipeline that can be integrated with GitLab, a code repository used by existing recommendation platforms, to add newly developed recommendation models and create a structure that can continuously improve the quality of recommendation services through periodic retraining and redistribution of models.
In the following sections, we introduce the MLOps platform that we built to provide high-quality recommendations to our customers and the overall process of inferring a deep learning-based recommendation algorithm (Neural Collaborative Filtering) in real time and introducing it to LotteON.
Solution architecture
The following diagram illustrates the solution architecture for serving Neural Collaborative Filtering (NCF) algorithm-based recommendation models as MLOps. The main AWS services used are SageMaker, Amazon EMR, AWS CodeBuild, Amazon Simple Storage Service (Amazon S3), Amazon EventBridge, AWS Lambda, and Amazon API Gateway. We’ve combined several AWS services using Amazon SageMaker Pipelines and designed the architecture with the following components in mind:
- Data preprocessing
- Automated model training and deployment
- Real-time inference through model serving
- CI/CD structure
The preceding architecture shows the MLOps data flow, which consists of three decoupled passes:
- Code preparation and data preprocessing (blue)
- Training pipeline and model deployment (green)
- Real-time recommendation inference (brown)
Code preparation and data preprocessing
The preparation and preprocessing phase consists of the following steps:
- The data scientist publishes the deployment code containing the model and the training pipeline to GitLab, which is used by LotteON, and Jenkins uploads the code to Amazon S3.
- The EMR preprocessing batch runs through Airflow according to the specified schedule. The preprocessing data is loaded into MongoDB, which is used as a feature store along with Amazon S3.
Training pipeline and model deployment
The model training and deployment phase consists of the following steps:
- After the training data is uploaded to Amazon S3, CodeBuild runs based on the rules specified in EventBridge.
- The SageMaker pipeline predefined in CodeBuild runs, and sequentially runs steps such as preprocessing including provisioning, model training, and model registration.
- When training is complete (through the Lambda step), the deployed model is updated to the SageMaker endpoint.
Real-time recommendation inference
The inference phase consists of the following steps:
- The client application makes an inference request to the API gateway.
- The API gateway sends the request to Lambda, which makes an inference request to the model in the SageMaker endpoint to request a list of recommendations.
- Lambda receives the list of recommendations and provides them to the API gateway.
- The API gateway provides the list of recommendations to the client application using the Recommendation API.
Recommendation model using NCF
NCF is an algorithm based on a paper presented at the International World Wide Web Conference in 2017. It is an algorithm that covers the limitations of linear matrix factorization, which is often used in existing recommendation systems, with collaborative filtering based on the neural net. By adding non-linearity through the neural net, the authors were able to model a more complex relationship between users and items. The data for NCF is interaction data where users react to items, and the overall structure of the model is shown in the following figure (source: https://arxiv.org/abs/1708.05031).
Although NCF has a simple model architecture, it has shown a good performance, which is why we chose it to be the prototype for our MLOps platform. For more information about the model, refer to the paper Neural Collaborative Filtering.
In the following sections, we discuss how this solution helped us build the aforementioned MLOps components:
- Data preprocessing
- Automating model training and deployment
- Real-time inference through model serving
- CI/CD structure
MLOps component 1: Data preprocessing
For NCF, we used user-item interaction data, which requires significant resources to process the raw data collected at the application and transform it into a form suitable for learning. With Amazon EMR, which provides fully managed environments like Apache Hadoop and Spark, we were able to process data faster.
The data preprocessing batches were created by writing a shell script to run Amazon EMR through AWS Command Line Interface (AWS CLI) commands, which we registered to Airflow to run at specific intervals. When the preprocessing batch was complete, the training/test data needed for training was partitioned based on runtime and stored in Amazon S3. The following is an example of the AWS CLI command to run Amazon EMR:
MLOps component 2: Automated training and deployment of models
In this section, we discuss the components of the model training and deployment pipeline.
Event-based pipeline automation
After the preprocessing batch was complete and the training/test data was stored in Amazon S3, this event invoked CodeBuild and ran the training pipeline in SageMaker. In the process, the version of the result file of the preprocessing batch was recorded, enabling dynamic control of the version and management of the pipeline run history. We used EventBridge, Lambda, and CodeBuild to connect the data preprocessing steps run by Amazon EMR and the SageMaker learning pipeline on an event-based basis.
EventBridge is a serverless service that implements rules to receive events and direct them to destinations, based on the event patterns and destinations you establish. The initial role of EventBridge in our configuration was to invoke a Lambda function on the S3 object creation event when the preprocessing batch stored the training dataset in Amazon S3. The Lambda function dynamically modified the buildspec.yml file, which is indispensable when CodeBuild runs. These modifications encompassed the path, version, and partition information of the data that needed training, which is crucial for carrying out the training pipeline. The subsequent role of EventBridge was to dispatch events, instigated by the alteration of the buildspec.yml file, leading to running CodeBuild.
CodeBuild was responsible for building the source code where the SageMaker pipeline was defined. Throughout this process, it referred to the buildspec.yml file and ran processes such as cloning the source code and installing the libraries needed to build from the path defined in the file. The Project Build tab on the CodeBuild console allowed us to review the build’s success and failure history, along with a real-time log of the SageMaker pipeline’s performance.
SageMaker pipeline for training
SageMaker Pipelines helps you define the steps required for ML services, such as preprocessing, training, and deployment, using the SDK. Each step is visualized within SageMaker Studio, which is very helpful for managing models, and you can also manage the history of trained models and endpoints that can serve the models. You can also set up steps by attaching conditional statements to the results of the steps, so you can adopt only models with good retraining results or prepare for learning failures. Our pipeline contained the following high-level steps:
- Model training
- Model registration
- Model creation
- Model deployment
Each step is visualized in the pipeline in Amazon SageMaker Studio, and you can also see the results or progress of each step in real time, as shown in the following screenshot.
Let’s walk through the steps from model training to deployment, using some code examples.
Train the model
First, you define a PyTorch Estimator to use for training and a training step. This requires you to have the training code (for example, train.py) ready in advance and pass the location of the code as an argument of the source_dir
. The training step runs the training code you pass as an argument of the entry_point
. By default, the training is done by launching the container in the instance you specify, so you’ll need to pass in the path to the training Docker image for the training environment you’ve developed. However, if you specify the framework for your estimator here, you can pass in the version of the framework and Python version to use, and it will automatically fetch the version-appropriate container image from Amazon ECR.
When you’re done defining your PyTorch Estimator, you need to define the steps involved in training it. You can do this by passing the PyTorch Estimator you defined earlier as an argument and the location of the input data. When you pass in the location of the input data, the SageMaker training job will download the train and test data to a specific path in the container using the format /opt/ml/input/data/<channel_name>
(for example, /opt/ml/input/data/train
).
In addition, when defining a PyTorch Estimator, you can use metric definitions to monitor the learning metrics generated while the model is being trained with Amazon CloudWatch. You can also specify the path where the results of the model artifacts after training are stored by specifying estimator_output_path
, and you can use the parameters required for model training by specifying model_hyperparameters
. See the following code:
Create a model package group
The next step is to create a model package group to manage your trained models. By registering trained models in model packages, you can manage them by version, as shown in the following screenshot. This information allows you to reference previous versions of your models at any time. This process only needs to be done one time when you first train a model, and you can continue to add and update models as long as they declare the same group name.
See the following code:
Add a trained model to a model package group
The next step is to add a trained model to the model package group you created. In the following code, when you declare the Model class, you get the result of the previous model training step, which creates a dependency between the steps. A step with a declared dependency can only be run if the previous step succeeds. However, you can use the DependsOn option to declare a dependency between steps even if the data is not causally related.
After the trained model is registered in the model package group, you can use this information to manage and track future model versions, create a real-time SageMaker endpoint, run a batch transform job, and more.
Create a SageMaker model
To create a real-time endpoint, an endpoint configuration and model is required. To create a model, you need two basic elements: an S3 address where the model’s artifacts are stored, and the path to the inference Docker image that will run the model’s artifacts.
When creating a SageMaker model, you must pay attention to the following steps:
- Provide the result of the model training step, step_train.properties.ModelArtifacts.S3ModelArtifacts, which will be converted to the S3 path where the model artifact is stored, as an argument of the
model_data
. - Because you specified the PyTorchModel class,
framework_version
, andpy_version
, you use this information to get the path to the inference Docker image through Amazon ECR. This is the inference Docker image that is used for model deployment. Make sure to enter the same PyTorch framework, Python version, and other details that you used to train the model. This means keeping the same PyTorch and Python versions for training and inference. - Provide the inference.py as the entry point script to handle invocations.
This step will set a dependency on the model package registration step you defined via the DependsOn option.
Create a SageMaker endpoint
Now you need to define an endpoint configuration based on the created model, which will create an endpoint when deployed. Because the SageMaker Python SDK doesn’t support the step related to deployment (as of this writing), you can use Lambda to register that step. Pass the necessary arguments to Lambda, such as instance_type
, and use that information to create the endpoint configuration first. Because you’re calling the endpoint based on endpoint_name
, you need to make sure that variable is defined with a unique name. In the following Lambda function code, based on the endpoint_name
, you update the model if the endpoint exists, and deploy a new one if it doesn’t:
To get the Lambda function into a step in the SageMaker pipeline, you can use the SDK associated with the Lambda function. By passing the location of the Lambda function source as an argument of the function, you can automatically register and use the function. In conjunction with this, you can define LambdaStep and pass it the required arguments. See the following code:
Create a SageMaker pipeline
Now you can create a pipeline using the steps you defined. You can do this by defining a name for the pipeline and passing in the steps to be used in the pipeline as arguments. After that, you can run the defined pipeline through the start function. See the following code:
After this process is complete, an endpoint is created with the trained model and is ready for use based on the deep learning-based model.
MLOps component 3: Real-time inference with model serving
Now let’s see how to invoke the model in real time from the created endpoint, which can also be accessed using the SageMaker SDK. The following code is an example of getting real-time inference values for input values from an endpoint deployed via the invoke_endpoint
function. The features you pass as arguments to the body are passed as input to the endpoint, which returns the inference results in real time.
When we configured the inference function, we had it return the items in the order that the user is most likely to like among the items passed in. The preceding example returns items from 1–25 in order of likelihood of being liked by the user at index 0.
We added business logic to the feature, configured it in Lambda, and connected it with an API gateway to implement the API’s ability to return recommended items in real time. We then conducted performance testing of the online service. We load tested it with Locust using five g4dn.2xlarge instances and found that it could be reliably served in an environment with 1,000 TPS.
MLOps component 4: CI/CD structure
A CI/CD structure is a fundamental part of DevOps, and is also an important part of organizing an MLOps environment. AWS CodeCommit, AWS CodeBuild, AWS CodeDeploy, and AWS CodePipeline collectively provide all the functionality you need for CI/CD, from code shaping to deployment, build, and batch management. The services are not only linked to the same code series, but also to other services such as GitHub and Jenkins, so if you have an existing CI/CD structure, you can use them separately to fill in the gaps. Therefore, we expanded our CI/CD structure by linking only the CodeBuild configuration described earlier to our existing CI/CD pipeline.
We linked our SageMaker notebooks with GitLab for code management, and when we were done, we replicated them to Amazon S3 via Jenkins. After that, we set the S3 path to the default repository path of the NCF CodeBuild project as described earlier, so that we could build the project with CodeBuild.
Conclusion
So far, we’ve seen the end-to-end process of configuring an MLOps environment using AWS services and providing real-time inference services based on deep learning models. By configuring an MLOps environment, we’ve created a foundation for providing high-quality services based on various algorithms to our customers. We’ve also created an environment where we can quickly proceed with prototype development and deployment. The NCF we developed with the prototyping algorithm was also able to achieve good results when it was put into service. In the future, the MLOps platform can help us quickly develop and experiment with models that match LotteON data to provide our customers with a progressively higher-quality recommendation experience.
Using SageMaker in conjunction with various AWS services has given us many advantages in developing and operating our services. As model developers, we didn’t have to worry about configuring the environment settings for frequently used packages and deep learning-related frameworks because the environment settings were configured for each library, and we felt that the connectivity and scalability between AWS services using AWS CLI commands and related SDKs were great. Additionally, as a service operator, it was good to track and monitor the services we were running because CloudWatch connected the logging and monitoring of each service.
You can also check out the NCF and MLOps configuration for hands-on practice on our GitHub repo (Korean).
We hope this post will help you configure your MLOps environment and provide real-time services using AWS services.
About the Authors
SeungBum Shim is a data engineer in the Lotte E-commerce Recommendation Platform Development Team, responsible for discovering ways to use and improve recommendation-related products through LotteON data analysis, and developing MLOps pipelines and ML/DL recommendation models.
HyeKyung Yang is a research engineer in the Lotte E-commerce Recommendation Platform Development Team and is in charge of developing ML/DL recommendation models by analyzing and utilizing various data and developing a dynamic A/B test environment.
Jieun Lim is a data engineer in the Lotte E-commerce Recommendation Platform Development Team and is in charge of operating LotteON’s personalized recommendation system and developing personalized recommendation models and dynamic A/B test environments.
Jesam Kim is an AWS Solutions Architect and helps enterprise customers adopt and troubleshoot cloud technologies and provides architectural design and technical support to address their business needs and challenges, especially in AIML areas such as recommendation services and generative AI.
Gonsoo Moon is an AWS AI/ML Specialist Solutions Architect and provides AI/ML technical support. His main role is to collaborate with customers to solve their AI/ML problems based on various use cases and production experience in AI/ML.
8 new accessibility updates across Lookout, Google Maps and more
For Global Accessibility Awareness Day, here’s new updates to our accessibility products.Read More
Bringing Gemini to Google Workspace for Education
Gemini comes to Google Workspace for Education with generative AI and more data protection.Read More
Fight for Honor in ‘Men of War II’ on GFN Thursday
Whether looking for new adventures, epic storylines or games to play with a friend, GeForce NOW members are covered.
Start off with the much-anticipated sequel to the Men of War franchise or cozy up with some adorable pals in Palworld, both part of five games GeForce NOW is bringing to the cloud this week.
No Guts, No Glory
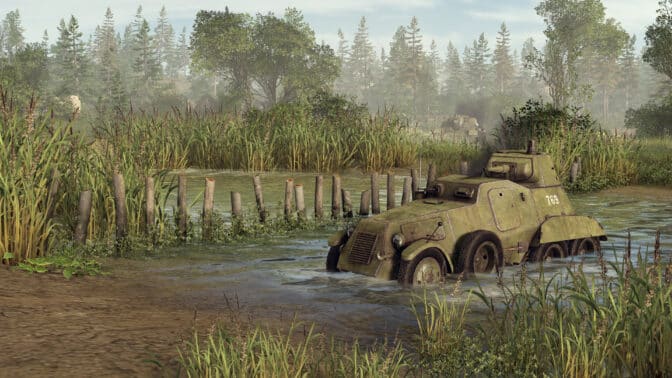
Get transported to the battlefields of World War II with historical accuracy and attention to detail in Men of War II, the newest entry in the real-time strategy series from Fulqrum Publishing.
The game features an extensive roster of units, including tanks, airplanes and infantry. With advanced enemy AI and diverse gameplay modes, Men of War II promises an immersive experience for both history enthusiasts and casual gamers.
Gear up, strategize and prepare to rewrite history. Get an extra fighting chance with a GeForce NOW Ultimate membership, which streams at up to 4K resolution and provides longer gaming sessions and faster access to games over a free membership.
Cloud Pals
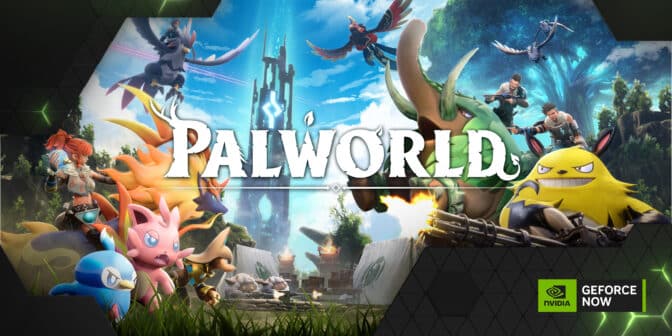
Step into a world teeming with enigmatic creatures known as “Pals” in the action-adventure survival game Palworld from Pocketpair. Navigate the wilderness, gather resources and construct a base to capture, tame and train Pals, each with distinct abilities. Explore the world, uncover secrets and forge alliances or rivalries with other survivors in online co-op play mode.
Embark on adventure with these trusty Pals through a GeForce NOW membership. With a Priority membership, enjoy up to six hours of uninterrupted gaming sessions, while Ultimate members can extend their playtime to eight hours.
Master New Games
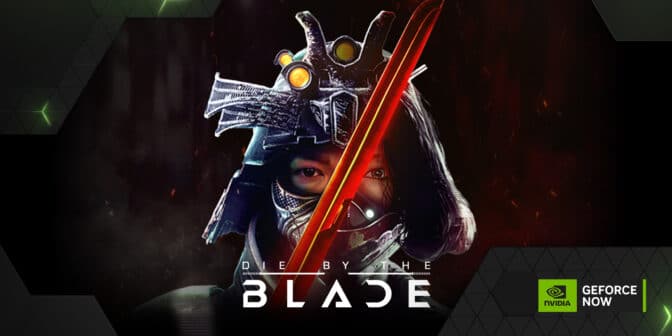
Vanquish foes with a single strike in 1v1 weapon-based fighter Die by the Blade from Grindstone. Dive into a samurai punk world and wield a range of traditional Japanese weapons. Take up arms and crush friends in local or online multiplayer, or take on unknown warriors in online ranked matches. Outwit opponents in intense, tactical battles and master the art of the one-hit kill.
Check out the list of new games this week:
- Men of War II (New release on Steam, May 15)
- Die by the Blade (New release on Steam, May 16)
- Colony Survival (Steam)
- Palworld (Steam)
- Tomb Raider: Definitive Edition (Xbox, available on PC Game Pass)
What are you planning to play this weekend? Let us know on X or in the comments below.
If you could be any video game character for a day, who would you choose and what would you do?
—
NVIDIA GeForce NOW (@NVIDIAGFN) May 15, 2024
What’s Your Story: Jacki O’Neill
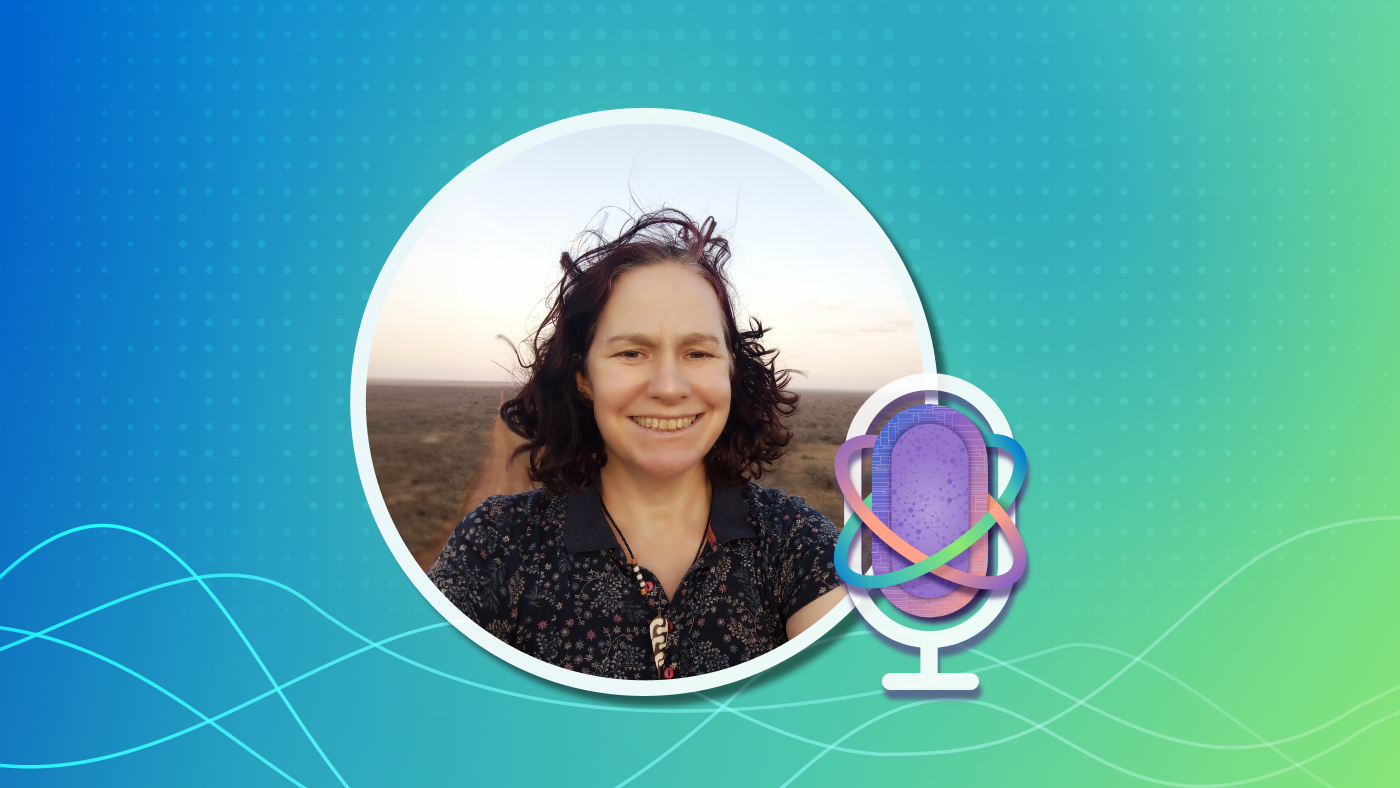
In the Microsoft Research Podcast series What’s Your Story, Johannes Gehrke explores the who behind the technical and scientific advancements helping to reshape the world. A systems expert whose 10 years with Microsoft spans research and product, Gehrke talks to members of the company’s research community about what motivates their work and how they got where they are today.
In this episode, Gehrke is joined by Jacki O’Neill, director of Microsoft Research Africa, Nairobi (formerly the Microsoft Africa Research Institute, or MARI) in Kenya. O’Neill pitched the idea for the lab after seeing an opportunity to expand the Microsoft research portfolio. She shares how a desire to build tech that can have global societal impact and a familial connection to the continent factored into the decision; how a belief that life is meant to be exciting has allowed her to take big personal and professional swings; and how her team in Nairobi is applying their respective expertise in human-computer interaction, machine learning, and data science to pursue globally equitable AI.
To learn more about the global impact of AI, efforts to make AI more equitable, and related topics, register for Microsoft Research Forum (opens in new tab), a series of panel discussions and lightning talks around science and technology research in the era of general AI.
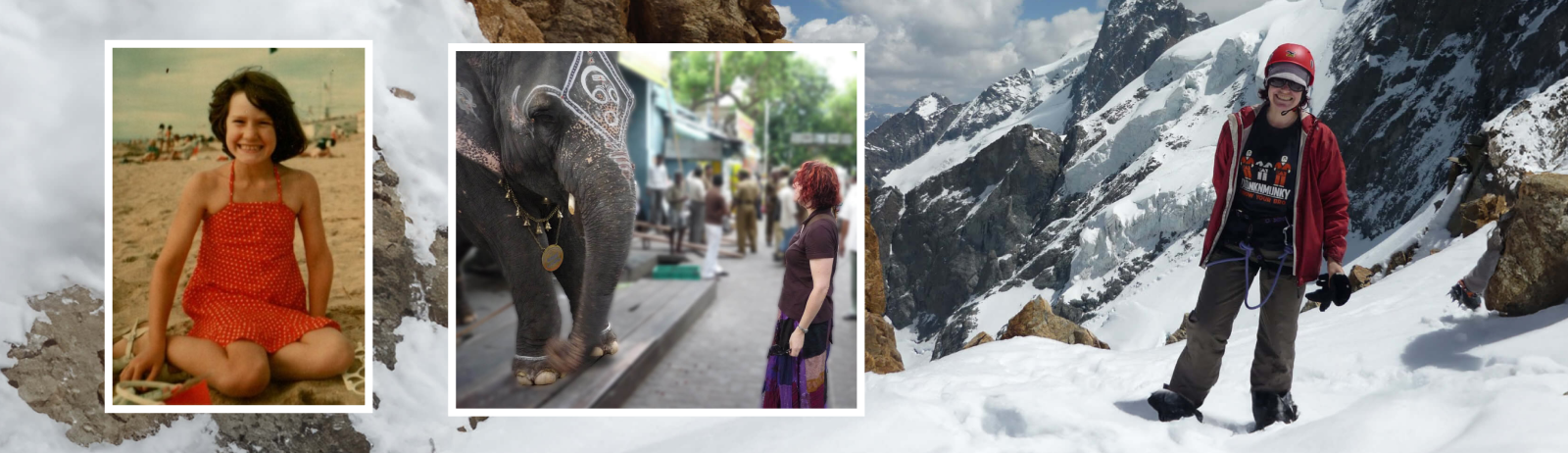
Learn more:
Subscribe to the Microsoft Research Podcast:
Editor’s note, May 16, 2024 – Since the recording of this podcast episode, the name of the Microsoft Africa Research Institute (MARI) has changed. The name of the lab is now Microsoft Research Africa, Nairobi.
Transcript
[TEASER] [MUSIC PLAYS UNDER DIALOGUE]JACKI O’NEILL: I love living in different places, and those experiences are what help us innovate better and design things that are, like, taking another point of view, more creative, I think. Just sparks things in your, in your head. And, I mean, it’s so much fun.
[TEASER ENDS]JOHANNES GEHRKE: Microsoft Research works at the cutting edge. But how much do we know about the people behind the science and technology that we create? This is What’s Your Story, and I’m Johannes Gehrke. In my 10 years with Microsoft, across product and research, I’ve been continuously excited and inspired by the people I work with, and I’m curious about how they became the talented and passionate people they are today. So I sat down with some of them. Now, I’m sharing their stories with you. In this podcast series, you’ll hear from them about how they grew up, the critical choices that shaped their lives, and their advice to others looking to carve a similar path.
[MUSIC FADES]In this episode, I’m talking with Jacki O’Neill, director of the Microsoft Africa Research Institute—or MARI, for short—in Nairobi, Kenya. Jacki’s decadelong career at Microsoft began at the company’s India research lab, where she applied her ethnographic and human-computer interaction expertise to advancing equity in the country.
After the opening of two Microsoft software engineering centers in Africa, Jacki made the case for a research lab on the continent. She now leads the MARI team in making technology more inclusive, a role that allows her to pursue her goal of positive local change with global impact. Here’s my conversation with Jacki, beginning with her time growing up in Plymouth, England.
GEHRKE: We just had a discussion maybe a couple of years ago, right, when you were just in transition to Africa. So it’s really great to have you here and both learn a little bit what’s happening there, but also to learn a bit more about your story. Where did you grow up, and how did you end up here at Microsoft?
O’NEILL: Yeah, thanks for asking that. I’ve had a very, well, it’s definitely not been a straight road to get here, but the windy roads are the most interesting ones. I grew up in Plymouth, which is a dockyard and naval town in the southwest of England, so a socially deprived working-class town. So when I was growing up, it was a thriving working-class town, but of course with those industries, you know, they didn’t, they didn’t pass so well through those years. So, you know, by the time I was leaving school, it was quite a deprived city and still is. I think that it’s really important to be in those type of places, though, because you get a very rich view of life, and I left them as soon as I could, [LAUGHS] so …
GEHRKE: When you went to university?
O’NEILL: Went to, well, I went and I was a cook for a year in the Lake District, which is a very beautiful part of the UK, and then went to university.
GEHRKE: Where’s the Lake District?
O’NEILL: It is northwest, and it’s all hills. It’s, like, Wordsworth Country. It’s all hills and poetry and beautiful houses. And, yeah, it was a fantastic time working as a cook there. And then I went to Manchester to do my degree.
GEHRKE: OK. And what is your degree in?
O’NEILL: Ah, so, yes, I had, I did a social science degree to start with. I started at the time when you could get a degree in anything and get any job at the end of it. But by the time I came out of my degree, it was a recession.
GEHRKE: But did you have, did you have specific plans while you were studying of what you want, you know, what profession you wanted to go into?
O’NEILL: Not really. I didn’t. I think I’d, I think like many young people, I didn’t really know, but I felt that I would find something interesting when I came out. And then, you know, I just worked lots of different jobs. [LAUGHS]
GEHRKE: What is your favorite college course?
O’NEILL: My favorite college course—in my degree? Gosh, that’s a good question. It was all so long ago. [LAUGHS]
GEHRKE: OK …
O’NEILL: My favorite, I guess, yeah, no, I, so, I did … my degree was in psychology. I worked, and then I did my master’s in computer science and then my PhD in human-computer interaction.
GEHRKE: That’s quite a change, right, from psychology into computer science, then.
O’NEILL: Yes, yes. And I just, you know, I’d always just wanted to do computing, but when I was at school, it was … we had one computer in the school, and so it was, like, a computer at home or you don’t do computer science. So, you know, I didn’t do it.
GEHRKE: Right.
O’NEILL: So then as computers became more prominent, more available, you know, I was working in libraries, and they started computerizing, and I worked on that project, and then that led me to do a master’s. And so I was like, hey, this is the opportunity to really get into this area, and I loved it. It was fantastic. And Manchester’s computer science department is one of the top departments, and I had an amazing … Carole Goble was my thesis supervisor. She was absolutely amazing and strong for women in computing. But at the end of it, I was like, OK, so I didn’t want to do pure social science and I didn’t want to do pure computer science. What I want to do is do human-computer science, so where you really merge the two. And that’s how I got into HCI, and I think that’s where I started finding my favorite courses. You know, I loved the research methods. I loved those types of things.
GEHRKE: And what is your PhD about?
O’NEILL: Ooh, it was very boring. [LAUGHTER] My PhD was in computer-supported cooperative work [CSCW], and …
GEHRKE: OK. Oh, yeah. Very relevant now, right?
O’NEILL: Yeah, very relevant now. And that was a really exciting time for CSCW, as well, because there were so many different labs. There were Sun Systems, there was Xerox, there was Microsoft—all doing really cool, like, collaborative technologies. So it seemed like a brilliant area to go into. But I was looking at, can we support networking events for businesses?
GEHRKE: Wow. Uh-huh …
O’NEILL: So it was just at the time of the first, you know, things like Webex and things, you know, the first collaborative seminar-y …
GEHRKE: Yeah, so you’re way ahead of the social networks, right, and everything, right?
O’NEILL: Yeah, yeah.
GEHRKE: And there was a whole conference at that point in time, right? CSCW, I think I remember. Wasn’t there …
O’NEILL: Yes, yes, yes.
GEHRKE: So it was and still is, I think, a really big field.
O’NEILL: Yes, it’s a, it’s a, it’s really interesting. And I think one of the things that’s interesting with the foundational models now is many of the things that people like me, HCI people, have been wanting to happen—”Oh, if only we can enable people to interact with technology like this”—are now suddenly possible, which is quite exciting.
GEHRKE: Yeah, so we’ll get to that in a little bit because I think, you know, as you said, the whole field of HCI is now changing with foundational models and what the interfaces are, will be. I think it’s a really interesting, deep research question right now. So, so, OK, so you got your PhD; you’re in Manchester. What’s the next step in your career? Where did you go next?
O’NEILL: Yeah, I actually got a job before I finished my PhD. So I took quite a long time to do my PhD. I think it was seven years in the end, partly because I was teaching. When I was doing—like, lecturing when I was doing my PhD, and I also had a job as a consultant occasionally, working with, I think, I worked with the Co-op Bank. I worked with some usability companies, and you could, I could make enough money to live for a term on, like, two weeks’ consultancy because I didn’t have very high costs. [LAUGHS]
GEHRKE: Right. You lived as a grad student, right?
O’NEILL: Yes. Yeah. Yeah. And, actually, you know, I was living in Manchester. I was living in a squat, so I wasn’t paying any rent, [LAUGHS] so …
GEHRKE: Oh, really?
O’NEILL: Yes. So I didn’t have very many costs.
GEHRKE: OK.
O’NEILL: Which was very handy. So I didn’t have any real incentive to finish my PhD until I got a job, you know. When I finished my master’s, I looked at the job market, and with my computer science master’s, the main job was database manager, [LAUGHS] which didn’t appeal.
GEHRKE: That sounds now really interesting. [LAUGHTER]
O’NEILL: Yeah. So I, actually, that’s why I ended up doing a PhD, because I was like, I don’t want to go back to work yet. You know, I’ve been working for five years before. So, so, yeah, I just was enjoying doing a PhD and doing pieces of work here and there. And then I got a job at Xerox in Cambridge, and then that’s when I got motivated to finish my PhD because working and doing a PhD at the same time is not much fun.
GEHRKE: Right, right. So you got your PhD, had your job lined up, and then you’re starting at Xerox. What were you doing in Xerox?
O’NEILL: Human-computer interaction. Yeah, it was a really exciting time. There was so much going on in the industry. I was so delighted. It was like my dream job to be in industry and to maybe create cool interfaces and, you know, cool collaborative systems. So … and then they closed the lab [LAUGHS] within six months. It wasn’t my fault.
GEHRKE: So quickly?
O’NEILL: Mm-hmm.
GEHRKE: Wow. And what did you do then? I mean, this is your first big job, and …
O’NEILL: Yes …
GEHRKE: … such a quick setback.
O’NEILL: They offered me a job in their lab in France. So I stayed in the UK for a while and worked half in France, half in the UK, and then I shifted to France full time.
GEHRKE: OK. Oh, wow. So do you … where in France did you live then?
O’NEILL: Grenoble.
GEHRKE: OK, yeah. In the middle of …
O’NEILL: In the French Alps.
GEHRKE: … the French Alps. Exactly. Beautiful place.
O’NEILL: Absolutely … yes. Yeah. Skiing, climbing, hiking. So much fun.
GEHRKE: And, OK, so you’re at Xerox PARC in the French Alps. What’s, what’s next?
O’NEILL: They were opening, Xerox was opening a research lab in India. And I’d always wanted to travel. You know, I’d always wanted … and I never really had the money or the opportunity to travel. So when they said they were opening it, I just went to my boss and said, hey, I don’t know what you’d want me to do, but if there’s any opportunities for me to do anything to help …
GEHRKE: Wow.
O’NEILL: … the opening of India, I’d love to. And I went out for a month and then I went out for three months.
GEHRKE: I mean, both of these sound like really bold steps to me. First of all, I mean, Grenoble is probably pure French speaking, right? And, I don’t know, did you have high school French or you were good … [LAUGHS]
O’NEILL: I had high school French, yes, and then we drove, we drove from the UK to Grenoble listening to “learn French” tapes [LAUGHS] …
GEHRKE: OK, wow … [LAUGHS]
O’NEILL: …in the car. Yeah.
GEHRKE: Wow. And that was enough then to get by with a daily …
O’NEILL: Actually, so it was great in France because they expect you to learn the language, so you have French lessons at work. And then, actually, I did an evening class, as well, that was paid for by work, a really intensive one-month, like two hours a night, every night of the week. And that really helped. Yeah, it was, it’s fantastic.
GEHRKE: Wow, that’s really great. And then, and then you took the even bigger step to move to India, right. How was that like, and what was your experience there?
O’NEILL: Yeah, India is just magical. You know, initially, I just went for one month, then three months, and it was just—the people, the culture, the work I was doing, the research I was doing was like no research … you know, I’d spent a lot of time in call centers around Europe doing studies, ethnographic studies, and designing technology. Lots of time looking at photocopiers because I was with Xerox. [LAUGHS] And then so going to India, suddenly, you know, I’m looking at social enterprises. I’m looking at all sorts of businesses and different ways of life and different people. And it was just so rich and so amazing that I was like, OK, I really want to do this. And that’s actually when I applied to Microsoft because Microsoft had the Technology for Emerging Markets group there, which is world-class research in that space. So I was like, OK, if I want to keep on doing this, then that’s what I’m going to apply to. And luckily enough, I got the job, and that’s how I joined Microsoft.
GEHRKE: Wow. So, so, OK, so you’re now at Microsoft in India. That was in Bangalore, right, where our research lab there is?
O’NEILL: Mm-hmm.
GEHRKE: And so what, what were you working on there for the next few years?
O’NEILL: Yeah. So initially, I looked at a few different things. I joined some existing projects. So I was on MEC, which was the educational platform, looking at whether we could bring the power of MOOCs [Massive Open Online Courses] to Indian education to improve the level of education because they have amazing colleges at the top, but, actually, the vast majority of students go to these intermediate colleges, and the teaching level really varies. And so the idea was, can you help with blended learning? Can you help the teachers teach better? That turns out to be really challenging. And, actually, the system ended up being used by the students to teach themselves.
GEHRKE: Oh, like for independent learning?
O’NEILL: Mm-hmm. Mm-hmm. And that was really, so that was interesting, doing some studies there. I looked at … Indrani [Medhi Thies] had done an amazing project where they’d built “Facebook for Farmers.” So I did a study of that, which was really, really fun. And then I worked in financial inclusion, one of my big areas. I spent about five years working with auto-rickshaw drivers in Bangalore, designing technologies to help them understand the loans they’d taken out, which was really, really fun. They’re a very great community to work [with]. You don’t get any nonsense from an auto-rickshaw driver. [LAUGHS]
GEHRKE: Well, I was just thinking, what was it like to, like, live in India and just move there and start out there?
O’NEILL: Uh, it was, I mean, it was fantastic. It’s a great place to live. The people are amazing. The food is amazing. Moving with Microsoft makes it very easy because Microsoft takes care of you when you move so you’re not, you know, some of the stresses that you might have around the move are taken care of. I had a young family. I had a 2-year-old son when we moved out there and within a year had another one, which was not 100 percent planned, because you don’t usually move to a new company and then have a baby. You’re like, oh, sorry. [LAUGHS] But that was all fine. Yeah.
GEHRKE: And, and, you know, you worked with all of these different communities in India, right. How did you connect to the communities? I mean, these were teachers …
O’NEILL: Yeah, you need to, you really need to go with people, so you have to convince some organization that what you’re going to do is going to be beneficial to them and useful for them. And then if they’re trusted by the community, they give you access. And that’s really great because you do have access that you wouldn’t otherwise have. You know, if you’re really wanting to build technologies to support people, you really need to understand what they care about—what do they want help with?—and you only get that if you’ve got a trusted relationship with them. So we worked with, there was one organization that worked with the auto-rickshaw drivers’ wives. It was about empowering women, and we got access to the drivers initially through that organization.
GEHRKE: That’s amazing. I mean, you know, I’ve visited India many times, but I can only imagine how it is to live there, actually. So do you have some of the stories of what is, sort of, most surprising for you given that you’ve lived there?
O’NEILL: Yeah … what’s most surprising? I think, so one thing is, one thing is people want to tell you what they think you want to hear. So if you’re lost, you need to ask quite a few people for directions and then make some sort of assessment about whether the person was just saying “yes, yes, that way” because he knew the way or “yes, yes that way” because he just didn’t want to tell you that he didn’t know. And so you have to, sort of, judge. [LAUGHS] So that’s one, like, useful piece of …
GEHRKE: So the first few times you went in the wrong direction? [LAUGHS]
O’NEILL: Yes, exactly. And then you’re like, “But they said …”; you ask someone else, and they’re like, “No, it’s over there.” And then someone … so that’s … the most useful piece of advice I could give to anyone who’s visiting India, is when you cross the road, just find someone else who’s already crossing the road and cross with them.
GEHRKE: Because it’s so dangerous if you go by yourself potentially?
O’NEILL: Yes, yeah. You get used to it quite quickly, and there’s obviously something that changes in you when you’ve been there a while. You know, when you first go there, all the auto-rickshaw drivers are going to overcharge you and drive around the block twice and all of those things. And I find after about four to five weeks when you’ve been there, they know, like, there must be something that changes in your attitude because they actually know that you’re there longer term and you’re not going to take any nonsense.
GEHRKE: So, so do you behave differently? What’s the change there?
O’NEILL: I don’t know. That’s, I’ve tried to think about this, but I think, I don’t know, it must be just an air of confidence or an air of certainty or something. But, yeah, it’s like something just clicks or changes.
GEHRKE: That’s so interesting. Is it only for the drivers, or is it in other aspects of your life, as well, where, sort of, you get treated differently because you suddenly have become a native?
O’NEILL: I think you notice it most in the drivers because they’re the ones that you’re interacting so much with to get about, you know, to get … you’re always getting a tuk-tuk to go from here to there. And they really do, you know, if they can make extra money out of you, they are going to make extra money out of you.
GEHRKE: They smell it, that you’re a tourist.
O’NEILL: Yeah, yeah, yes. [LAUGHS]
GEHRKE: And then so you were in India and then another opportunity came along. So tell us a little bit about that opportunity, where you ended up now.
O’NEILL: Yes, yes. So when I heard that the ADCs were opening—the Africa Development Center, so our software engineering center in Nairobi and Lagos—I thought that that was a great time to pitch for research in Africa for Microsoft. It seemed like a bit of a hole in our portfolio. I have family connections to Africa. So, actually, one of the reasons for joining Microsoft was partly because I thought there might be opportunities eventually in Africa because we had a great Africa startup program, for example. So, you know, but there wasn’t any research there. And so when I heard the ADCs were open, I just put together a, like, pitch for setting up research in Africa within the ADCs, and, you know, all sorts of people really helped me hone that pitch. And then I flew at the end of February 2020. I flew …
GEHRKE: Oh, just right before the pandemic.
O’NEILL: Mm-hmm. I flew to … I was in Barcelona for a Future of Work event, and then I flew to Nairobi and then Lagos to meet the people who were running the ADCs and to think about where, which one I would want to set up research in if such a thing were to happen. And I did that. I decided that Nairobi was the right one. And when I went there, Jack Ngare ran the ADC, and he was so enthusiastic about having research there. So I did a pitch and got some funding just—I think if it had been two weeks later, I’m not sure. But, you know, it was just before we knew how bad COVID was going to be, so I was very lucky with timing.
GEHRKE: And, I mean, you’ve made these amazing moves throughout your career, right. You, sort of, raised your hand for India when the lab was open; now here in Africa. Why, and how? I’m just, I mean, so curious because people make the most unexpected turns in their careers from time to time. But it’s more like because, you know, they lose their current job or they, their manager moves away and they really think about their career. But you, like, raise your hand from time to time and make these really bold and amazing moves.
O’NEILL: Yeah, I mean, life’s meant to be exciting, isn’t it?
GEHRKE: OK …
O’NEILL: I think. You know, life’s meant to be exciting. I love living in different places and, you know, as an ethnographer, as a person interested in human-computer interaction, it’s, like, those experiences are what help us innovate better and design things that are, like, taking another point of view, more creative, I think. Like, just sparks things in your, in your head. And, I mean, it’s so much fun. Like, I don’t understand why everyone doesn’t do it. [LAUGHS]
GEHRKE: So it’s just really amazing. So if I think about, you know, India, where you said, right, the experience for you was that the drivers were treating you suddenly differently. Did you have a similar experience in Africa, or what is one of the or a few of the defining experiences and stories there?
O’NEILL: Yeah, I think … so the animals are amazing in Kenya. They’ve done such an amazing job at conservation. I imagine that they would, you would only see, like, these big animals in the national parks, but—they’re not everywhere. They’re not going to be, you’re not going to find a hippo walking down the road in Nairobi. But they are all over the place. So you can go camping in Lake Naivasha, which is just an hour and a half from Nairobi, and I was camping with a friend, and the kids were in their tent, and my friend was in her tent, and I was just sitting by the fire. It’s about 10 o’clock. I said, yeah, I might go to bed in a minute. And then I just heard this snort, and I get up with my torch, and I look, and there’s a hippo, [LAUGHS] like, probably less than a meter and a half …
GEHRKE: Wow …
O’NEILL: … away from me. So I carefully went and sat back down by the fire and waited for a while before I moved. [LAUGHS]
GEHRKE: So are they dangerous in that aspect, if you’ve startled them or so … ?
O’NEILL: Yeah, I think … they say that you should never get between a hippo and the water. So, luckily, I was on the other side of the, [LAUGHS] of the hippo and the water. But they are big. I mean, they can be very grumpy.
GEHRKE: And so you should, just, shouldn’t startle them or … ? I’m just trying to understand what’s the recommended behavior. Don’t get between the hippo and the water.
O’NEILL: Yes, that’s recommended, and don’t, yeah, don’t startle them, and just, you know, stay very, stay very calm. So, actually, when you’re camping, if you don’t have an electric fence around the campsite, then you shouldn’t come out of your tent at night. So don’t drink too much beer before you go to bed, [LAUGHTER] because it’s the “zip.” When you unzip it, you can really startle … If there’s any wild animals, lions, or whatever around, then you can really scare them. And you don’t want to scare a lion.
GEHRKE: Yeah, I was thinking, just, actually, about the lions or so, right. I mean, they could be probably even more dangerous than the hippos or, or not really?
O’NEILL: Hippos are actually more dangerous than lions. Yeah, lions will generally not attack you. And apparently, the thing—I haven’t had to try this, I’m glad to say—but the thing you should do if you encounter a lion is just look them in the eye, and then they’ll go off.
GEHRKE: Stare them down.
O’NEILL: Mm-hmm.
GEHRKE: OK.
O’NEILL: I hope I never have to try that because they are quite scary … [LAUGHS]
GEHRKE: I hope I never have to do that but good advice …
O’NEILL: Yes, yeah, yeah. I think hippos are more likely to charge at you. Like, a lion’s more likely to go off in the other direction.
GEHRKE: And what’s the daily life like, you know, living in Nairobi, right? I mean, is it, I mean, it must be very, very different from living in both India, as well as, you know, Great Britain or here.
O’NEILL: Yeah. I mean it is very different. The traffic’s bad but not as crazy as India. Like, I drive in Kenya. I didn’t drive in India because it was a bit too scary with the bikes and everything. It’s a really, it’s a really nice pace, I think, in Nairobi. It’s a beautiful city. There’s nightlife, and there’s cafes and restaurants, but you’ve got countryside so close. You know, compared to Bangalore, it’s quite a small city. And the weather is amazing, and the people are really friendly and kind, and, you know, it’s just, it’s a very nice, it’s a very nice place to live.
GEHRKE: That’s amazing, and you now are leading the Microsoft Africa Research Institute there, right?
O’NEILL: Yes.
GEHRKE: What is the focus of the institute, and what are you studying there?
O’NEILL: Mm-hmm. Yeah, we’re mainly focused on foundational models. It won’t be a surprise to anybody. [LAUGHS] Which actually, you know, it’s worked out very well for us because, you know, we have a mixed disciplinary team. We have HCI and AI and ML and data science.
GEHRKE: And all local?
O’NEILL: All local. Yeah. And, yeah, we’re looking at multilingual languages in models. So we’re working with MSR [Microsoft Research] India, thinking about how can you benchmark these models for different languages. And we’re thinking all the way along the scale from your high-resource, you know, French and German, to your mid-resource Swahili, Hindi, all the way to your low-resource languages because, you know, the vast majority of training data is in English. So we’ve been working a lot. That’s nice because we’re having, you know, in a very short amount of time, you know, four or five months, we’re having both scientific impact with papers but also product impact, working with the Copilot Language Globalization team as they’re rolling out Copilot in different languages.
GEHRKE: I see. So the research that you have will go into, let’s say, Word or PowerPoint or so to make it available in some of the languages from the continent.
O’NEILL: Yes, exactly. Because it’s not just about translation. It’s also if you think about RAI, responsible AI, you know, a lot of that is language based. And so how do … you can’t just translate this to words. You have to find the right list of words in those languages. And then what about things like tone and stuff? So that’s one area. And then related to that, it’s in a much bigger space of equity, the models and equity. You know, what’s going to happen to the digital divide with these models? In some ways, you could imagine that they may be flattening it, but in other ways, they could be increasing it. So we really are trying to map out how … the different elements of the digital divide as it plays out in these models. Because you obviously have your traditional things like access to devices, access to, you know, infrastructure, and things like that. But there’s also the data divide. So not only is most of the training material in English; it’s also mostly from America and the Global North. So it embodies very particular world views. And if you think about data on Africa, data on Africa tends to be collected by particular organizations. So there’s lots of data on poverty and disease and forced migration and things like that. Not much data on, like, the stories, the creativity, wealth, innovation. So what does that mean? Even if the models can speak perfectly, which they can’t yet, but they’ll eventually get quite good at, you know, even smaller languages like Luo, if that model is just translating English content into Luo, that’s not necessarily what we want from a model. So there’s some really interesting questions there to be answered.
GEHRKE: Well, it seems to me like it’s clearly also a question of, like, getting the right kind of data. So where do you get the data, and how do you get the data?
O’NEILL: Yeah, that’s a big question. And it was already a challenge, you know, before these models. You know, many people have been working with Masakhane, which is one of the African NLP communities which is around creating datasets in African languages for training the models. So that was, you know, getting good quality training data is already a challenge. Sriram [Rajamani] from MSR India, though, was telling me of a really interesting project they’ve got going on in India with the Indian government where they are trying to collect data from each region of India so that they can use it to train the OpenAI models, which would be really cool. And we should think about, is that what we can do for different African countries and contexts?
GEHRKE: Exactly. It seems to be very much like a citizen science project, right, where you, sort of, involve the citizens that speak different dialects and then involve them in collecting the right kind of data.
O’NEILL: Yeah, yeah. And maybe collecting the stories, you know, and the cultural attributes and assets from different places.
GEHRKE: That’ll be really, really exciting probably also about preservation of the culture and history, right.
O’NEILL: Yes, yes. But challenging.
GEHRKE: But challenging. [LAUGHTER]
O’NEILL: Yeah.
GEHRKE: So that’s one big aspect of the work. Anything else that’s happening there?
O’NEILL: Yeah. So we’re doing a lot of work, you’ll be unsurprised to hear, on Future of Work and AI. And so we’ve got a project on modern work and LLMs, so looking at the work that enterprise workers, frontline and knowledge workers, are doing and then what bits of their job they would like to get rid of if they could and what bits they would keep and how we can use LLMs to support them. And we’ve also, like, Maxamed [Axmed] on my team, also worked with The Garage to train them up in foundational models, both the LLMs and the vision models, and then they’ve introduced them to a whole load of small businesses in Kenya.
GEHRKE: Oh, wow.
O’NEILL: So that’s really interesting. You got everyone from like car salespeople to lawyers who are now using, like, LLMs as part of their everyday work, which is amazing.
GEHRKE: As part of like composing messages or part of … what’s …
O’NEILL: Yeah. Writing contracts, sales documents for cars, all sorts of really interesting things.
GEHRKE: Oh, wow.
O’NEILL: So we’re going to go out and look at what they’re doing and think about how, you know, what else is needed, what, what more do they need.
GEHRKE: What’s the prevalent form factor in terms of if I think about, like, a computer there? Is it my, is it a mobile phone? Is it a tablet?
O’NEILL: Yeah.
GEHRKE: It’s a mobile phone?
O’NEILL: It’s a mobile phone. Yeah.
GEHRKE: So you have to rethink also, probably, all the interfaces.
O’NEILL: Yes, I mean …
GEHRKE: You mentioned that early on, right, as you think about the next generation of HCI with AI in it, right.
O’NEILL: Yes, yes. I mean conversational interfaces. The idea that you can talk to your phone or enter existing text, you know. If you look at small businesses, a lot of their interactions with customers are on chat. If you can enter that chat into an LLM and extract structured data from it, then suddenly you’ve got all this data that’s been lost to the business becomes usable. So it’s a really exciting space, and I think voice interfaces are going to become really, really, really big. And that’s why there’s opportunities for leapfrogging, because suddenly everyone with a mobile phone potentially has a really powerful office productivity tool in their hand and can do things … you know, many of the small businesses, they don’t employ a designer; they don’t employ an accountant. But now they could maybe have an accountant or a designer in their pocket, which enables them to do more, which is definitely the more positive side of the future of work than some of the …
GEHRKE: Right. You know, this whole enablement story of people is just really amazing, what you can do with LLMs and especially with voice interfaces, as well. Let me conclude maybe with a question about your career. I mean, it seems like you’ve always amazingly managed to somewhat align your career moves with your passion. You moved to India because you’re just excited to live in India. You moved then to, you know, Microsoft Research, but then you moved to Africa again for, what I hear, is a little bit the adventure, as well, right?
O’NEILL: Yes.
GEHRKE: So what’s your advice for people who want to, sort of, align these two and who want to not only work but also want to work on something they’re really passionate about? How do you manage to create that alignment?
O’NEILL: That is a good question. I don’t know. It just, sort of, happens. I mean, I think you have to, you have to be passionate about it; you have to talk about it and decide what you want to do. You know, I never really imagined MARI would happen. But I just started talking to people, and people were saying, before I did the pitch, people were saying to me, oh, what would you like to do in five years, Jacki? And I was like, oh, you know what? If I had my way, I’d love to run a research center in Africa. And then within a couple of years … it was nothing more than an idea in my head. So I think that you have to have the ideas, verbalize it, and maybe it can happen.
GEHRKE: And why a research center in Africa? What’s personal for you there?
O’NEILL: So my children are African; my children are Cameroonian. So I wanted them to grow, spend some time on the continent, and, you know, as a family, we’d always had that idea of moving to the continent eventually. So that was part, that was a personal motivation in there as well as the passion. Yeah.
GEHRKE: So it’s, well, sort of, the confluence of, I guess, opportunity but then also drive on your side? Because that’s what I’ve heard. Very often in careers, that it’s not only about, well, this is what I finally want to do but also watching out for that opportunity.
O’NEILL: Yes.
GEHRKE: So it seems like that played a big role here, as well. And so when you heard about, you know, that there was an Africa Development Center, how did you, what were your next steps then? I mean, you must have been excited, but you also had to take some action.
O’NEILL: Yeah, I mean, I created, [LAUGHS] I created a small pitch, a small set of slides, and then I just started talking to everybody I knew who was doing anything. I didn’t have any contact with the ADCs.
GEHRKE: So you created that energy and excitement about it?
O’NEILL: I just started to, you know, every time anyone would come to India, you know, I was just like, oh, this is what I’d like to do. And you just almost talk it into being, I think.
GEHRKE: And were there some setbacks, or was it just like a straight line from, sort of, the excitement all the way up to realization?
O’NEILL: No, I mean, I didn’t, I don’t think I ever really imagined it would happen, you know. But you’re just doing it, and you’re plugging away, and then taking the, you know, taking the advice of people.
GEHRKE: Really an awesome story. So maybe as a last question, where do you see the center being in like three to five years? I mean, you’re starting off right now, but I’m sure you have really big ambitions for the center, and there’s so much to do on the whole continent.
O’NEILL: No, absolutely. I think that I have a few ambitions. So the most important, I think, I want it to be really established as this thing that’s really beneficial to Microsoft, that Microsoft is like, really, “Yeah, the guys at MARI, they’re doing great research. We really like them.” So that it, sort of, exists without me, you know. At the moment, I think I’m the driver of it. I would …
GEHRKE: So you want to grow the next generation that is basically going to be the next generation of leaders?
O’NEILL: Yes, exactly, exactly. And then I think also grow, I would love to help in growing Microsoft’s market in Africa. We don’t have a particularly big market in Africa, but I think there’s a lot of opportunity, especially now with these, with these large language models. I think that we … so that would be really exciting, you know, if we can help. I don’t see our success only being about growing the African market, but I think it’s part of what we can do, and if we can grow that market, as well as do research that’s relevant for Redmond and relevant globally, that’s really, that’s really exciting, I think, you know. So everything we do, I think, has to have a relevance globally. And I think, you know, at the beginning I was talking about different ways of viewing the world and how that leads to innovation. I think by having researchers who are African, based in Africa, doing this great research, we can create better products for everyone.
GEHRKE: That’s such a great finishing note. Thank you so much for the great conversation, Jacki.
O’NEILL: Thank you, Johannes. It’s been fun.
[MUSIC]To learn more about Jacki or to see photos of Jacki living and working abroad, visit aka.ms/ResearcherStories (opens in new tab).
[MUSIC FADES]
The post What’s Your Story: Jacki O’Neill appeared first on Microsoft Research.
NVIDIA, Teradyne and Siemens Gather in the ‘City of Robotics’ to Discuss Autonomous Machines and AI
Senior executives from NVIDIA, Siemens and Teradyne Robotics gathered this week in Odense, Denmark, to mark the launch of Teradyne’s new headquarters and discuss the massive advances coming to the robotics industry.
One of Denmark’s oldest cities and known as the city of robotics, Odense is home to over 160 robotics companies with 3,700 employees and contributes profoundly to the industry’s progress.
Teradyne Robotics’ new hub there, which includes cobot company Universal Robots (UR) and autonomous mobile robot (AMR) company MiR, is set to help employees maximize collaborative efforts, foster innovation and provide an environment to revolutionize advanced robotics and autonomous machines.
The grand opening showcased the latest AI robotic applications and featured a panel discussion on the future of advanced robotics. Speakers included Ujjwal Kumar, group president at Teradyne Robotics; Rainer Brehm, CEO of Siemens Factory Automation; and Deepu Talla, vice president of robotics and edge computing at NVIDIA.
“The advent of generative AI coupled with simulation and digital twins technology is at a tipping point right now, and that combination is going to change the trajectory of robotics,” commented Talla.
The Power of Partnerships
The discussion comes as the global robotics market continues to grow rapidly. The cobots market in Europe was valued at $286 million in 2022 and is projected to reach $6.7 billion by 2032, at a yearly growth rate of more than 37%.
Panelists discussed why teaming up is key to innovation for any company — whether a startup or an enterprise — and how physical AI is being used across businesses and workplaces, stressing the game-changing impact of advanced robotics.
The alliance between NVIDIA and Teradyne Robotics, which includes an AI-based intra-logistics solution alongside Siemens, showcases the strength of collaboration across the ecosystem. NVIDIA’s prominent role as a physical AI hardware provider is boosting the cobot and AMR sectors with accelerated computing, while its collaboration with Siemens is transforming industrial automation.
“NVIDIA provides all the core AI capabilities that get integrated into the hundreds and thousands of companies building robotic platforms and robots, so our approach is 100% collaboration,” Talla said.
“What excites me most about AI and robots is that collaboration is at the core of solving our customers’ problems,” Kumar added. “No one company has all the technologies needed to address these problems, so we must work together to understand and solve them at a very fast pace.”
Accelerating Innovation With AI
AI has already made huge strides across industries and plays an important role in enhancing advanced robotics. Leveraging machine learning, computer vision and natural language processing, AI gives robots the cognitive capability to understand, learn and make decisions.
“For humans, we have our senses, but it’s not that easy for a robot, so you have to build these AI capabilities for autonomous navigation,” Talla said. “NVIDIA’s Isaac platform is enabling increased autonomy in robotics with rapid advancements in simulation, generative AI, foundation models and optimized edge computing.”
NVIDIA is working closely with the UR team to infuse AI into UR’s robotics software technology. In the case of autonomous mobile robots that move things from point A to B to C, it’s all about operating in unstructured environments and navigating autonomously.
Brehm emphasized the need to scale AI by industrializing it, allowing for automated deployment, inference and monitoring of models. He spoke about empowering customers to utilize AI effortlessly, even without AI expertise. “We want to enhance automation for more skill-based automation systems in the future,” he said.
As a leading robotics company with one of the largest installed bases of collaborative and AMRs, Teradyne has identified a long list of industry problems and is working closely with NVIDIA to solve them.
“I use the term ‘physical AI’ as opposed to ‘digital AI’ because we are taking AI to a whole new level by applying it in the physical world,” said Kumar. “We see it helping our customers in three ways: adding new capabilities to our robots, making our robots smarter with advanced path planning and navigation, and further enhancing the safety and reliability of our collaborative robots.”
The Impact of Real-World Robotics
Autonomous machines, or AI robots, are already making a noticeable difference in the real world, from industries to our daily lives. Industries such as manufacturing are using advanced robotics to enhance efficiency, accuracy and productivity.
Companies want to produce goods close to where they are consumed, with sustainability being a key driver. But this often means setting up shop in high-cost countries. The challenge is twofold: producing at competitive prices and dealing with shrinking, aging workforces that are less available for factory jobs.
“The problem for large manufacturers is the same as what small and medium manufacturers have always faced: variability,” Kumar said. “High-volume industrial robots don’t suit applications requiring continuous design tweaks. Collaborative robots combined with AI offer solutions to the pain points that small and medium customers have lived with for years, and to the new challenges now faced by large manufacturers.”
Automation isn’t just about making things faster; it’s also about making the most of the workforce. In manufacturing, automation aids smoother processes, ramps up safety, saves time and relieves pressure on employees.
“Automation is crucial and, to get there, AI is a game-changer for solving problems,” Brehm said.
AI and computing technologies are set to redefine the robotics landscape, transforming robots from mere tools to intelligent partners capable of autonomy and adaptability across industries.
Feature image by Steffen Stamp. Left to right: Fleur Nielsen, head of communications at Universal Robots; Deepu Talla, head of robotics at NVIDIA; Rainer Brehm, CEO of Siemens Factory Automation; and Ujjwal Kumar, president of Teradyne Robotics.
100 things we announced at I/O 2024
Learn more about (almost) everything announced at Google I/O 2024.Read More